DOI:
10.1039/D4SD00248B
(Paper)
Sens. Diagn., 2024,
3, 1947-1956
Detection of SARS-CoV-2 and noroviruses in cold-chain food samples using aptamer-functionalized graphene field-effect transistors†
Received
4th July 2024
, Accepted 4th October 2024
First published on 8th October 2024
Abstract
Given the susceptibility of Severe Acute Respiratory Syndrome Coronavirus (SARS-CoV-2) and Norovirus (NoV) to survive in cold chain food, thereby posing significant public health risks, we present a novel approach for biosensor development utilizing a graphene field-effect transistor (GFET) modified with nucleic acid aptamers. The biosensor's innovative design incorporates 1-pyrenebutyric acid N-hydroxysuccinimide ester (PBASE) as a coupling agent to facilitate the attachment of nucleic acid aptamers onto channel graphene. This modification induces a redistribution of charge on the graphene surface, resulting in a shift of the Dirac point upon target capture by the nucleic acid aptamer. Through this pioneering methodology, we successfully engineered SARS-CoV-2 GFET and NoV GFET biosensors capable of detecting trace amounts of SARS-CoV-2 and norovirus within a rapid 5-minute timeframe, showcasing detection limits of 33 fg mL−1 and 6.17 pg mL−1, respectively. Subsequently, we applied these sensors to detect SARS-CoV-2 in frozen meat and norovirus in shellfish, yielding promising results with excellent specificity and stability. This groundbreaking sensing mechanism holds significant promise for the detection of foodborne viruses across a diverse range of food samples.
1. Introduction
Foodborne viruses are pathogens transmitted through contaminated food and water, leading to human illness.1 These viruses are highly contagious and can cause widespread outbreaks in communal settings, resulting in significant public health crises. While microbial contamination of food has long been a concern, foodborne viruses pose an equally serious threat.2 The global crisis triggered by the Severe Acute Respiratory Syndrome Coronavirus (SARS-CoV-2) in 2019, known as Coronavirus Disease 2019 (COVID-19), has underscored the severity of viral outbreaks.3 Although SARS-CoV-2 in cold-chain foods is not strictly classified as a foodborne virus,4–6 its detection in such foods in various regions poses a significant risk to public health and local economies.7 Furthermore, Norovirus (NoV) can cause acute enteritis through fecal-oral transmission, leading to systemic symptoms like fever, headache, and muscle pain in individuals who consume NoV-infected shellfish.8,9 The development of rapid and accurate detection methods for SARS-CoV-2 and norovirus in food is crucial for precise virus prevention and control efforts.
Currently, the methods used for the detection of new coronaviruses and noroviruses are mainly divided into two categories: those based on viral antigen detection, such as lateral flow immunoassay,10,11 nano-plasma analysis12,13 and enzyme-linked immunosorbent assay,14,15etc. Although these methods can provide a relatively rapid detection of viruses, they cannot realize the accurate detection of trace viruses in food due to their low detection sensitivity. Another category is based on molecular diagnostic tests, such as real-time reverse transcriptase polymerase chain reaction (RT-PCR) technology, which is used as the gold standard for the diagnosis of SARS-COV-2 and norovirus,16–18 and the recently developed CRISPR-Cas technology,19etc. However, these methods are demanding, cumbersome, and require specialized laboratories. Meanwhile, the complicated sample pre-treatment process leads to a long assay time, which cannot meet the demand for rapid, continuous and on-site assays. Therefore, the development of biosensor platforms capable of performing highly sensitive, rapid and accurate detection of SARS-CoV-2 and norovirus is crucial.
In recent years, electrochemical detection has attracted much attention due to its advantages of low cost, short time, high sensitivity, simplicity and high precision. Electrochemical analysis20 is a means of analyzing samples based on their electrochemical properties, and a typical electrochemical analysis system consists of three core components: an electrochemical sensor, an electrochemical detector, and an electrolyte. Gate-modified bioprobes such as nucleic acid aptamers or antibodies have been developed to detect changes in electrochemical signals on the gate surface by methods such as cyclic voltammetry (CV), square wave voltammetry (SWV), and differential pulse voltammetry (DPV), thus enabling the detection of SARS-COV-2 and norovirus.21–23 Besides, field-effect transistor-based electrochemical sensors have shown great potential for development in fields such as biochemistry. Among them, graphene field effect transistors (GFETs) are high-performance field effect transistor sensors prepared with graphene as semiconductor material.24 Due to its excellent carrier mobility and tunable electronic properties, graphene is very sensitive to any charged molecules near its surface, and has become a research hotspot due to its excellent electrical conductivity. The high sensitivity of GFETs stems from the charge transfer between it and the substrate, which leads to the change of the energy of the Fermi surface, and thus affects its electrical conductivity.25
Nucleic acid aptamers are short single-stranded nucleic acid molecules that serve as molecular recognition elements capable of selectively binding to targets such as proteins, peptides, small molecules, and metal ions.26,27 In comparison to antibodies, nucleic acid aptamers are chemically synthesized, cost-effective, and easily controlled. They exhibit excellent stability in various ionic environments, pH levels, temperatures, and storage conditions, making them widely utilized in food analysis.28 The conventional antigen-based immunoassay method faces challenges like antigen inactivation and nonspecific interactions with viral components, resulting in inaccurate detection outcomes. Therefore, leveraging nucleic acid aptamer-modified graphene field-effect transistors (GFETs) holds promise for developing cost-effective and stable biosensors with superior performance. In this work, we used the SARS-CoV-2 spike-in protein-binding aptamer sequence identified as ‘CoV2-RBD-1C’ from the study of Yanling Song et al.;29 the norovirus aptamer sequence used was identified as ‘NV-APTL-1’, from a study by Dapeng Wang et al.30 This study proposes the integration of nucleic acid aptamers with the GFET sensing platform to create high-performance biosensors for the rapid detection of SARS-CoV-2 and norovirus in food (as shown in Fig. 1).
 |
| Fig. 1 Schematic diagram of Aptamer-GFET sensor. | |
2. Experimental section
2.1. Materials
The aptamer sequence against SARS-CoV-2 spiking protein: 5′-CAGCA-CCGAC-CTTGT-GCTTT-GGGAG-TGCTG-GTCCA-AGGGC-GTTAA-TGGAC-A-3′, was synthesized by Sangon Biotech (Shanghai, China) was synthesized. The aptamer sequence of recombinant antigen against norovirus: CGATC-AAACG-TTCAA-GCGGG-GCCCG-GAGGC-GTGAC-TTGGA-CAGGC-AGGCG-TTACG-ATGCA-TCCCG-CAAAT-GACGC-ATGA, synthesized by Sangon Biotech (Shanghai, China) was synthesized. Reagents including polydimethylsiloxane (PDMS), PBS buffer (pH 7.2–7.4, containing 2 mM MgCl2), isopropanol, ethanolamine, and acetone were purchased from Aladdin (Shanghai, China). SARS-CoV-2 spiking protein and interfering substances were purchased from Sangon Biotech (Shanghai, China). Graphene was purchased from Hefei Microcrystalline Materials. Recombinant norovirus-like particles (VLP), recombinant rotavirus, recombinant astrovirus-like particles and recombinant Vibrio cholerae were purchased from ONECLONE Biotech, Inc. (Xiamen, China).
2.2. Fabrication of GFET biosensors
A glass sheet with a thickness of 1 mm, a length of 1.5 cm, and a width of 1 cm was used as the substrate for graphene field effect transistors. The substrates were ultrasonically cleaned with isopropanol, anhydrous ethanol, and deionized water for 2 times for 15 min each, and then dried with high-purity nitrogen and on a heated platform at 120 °C for 15 min to ensure that the surface of the substrate was clean and free of water stains. Next, the glass substrate was combined with a customized mask plate using high-temperature tape, and the Cr/Au (10 nm/90 nm) electrodes with patterning were prepared by coating the surface of the glass substrate using magnetron sputtering. Graphene was transferred onto the channel between the source and drain using wet transfer and dried on a hot bench at 100 °C for 20 min to make the graphene fit tightly on the electrode surface, followed by immersion in acetone at 55 °C for 30 min. The device as a whole was annealed at 300 °C for 2 h. Nitrogen was passed through the annealing process to prevent the graphene from being oxidized to remove the polymethylmethacrylate (PMMA) from the graphene surface. Finally, the graphene channels were isolated with a polydimethylsiloxane (PDMS) layer to form an assay chamber with a volume of 200 μL. The sensor consists of a source (source, S), a drain (drain, D), and an external silver chloride reference electrode (gate, G). The dimensions of the graphene channel were 3 mm in length and 0.2 mm in width.
2.3. Functionalization of GFET biosensors
Functionalization of channel graphene by π–π stacking of the pyrene portion of 1-pyrenebutyric acid N-hydroxysuccinimide ester (PBASE), a highly efficient interfacial coupling agent, was used as a probe connector.31 The esterified end of PBASE can then react with the terminal amine in the nucleic acid aptamer molecule to immobilize the 5′-NH2-modified nucleic acid probe to form a stable amide bond, thus modifying the nucleic acid aptamer to the channel graphene surface. PBASE was dissolved in N,N-dimethylformamide (DMF) at a concentration of 5 mM. The GFET sensors were rinsed three times with 1× PBS before all modifications and tests. Buffer three times to ensure a clean surface. 100 μL of 5 mM PBASE solution was placed on the channel graphene surface for 2 h, and then unbound PBASE was rinsed with DMF and 1× PBS buffer. Nucleic acid aptamers at a concentration of 2 μM were placed on the channel graphene surface, and after overnight incubation, the electrodes were rinsed thoroughly with 1× PBS buffer three times. A further 30 min of occlusion with 10 mM ethanolamine solution was used to deactivate and block unbound carboxylic acid reactive groups on the graphene surface, improving the specificity of the sensor by avoiding interference with detection by interfering agents.32
2.4. Electrochemical measurements
Prior to each measurement, 200 μL of ultrapure water was injected into the solution cell of the GFET sensor for 20 minutes to eliminate the interference of dust on the graphene channel surface. The ultrapure water was then aspirated and 200 μL of 0.1× PBS buffer (pH 7.2–7.4, containing 2 mM MgCl2) was injected into the solution cell to start electrochemical measurements. The electrochemical measurements were performed by connecting the GFET device to two Keithley 2400 digital source meters and transfer characteristic curves were performed by controlling the gate voltage (VG) and source-drain voltage (VDS) through LabVIEW software. The transfer characteristic curves and leakage currents (Fig. S1†) were measured by scanning the VGS over the range of −0.2 to 0.6 V at a rate of 0.01 V s−1 with a fixed VDS of 0.05 V. The signal noise was measured at room temperature. Signal noise was measured by aspirating 200 μL of 0.1× PBS buffer into the GFET sensor that had been modified with nucleic acid aptamer and closed with ethanolamine under room temperature and pressure, scanning the transfer characteristic curve using a digital source meter, reading the gate voltage data (VDirac) corresponding to the Dirac point of the transfer characteristic curve, and scanning three times successively to obtain the Dirac point displacement value.
3. Results and discussion
3.1. Characterization of GFET biosensors
Fig. 2a illustrates the scanning electron microscopy (SEM) images in graphene transferred onto a glass substrate, showcasing a pristine graphene layer with a smooth surface. This indicates successful removal of impurities on the substrate and polymethylmethacrylate (PMMA) residues from the graphene surface, thereby eliminating potential influences on the electronic properties of graphene.33 The surface morphology of channel graphene (Fig. 2b) and the 1-pyrenebutyric acid N-hydroxysuccinimide ester (PBASE)-modified surface (Fig. 2c) was characterized using atomic force microscopy (AFM), revealing a root-mean-square (RMS) roughness of 1.18 nm for bare graphene and 1.70 nm after PBASE derivatization, demonstrating an improvement in surface roughness post-derivatization with PBASE. Fig. 2d depicts the interaction of the pyrene group in PBASE with graphene through π–π interactions, leading to D and D′ peaks and an enhancement of G peaks, indicating the impact of PBASE functionalization on the symmetry and cleanliness of graphene.34 The transfer characteristic curves of the graphene field-effect transistors (GFETs) before and after functionalization were measured in 0.1× PBS buffer solution using a digital source meter (Keithley 2400) (Fig. 2e). PBASE functionalization induced a notable positive shift in the VDirac (gate voltage VG at minimum drain-source current IDS), attributed to the p-doping effect of pyrene groups. Subsequent nucleic acid aptamer modification caused a negative shift in VDirac, confirming successful aptamer attachment to the GFET. These characterization results collectively validate the successful modification of the nucleic acid aptamer onto the GFET. Fig. 2f displays the IDS–VDS output curves of the GFET as VG varies from 0 to −1 V in steps of −0.2 V. The GFET exhibits a negative shift in the IDS–VDS output curve, with increasing IDS values as VG increases, consistent with expectations for p-type semiconductors.35 Additionally, the linear IDS–VDS curve demonstrates good ohmic characteristics of the tested device, indicating the GFET sensor's capability to provide reliable detection signals.
 |
| Fig. 2 (a) SEM photograph of graphene on glass substrate. Atomic force microscopy (AFM) characterization of clean graphene (b) and functionalized PBASE graphene (c). (d) Raman spectra of graphene before and after functionalized PBASE. (e) Transfer characteristic curves during GFET sensor modification, the transfer characteristic curves were measured by scanning the VG in the range of −0.2–0.6 V at a rate of 0.01 V s−1 with a fixed VDS of 0.05 V. The transfer characteristic curves were measured by scanning the VG in the range of −0.2–0.6 V at a fixed VDS of 0.05 V. (f) IDS–VDS output curve of the GFET sensor for modified nucleic acid aptamers with VG ranging from 0 V to −1 V in steps of −0.2 V and drain-source voltage (VDS) from 0 to 100 mV in incremental steps of 10 mV. | |
3.2. Sensing mechanism of GFET biosensors
To explore the performance mechanism of graphene field-effect transistor (GFET) biosensors, we present the Dirac point offset equation based on the electric double layer (EDL) model:36–38
, where Δq is the amount of charge change on the graphene channel surface after the capture of the target by the nucleic acid aptamer on the graphene surface, C the total capacitance of the gate, Δn the amount of change in the carrier density of the graphene, e is the electron charge, μ the carrier mobility in the graphene, and N the number of target molecules bound on the graphene surface. This equation reveals a positive correlation between ΔVDirac, graphene carrier mobility, and the quantity of target molecules attached to the graphene surface. In 0.1× PBS buffer environment, both the SARS-CoV-2 spike protein and norovirus-like particles exhibit negative charges. When the nucleic acid aptamer captures the target, the negatively charged protein is concentrated on the graphene surface. Furthermore, the negative charge of the phosphate backbone of the nucleic acid aptamer causes conformational changes upon target capture,21 resulting in alterations to the carrier mobility of graphene and reorganization of the graphene surface charge. These changes lead to a shift in the Dirac point.39
3.3. Detection of SARS-CoV-2 and norovirus
To assess the efficacy of the SARS-CoV-2 graphene field-effect transistor (GFET) sensor, we introduced diluted SARS-CoV-2 spike proteins into the sensor using 0.1× PBS buffer at concentrations ranging from 1 pg mL−1 to 100 ng mL−1. The outcomes are depicted in Fig. 3b, where upon adding 1 pg mL−1 of SARS-CoV-2 spike proteins to the sensor for 5 minutes (Fig. S2†), the measured transfer characteristic curve shifted to the left. This shift persisted and intensified with increasing concentrations of SARS-CoV-2 spike protein (Fig. S3†). The Dirac shift (ΔVDirac) is calculated as the disparity between the Dirac points of the transfer curves before and after sample addition: ΔVDirac = VD0 − VD1, where VD0 represents the Dirac point before sample addition, and VD1 the Dirac point after sample addition for 5 minutes. A linear regression analysis was conducted correlating spiked concentration with Dirac point offset (ΔVDirac) (Fig. 3c), yielding a correlation coefficient of R2 = 0.9844. The linear detection range spanned from 1 pg mL−1 to 100 ng mL−1, with a corresponding Dirac point offset (ΔVDirac) of 20.14 mV at a SARS-CoV-2 spike protein concentration of 1 pg mL−1. Prior to the assay, the SARS-CoV-2 GFET underwent three transfer characteristic curve scans to assess sensor noise, revealing a noise level of only 3 mV, as illustrated in Fig. 3a. The limit of detection (LOD) was obtained by tripling (3σ) the response noise level of the blank device.40 The SARS-CoV-2 GFET blank noise level was 3 mV, and taking its triple value of 9 mV and substituting it into the linear fitting equation, the limit of detection was deduced to be 33 fg ml−1. Because of the high sensitivity of the electrochemical device in the detection process as well as the self-amplification characteristic of the signal of the field effect transistor, we can recognize signal changes at the aptamer's signal change is recognized at the instant the target is captured by the aptamer, so we fabricated the SARS-CoV-2 GFET with lower LOD.
 |
| Fig. 3 (a) Noise signal analysis of SARS-CoV-2 GFET. (b) Transfer characteristic curves of SARS-CoV-2 GFET at various concentrations of SARS-CoV-2 (diluted with 0.1× PBS); (c) the linear relationship between SARS-CoV-2 concentration and ΔVDirac. (d) Investigation of substrate effects by introducing tap water to the SARS-CoV-2 GFET. (e) Transfer characteristic curves of SARS-CoV-2 GFET at different concentrations of SARS-CoV-2 (diluted using tap water); (f) the linear correlation between SARS-CoV-2 concentration and ΔVDirac. (g) Analysis of noise signal for norovirus GFET; (h) transfer characteristic curves of norovirus GFET at varying concentrations of norovirus-like particles; (i) the linear correlation between the concentration of norovirus-like particles and ΔVDirac (n = 3). | |
To evaluate the sensor's suitability for cold-chain food applications, we selected ice from the surface of frozen meat in the cold-chain food supply as the target for detection. SARS-CoV-2 spike protein was spiked into tap water for testing. A volume of 20 μL of tap water containing SARS-CoV-2 spike protein was added to 180 μL of the sensor's 0.1× PBS buffer system, ensuring a 10-fold dilution for detection and a low detection limit of 1 pg mL−1. Initially, 20 μL of tap water was introduced to the SARS-CoV-2 GFET biosensor to investigate any potential impact of the tap water matrix on sensor performance. As depicted in Fig. 3e, the sensor's Dirac point shifted 5 minutes after the tap water addition, indicating that the tap water matrix did not interfere with SARS-CoV-2 spike protein detection. Subsequent addition of tap water spiked with varying concentrations of SARS-CoV-2 spike protein resulted in a continuous leftward shift of the transfer curve (Fig. 3e) and linear fitting based on spiked concentration and Dirac point shift (ΔVDirac) (Fig. 3f). This analysis yielded a correlation coefficient of R2 = 0.9908, highlighting the linear detection range of SARS-CoV-2 spike protein in tap water by the SARS-CoV-2 GFET sensor spanning from 1 pg mL−1 to 10 ng mL−1. At a spiked concentration of 1 pg mL−1 in tap water, the sensor's Dirac point offset (ΔVDirac) reached 9.04 mV. The theory of Debye shielding elucidates the shielding effect of counter charges around ions due to electrostatic interactions in an electrolyte solution, with the Debye length typically defined as the farthest effective distance.41 The Debye length is defined by the inverse square root of the ionic strength, which is typically 2.3 nm for 0.1× PBS,42,43 aligning with the range of the nucleic acid aptamer on the graphene surface.42 Thus, the addition of a small amount of tap water does not compromise the sensor's detection performance. Additionally, S. Wang et al.44 demonstrated that the Dirac point displacement remains unaffected by buffer concentration variations at low PBS buffer concentrations. Consequently, although the addition of tap water to the sensor's 0.1× PBS buffer slightly alters the ion concentration in the detection system, this adjustment does not impede the sensor's detection capabilities.
To assess the performance of the norovirus graphene field-effect transistor (GFET) sensor, we introduced diluted recombinant norovirus-like particles (VLP) spiked with 0.1× PBS buffer into the sensor individually. In Fig. 3h, the shifts in the transfer characteristic curves are displayed after 5 minutes of adding various concentrations of norovirus, and a linear regression analysis was conducted based on the spiked concentration and Dirac point offset (ΔVDirac) (Fig. 3i). This analysis yielded a high correlation coefficient of R2 = 0.9997, establishing a linear detection range between 10 pg mL−1 and 100 ng mL−1. At a norovirus concentration of 1 pg mL−1, the Dirac point offset (ΔVDirac) reached 9.85 mV. Additionally, we conducted three transfer characteristic curve scans of the norovirus GFET sensor to evaluate sensor noise, as illustrated in Fig. 3g, revealing a noise level of only 3 mV. The norovirus GFET blank noise level was 3 mV, and its triple value of 9mv was taken and substituted into the linear fitting formula to derive a detection limit of 6.17 pg mL−1.
3.4. Specificity of GFET biosensors
To evaluate the specificity of the SARS-CoV-2 GFET and norovirus GFET sensors, we employed interferents relevant to the assay targets as well as meat-based food samples for the measurements. The interferents used in the study can be categorized into two main groups: common foodborne viruses related to SARS-CoV-2 and noroviruses, including astroviruses, circoviruses, and Vibrio cholerae; and typical components found in meat foods, such as bovine serum proteins, myoglobin, hemoglobin, collagen, and lipopolysaccharides. In Fig. 4a, it is evident that the ΔVDirac of the SARS-CoV-2 GFET significantly increased upon the addition of 1 ng mL−1 SARS-CoV-2 spiking protein compared to the other interferences, despite the interferences being present at concentrations 100 and 1000 times higher than the target. Fig. 4b illustrates the ΔVDirac measurements when norovirus-like particles and interfering substances were introduced to the norovirus GFET. The results show that the norovirus GFET effectively distinguished between the norovirus and other interfering substances, despite the significantly higher concentration of the interfering substances compared to the norovirus-like particles. This discrimination ability is attributed to the nucleic acid aptamer's specific binding to the SARS-CoV-2 spiking protein and norovirus, which demonstrates excellent anti-interference capabilities against other substances. Additionally, the ethanolamine confinement treatment applied to the SARS-CoV-2 GFET and norovirus GFET biosensors effectively prevented interference from affecting the graphene's electronic properties. These findings highlight the superior anti-interference abilities and stability of the SARS-CoV-2 GFET and norovirus GFET in the absence of target detection substances. Overall, these results validate the potential of our developed GFET biosensors for detecting target viruses in food samples.
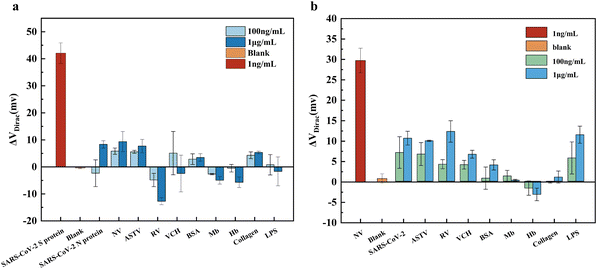 |
| Fig. 4 (a) ΔVDirac response of SARS-CoV-2 GFET biosensor to SARS-CoV-2 spike protein in the presence of other interfering substances. (b) ΔVDirac response of norovirus GFET biosensor to norovirus-like particles in the presence of other interfering substances. Error bars represent the standard deviation calculated from a minimum of three independent measurements (n = 3). | |
3.5. Detection using GFET biosensors in cold-chain food samples
To evaluate the functionality of the SARS-CoV-2 GFET and norovirus GFET biosensors we developed for real-world applications, we conducted testing using selected samples to replicate the presence of SARS-CoV-2 and norovirus. Given that viruses have been found to survive longer at lower temperatures typical of cold-chain food transportation compared to ambient temperatures, instances of positive SARS-CoV-2 tests in cold-chain foods were frequently reported during the SARS-CoV-2 pandemic. Based on this, we chose beef, pork, shrimp and salmon in the cold chain food as the test object, and froze the meat mixed with tap water at 1
:
1 mass, removed the ice melt water on the meat surface, and labeled the target markers for testing. The outcomes of our testing are detailed in Fig. 5a (p < 0.05).
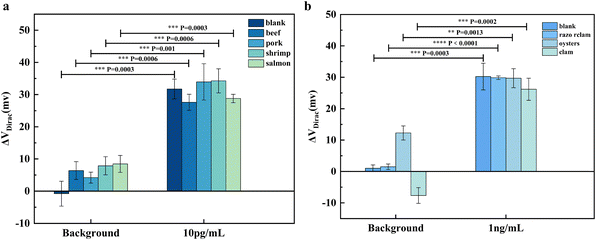 |
| Fig. 5 (a) SARS-CoV-2 GFET for SARS-CoV-2 detection on frozen meat surface ice by standard addition method. (b) Norovirus GFET for norovirus detection on shellfish digestive glands by standard addition method (n = 3). P values were generated by two-tailed Student's t-test: ****P < 0.0001, ***P < 0.001, **P < 0.01, *P < 0.05. | |
When the tap water, obtained after melting the ice on the frozen items' surfaces, was introduced to the SARS-CoV-2 GFET, a slight shift in the Dirac point was observed. This shift was attributed to the presence of lipids and proteins from the frozen items in the tap water, causing interference with the sensor. Nevertheless, the sensor successfully detected SARS-CoV-2 following the addition of SARS-CoV-2 spiking proteins, and the results obtained from the actual samples aligned with those from the buffer control, demonstrating the sensor's ability to accurately identify SARS-CoV-2.
Filter-feeding shellfish such as razor clams, oysters, and clams derive nutrients by filtering water,45 a biological behavior that renders them highly vulnerable to microorganisms and viruses present in the water, with norovirus infection being a common concern. Consequently, we selected razor clams, oysters, and clams as our test specimens. To prepare for testing, their digestive glands were homogenized by the addition of 0.1× PBS buffer, followed by centrifugation of the supernatant for the spiked dilution test after thorough mixing. The outcomes of the detection process are illustrated in Fig. 5b.
Given that oysters are abundant in protein and fat content, their blank samples posed some interference to the sensor; however, the norovirus GFET successfully and accurately detected norovirus during the final detection phase (p < 0.05). These findings suggest that our developed SARS-CoV-2 GFET and norovirus GFET biosensors exhibit efficacy for the swift detection of SARS-CoV-2 in cold-chain food and norovirus in shellfish. The sensors have demonstrated promising detection capabilities in practical applications.
3.6. Stability of GFET biosensors
To evaluate the stability of the SARS-CoV-2 GFET versus norovirus GFET biosensors for detection of target viruses, we used one SARS-CoV-2 GFET biosensor versus one norovirus GFET biosensor for seven consecutive days for SARS-CoV-2 and norovirus, respectively. After the completion of each assay, the assay sample in the sensor was rinsed out using 1× PBS buffer, and then the device was immersed in 1× PBS buffer for 5 min, after which the sensor was rinsed again with 1× PBS buffer, and finally, 1× PBS buffer was added and stored at room temperature for the next use. As depicted in Fig. 6a, the SARS-CoV-2 GFET biosensor successfully detected SARS-CoV-2 spiking proteins at concentrations of 10 and 100 pg mL−1 over a continuous 7-day period. The biosensor consistently exhibited the same ΔVDirac response for identical concentrations of SARS-CoV-2 spiking proteins throughout the 7-day duration. Furthermore, upon an increase in the spiking protein concentration, ΔVDirac exhibited a proportional increment, indicating the sensor's sustained capability to detect and maintain a reliable concentration-dependent response to the spiking protein over the 7-day period. In Fig. 6b, the norovirus GFET biosensor demonstrated the detection of norovirus-like particles at concentrations of 100 pg mL−1 and 1 ng mL−1 over 7 consecutive days. While the detection performance of this sensor exhibited a slight decline on days 5 and 6, potentially attributed to the shedding of nucleic acid aptamer on the graphene channel's surface, the detection results remained consistent and reliable within the 7-day timeframe.
 |
| Fig. 6 (a) Stability of SARS-CoV-2 GFET biosensor to detect SARS-CoV-2 for 7 consecutive days. (b) Stability of Norovirus GFET biosensor for detecting Norovirus for 7 consecutive days (n = 3). | |
In comparison to antibodies, nucleic acid aptamers exhibit prolonged stability when stored at room temperature, with their activity and performance maintained by storing the prepared biosensor in PBS buffer. Furthermore, the biosensor's treatment with ethanolamine closure effectively shielded the inactivated sites on the graphene, thereby slowing down the shedding of the nucleic acid aptamer due to electrostatic adsorption during storage. These findings validate the robust stability of the SARS-CoV-2 GFET and norovirus GFET biosensors developed by our team under standard storage conditions, ensuring dependable results for the detection of SARS-CoV-2 and norovirus. The exceptional stability of the SARS-CoV-2 GFET and norovirus GFET biosensors holds significant implications for their utilization in food testing applications. Their sustained stability ensures reliable and consistent results for high-throughput detection, enhancing the efficacy of food testing processes.
4. Conclusion
In this study, we engineered the SARS-CoV-2 GFET biosensor and the norovirus GFET biosensor, evaluating their efficacy for ultra-sensitive and swift detection of SARS-CoV-2 and norovirus. Our sensors have higher detection sensitivity and can detect SARS-CoV-2 and norovirus in food in a short period of time compared to sensors in the same field that have been developed so far (Tables S1 and S2†). The SARS-CoV-2 GFET biosensor demonstrated an impressive detection limit of 33 fg mL−1, with a linear detection range spanning from 1 pg mL−1 to 100 ng mL−1, encompassing a dynamic range of six orders of magnitude. Notably, this biosensor exhibited a rapid detection time of just 5 minutes, alongside exceptional specificity and stability for SARS-CoV-2 detection. Furthermore, we developed a norovirus GFET biosensor utilizing channel graphene-modified nucleic acid aptamer, enabling the rapid detection of norovirus with a remarkable low detection limit of 6.17 pg mL−1. This biosensor features a linear detection range from 10 pg mL−1 to 100 ng mL−1, covering a dynamic range of 5 orders of magnitude. Similar to the SARS-CoV-2 GFET biosensor, the norovirus GFET biosensor boasts a swift detection time of 5 minutes, complemented by outstanding specificity and stability for norovirus detection.
Subsequently, we employed the SARS-CoV-2 GFET biosensor for the direct detection of SARS-CoV-2 in frozen beef, pork, shrimp, and salmon samples, yielding promising outcomes. Likewise, the norovirus GFET biosensor exhibited favorable detection results when employed for norovirus detection in razor clam, oyster, and clam samples. The efficacy of these biosensors in detecting target pathogens is attributed to the utilization of channel graphene and modified nucleic acid aptamers. The approach outlined in this study integrates nucleic acid aptamers with the GFET sensing platform, enabling the precise and rapid detection of foodborne hazards with high selectivity and sensitivity. We are confident that this technology holds immense potential in the realm of rapid food testing, facilitating the efficient monitoring of food transportation and processing. Furthermore, it presents innovative avenues for the development of biosensors tailored for the detection of harmful substances in food.
Data availability
Data for this article, including datasets for figures are available at Science Data Bank at https://doi.org/10.57760/sciencedb.10026.
Conflicts of interest
There are no conflicts to declare.
Acknowledgements
This study was supported by the National Key R&D Program of China (2022YFB3207204), the National Natural Science Foundation of China (32172294), and the Fundamental Research Funds for the Central Universities (JZ2024HGTG0283).
References
- H. Ezzatpanah, V. M. Gómez-López, T. Koutchma, F. Lavafpour, F. Moerman, M. Mohammadi and D. Raheem, New food safety challenges of viral contamination from a global perspective: Conventional, emerging, and novel methods of viral control, Compr. Rev. Food Sci. Food Saf., 2022, 21, 904–941 CrossRef PubMed.
- X. Liao, W. Shen, Y. Wang, L. Bai and T. Ding, Microbial contamination, community diversity and cross-contamination risk of food-contact ice, Food Res. Int., 2023, 164, 112335 CrossRef PubMed.
- H.-J. Jang, W. Zhuang, X. Sui, B. Ryu, X. Huang, M. Chen, X. Cai, H. Pu, K. Beavis, J. Huang and J. Chen, Rapid, Sensitive, Label-Free Electrical Detection of SARS-CoV-2 in Nasal Swab Samples, ACS Appl. Mater. Interfaces, 2023, 15, 15195–15202 CrossRef.
- D. Li, M. Y. Zhao and T. H. M. Tan, What makes a foodborne virus: comparing coronaviruses with human noroviruses, Curr. Opin. Food Sci., 2021, 42, 1–7 CrossRef PubMed.
- R. Yekta, L. Vahid-Dastjerdi, S. Norouzbeigi and A. M. Mortazavian, Food products as potential carriers of SARS-CoV-2, Food Control, 2021, 123, 107754 CrossRef PubMed.
- Y. Geng and Y. Wang, Stability and transmissibility of SARS-CoV-2 in the environment, J. Med. Virol., 2022, 95, 1–11 Search PubMed.
- R. T. da Silva, M. M. de Souza Grilo, T. C. Pimentel, F. A. de Lucena, D. W. Schaffner, G. T. de Souza Pedrosa and M. Magnani, An overview of foodborne viruses and SARS-CoV-2 in foods and food-contact surfaces: survival, transfer, surrogates use, and mathematical modeling, Curr. Opin. Food Sci., 2024, 55, 101119 CrossRef.
- T. C. Ekundayo, B. E. Igere, Y. D. Oluwafemi, C. D. Iwu and O. O. Olaniyi, Human norovirus contamination in water sources: A systematic review and meta-analysis, Environ. Pollut., 2021, 291, 118164 CrossRef PubMed.
- E. Bigoraj, I. Kozyra, A. Kaupke, Z. Osiński, J. Lowther and A. Rzeżutka, Prevalence and quantitative assessment of foodborne viruses on the imported mussels in Polish market, Food Control, 2024, 157, 110145 CrossRef.
- Q. Sun, Q. Ning, T. Li, Q. Jiang, S. Feng, N. Tang, D. Cui and K. Wang, Immunochromatographic enhancement strategy for SARS-CoV-2 detection based on nanotechnology, Nanoscale, 2023, 15, 15092–15107 RSC.
- H. Ushijima, N. T. K. Pham, S. A. Hoque, A. Nomura, K. Kumthip, Y. Shimizu-Onda, S. Okitsu, K. Kawata, N. Hanaoka, W. E. G. Müller, N. Maneekarn, S. Hayakawa and P. Khamrin, Evaluation of a novel triplex immunochromatographic test for rapid simultaneous detection of norovirus, rotavirus, and adenovirus on a single strip test, J. Infect. Public Health, 2024, 17, 619–623 CrossRef PubMed.
- J. Liu, P. Chen, X. Hu, L. Huang, Z. Geng, H. Xu, W. Hu, L. Wang, P. Wu and G. L. Liu, An ultra-sensitive and specific nanoplasmonic-enhanced isothermal amplification platform for the ultrafast point-of-care testing of SARS-CoV-2, Chem. Eng. J., 2023, 451, 138822 Search PubMed.
- J. Kaplon, L. Théry, M. Bidalot, N. Grangier, J. Frappier, L. S. Aho Glélé, A. de Rougemont, K. Ambert-Balay and A. M. Caliendo, Diagnostic Accuracy of Four Commercial Triplex Immunochromatographic Tests for Rapid Detection of Rotavirus, Adenovirus, and Norovirus in Human Stool Samples, J. Clin. Microbiol., 2020, 59, e01749-20 CrossRef PubMed.
- P. Ma, J. Liu, S. Pang, W. Zhou, H. Yu, M. Wang, T. Dong, Y. Wang, Q. Wang and A. Liu, Biopanning of specific peptide for SARS-CoV-2 nucleocapsid protein and enzyme-linked immunosorbent assay-based antigen assay, Anal. Chim. Acta, 2023, 1264, 341300 CrossRef PubMed.
- Q. Zhao, D. Lu, G. Zhang, D. Zhang and X. Shi, Recent improvements in enzyme-linked immunosorbent assays based on nanomaterials, Talanta, 2021, 223, 121722 CrossRef.
- A. Navarro, L. Gómez, I. Sanseverino, M. Niegowska, E. Roka, R. Pedraccini, M. Vargha and T. Lettieri, SARS-CoV-2 detection in wastewater using multiplex quantitative PCR, Sci. Total Environ., 2021, 797, 148890 CrossRef.
- Y. Watanabe, R. Oikawa, T. Suzuki, H. Funabashi, D. Asai, Y. Hatori, H. Takemura, H. Yamamoto and F. Itoh, Evaluation of a new point-of-care quantitative reverse transcription polymerase chain test for detecting severe acute respiratory syndrome coronavirus 2, J. Clin. Lab. Anal., 2021, 35, 1–8 CrossRef.
- D. Liu, Z. Zhang, Q. Wu, P. Tian, H. Geng, T. Xu and D. Wang, Redesigned Duplex RT-qPCR for the Detection of GI and GII Human Noroviruses, Engineering, 2020, 6, 442–448 CrossRef.
- N. Liu, R. Liu and J. Zhang, CRISPR-Cas12a-mediated label-free electrochemical aptamer-based sensor for SARS-CoV-2 antigen detection, Bioelectrochemistry, 2022, 146, 108105 CrossRef CAS PubMed.
- A. Villalonga, A. Sánchez, B. Mayol, J. Reviejo and R. Villalonga, Electrochemical biosensors for food bioprocess monitoring, Curr. Opin. Food Sci., 2022, 43, 18–26 CrossRef.
- A. Idili, C. Parolo, R. Alvarez-Diduk and A. Merkoçi, Rapid and Efficient Detection of the SARS-CoV-2 Spike Protein Using an Electrochemical Aptamer-Based Sensor, ACS Sens., 2021, 6, 3093–3101 CrossRef PubMed.
- H. Cho, S. Shim, W. W. Cho, S. Cho, H. Baek, S.-M. Lee and D.-S. Shin, Electrochemical Impedance-Based Biosensors for the Label-Free Detection of the Nucleocapsid Protein from SARS-CoV-2, ACS Sens., 2022, 7, 1676–1684 CrossRef PubMed.
- H. Jiang, Z. Sun, C. Zhang and X. Weng, 3D-architectured aptasensor for ultrasensitive electrochemical detection of norovirus based on phosphorene-gold nanocomposites, Sens. Actuators, B, 2022, 354, 131232 CrossRef.
- M. Sun, C. Zhang, S. Lu, S. Mahmood, J. Wang, C. Sun, J. Pang, L. Han and H. Liu, Recent Advances in Graphene Field-Effect Transistor Toward Biological Detection, Adv. Funct. Mater., 2024, 2024, 2405471 CrossRef.
- A. A. Mohamed, H. Noguchi, M. Tsukiiwa, C. Chen, R. S. Heath, M. Q. E. Mubarak, T. Komikawa, M. Tanaka, M. Okochi, S. P. de Visser, Y. Hayamizu and C. F. Blanford, A GFET Nitrile Sensor Using a Graphene-Binding Fusion Protein, Adv. Funct. Mater., 2022, 32, 2207669 CrossRef.
- R. Liu, F. Zhang, Y. Sang, I. Katouzian, S. M. Jafari, X. Wang, W. Li, J. Wang and Z. Mohammadi, Screening, identification, and application of nucleic acid aptamers applied in food safety biosensing, Trends Food Sci. Technol., 2022, 123, 355–375 CrossRef.
- L. F. Yang, M. Ling, N. Kacherovsky and S. H. Pun, Aptamers 101: aptamer discovery andin vitroapplications in biosensors and separations, Chem. Sci., 2023, 14, 4961–4978 RSC.
- C. Li, L. Guo, X. Sang, X. Jiang, H. Wang, P. Qin and L. Huang, Colorimetric aptasensor based on spherical nucleic acid-induced hybridization chain reaction for sensitive detection of exosomes, Talanta, 2023, 258, 124453 CrossRef.
- Y. Song, J. Song, X. Wei, M. Huang, M. Sun, L. Zhu, B. Lin, B. Lin, H. Shen, Z. Zhu and C. Yang, Discovery of Aptamers Targeting the Receptor-Binding Domain of the SARS-CoV-2 Spike Glycoprotein, Anal. Chem., 2020, 92, 9895–9900 CrossRef PubMed.
- D. Liu, Z. Zhang, Y. Yin, F. Jia, Q. Wu, P. Tian and D. Wang, Development and evaluation of a novel in situ target-capture approach for aptamer selection of human noroviruses, Talanta, 2019, 193, 199–205 CrossRef PubMed.
- Y. Oishi, H. Ogi, S. Hagiwara, M. Otani and K. Kusakabe, Theoretical Analysis on the Stability of 1-Pyrenebutanoic Acid Succinimidyl Ester Adsorbed on Graphene, ACS Omega, 2022, 7, 31120–31125 CrossRef PubMed.
- X. Wang, Z. Hao, T. R. Olsen, W. Zhang and Q. Lin, Measurements of aptamer–protein binding kinetics using graphene field-effect transistors, Nanoscale, 2019, 11, 12573–12581 RSC.
- Y. Ahn, H. Kim, Y.-H. Kim, Y. Yi and S.-I. Kim, Procedure of removing polymer residues and its influences on electronic and structural characteristics of graphene, Appl. Phys. Lett., 2013, 102, 091602 CrossRef.
- G. Seo, G. Lee, M. J. Kim, S.-H. Baek, M. Choi, K. B. Ku, C.-S. Lee, S. Jun, D. Park, H. G. Kim, S.-J. Kim, J.-O. Lee, B. T. Kim, E. C. Park and S. I. Kim, Rapid Detection of COVID-19 Causative Virus (SARS-CoV-2) in Human Nasopharyngeal Swab Specimens Using Field-Effect Transistor-Based Biosensor, ACS Nano, 2020, 14, 5135–5142 CrossRef PubMed.
- F. Teng, K. Hu, W. Ouyang and X. Fang, Photoelectric Detectors Based on Inorganic p-Type Semiconductor Materials, Adv. Mater., 2018, 29, 1706262 CrossRef.
- N. Dontschuk, A. Stacey, A. Tadich, K. J. Rietwyk, A. Schenk, M. T. Edmonds, O. Shimoni, C. I. Pakes, S. Prawer and J. Cervenka, A graphene field-effect transistor as a molecule-specific probe of DNA nucleobases, Nat. Commun., 2015, 6, 6563 CrossRef.
- E. Uesugi, H. Goto, R. Eguchi, A. Fujiwara and Y. Kubozono, Electric double-layer capacitance between an ionic liquid and few-layer graphene, Sci. Rep., 2013, 3, 1595 CrossRef PubMed.
- W. Fu, L. Jiang, E. P. van Geest, L. M. C. Lima and G. F. Schneider, Sensing at the Surface of Graphene Field-Effect Transistors, Adv. Mater., 2016, 29, 1603610 CrossRef PubMed.
- S. Wang, M. Z. Hossain, K. Shinozuka, N. Shimizu, S. Kitada, T. Suzuki, R. Ichige, A. Kuwana and H. Kobayashi, Graphene field-effect transistor biosensor for detection of biotin with ultrahigh sensitivity and specificity, Biosens. Bioelectron., 2020, 165, 112363 CrossRef PubMed.
- C. Dai, M. Guo, Y. Wu, B.-P. Cao, X. Wang, Y. Wu, H. Kang, D. Kong, Z. Zhu, T. Ying, Y. Liu and D. Wei, Ultraprecise Antigen 10-in-1 Pool Testing by Multiantibodies Transistor Assay, J. Am. Chem. Soc., 2021, 143, 19794–19801 CrossRef.
- I. M. Bhattacharyya and G. Shalev, Electrostatically Governed Debye Screening Length at the Solution-Solid Interface for Biosensing Applications, ACS Sens., 2019, 5, 154–161 CrossRef.
- T. Rodrigues, V. Mishyn, Y. R. Leroux, L. Butruille, E. Woitrain, A. Barras, P. Aspermair, H. Happy, C. Kleber, R. Boukherroub, D. Montaigne, W. Knoll and S. Szunerits, Highly performing graphene-based field effect transistor for the differentiation between mild-moderate-severe myocardial injury, Nano Today, 2022, 43, 101391 CrossRef.
- N. Nakatsuka, K. A. Yang, J. M. Abendroth, K. M. Cheung, X. Xu, H. Yang, C. Zhao, B. Zhu, Y. S. Rim, Y. Yang, P. S. Weiss, M. N. Stojanović and A. M. Andrews, Aptamer–field-effect transistors overcome Debye length limitations for small-molecule sensing, Science, 2018, 362, 6412 CrossRef PubMed.
- S. Wang, M. Sun, Y. Zhang, H. Ji, J. Gao, S. Song, J. Sun, H. Liu, Y. Zhang and L. Han, Ultrasensitive Antibiotic Perceiving Based on Aptamer-Functionalized Ultraclean Graphene Field-Effect Transistor Biosensor, Anal. Chem., 2022, 94, 14785–14793 CrossRef.
- M. Shaffer, K. Huynh, V. Costantini, K. Bibby and J. Vinjé, Viable Norovirus Persistence in Water Microcosms, Environ. Sci. Technol. Lett., 2022, 9, 851–855 CrossRef PubMed.
|
This journal is © The Royal Society of Chemistry 2024 |
Click here to see how this site uses Cookies. View our privacy policy here.