Amplitude multiplexed wastewater surveillance for campus health: tracking SARS-CoV-2, influenza A, and norovirus†
Received
5th February 2024
, Accepted 12th March 2024
First published on 15th March 2024
Abstract
The University of Southern California implemented a wastewater-based surveillance program (USC-WS) to proactively prevent viral outbreaks and maintain a safe campus environment for students. The main objective of this project was to develop a novel amplitude multiplex assay to quantify SARS-CoV-2, influenza A, norovirus, and PMMoV. The study aimed to 1) describe the temporal trends of the target viruses from the start of Fall 2022 to the end of the Spring 2023 academic semester; 2) establish correlations between USC-WS and USC Student Health clinical data; and 3) assess the impact of varying wastewater lead-time and PMMoV normalizations on correlations with clinical data. Two-step RT-ddPCR assays quantified the viral targets in an amplitude multiplex to overcome the limitation of two fluorescent channels on the QX200 Droplet Reader. Statistical analysis demonstrated statistically significant (p-value < 0.05 and Pearson r ≥ 0.50) correlations between SARS-CoV-2 and influenza A wastewater data and reported clinical case data, but not for norovirus. Further, normalization by PMMoV improved correlations for SARS-CoV-2 and norovirus, but not for influenza A. We also found that SARS-CoV-2 wastewater data provides an earlier lead-time to the onset of clinical cases than influenza A. This study validated the amplitude multiplex of SARS-CoV-2, influenza A, norovirus, and PMMoV for wastewater surveillance and the lead-time building-scale wastewater can provide ahead of clinical cases.
Water impact
Wastewater surveillance is independent from healthcare accessibility, testing availability, or sick individuals seeking healthcare, and can be utilized as an early warning system for disease outbreaks. This study implemented wastewater surveillance on a university campus to monitor infectious communal diseases utilizing ddPCR and a novel amplitude multiplex to quantify SARS-CoV-2, influenza A, norovirus, and PMMoV. The findings demonstrate effective campus wastewater monitoring and help guide future surveillance projects.
|
1. Introduction
The rapid expansion of wastewater-based epidemiology (WBE) as a result of the COVID-19 pandemic enabled widespread and real-time wastewater-based monitoring of community health.1,2 Wastewater surveillance (WS) allows for the early detection of infectious diseases within a community and circumvents the difficulties of accessibility and availability of individual clinical testing, as well as the shift towards at-home tests that do not get reported. Infected individuals may shed genetic material into wastewater before showing symptoms,3,4 providing an opportunity for health officials to intervene and mitigate an outbreak. WS is beneficial for identifying patterns in viral prevalence, monitoring changes over time, and informing effective allocation of healthcare resources.5 Further, WS provides significantly reduced costs and resource intensity relative to individual clinical testing, especially for large populations in areas where healthcare resources may be strained.6–8
As government funding for COVID-19 testing decreased, a drastic reduction in testing followed.9 Maintaining government-supported clinical testing is not economically viable,10 and the burden of testing and reporting in the United States has been shifted to the individual. On May 11th, 2023, the United States ended the COVID-19 Public Health Emergency Declaration.11 In Los Angeles (LA) County, the number of 7-day average daily tests reported declined 61% in three months, from 11
300 in May to 4400 in August 2023.12 While clinical testing is the gold standard for evaluating community health, it is bound by a population's behavior, asymptomatic cases, testing accessibility, and availability.13,14 With the tremendous fall in testing for COVID-19 and lack of testing for other diseases, WS is emerging as our most reliable indicator of tracking trends or disease emergence within a community.
In metropolitan areas across the United States, such as Los Angeles, Chicago, and Boston, large-scale centralized water reclamation facilities (WRFs) serve millions of people.15–17 For example, the expansive sewershed associated with the Los Angeles Sanitation and Environment's Hyperion Water Reclamation Plant (HWRP) serves over 4 million people,15 allowing WS to provide a broad snapshot of community health. HWRP is a partner in a large national wastewater monitoring project called WastewaterSCAN, which measures concentrations of a range of viral targets in wastewater and shares data publicly to inform public health response to changes in pathogen levels and trends. However, mitigating localized outbreaks or allocating health resources in such a large sewer shed is difficult. WS in communal living situations, such as university residential buildings, allows for more focused monitoring of a smaller population. These populations often have an increased risk of gastrointestinal and upper respiratory infections due to resident proximity and individual hygiene habits.18–20 Research has demonstrated that building-scale WS is sufficiently sensitive to identify a single SARS-CoV-2 infection in a building.21,22
The University of Southern California (USC) implemented a wastewater-based surveillance program (USC-WS) to monitor student health by providing an early warning system for potential viral outbreaks beginning in Fall 2021. Real-time WS served as a complementary tool to clinical testing and allowed for a targeted approach for improving student health; several universities have successfully implemented WS, focusing on SARS-CoV-2.21,23–25 As the pandemic progressed, building-scale WS of SARS-CoV-2 and influenza became more common.26,27 USC-WS was initially designed to mitigate SARS-CoV-2 infections; however, as the COVID-19 pandemic subsided, the program expanded to include important seasonal respiratory and enteric pathogens, including influenza A, norovirus, as well as PMMoV for normalization. The study aims to establish correlations between USC-WS, WastewaterSCAN's LA County HWRP data, and USC Student Health Center (USC-SH) clinical case data and assess the impact of varying wastewater lead-time and PMMoV normalization on correlations with clinical cases.
2. Methods
2.1. Studied population
USC is a private research university in South Los Angeles, California, with an overall enrollment of 49
000 students, with roughly 21
000 undergraduate and 28
000 graduate students. The University Park Campus covers 229 acres and is home to approximately 7000 undergraduate students residing on campus. USC-SH provides medical care and diagnostic testing for influenza, norovirus, and other diseases to all students on campus. USC-SH also offered mass testing for SARS-CoV-2 to all students and employees throughout the pandemic and continues to provide diagnostic testing to eligible students. USC-WS is a coordinated joint effort between the Smith research group in the Astani Department of Civil and Environmental Engineering, USC-SH, USC Environmental Health & Safety, and USC Facilities Management Services. Deidentified and aggregated clinical testing data was collected from USC-SH. LA County wastewater data from 6 January 2023 to 1 May 2023 for HWRP was provided by the WastewaterSCAN program. HWRP was selected due to the USC University Park Campus residing within the HWRP sewershed.
2.2. Sample collection
USC-WS encompasses 10 student residential buildings: 8 dormitories and 2 on-campus apartments (Fig. 1A). The associated surveillance system utilized 26 sewage junctions sampled twice a week as twenty-four-hour time-weighted composite samples throughout the Fall 2022 to Spring 2023 academic year with glacier transportable samplers (Teledyne Isco, Lincoln, NE). To increase granularity in temporal data, two residential buildings (ESI† Table S1) were sampled daily, Monday through Friday. Due to low occupancy and wastewater flow on campus, samples were not collected from December 17th, 2022, through January 8th, 2023. Each refrigerated autosampler collected approximately 50 mL of wastewater every hour and held the samples at 4 °C. Wastewater samples were collected and then transported to the on-campus laboratory, where they were processed and analyzed with the goal of reporting to USC-SH the same day (Fig. 1B).
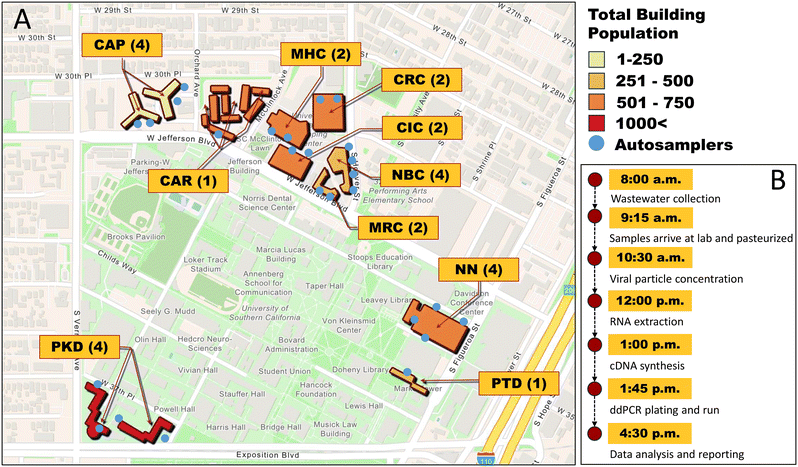 |
| Fig. 1 USC-WS sample collection map: describing the site, population, and number of autosamplers (A). The daily workflow of the lab team (B). | |
2.3. Viral particle concentration, RNA extraction, and quantification
All collected samples were pasteurized in a water bath at 60 °C for one hour to inactivate viruses.28 For buildings with multiple samplers, samples from each autosampler were equally pooled by volume and aliquoted into a 50 mL sterile conical bottom centrifuge tube (Corning Incorporated, NY). Each aliquot was amended with 500 μL of 2 M MgCl2, a cationic conditioning solution, to promote the formation of salt bridges within electronegative membrane filters (EMF). Composite wastewater samples were filtered in duplicate using HA filtration29 through a 0.45 μM S-Pak® membrane filter (Millipore-Sigma, USA) with a Rocker 400 vacuum filtration system (Rocker Scientific Co., Taiwan). Inactivated SARS-CoV-2, influenza A (Vircell S.L., Granada, Spain), and surrogate murine norovirus (Gold Standard Diagnostics, Budapest, Hungary) were used as endogenous extraction controls spiked into wastewater weekly at 2.50 × 104 copies for SARS-CoV-2 and influenza A and 1.0 × 104 copies for murine norovirus.
RNA was automatically extracted from EMFs using the Maxwell™ 16 LEV simplyRNA Blood kit (Promega Corporation, Madison, WI) on a Maxwell® 16 Instrument (Promega Corporation, Madison, WI) until March 2023. In March 2023, we switched to the Maxwell™ RSC simplyRNA Blood kit (Promega Corporation, Madison, WI) on a Maxwell® RSC 48 Instrument (Promega Corporation, Madison, WI). A modified RNA extraction protocol was used. In brief, after filtration, EMFs were stored in a 2 mL tube with 600 μL of cell lysis buffer, 60 μL of proteinase K solution, and 0.5 g of zirconia beads (BioSpec Products Inc, Bartlesville, OK). Samples were vortexed at 3000 rpm for 15 seconds and then incubated at 56 °C for 10 minutes. This was followed by bead beating at 2400 RPM for 2 minutes on a Mini-BeadBeater 96 (BioSpec Products Inc, Bartlesville, OK) and centrifuged at 12
500 RPM for 2 minutes. 300 μL of supernatant was aspirated from the tube and dispensed into the first well of the cartridge. An additional 300 μL of cell lysis buffer was added to the original sample tube, followed by another beat beating and centrifugation round. A subsequent 300 μL of supernatant was added to the cartridge (total of 600 μL). We then proceeded with the kit-specific automated extraction method according to the instrument manufacturer and eluted into 40 μL. Samples were immediately processed after extraction.
Two-step RT digital droplet PCR (ddPCR) assays were performed to quantify SARS-CoV-2, influenza A, norovirus, and PMMoV in a multiplex assay (ESI† Table S2) in compliance with dMIQE guidelines.30 Our multiplex assay targeted conserved genomic regions and utilized degenerate primers to overcome single nucleotide polymorphisms. The assays targeted the dominant strains of the pathogens of interest verified by Primer-BLAST,31 such as norovirus GI and GII, influenza A H1N1 and H3N2, and COVID-19 variants, including omicron. Following the manufacturer's instructions (ESI† Table S3), 2 μL of extracted RNA was reverse transcribed to cDNA using the iScript™ cDNA Synthesis kit (Bio-Rad, Hercules, CA, USA). Immediately after, 1 μL of cDNA was amplified using the 22 μL reaction set-up of ddPCR Multiplex Supermix (Bio-Rad, Hercules, CA, USA), nuclease-free water, and 20× primer/probe concentration (ESI† Table S4) for Hi-FAM and Hi-HEX and 12.5× concentration for Lo-FAM and Lo-HEX (Integrated DNA Technologies, Coralville, IA). Droplet generation was performed by Bio-Rad Automating Droplet Generator (Bio-Rad, Hercules, CA, USA) and followed by amplification of the viral nucleic acid targets on a Bio-Rad C1000 Touch Thermal Cycler (Bio-Rad, Hercules, CA, USA). The thermal cycling conditions included enzyme activation at 95 °C for 10 minutes, 40 cycles of denaturation at 94 °C for 30 seconds and annealing/extension at 56 °C for 60 seconds, enzyme deactivation at 98 °C for 10 minutes, and a 30 minute hold at 4 °C for the reabsorption of droplets back into solution. The amplification data was analyzed on a QX200 Droplet Reader (Bio-Rad, Hercules, CA, USA) using QuantaSoft Analysis Pro (1.0.596; Bio-Rad, Hercules, CA, USA). A positive control of synthetic RNA (Promega Corporation, Madison, WI and Twist Biosciences, San Francisco, CA) of the viral targets (ESI† Table S5) was tested in duplicate for each run to ensure correct amplification in the expected amplitude regions (ESI† Table S7). Positive detection was considered when the limit of detection (LOD) thresholds were met (ESI† Table S6). Samples were not diluted for analysis, nor were the droplets saturated at any point. The differences in concentrations can be observed in ESI† Table S8.
2.4. ddPCR assay validation
To find the proper amplitude regions, synthetic RNA of the viral targets was amplified with varying fluorophore concentration ratios (20× :
10×, 20× :
12.5×) (ESI† Fig. S1) and temperature gradients for annealing/extension on the thermal cycler (55 °C to 65 °C) in triplicate (ESI† Fig. S2). We observed the best separation of clusters using the 20× :
12.5× concentrations and 56 °C annealing/extension step (Fig. 2). Then, serial dilutions of the positive control were quantified in triplicate to assess the LOD (ESI† Table S6). The serial dilutions also established the thresholds for identifying partition clusters (ESI† Table S7). Fluorophore variations and temperature gradient experiments are necessary to deal with intermediate fluorescence (rain) between droplet partitions (not pictured).
 |
| Fig. 2 Graphical output for 2-step RT-ddPCR amplitude multiplex assay using fluorophore ratio of 20× and 12.5× and an annealing/elongation temperature of 56 °C. The illustration utilized the QX200™ Droplet Digital™ PCR System (Bio-Rad, Hercules, CA, USA) and was visualized using QuantaSoft Analysis Pro (1.0.596; Bio-Rad, Hercules, CA, USA). | |
2.5. Viral target quantification
To calculate the viral target copy number, we followed Bio-Rad's instructions for ddPCR absolute quantification with conversion factors applied to achieve copies per L (eqn (1)); | 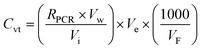 | (1) |
where Cvt is the concentration of our viral target, RPCR is the raw data output from QuantaSoft Analysis Pro (1.0.596; Bio-Rad, Hercules, CA, USA), Vw is the volume within the reaction well, Vi is the volume of DNA input, Ve is the elution volume, VF is the volume of the wastewater filtered.
Norovirus GII and PMMoV RNA concentrations obtained from the WastewaterSCAN project were measured in wastewater solids isolated from influent collected three times a week at HWRP. Norovirus and PMMoV concentrations between 6 Jan 2023 and 1 May 2023 are included in this paper. A subset of these data (between 6 Jan 2023 and 9 April 2023) has already been published in Boehm et al.32 The remaining data has not been published previously, but samples were collected and analyzed using the same methods as described by Boehm et al.32 Norovirus GII concentrations were normalized by PMMoV and multiplied by 1 million; PMMoV normalization is suggested by the wastewater surveillance community33 and applied this approach to achieve normalized values (eqn (2));
| 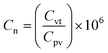 | (2) |
where
Cn is the normalized concentration,
Cvt is the concentration of our viral target, and
Cpv is the concentration of PMMoV.
2.6. Statistical analysis
All figures and statistical analyses were generated using R version 4.3.1. Pearson correlation coefficients were used to assess the strength of the linear relationships between USC-WS and USC Student Health clinical cases, and one-way ANOVA tests were used to determine the statistical significance. Data was tested for normality using the Shapiro–Wilk test and was log
10 transformed prior to statistical analysis. The data point was excluded from statistical analyses if all target genes were non-detect for a given sample. The correlations were also assessed using a one-week lead-time for the wastewater data, as SARS-CoV-2 has been demonstrated to appear in wastewater up to 8 days before clinical diagnosis.34–36 Since the clinical data was tracked weekly, average weekly concentrations from all test sites were used.
3. Results and discussion
3.1. Two-step RT-ddPCR amplitude multiplexing demonstrates clearly identifiable clusters and accurate quantification of viral targets
Quantitative polymerase chain reaction (qPCR) is a standard quantification method for genetic targets. Droplet digital PCR (ddPCR) offers an alternative quantification method for WS. ddPCR is known for its robustness, offering greater tolerance to variability in reaction conditions and sample quality common with wastewater matrices, reducing the risk of false-negative results.37–39 With broad implementation in WS workflows,23,37,40–44 ddPCR has become a vital quantification tool.
To overcome the limitation of two fluorescent channels on the QX200 Droplet Reader (Bio-Rad, Hercules, CA, USA), an amplitude multiplex was employed. Amplitude multiplexing utilizes fluorescent probes of the same dye (e.g., FAM) and varies the final concentration in the PCR reaction mix, causing distinct relative fluorescent units (RFUs) for each target.45 When multiplexing four targets, up to 16 clusters can appear on the 2D amplitude space, indicating each permutation that can be present in a droplet, such as negative, single, double, triple, or quadruple positive. We targeted SARS-CoV-2 and norovirus on the FAM channel as the high and low concentrations, respectively. For the HEX channel, influenza A and PMMoV were the high and low concentrations, respectively (Fig. 2).
When analyzing data for one or two targets, QuantaSoft (1.7; Bio-Rad, Hercules, CA, USA) can auto-analyze droplet data; however, more than two targets require using QuantaSoft Analysis Pro (1.0.596; Bio-Rad, Hercules, CA, USA), and the user must define thresholds for each target emphasizing the need of establishing typical RFU amplitude regions of targets on the 2D plane (ESI† Table S7). Newer digital droplet readers can use up to 6 fluorescent channels; however, users can still employ amplitude multiplexing and achieve up to 12 targets.
3.2. Disease viral loads in wastewater of SARS-CoV-2 and influenza A were highest during the fall and winter, while norovirus appeared sporadically
Over the academic year, 607 wastewater samples were collected from residential buildings on the University Park Campus (ESI† Fig. S3). Prevention protocols were enacted when a building displayed two consecutive positive wastewater results. When consecutive positive wastewater detection occurred for SARS-CoV-2, rapid tests were delivered to the on-campus student housing residences, and students were notified via email, encouraged to test, and report positive results to USC-SH. For influenza A, students were notified and encouraged to get tested if symptomatic; for norovirus, students were notified and encouraged to seek treatment if symptomatic; enhanced cleaning protocols were employed by the Facilities Management Services Team.
From September 5, 2022, to May 4, 2023, USC-SH reported 2938 students with COVID-19, 162 with influenza A, and 892 with gastroenteritis symptoms. Norovirus tests are not commonly performed at USC-SH – only two tests were conducted during the academic year – therefore, the norovirus data is compared with gastroenteritis symptoms. For COVID-19, the weekly positive count ranged from 13 to 299 infections; for influenza A, positive weekly cases ranged from 0 to 26. The largest positive case count for COVID-19 coincided with the week students returned from Thanksgiving Break (Fig. 3A). The week before the onset of COVID-19 clinical case peak, the average viral load of SARS-CoV-2:PMMoV was 3.66
log10 (Fig. 3A and D). Influenza A infections peaked during the week of Thanksgiving Break (Fig. 3B). The week of the influenza A clinical case peak average influenza A:PMMoV viral load of 2.88
log10 was observed (Fig. 3B and E). Norovirus:PMMoV had four spikes during the year, but the largest viral load occurred a week before the Fall Recess at 2.70
log10 (Fig. 3C); however, many of the results found non-detects in the wastewater (Fig. 3F). Even though norovirus accounts for almost 20% of all acute gastroenteritis,46 our lack of finding norovirus in wastewater may be attributed to the small population within our sewershed. Individuals may have brought norovirus back to campus as three of the four spikes occurred during heavy population movement on campus (Fig. 3C).
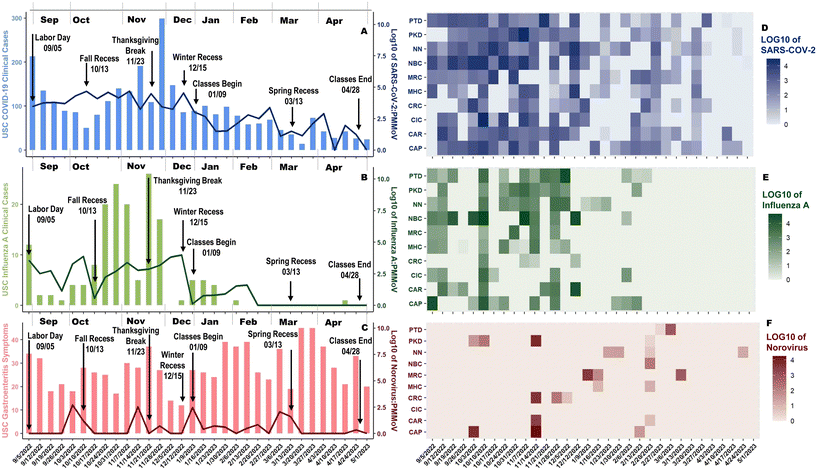 |
| Fig. 3 Temporal evaluation of viral targets and clinical case data demonstrating SARS-CoV-2, influenza A (A and B). Norovirus is temporally evaluated against gastroenteritis symptoms (C) due to only two norovirus tests provided during this academic year. The timeline represents events that significantly alter the student population on campus. The heatmap describes infection rates of the target diseases afflicting student residential buildings (D–F). All non-detects are classified as 0. | |
The wastewater viral loads of SARS-CoV-2 remained relatively consistent from September through December, then trended down until the end of the spring semester (Fig. 3D). Influenza A wastewater viral loads experienced spikes until consistently increasing from November through December, and upon students returning to campus, low viral loads were present until completely receding from campus in the spring (Fig. 3E). For SARS-CoV-2, clinical cases dropped, climbed to a peak in November, and faded until the year's end. On the other hand, influenza A rose between October through December before disappearing in the spring. Influenza A and SARS-CoV-2 have been demonstrated to spike seasonally in the fall and winter months,47,48 similar to USC-WS data. No discernable trend was associated with norovirus and gastroenteritis symptoms, which can be attributed to a lack of norovirus testing, asymptomatic carriers,49 and many conditions that can cause these symptoms, such as rotavirus, adenovirus, and Salmonella.50–52 Interestingly, the three highest peaks for COVID-19 clinical diagnosis also corresponded with tests given at USC-SH during the weeks of September 5th, November 14th, and November 28th. Similarly, influenza A also had its three highest case counts when the most tests were provided during the weeks of October 31st, November 7th, and November 21st. This suggests that discrepancies between wastewater viral loads and clinical cases may be related to a lack of clinical testing and that actual case counts for SARS-CoV-2 and influenza A were likely much higher.
3.3. Using PMMoV and lead times to improve correlations with clinical cases varies among diseases
Given differences in waste input, gray water dilution, and population fluctuations, normalizing ddPCR quantification data from wastewater is essential to accurately assess the prevalence of infectious agents.53–56 The CDC recommends using PMMoV as a population fecal indicator to normalize SARS-CoV-2.33,57,58 PMMoV is introduced through human dietary habits and is one of the most abundant RNA viruses in human waste.59–61 Several building-scale WS studies have quantified wastewater's predictive capability for SARS-CoV-2,62,63 as correlations are strongest with a one-week lead-time to the onset of clinical cases. USC-WS corroborates both findings as SARS-CoV-2 was the most statistically significant when normalizing with PMMoV and leading the onset of COVID-19 cases by one week (Table 1B).
Table 1 Pearson correlations and one-way ANOVA during the Fall 2022–Spring 2023 USC academic year. Each section is varied by correlating wastewater prevalence by one week ahead of or the same week as clinical diagnosis, as well as if the data was normalized by PMMoV (B) or not (A). Norovirus is compared with gastroenteritis symptoms reported by the student health center. Significant values (p-value < 0.05 and r ≥ 0.50) are bolded
A
|
SARS-CoV-2 non-normalized |
Influenza A non-normalized |
Norovirus non-normalized |
Lead-time to clinical cases |
p-Value |
Pearson r |
p-Value |
Pearson r |
p-Value |
Pearson r |
None |
0.002
|
0.52
|
0.001
|
0.55
|
0.625 |
−0.09 |
One week |
0.001
|
0.58
|
0.008
|
0.46 |
0.995 |
0.00 |
B
|
SARS-CoV-2:PMMoV normalized |
Influenza A:PMMoV normalized |
Norovirus:PMMoV normalized |
Lead-time to clinical cases |
p-Value |
Pearson r |
p-Value |
Pearson r |
p-Value |
Pearson r |
None |
0.004
|
0.49 |
0.004
|
0.49 |
0.744 |
−0.06 |
One week |
p < 0.001
|
0.62
|
0.053 |
0.35 |
0.586 |
0.10 |
Few studies have correlated building-scale WS influenza A data with clinical cases, and the studies that have conducted such correlations demonstrated influenza A's presence in wastewater leading clinical cases between 2–4 days.64 However, studies have not evaluated the validity of PMMoV normalization for influenza A. USC-WS exhibited the highest correlations when influenza A wastewater viral loads were not normalized by PMMoV and compared the same week as the onset of clinical diagnoses (Table 1A). This may be attributed to influenza A having a shorter incubation period or viral shedding occurrence before the onset of symptoms than SARS-CoV-2.65,66 Therefore, the intervention window to prevent an influenza outbreak is significantly reduced. Furthermore, PMMoV may be unreliable for normalizing a disease that primarily exists in the colder months, as PMMoV exhibits a strong association with temperature and consumption of peppers in warmer seasons.59
Similar to the results of USC-WS (Table 1), using fecal indicators, including PMMoV, crAssphage, and F Colifage, can be inconsistent with improving the correlation between a viral target and clinical data.41,56,59,67,68 However, other normalization methods may require the use of liquid chromatography–mass spectrometry as it can quantify biomarkers and chemical tracers, such as caffeine, paraxanthine, and creatinine.69 Moreover, using wastewater flows can also serve as a normalization method and convert the concentration into mass loads. The method of normalization depends on the application. For example, PMMoV and other fecal indicators normalize to fecal volume to account for the environmental effects that our viral targets endure and population fluctuations. Normalization correlation inconsistencies call for further research into normalization techniques and their impact on different scales and viral targets.
3.4. Norovirus wastewater data corresponds to an outbreak in Los Angeles County
Due to the infrequency of clinical norovirus testing,70 statistical analysis with gastroenteritis symptoms reported to USC-SH did not result in correlations between wastewater data and clinical cases (Table 1). The clinical data could present potential biases, given that reported gastroenteritis symptoms may not be related to norovirus infections. Instead, WS data was temporally compared against WastewaterSCAN's HWRP norovirus wastewater data.
Surges in USC-WS and WastewaterSCAN's HWRP average norovirus viral load were consistent (Pearson r = 0.42) with one another and peaked during the weeks of March 13th and March 20th, respectively (Fig. 4). The upswing in viral loads corresponded with an elementary school closure due to a norovirus outbreak beginning on March 17th, 2023,71 confirming the strong presence of norovirus in LA County during this time. With a short incubation time,72 rapid intervention is necessary after an upward trend in norovirus wastewater viral load to prevent future outbreaks and school closures. As WS continues to expand, developing wastewater monitoring systems that include populations at risk for communal diseases (e.g., elementary schools, nursing homes, apartment complexes) can contain and prevent further outbreaks of highly virulent diseases.
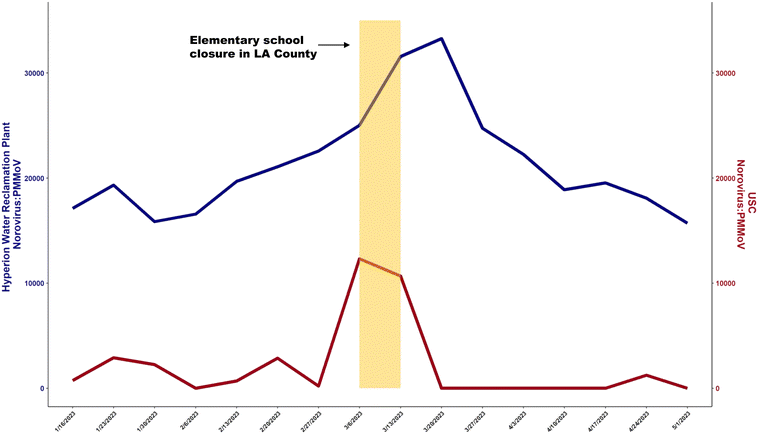 |
| Fig. 4 Temporal WastewaterSCAN HWRP (primary y-axis) and USC-WS (secondary y-axis) weekly average of norovirus normalized by PMMoV. The highlighted section denotes the week prior to the elementary school closure on March 17th, 2023. | |
3.5. Inherent biases in wastewater surveillance and future insights
As WS continues to expand, researchers face several challenges that contribute to data variability, complexity in interpretation, and thresholds for intervention. Wastewater's complex matrix can degrade RNA and introduce inhibitors that alter RNA extraction and PCR amplification efficiency of viral targets.73,74 With the rapid expansion of WS, there is substantial variability in laboratory workflows, ranging from sample collection, viral particle concentration techniques, nucleic acid extraction, and target quantification.29,56,75 The differences in methods between labs can drastically alter extraction efficiencies and quantification accuracy of viral targets.2 Viral particle shedding can vary in individuals, both from the presence of the virus in the gastrointestinal tract and varying shedding rates depending on where the individual is in the infection cycle,76 hindering estimates of the total number of people shedding. Moreover, we face the difficulty of interpreting results in the absence of clinical data, illustrating the need to develop frameworks for validating WS data beyond correlations with clinical cases. In addition, WS needs collaborative efforts between wastewater facilities and public health officials to coordinate a complex surveillance network (from individual buildings to centralized treatment facilities). For example, at a WRF, the presence of pathogens can be observed, while building-scale can identify hotspots of infections. In conjunction, they offer a comprehensive approach for public health.
4. Conclusions
The objective of this study was to target highly communicable diseases for outbreak prevention at USC. This study validated using a WS amplitude multiplex of SARS-CoV-2, influenza A, norovirus, and fecal indicator PMMoV. SARS-CoV-2's presence in building-scale wastewater can predicate clinical cases by one week, while influenza A's displays a higher correlation the same week as the onset of clinical cases, indicating a shorter intervention window for influenza A. As displayed by USC-WS and WastewaterSCAN's HWRP data, wastewater surveillance of norovirus can detect outbreaks in the community. The early notification system that USC-WS provided allowed USC-SH to notify at-risk residents in student housing and authorized Facilities Management Services to invoke advanced cleaning protocols based on the viral target detected. Further, we demonstrated that PMMoV normalized data provides increased correlation strength with SARS-CoV-2 but not influenza A. This suggests that further research of alternative normalization methods may be required for future implementations of WS. Proactive building-scale wastewater surveillance offers an effective strategy to monitor several infectious diseases, paving the way for a healthier community.
Conflicts of interest
There are no conflicts to declare.
Acknowledgements
This work was supported by the University of Southern California Office of the Provost. Michael A. Saldana was partially supported by a Provost Fellowship from USC. Statewide Services Inc. (La Quinta, CA) regularly maintained the autosamplers. We thank USC Student Health and Facilities Management Services for their collaboration and intervention measures. We also thank Dani Fedele, Anushree Talwalker, Bhavik Shah, Hanrui Li, and the rest of the Smith Lab members at the University of Southern California for their assistance in sample processing throughout the duration of the study. Permission to use WastewaterSCAN data was received from Alexandria Boehm and Marlene Wolfe; we acknowledge their input on parts of the manuscript related to describing that data.
References
- D. Polo, M. Quintela-Baluja, A. Corbishley, D. L. Jones, A. C. Singer and D. W. Graham,
et al. Making waves: Wastewater-based epidemiology for COVID-19 – approaches and challenges for surveillance and prediction, Water Res., 2020, 186, 116404 CrossRef CAS PubMed.
-
V. Munteanu, M. Saldana, N. K. Sharma, W. O. Ouyang, E. Aßmann and V. Gordeev, et al., SARS-CoV-2 Wastewater Genomic Surveillance: Approaches, Challenges, and Opportunities, arXiv, 2023, preprint, arXiv:2309.13326v2, DOI:10.48550/arXiv.2309.13326.
-
F. Wu, A. Xiao, J. Zhang, K. Moniz, N. Endo and F. Armas, et al., SARS-CoV-2 titers in wastewater foreshadow dynamics and clinical presentation of new COVID-19 cases, medRxiv, 2020, preprint, DOI:10.1101/2020.06.15.201177477.
- X. Li, J. Kulandaivelu, Y. Guo, S. Zhang, J. Shi and J. O'Brien,
et al. SARS-CoV-2 shedding sources in wastewater and implications for wastewater-based epidemiology, J. Hazard. Mater., 2022, 432, 128667 CrossRef CAS PubMed.
- J. O'Keeffe, Wastewater-based epidemiology: current uses and future opportunities as a public health surveillance tool, Environ. Health Rev., 2021, 64(3), 44–52 CrossRef.
- B. K. Yoo, R. Iwamoto, U. Chung, T. Sasaki and M. Kitajima, Economic Evaluation of Wastewater Surveillance Combined with Clinical COVID-19 Screening Tests, Japan, Emerging Infect. Dis., 2023, 29(8), 1608–1617 Search PubMed.
- J. Wright, E. M. Driver, D. A. Bowes, B. Johnston and R. U. Halden, Comparison of high-frequency in-pipe SARS-CoV-2 wastewater-based surveillance to concurrent COVID-19 random clinical testing on a public U.S. university campus, Sci. Total Environ., 2022, 820, 152877 CrossRef CAS PubMed.
- J. I. Levy, K. G. Andersen, R. Knight and S. Karthikeyan, Wastewater surveillance for public health, Science, 2023, 379(6627), 26–27 CrossRef CAS PubMed.
- A. D. Usher, FIND documents dramatic reduction in COVID-19 testing, Lancet Infect. Dis., 2022, 22(7), 949 CrossRef CAS PubMed.
- O. Filchakova, D. Dossym, A. Ilyas, T. Kuanysheva, A. Abdizhamil and R. Bukasov, Review of COVID-19 testing and diagnostic methods, Talanta, 2022, 244, 123409 CrossRef CAS PubMed.
- COVID-19 and Your Health, https://www.cdc.gov/coronavirus/2019-ncov/your-health/end-of-phe.html, (Accessed September 2023).
- LA County COVID-19 Surveillance Dashboard, https://dashboard.publichealth.lacounty.gov/covid19_surveillance_dashboard/, (Accessed September 2023).
- L. Zhao, Y. Zou, R. E. David, S. Withington, S. McFarlane and R. A. Faust,
et al. Simple methods for early warnings of COVID-19 surges: Lessons learned from 21 months of wastewater and clinical data collection in Detroit, Michigan, United States, Sci. Total Environ., 2023, 864, 161152 CrossRef CAS PubMed.
- P. Gahlot, K. D. Alley, S. Arora, S. Das, A. Nag and V. K. Tyagi, Wastewater surveillance could serve as a pandemic early warning system for COVID-19 and beyond, Wiley Interdiscip. Rev.: Water, 2023, 10(4), e1650 CrossRef.
- Hyperion Water Reclamation Plant, https://www.lacitysan.org/san/faces/home/portal/s-lsh-wwd/s-lsh-wwd-cw/s-lsh-wwd-cw-p/s-lsh-wwd-cw-p-hwrp?_afrLoop=10842315954174656&_afrWindowMode=0&_afrWindowId=null&_adf.ctrl-state=cd88qlcxv_1#!%40%40%3F_afrWindowId%3Dnull%26_afrLoop%3D10842315954174656%26_afrWindowMode%3D0%26_adf.ctrl-state%3Dcd88qlcxv_5, (Accessed September 2023).
- Stickney Water Reclamation Plant, https://legacy.mwrd.org/irj/portal/anonymous/stickney, (Accessed September 2023).
- Massachusetts Water Resources Authority, https://www.mwra.com/02org/html/whatis.htm, (Accessed September 2023).
- V. Kak, Infections in Confined Spaces: Cruise Ships, Military Barracks, and College Dormitories, Infect. Dis. Clin. North Am., 2007, 21(3), 773–784 CrossRef PubMed.
- Y. Sun, Z. Wang, Y. Zhang and J. Sundell, In China, Students in Crowded Dormitories with a Low Ventilation Rate Have More Common Colds: Evidence for Airborne Transmission, PLoS One, 2011, 6(11), e27140 CrossRef CAS PubMed.
- C. White, R. Kolble, R. Carlson and N. Lipson, The Impact of a Health Campaign on Hand Hygiene and Upper Respiratory Illness Among College Students Living in Residence Halls, J. Am. Coll. Health, 2023, 53(4), 175–181 CrossRef PubMed.
- C. Gibas, K. Lambirth, N. Mittal, M. A. I. Juel, V. B. Barua and L. R. Brazell,
et al. Implementing building-level SARS-CoV-2 wastewater surveillance on a university campus, Sci. Total Environ., 2021, 782, 146749 CrossRef CAS PubMed.
- O. E. Hart and R. U. Halden, Computational analysis of SARS-CoV-2/COVID-19 surveillance by wastewater-based epidemiology locally and globally: Feasibility, economy, opportunities and challenges, Sci. Total Environ., 2020, 730, 138875 CrossRef CAS PubMed.
- R. Gonzalez, K. Curtis, A. Bivins, K. Bibby, M. H. Weir and K. Yetka,
et al. COVID-19 surveillance in Southeastern Virginia using wastewater-based epidemiology, Water Res., 2020, 186, 116296 CrossRef CAS PubMed.
- L. M. Langan, M. O'Brien, Z. C. Rundell, J. A. Back, B. J. Ryan and C. K. Chambliss,
et al. Comparative Analysis of RNA-Extraction Approaches and Associated Influences on RT-qPCR of the SARS-CoV-2 RNA in a University Residence Hall and Quarantine Location, ACS ES&T Water, 2022, 2(11), 1929–1943 Search PubMed.
- W. Q. Betancourt, B. W. Schmitz, G. K. Innes, S. M. Prasek, K. M. Pogreba Brown and E. R. Stark,
et al. COVID-19 containment on a college campus via wastewater-based epidemiology, targeted clinical testing and an intervention, Sci. Total Environ., 2021, 779, 146408 CrossRef CAS PubMed.
- M. Wolken, T. Sun, C. McCall, R. Schneider, K. Caton and C. Hundley,
et al. Wastewater surveillance of SARS-CoV-2 and influenza in preK-12 schools shows school, community, and citywide infections, Water Res., 2023, 231, 119648 CrossRef CAS PubMed.
- M. K. Wolfe, D. Duong, K. M. Bakker, M. Ammerman, L. Mortenson and B. Hughes,
et al. Wastewater-Based Detection of Two Influenza Outbreaks, Environ. Sci. Technol. Lett., 2022, 9(8), 687–692 CrossRef CAS.
- M. F. Espinosa, A. N. Sancho, L. M. Mendoza, C. R. Mota and M. E. Verbyla, Systematic review and meta-analysis of time-temperature pathogen inactivation, Int. J. Hyg. Environ. Health, 2020, 230, 113595 CrossRef CAS PubMed.
- Z. W. LaTurner, D. M. Zong, P. Kalvapalle, K. R. Gamas, A. Terwilliger and T. Crosby,
et al. Evaluating recovery, cost, and throughput of different concentration methods for SARS-CoV-2 wastewater-based epidemiology, Water Res., 2021, 197, 117043 CrossRef CAS PubMed.
- The dMIQE Group and J. F. Huggett, The Digital MIQE Guidelines Update: Minimum Information for Publication of Quantitative Digital PCR Experiments for 2020, Clin. Chem., 2020, 66(8), 1012–1029 CrossRef PubMed.
- J. Ye, G. Coulouris, I. Zaretskaya, I. Cutcutache, S. Rozen and T. L. Madden, Primer-BLAST: A tool to design target-specific primers for polymerase chain reaction, BMC Bioinf., 2012, 13(1), 134 CrossRef CAS PubMed.
- A. B. Boehm, M. K. Wolfe, B. J. White, B. Hughes, D. Duong, N. Banaei and A. Bidwell, Human norovirus (HuNoV) GII RNA in wastewater solids at 145 United States wastewater treatment plants: comparison to positivity rates of clinical specimens and modeled estimates of HuNoV GII shedders, J. Exposure Sci. Environ. Epidemiol., 2023 DOI:10.1038/s41370-023-00592-4.
- J. S. McClary-Gutierrez, Z. T. Aanderud, M. Al-faliti, C. Duvallet, R. Gonzalez and J. Guzman,
et al. Standardizing data reporting in the research community to enhance the utility of open data for SARS-CoV-2 wastewater surveillance, Environ. Sci.: Water Res. Technol., 2021, 7(9), 1545–1551 RSC.
-
L. B. Stadler, K. B. Ensor, J. R. Clark, P. Kalvapalle, Z. W. LaTurner and L. Mojica, et al., Wastewater Analysis of SARS-CoV-2 as a Predictive Metric of Positivity Rate for a Major Metropolis, medRxiv, 2020, preprint, DOI:10.1101/2020.11.04.20226191v1.
- J. Peccia, A. Zulli, D. E. Brackney, N. D. Grubaugh, E. H. Kaplan and A. Casanovas-Massana,
et al. Measurement of SARS-CoV-2 RNA in wastewater tracks community infection dynamics, Nat. Biotechnol., 2020, 38(10), 1164–1167 CrossRef CAS PubMed.
- G. Medema, L. Heijnen, G. Elsinga, R. Italiaander and A. Brouwer, Presence of SARS-Coronavirus-2 RNA in Sewage and Correlation with Reported COVID-19 Prevalence in the Early Stage of the Epidemic in The Netherlands, Environ. Sci. Technol. Lett., 2020, 7(7), 511–516 CrossRef CAS PubMed.
- Y. Cao, M. R. Raith and J. F. Griffith, Droplet digital PCR for simultaneous quantification of general and human-associated fecal indicators for water quality assessment, Water Res., 2015, 70, 337–349 CrossRef CAS PubMed.
- P. Mazumder, S. Dash, R. Honda, C. Sonne and M. Kumar, Sewage surveillance for SARS-CoV-2: Molecular detection, quantification, and normalization factors, Curr. Opin. Environ. Sci. Health., 2022, 28, 100363 CrossRef PubMed.
-
R. Gonzalez, A. Larson, H. Thompson, E. Carter and X. F. Cassi, Redesigning SARS-CoV-2 clinical RT-qPCR assays for wastewater RT-ddPCR, medRxiv, 2022, preprint, DOI:10.1101/2021.03.02.21252754v2.
- M. Ciesielski, D. Blackwood, T. Clerkin, R. Gonzalez, H. Thompson and A. Larson,
et al. Assessing sensitivity and reproducibility of RT-ddPCR and RT-qPCR for the quantification of SARS-CoV-2 in wastewater, J. Virol. Methods, 2021, 297, 114230 CrossRef CAS PubMed.
- P. M. D'Aoust, E. Mercier, D. Montpetit, J. J. Jia, I. Alexandrov and N. Neault,
et al. Quantitative analysis of SARS-CoV-2 RNA from wastewater solids in communities with low COVID-19 incidence and prevalence, Water Res., 2021, 188, 116560 CrossRef PubMed.
- L. Heijnen, G. Elsinga, M. de Graaf, R. Molenkamp, M. P. G. Koopmans and G. Medema, Droplet digital RT-PCR to detect SARS-CoV-2 signature mutations of variants of concern in wastewater, Sci. Total Environ., 2021, 799, 149456 CrossRef CAS PubMed.
-
A. Hinkle, H. D. Greenwald, M. Metzger, M. Thornton, L. C. Kennedy and K. Loomis, et al.Comparison of RT-qPCR and Digital PCR Methods for Wastewater-Based Testing of SARS-CoV-2, medRxiv, 2022, preprint, DOI:10.1101/2022.06.15.22276459v1.
- E. G. Lou, N. Sapoval, C. McCall, L. Bauhs, R. Carlson-Stadler and P. Kalvapalle,
et al. Direct comparison of RT-ddPCR and targeted amplicon sequencing for SARS-CoV-2 mutation monitoring in wastewater, Sci. Total Environ., 2022, 833, 155059 CrossRef CAS PubMed.
- A. S. Whale, J. F. Huggett and S. Tzonev, Fundamentals of multiplexing with digital PCR, Biomol. Detect. Quantif., 2016, 10, 15–23 CrossRef CAS PubMed.
- S. M. Ahmed, A. J. Hall, A. E. Robinson, L. Verhoef, P. Premkumar and U. D. Parashar,
et al. Global prevalence of norovirus in cases of gastroenteritis: a systematic review and meta-analysis, Lancet Infect. Dis., 2014, 14(8), 725–730 CrossRef PubMed.
- T. L. Wiemken, F. Khan, L. Puzniak, W. Yang, J. Simmering and P. Polgreen,
et al. Seasonal trends in COVID-19 cases, hospitalizations, and mortality in the United States and Europe, Sci. Rep., 2023, 13, 3886 CrossRef CAS PubMed.
- M. J. Hoogeveen and E. K. Hoogeveen, Comparable seasonal pattern for COVID-19 and flu-like illnesses, One Health, 2021, 13, 100277 CrossRef CAS.
- F. Miura, R. Matsuyama and H. Nishiura, Estimating the Asymptomatic Ratio of Norovirus Infection During Foodborne Outbreaks With Laboratory Testing in Japan, J. Epidemiol., 2018, 28(9), 382–387 CrossRef PubMed.
- V. Wiegering, J. Kaiser, D. Tappe, B. Weißbrich, H. Morbach and H. J. Girschick, Gastroenteritis in childhood: a retrospective study of 650 hospitalized pediatric patients, Int. J. Infect. Dis., 2011, 15(6), e401–e407 CrossRef PubMed.
- A. Guarino, J. Aguilar, J. Berkley, I. Broekaert, R. Vazquez-Frias and L. Holtz,
et al. Acute Gastroenteritis in Children of the World: What Needs to Be Done?, J. Pediatr. Gastroenterol. Nutr., 2020, 70(5), 694 CrossRef PubMed.
- B. A. Lopman, M. H. Reacher, I. B. Vipond, J. Sarangi and D. W. G. Brown, Clinical Manifestation of Norovirus Gastroenteritis in Health Care Settings, Clin. Infect. Dis., 2004, 39(3), 318–324 CrossRef PubMed.
- M. J. Conway, S. Kado, B. K. Kooienga, J. S. Sarette, M. H. Kirby and A. D. Marten,
et al. SARS-CoV-2 wastewater monitoring in rural and small metropolitan communities in Central Michigan, Sci. Total Environ., 2023, 894, 165013 CrossRef CAS PubMed.
-
C. Li, M. Bayati, S. Y. Hsu, H. Y. Hsieh, W. Lindsi and A. Belenchia, et al., Population Normalization in SARS-CoV-2 Wastewater-Based Epidemiology: Implications from Statewide Wastewater Monitoring in Missouri, medRxiv, 2022, preprint, DOI:10.1101/2022.09.08.22279459v1.
-
S. Y. Hsu, M. B. Bayati, C. Li, H. Y. Hsieh, A. Belenchia and J. Klutts, et al. Biomarkers Selection for Population Normalization in SARS-CoV-2 Wastewater-based Epidemiology, medRxiv, 2023, preprint, DOI:10.1101/2022.03.14.22272359.
- S. Feng, A. Roguet, J. S. McClary-Gutierrez, R. J. Newton, N. Kloczko and J. G. Meiman,
et al. Evaluation of Sampling, Analysis, and Normalization Methods for SARS-CoV-2 Concentrations in Wastewater to Assess COVID-19 Burdens in Wisconsin Communities, ACS ES&T Water, 2021, 1(8), 1955–1965 Search PubMed.
- National Wastewater Surveillance System, https://www.cdc.gov/nwss/testing.html, (Accessed June 2023).
- S. Karthikeyan, J. I. Levy, P. De Hoff, G. Humphrey, A. Birmingham and K. Jepsen,
et al. Wastewater sequencing reveals early cryptic SARS-CoV-2 variant transmission, Nature, 2022, 609(7925), 101–108 CrossRef CAS.
- R. Maal-Bared, Y. Qiu, Q. Li, T. Gao, S. E. Hrudey and S. Bhavanam,
et al. Does normalization of SARS-CoV-2 concentrations by Pepper Mild Mottle Virus improve correlations and lead time between wastewater surveillance and clinical data in Alberta (Canada): comparing twelve SARS-CoV-2 normalization approaches, Sci. Total Environ., 2023, 856, 158964 CrossRef CAS PubMed.
- Y. Qiu, J. Yu, K. Pabbaraju, B. E. Lee, T. Gao and N. J. Ashbolt,
et al. Validating and optimizing the method for molecular detection and quantification of SARS-CoV-2 in wastewater, Sci. Total Environ., 2022, 812, 151434 CrossRef CAS PubMed.
- T. Zhang, M. Breitbart, W. H. Lee, J. Q. Run, C. L. Wei and S. W. L. Soh,
et al. RNA Viral Community in Human Feces: Prevalence of Plant Pathogenic Viruses, PLoS Biol., 2005, 4(1), e3 CrossRef PubMed.
- M. Landstrom, E. Braun, E. Larson, M. Miller and G. H. Holm, Efficacy of SARS-CoV-2 wastewater surveillance for detection of COVID-19 at a residential private college, FEMS Microbes, 2022, 3, xtac008 CrossRef PubMed.
- J. Lee, N. Acosta, B. J. Waddell, K. Du, K. Xiang and J. Van Doorn,
et al. Campus node-based wastewater surveillance enables COVID-19 case localization and confirms lower SARS-CoV-2 burden relative to the surrounding community, Water Res., 2023, 244, 120469 CrossRef CAS PubMed.
- T. de Melo, G. Islam, D. B. D. Simmons, J. P. Desaulniers and A. E. Kirkwood, An alternative method for monitoring and interpreting influenza A in communities using wastewater surveillance, Front. Public Health, 2023, 11, 1141136 CrossRef PubMed.
- Z. Wu, D. Harrich, Z. Li, D. Hu and D. Li, The unique features of SARS-CoV-2 transmission: Comparison with SARS-CoV, MERS-CoV and 2009 H1N1 pandemic influenza virus, Rev. Med. Virol., 2021, 31(2), e2171 CrossRef CAS PubMed.
- E. Petersen, M. Koopmans, U. Go, D. H. Hamer, N. Petrosillo and F. Castelli,
et al. Comparing SARS-CoV-2 with SARS-CoV and influenza pandemics, Lancet Infect. Dis., 2020, 20(9), e238–e244 CrossRef CAS PubMed.
- F. Wu, A. Xiao, J. Zhang, K. Moniz, N. Endo and F. Armas,
et al. SARS-CoV-2 RNA concentrations in wastewater foreshadow dynamics and clinical presentation of new COVID-19 cases, Sci. Total Environ., 2022, 805, 150121 CrossRef CAS PubMed.
- A. L. Rainey, S. Liang, J. H. Bisesi Jr, T. Sabo-Attwood and A. T. Maurelli, A multistate assessment of population normalization factors for wastewater-based epidemiology of COVID-19, PLoS One, 2023, 18(4), e0284370 CrossRef CAS PubMed.
- S. Y. Hsu, M. Bayati, C. Li, H. Y. Hsieh, A. Belenchia and J. Klutts,
et al. Biomarkers selection for population normalization in SARS-CoV-2 wastewater-based epidemiology, Water Res., 2022, 223, 118985 CrossRef CAS PubMed.
-
Norovirus Infection, https://www.mayoclinic.org/diseases-conditions/norovirus/diagnosis-treatment/drc-20355302, (Accessed August 2023) Search PubMed.
- Norovirus Outbreak Closes Long Beach Elementary School, https://laist.com/news/health/norovirus-outbreak-closes-long-beach-elementary-school, (Accessed August 2023).
- CDC Yellow Book, https://wwwnc.cdc.gov/travel/yellowbook/2024/infections-diseases/norovirus, (Accessed August 2023).
- L. C. Scott, A. Aubee, L. Babahaji, K. Vigil, S. Tims and T. G. Aw, Targeted wastewater surveillance of SARS-CoV-2 on a university campus for COVID-19 outbreak detection and mitigation, Environ. Res., 2021, 200, 111374 CrossRef CAS PubMed.
- L. Parra-Arroyo, M. Martínez-Ruiz, S. Lucero, M. A. Oyervides-Muñoz, M. Wilkinson and E. M. Melchor-Martínez,
et al. Degradation of viral RNA in wastewater complex matrix models and other standards for wastewater-based epidemiology: A review, TrAC, Trends Anal. Chem., 2023, 158, 116890 CrossRef CAS.
- W. Ahmed, W. J. M. Smith, S. Metcalfe, G. Jackson, P. M. Choi and M. Morrison,
et al. Comparison of RT-qPCR and RT-dPCR Platforms for the Trace Detection of SARS-CoV-2 RNA in Wastewater, ACS ES&T Water, 2022, 2(11), 1871–1880 Search PubMed.
- M. Petala, M. Kostoglou, T. Karapantsios, C. I. Dovas, T. Lytras and D. Paraskevis,
et al. Relating SARS-CoV-2 shedding rate in wastewater to daily positive tests data: A consistent model based approach, Sci. Total Environ., 2022, 807, 150838 CrossRef CAS PubMed.
|
This journal is © The Royal Society of Chemistry 2025 |
Click here to see how this site uses Cookies. View our privacy policy here.