DOI:
10.1039/D4LC00835A
(Paper)
Lab Chip, 2025,
25, 1489-1501
A pumpless microfluidic co-culture system to model the effects of shear flow on biological barriers†
Received
3rd October 2024
, Accepted 14th January 2025
First published on 23rd January 2025
Abstract
Biological barriers formed by the endothelium and epithelium regulate nutrient exchange, disease development, and drug delivery. Organ-on-chip (OOC) systems effectively model these barriers by incorporating key biophysical cues like microscale dimensions, co-culture, and fluid flow-induced shear stress. The majority of microfluidic OOC platforms, however, require syringe and pump systems which are hindered by several limitations, including large footprints, elaborate designs, long setup times, and a high rate of failure (contamination, leakage, etc.). Here we describe VitroFlo, a pump-free microfluidic device designed for in vitro biological barrier modeling with 12 independent co-culture modules that can be simultaneously subjected to tunable, unidirectional flow with physiological shear stresses ranging from 0.01–10 dyn/cm2. We demonstrate application of the device to model vascular endothelial, blood–brain, and intestinal epithelial barriers, and confirm shear stress-dependent cell alignment, tight junction protein expression, barrier maturation, permeability, and paracrine signaling between co-cultured cells. The VitroFlo platform enables scalable and cost-effective modeling of physiological barriers to facilitate the translation of findings from in vitro systems to preclinical models.
Introduction
The use of in vitro cell systems to dissect complex physiological processes is instrumental in biomedical research and drug discovery. However, traditional in vitro models struggle to match the outcomes of preclinical models, in part due to the lack of key biophysical cues of the extracellular microenvironment in such systems. Fluid flow-induced shear stresses, in particular, are critical for biological barrier function as barrier-forming cells throughout the body – including the vasculature, blood–brain barrier and intestinal epithelium – are exposed to varying levels of shear stress, which modulate their maturation,1–3 homeostatic functions,4–7 barrier permeability,8–11 and immune cell adhesion and transmigration.12–14 For example, shear stresses of 4–20 dyn/cm2 cause endothelial cell alignment to direction of flow in the vasculature15–18 as well as increased expression of tight junction markers and barrier function in BBB models.9,19 Yet even perfusion level of fluid flow (<0.02 dyn/cm2) can be sufficient for inducing morphological changes in the barrier, such as cell differentiation and formation of villi structures in gut epithelial models.20,21 As these processes are central to basic physiological barrier function and drug responses, the greatest physiological fidelity will come from incorporation of shear stress into in vitro barrier models.
Traditionally, shear flow has been achieved in vitro using parallel plate flow chambers, cone and plate systems, and step flow chambers.15 While cells on these platforms recapitulate many of the typical shear responses observed in vivo, they have several notable limitations: they are often limited to single cell types, and have large footprints, single-sample throughput, a requirement for large cell and reagent volumes, and lengthy set ups that are cumbersome and involve external pumps, motors, and tubing. Microfluidic systems are well-suited for physiological barrier modeling and address some of the limitations of macroscale systems, including smaller sizes, reduced cell and reagent needs, increased scalability, and the ability to support multiple cell types in more physiologically relevant models. Accordingly, microfluidic-based organ-on-a-chip (OOC) systems have been developed to model biological barriers like the endothelium,10,22–29 blood brain barrier (BBB),19,30–34 and intestinal epithelium.20,35–40 For broader discussion of OOC biological barrier modeling the reader is referred to recent review articles.41–43
Typical microfluidic systems that incorporate shear flow for improved barrier modeling are small and cost-effective. However, these systems rely on syringe or peristaltic pump systems to deliver shear flow, increasing their footprint. Pumpless devices have been developed to mitigate this challenge,44–60 yet are limited by small media reservoirs which require frequent media changes; the inclusion of polydimethyl siloxane (PDMS), known to absorb small molecules; low throughput; or lack of unidirectional flow capabilities. Zhang et al. recently developed a polystyrene-based platform which addresses many of these concerns, enabling medium throughput culture of cells in a parallel channel configuration with unidirectional flow.58 However, such configurations necessitate the use of imaging to assess permeability, and may also limit sample collection.43
Herein, we describe the design and development of the VitroFlo device, a pumpless microfluidic system that uses gravity to deliver a dynamic range of physiological shear stresses (0.01–10 dyn/cm2). The VitroFlo device consists of 12 independent modules that can be investigated simultaneously in single culture or co-culture formats, including a membrane-base configuration, that enables assessment of barrier function using standard molecular permeability assays. The VitroFlo device utilizes a plate rocker with programmable angular velocity to produce constant forward flow through a small channel where cells are grown. During the reverse motion, media is routed through a backflow channel that minimizes the backflow of media over the cells, resulting in near-constant, unidirectional shear flow. Importantly, we demonstrate that the VitroFlo device can support cell growth and robust cell barrier function across a variety of physiological barrier models, including the vascular endothelium, the BBB, and the intestinal epithelium.
Results and discussion
VitroFlo design and function
The VitroFlo device is a uniquely designed microfluidic device that can generate up to 12 dyn/cm2 of unidirectional shear flow using a programmable plate rocker. The layout of the device allows for 12 modules to be fitted into a plate that has the same width and length as a standard tissue culture plate (Fig. 1A and S1†), and also facilitates imaging by microscopy through a thin, transparent layer of cyclic olefin copolymer (COC). Each module consists of two media reservoirs (top and bottom) and a fluidic channel (l = 20.0 mm, w = 2.0 mm, h = 0.28 mm) where cells are cultured. A standard well plate lid covers the reservoirs to prevent contamination while allowing gas exchange (Fig. S1†). The channels are defined by laser-cut tape layer sheets, which can be placed directly between the reservoir frame and COC creating a “2D device”, or assembled with a porous PET membrane to generate a “3D device” (Fig. 1B). As the plate is rocked forward, media from the top reservoir flows through the channel, exposing cells within the channel to a desired fluid shear determined by the angle of the programmable plate rocker. In 3D devices, cells within the bottom channel below the PET membrane are fed by the bottom media reservoir. The addition of a backflow wall and backflow channel within the VitroFlo device ensures that nearly unidirectional flow is maintained through the main channel where cells are grown (Fig. 1C–E); while the VitroFlo device is tilted forward (Fig. 1F), the height of the backflow wall prevents media flow through the backflow channel, directing fluid flow through the channel where cells are grown. During the reverse motion (Fig. 1G), the accumulated media flows with low resistance through the backflow channel and over the backflow wall, minimizing the backflow of media through the channel and shear experienced by the cells.
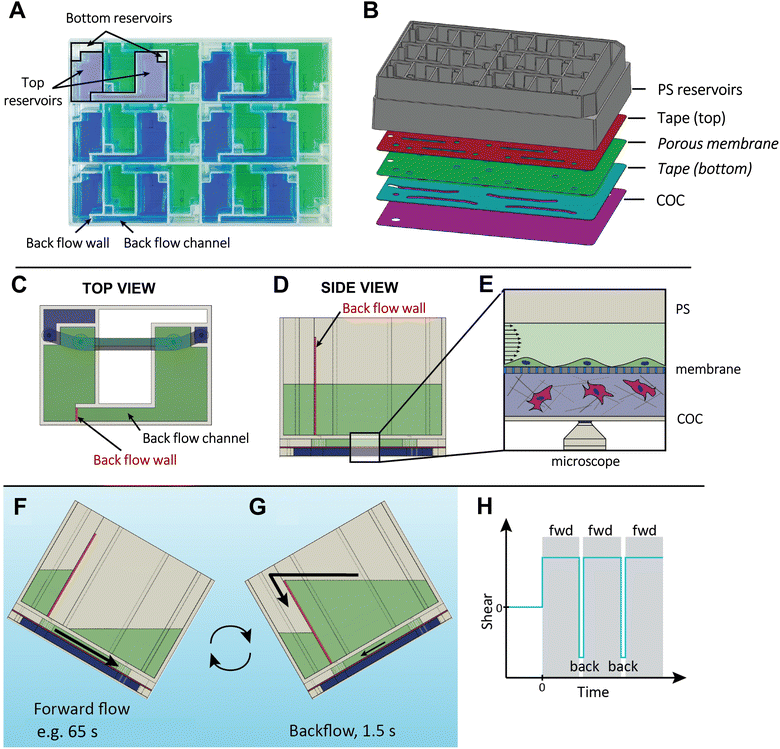 |
| Fig. 1 Overview of VitroFlo design and function. (A) Top view of VitroFlo plate, containing 12 modules each comprising top and bottom reservoirs, and cell culture channel(s). (B) Assembly of 3D device using polystyrene (PS) reservoirs, laser-cut tape layers, porous membrane and cyclic olefin copolymer (COC) capping layer. 2D devices are assembled similarly, excluding the membrane and bottom tape layer tape (italic). (C) Top view of upper reservoirs (green) with 3 mL volume each (combined 6 mL volume), and bottom reservoirs (blue) with 0.80 mL volume. (D) Top reservoirs feature a backflow channel and backflow wall, which enable re-circulating uni-directional fluid flow. (E) Cross-section view of cell culture channels separated by porous membrane in 3D device, with cells cultured on porous membrane in the top channel subjected to fluid flow. (F) Using a programmable plate rocker, uni-directional laminar flow is generated within the cell culture channel through tilting the plate over a programmable length of time (e.g., 65 s for 10 dyn/cm2) with cells experiencing constant shear and media collecting into the recipient upper reservoir. (G) Upon reaching the end of the range of motion, the rocker tilts back to the starting position over 1.5 s, during which media flows back to the donor upper reservoir through the backflow channel, minimizing back flow through the cell culture channel. (H) Graphical representation of shear flow force experienced by cells in top cell culture channel during rocking protocol; fwd = forward. | |
The magnitude of fluid shear experienced by cells is determined by the angle velocity of the programmable plate rocker that tilts the VitroFlo device at a specified angle and time duration, generating a nominal shear stress of 0.01–10 dyn/cm2 (Fig. 1F; Tables S1–S3; methodological details in ESI†). Once the end of the forward motion is reached, the rocker reverse-tilts rapidly, causing the accumulated fluid to flow back into the donor top reservoir (towards the left) through the backflow channel (Fig. 1G). This backflow motion lasts for 1.5 seconds and is designed to minimize the backflow of media through the channel where the cells are grown. This results in the cells experiencing a target shear flow over a defined period of time, e.g., for 65 seconds for 10 dyn/cm2, with a brief interruption of 1.5 seconds before resuming flow (Fig. 1H). Additionally, the plate rocker can be programmed to generate oscillatory (or bidirectional) flow depending on the desired application.
Flow rates experienced by cells was determined experimentally by analyzing individual frames captured from videos of plates rocking at different speeds, from which shear stresses were estimated (detailed methods in ESI†). Briefly, the flow rate in the channel was calculated by measuring the difference in height (dh) of the fluid between the two chambers (Fig. 2A–C and S2 and S3†), from which the wall shear stress across the floor was calculated. Using this approach, we verified shear flow using different rocker programs, including 1.5 dyn/cm2 (Fig. 2D and S4†), 3 dyn/cm2 (Fig. S5†), 5 dyn/cm2 (Fig. S6†), and 10 dyn/cm2 (Fig. 2E and S7†). This confirmed that forward flow was sustained for the duration of the forward cycle at the specified fluid shear, with the reverse cycle generating a reversal of flow for approximately 1 second. Measurement of volumes in multiple modules on a single plate confirmed consistent flow rates between modules (7.3% coefficient of variation). Wall shear stress was modelled along and across the channel, revealing uniform stress distribution during operation (Fig. 2F).
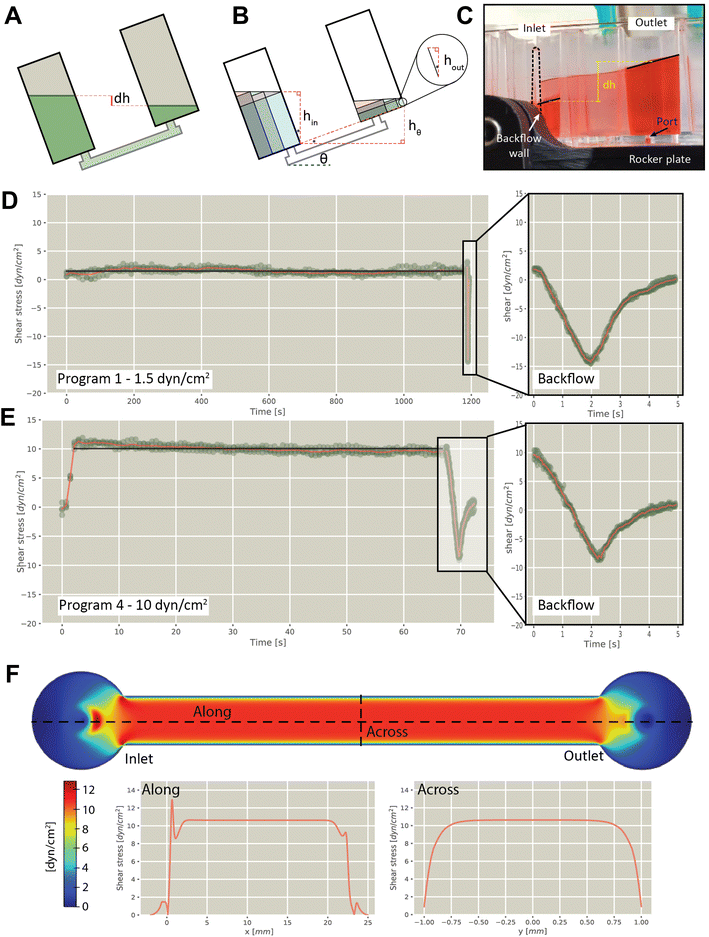 |
| Fig. 2 Evaluation of flow characteristics in the VitroFlo plate. (A) Schematic showing height difference (dh) between inlet and outlet reservoirs driving flow, created by tilting the device. (B) Reservoir volume divided into composite volumes to derive expression of dh as function of inlet volume and angle. (C) Photograph showing side view of VitroFlo device during back rocking cycle. (D) Experimentally determined shear stress for rocker program 1 (1.5 dyn/cm2; 20 min forward cycle) and (E) rocker program 4 (10 dyn/cm2; 65 s forward cycle). Symbols (green) show experimentally determined values, red line shows an average as a function of time and black line shows the plateau average. In the insets with the backflow data, the time scales are relative to the initiation of backflow. (F) Shear stress distribution in channel, as determined using COMSOL modeling. | |
Endothelial cell alignment in response to fluid shear
As a proof of concept, we first modelled the endothelium in the VitroFlo platform using human umbilical vein endothelial cells (HUVECs) (Fig. 3A). HUVECs were seeded in top channels onto the porous membranes, and either grown in static conditions or exposed to shear flow (10 dyn/cm2) for 96 hours. In both conditions, HUVECs formed fully confluent monolayers; however, HUVECs exposed to shear flow had a more elongated appearance after 96 hours compared to HUVECs cultured in static conditions (Fig. 3B). Cell–cell adhesions were visualized by immunostaining for VE-cadherin and alignment with respect to the direction of flow was quantified using the trainable WEKA segmentation plugin on ImageJ software (Fig. 3C and D). Whereas HUVECs grown in static conditions aligned randomly, application of shear stress resulted in 87% of HUVECs aligning within 30° of the flow direction (Fig. 3C) and a significant increase in aspect ratio, indicating elongation of cells in the direction of flow compared with static conditions (Fig. 3D; p < 0.05). These findings are consistent with previous reports showing increased cell alignment with the direction of flow in traditional flow systems,12,15,16,61
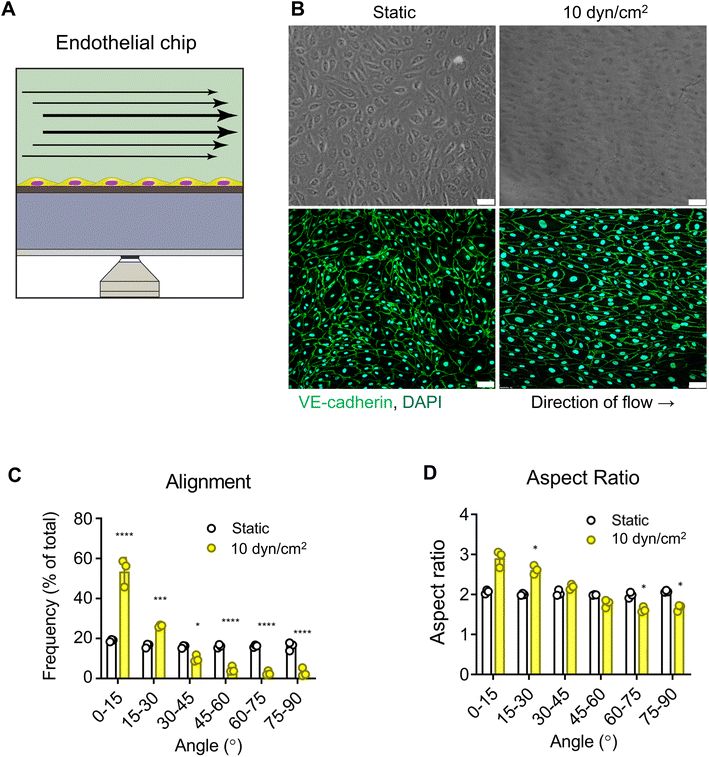 |
| Fig. 3 Endothelial barrier alignment in response to fluid shear. (A) Schematic of endothelial barrier model, with HUVEC grown to confluence in top channel of the 3D device (3 μm pore membrane) and subjected to 10 dyn/cm2 fluid shear for 96 h. (B) Brightfield (top) and confocal imaging (bottom) of cells immunostained for VE-cadherin (green), comparing static control and flow conditions. Scale bar = 50 μm. (C) Cell alignment evaluated for static and flow conditions using Trainable Weka Segmentation plugin in ImageJ, expressed as percentage of aligned cells, with 0° representing alignment parallel to the direction of fluid flow. (D) Cell elongation in the direction of flow was also measured and expressed as average aspect ratio. Data are mean ± SEM. **** p < 0.0001, *** p < 0.001, * p < 0.05. | |
Effect of shear flow and inflammation on endothelial barrier function
The design of the VitroFlo device enables cells to be cultured on a porous membrane for efficient assessment of barrier function in parallel with other downstream applications; using the device, we determined both endothelial monolayer integrity and barrier function using the same culture samples. To assess barrier function of HUVEC monolayers in the VitroFlo device, we performed FITC–dextran permeability assays on HUVECs exposed to shear flow (10 dyn/cm2) or static conditions for 96 hours (Fig. 4A). FITC–dextran was added to the top (donor) channel and samples were collected from the bottom (recipient) channel after 1 hour. Changes in FITC–dextran concentration over time, indicative of barrier function, were used to calculate the apparent permeability coefficient (Papp). Consistent with previous studies,23,62 cells exposed to shear flow had significantly decreased Papp compared to cells grown in static conditions, indicative of increased barrier function (Fig. 4B). Beyond increased barrier function in normal culture conditions, endothelial cells subjected to flow have also been shown to have increased protection against inflammation-induced barrier disruption.26,63–65 To test the protective effect of flow on HUVECs, we treated HUVEC monolayers that were cultured under static or flow conditions with TNF-α to induce inflammation, followed by co-culture with THP-1 monocytes and assessment of both monocyte cell adhesion and transmigration (Fig. 4C–F). THP-1 cells pre-labeled with fluorescent nuclear stain Hoechst-33342 were allowed to adhere for 1 hour onto HUVEC monolayers grown in 2D devices (Fig. 4C), and then counted and expressed as cells per unit area for all conditions. TNF-α treatment significantly increased THP-1 attachment onto HUVEC monolayers under static conditions but not in flow-conditioned HUVECs (Fig. 4D). Transmigration was assessed by inducing pre-labeled THP-1 cells to transmigrate through HUVEC monolayers grown on membranes in 3D devices, with transmigration quantified as cells per unit area after overnight incubation (Fig. 4E). Transmigration was significantly increased in TNF-α-treated monolayers under static conditions, but not in flow-conditioned HUVECs (Fig. 4F). Together, these data suggest that flow-conditioned HUVECs were protected from inflammation-induced monocyte attachment and transmigration.
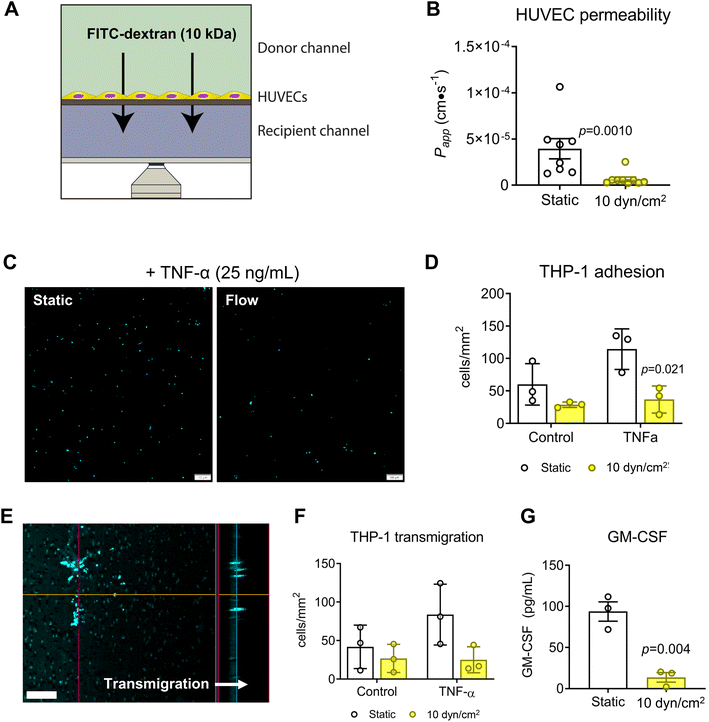 |
| Fig. 4 Effect of shear flow and inflammation on endothelial barrier function. HUVECs were seeded onto fibronectin-coated 3.0 μm pore-size PET membrane and exposed to static or flow (10 dyn/cm2) conditions for 96 hours. (A) Barrier function was assessed over 1 hour by molecular permeability assay using 10 kDa FITC–dextran in 3D devices, and (B) permeability coefficient (Papp) was calculated for monolayers under static and flow conditions. (C) THP-1 adhesion assay was performed after 96 hours in static or flow conditions following 4 hour treatment with 25 ng/mL TNF-α in 2D devices. THP-1 cells pre-labeled with Hoechst 33342 were added to endothelial monolayers and allowed to adhere for 1 hour. Scale bar = 100 μm. (D) THP-1 cell adhesion was quantified and expressed as number of cells per unit area. (E) Transmigration of pre-labeled THP-1 cells was assessed in 3D devices for control or TNF-α-treated HUVEC monolayers by adding 500 ng/mL MCP-1 to the recipient channel using confocal microscopy (scale bar = 50 μm) and (F) quantifying number of cells per unit area. (G) Secreted GM-CSF was assessed by ELISA for static vs. flow conditions. Data are mean ± SEM. | |
As the VitroFlo plate recirculates medium, the volume required is relatively low, even for higher flow rates, compared to what would be required for a syringe pump set up, for example. As such, factors secreted into the medium can accumulate and reach detectable concentrations. To demonstrate this capability, we measured secreted granulocyte-macrophage colony-stimulating factor (GM-CSF) in the conditioned medium by ELISA. Consistent with the monocyte adhesion and transmigration assays, levels of the pro-inflammatory cytokine GM-CSF were lower in HUVECs exposed to flow compared to static culture (Fig. 4G). Overall, these results indicate that endothelial cells exposed to fluid shear within the VitroFlo platform display increased barrier function and protection from inflammation, comparable to cells grown using traditional pump and syringe systems, but with greater ease of use.
Modeling the blood–brain barrier (BBB) response to shear flow
Next, we assessed the platform's capability for modeling the BBB by seeding HBMECs and astrocytes (ACs) onto opposite sides of a 0.4 μm-pore PET membrane (Fig. 5A). Confocal micrographs of monolayers showed that HBMECs exposed to shear flow (10 dyn/cm2 for 8 days) had cobblestone morphology, and increased ZO-1 expression at the cell peripheries compared to static condition (Fig. 5B and E). The lack of cell alignment (Fig. 5C) is corroborated by results in the literature where application of fluid shear had a range from no effect66 to both parallel and perpendicular cell alignment in studies using brain endothelial cells;67,68 there is also evidence to suggest that brain endothelial cells are inherently more resistant to cell elongation and alignment than peripheral endothelial cells.66 Nevertheless, there was a trend towards improved barrier function (Fig. 5D) consistent with the significant increase in ZO-1 expression at cell–cell junctions.
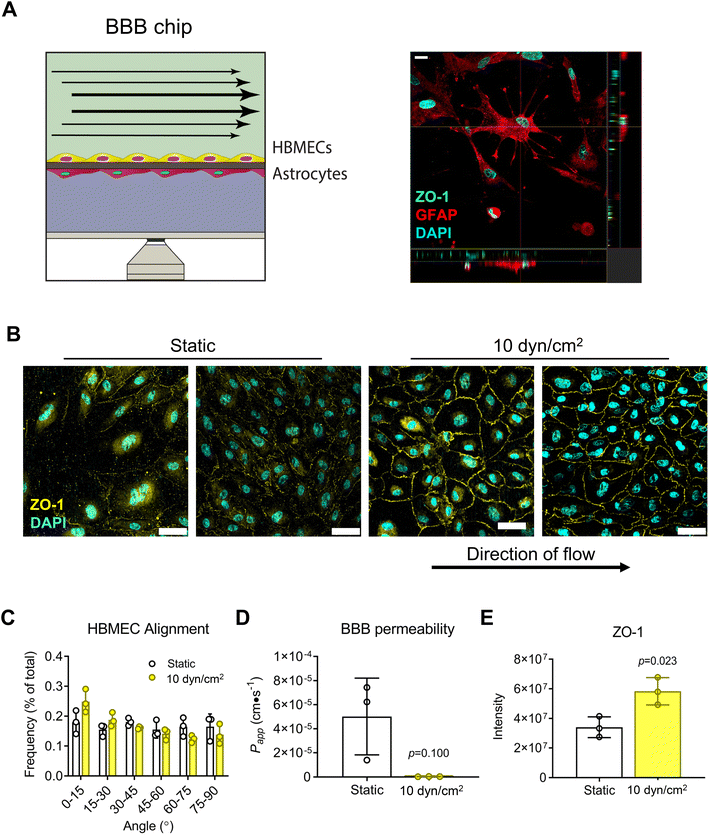 |
| Fig. 5 Blood–brain barrier (BBB) response to shear flow. (A) BBB chip with HBMECs and primary human astrocytes seeded on opposite sides of a 0.4 μm pore PET membrane, as confirmed by immunostaining for ZO-1 (green) and GFAP (red) for HBMECs and astrocytes, respectively. Scale bar = 20 μm. (B) Cells were cultured until HBMECs reached confluence, then exposed to static or flow (10 dyn/cm2) conditions for 8 days. HBMEC cell borders were visualized by immunostaining for ZO-1 (yellow), and (C) resulting confocal images used to quantify cell alignment. Scale bar = 50 μm. (D) Barrier function was assessed by FITC–dextran permeability assay and (E) ZO-1 immunostaining intensity quantification for flow vs. static control conditions. Data are mean ± SEM. | |
Modeling the intestinal epithelial barrier under perfusion
To model the intestinal epithelium, we used the Caco-2 cell line, a common cell line to model the intestinal epithelium in vitro.20,35,37,69,70 Caco-2 cells were seeded onto collagen I-coated 1.0 μm pore PET membranes and cultured under static or flow conditions for 14 days (Fig. 6A). A shear flow of 0.02 dyn/cm2 was applied (Table S3†) which is consistent with other intestinal epithelial models.20,37 Intestinal epithelial monolayer differentiation and integrity was assessed by immunostaining Caco-2 cells for tight-junction marker ZO-1 and differentiation marker mucin after 14 day culture under static or flow conditions (Fig. 6B). Caco-2 cells exposed to flow were more densely packed and had increased ZO-1 expression in contrast to cells grown in static culture (Fig. 6B). Muc-2 staining was significantly increased with flow suggesting increased differentiation (Fig. 6C). Another indicator of intestinal epithelial differentiation is an increase in cell height and the appearance of invaginations resulting from mechanosensitive cellular differentiation during the early stages of villus formation.2,20,35,37,71 Consistent with previous studies, Caco-2 cells grown in VitroFlo devices began to form early invaginations and acquire a columnar morphology with increased cell height in response to low shear flow (Fig. 7A and B). Furthermore, Caco-2 cells exposed to flow had significantly decreased Papp, consistent with increased barrier function (Fig. 7C). Together these data demonstrate enhanced intestinal epithelial barrier function in Caco-2 cells exposed to low shear flow (0.02 dyn/cm2) for 14 days in the VitroFlo device.
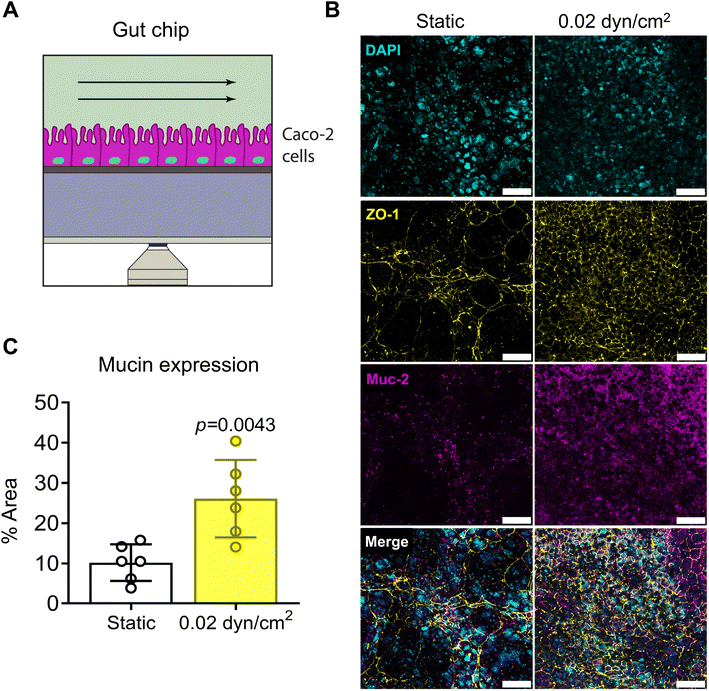 |
| Fig. 6 Gut barrier model in VitroFlo platform. (A) Schematic of gut barrier chip. Caco-2 cells seeded on collagen-coated porous PET membrane (1 μm pore-size) and cultured until confluent, then exposed to fluid flow (0.02 dyn/cm2) for 14 days. (B) Tight junction formation and Caco-2 differentiation assessed by immunostaining for ZO-1 and Muc-2, respectively. Scale bar = 50 μm. (C) Mucin expression was quantified as percentage of total image area for static and flow conditions. Data are mean ± SEM. | |
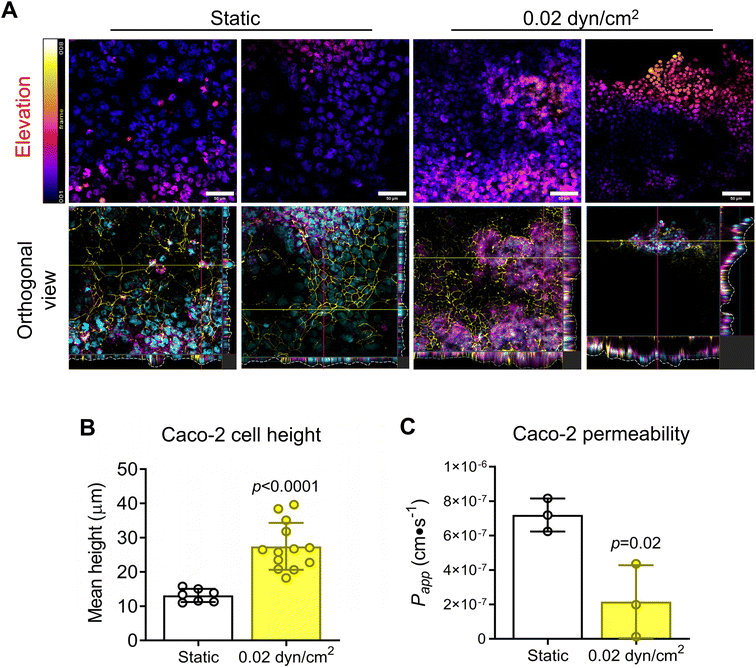 |
| Fig. 7 Gut barrier differentiation under flow. (A) Caco-2 cells seeded on collagen-coated porous PET membrane (1 μm pore-size), cultured until confluent, then exposed to fluid flow (0.02 dyn/cm2) for 14 days. Caco-2 cells were immunostained for ZO-1 (yellow), mucin (magenta) and nuclei visualized with DAPI (cyan). Confocal microscopy z-stacks were captured to analyze Caco-2 cell height and formation of invaginations in the cell layer. Scale bar = 50 μm. (B) Caco-2 cell height and (C) FITC–dextran permeability assay for flow vs. static control conditions. Data are mean ± SEM. | |
Conclusion
The VitroFlo platform enables facile physiological barrier modeling in vitro by incorporating co-culture of multiple cells types and physiological shear stress. Compared with traditional microfluidic barrier models, VitroFlo increases throughput, facilitates imaging and secreted protein analyses, and avoids the complexities and burden of pumps to generate fluid flow. Vascular, BBB, and intestinal barriers cultured on VitroFlo under physiological shear stresses showed enhanced tight junction formation, decreased permeability and inflammation, and barrier maturation compared to cells cultured on non-physiological static conditions. The ability of VitroFlo to effectively model a variety of physiological barriers in vitro without the use of elaborate equipment makes it a user-friendly, scalable, and cost-effective system with potential to improve knowledge translation from in vitro to preclinical models.
Methods
VitroFlo fabrication
The VitroFlo plate (Fig. 1 and S1†) has 12 modules comprised of top and bottom reservoirs connected by a channel where cells are cultured. It is fabricated from: an injection molded polystyrene (PS) top piece containing the reservoir architecture along with the backflow channel geometry (in-house design); two layers of double-sided tape (AR90106 NB, Adhesives Research Inc., Glen Rock, PA, USA); a porous membrane with either 0.4 μm (Sterlitech, Kent, WA, USA), 1 μm, or 3 μm diameter (IT4IP, Louvain-la-Neuve, Belgium) pores at a density of 2.0 × 106 pores per cm2; and a cyclic olefin copolymer (COC) bottom capping layer. To fabricate the complete system, two layers of double-sided tape are adhered to each other. The two tape layers are run through a laminator to ensure good adhesion and minimize air bubbles. Channel designs for top or bottom channels are laser cut (VLS350, Universal Laser Systems, Inc., Scottsdale, AZ, USA) in the tape layers. For assembly of the 3D version of the device, the top PS piece and the bottom COC capping layer are treated with an O2 plasma for 5 min (Harrick Plasma, Ithaca, NY, USA). The bottom layer double sided tape is then adhered to the COC capping layer and a roller is used to squeeze out any trapped air. The porous membrane is then stretched out on a table, attached to the bottom tape layer (with COC capping layer) trimmed to size, and rolled to remove any trapped air. The top tape layer is then attached to the bottom of the polystyrene top piece, rolled out, and then attached to the membrane. The VitroFlo device can also be assembled without a porous membrane or bottom tape layer if only 2D culture is required. Finally, the completed devices are left under vacuum over night to remove any residual air.
Shear stress finite element modeling
Finite element modeling of the shear stress profile in the VitroFlo plates was performed using COMSOL Multiphysics (version 5.5, COMSOL Inc., Burlington, MA, USA). A stationary laminar flow model with 229
473 domain mesh elements was used for the modelling and numerical simulations. The velocity profile was determined by solving the Navier–Stokes equation under stationary condition with a pressure defined by empirical measurements.
Cell culture
Cells were maintained at 37 °C, 5% CO2 and passaged according to supplier's instructions. Human umbilical vein cells (HUVECs; CC-2517; Lonza, Basel, CH) were cultured in VascuLife EnGS Endothelial media (LL-0002; Lifeline Cell Technology, Frederick, MD), and used at passages 2–5. Caco-2 cells (C2BBe1 clone, provided by Dr. Martha Brown, University of Toronto) were maintained in DMEM (11995065, Thermo Fisher Scientific) supplemented with 10% FBS (12483-020, Thermo Fisher Scientific), 1% penicillin–streptomycin (15140-122, Thermo Fisher Scientific), and 0.01 mg/mL human transferrin (T8158; Sigma Aldrich, St. Louis, MO). Primary human astrocytes (N7805100, Thermo Fisher Scientific) were cultured DMEM (10569-010, Thermo Fisher Scientific) supplemented with N-2 supplement (17502-048, Thermo Fisher Scientific) and 10% FBS. Immortalized human brain microvascular endothelial cells (HBMECs) (provided by Dr. Tara Moriarty, University of Toronto)72 were cultured in VascuLife VEGF-MV media (LL-0005; Lifeline Cell Technology). THP-1 monocytes were maintained in RPMI media (R8758; Sigma-Aldrich) supplemented with 10% FBS.
Endothelial barrier model
To model endothelial barriers, 3D VitroFlo devices with 3 μm-pore membranes were used. The topside of the membrane was coated with 1 mg/mL fibronectin (F1141; Sigma-Aldrich) for 1 hour at 37 °C. HUVECs were seeded onto fibronectin-coated surfaces at a seeding density of 50
000 cells/cm2 and cultured until confluent. Once confluent, cells were maintained at static or rocking conditions (10 dyn/cm2) for 96 hours with daily media changes. After 96 hours, a permeability assay was performed, as described below. Cells were then fixed with ice-cold 100% methanol for 10 minutes, and immunostained for appropriate markers. To assess secreted granulocyte macrophage-colony stimulating factor (GM-CSF) from HUVECs cultured under static or rocking conditions, media was collected during the last 48 hours and GM-CSF was assessed by ELISA (KHC2011; Thermo Fischer Scientific, Waltham, MA).
Endothelial cell alignment
HUVECs were immunostained for VE-cadherin in order to visualize cell borders (ab33168; Cambridge, UK). Confocal microscopy images were captured (3–5 images per channel at 10x magnification, covering approximately 50% of the channel area) from three biological replicates to analyze HUVEC alignment. Cell borders were detected using the Trainable Waikato Environment for Knowledge Analysis (WEKA) Segmentation plugin using ImageJ (National Institutes of Health, Bethesda, MD) as previously described.73 Briefly, the plugin is trained to identify cell borders for image segmentation into objects for analysis of cell counts aspect ratio along the horizontal axis (direction of flow), and degree of alignment with respect to the direction of flow.
Blood–brain barrier (BBB) model
To model the BBB, 3D VitroFlo devices with 0.4 μm-pore membranes were used. The underside of the membrane was coated with Geltrex™ (A1413301; Thermo Fischer Scientific), whereas the topside of the membrane was coated with 1 mg/mL fibronectin (F1141; Sigma-Aldrich). Primary human astrocytes (ACs) were seeded on the underside of the membrane at a seeding density of 50
000 cells/cm2 and allowed to attach for 1 hour. The devices were then flipped right side up and HBMECs were seeded on the topside of the membrane at a seeding density of 300
000 cells/cm2 and allowed to attach for 1 hour. HBMEC media was added to the top reservoirs (3 mL per reservoir for a combined 6 mL media), and AC media was added to the bottom reservoirs (0.8 mL media). Once confluent, cells were maintained under static or rocking conditions (10 dyn/cm2) for 96 hours, after which a permeability assay was performed, followed by methanol fixation for immunostaining.
Gut barrier model
To model the gut barrier, 3D VitroFlo devices with 1 μm pore PET membrane were used. The topside of the membranes was coated with 0.1 mg/mL with collagen type I (C7661; Sigma-Aldrich). Caco-2 cells were seeded at 20
000 cells/cm2 and cultured until confluent. Cells were then exposed to static conditions or fluid flow (0.02 dyn/cm2) for 14 days. This allowed to Caco-2 cells to become polarized and differentiated, as well as to form tight junctions. Cells were then fixed for immunostaining.
Permeability assay
Barrier permeability was assessed using 10 kDa FITC–dextran (FD10S; Sigma-Aldrich). Media from upper and lower reservoirs was aspirated and replaced with appropriate phenol-free media supplemented with FBS and pen–strep after 3 washes with PBS. At t = 0 minutes, FITC-Dextran was added to the top (donor) reservoirs at a final concentration of 50 ng/mL. The bottom reservoirs contained no FITC–dextran. 100 μL of media were sampled from the bottom (recipient) channel immediately. Plates were then placed back at 37 °C for 1 hour after which 100 μL was sampled from the bottom (recipient) channel again. A standard curve was generated by serial dilutions of 50 ng/mL FITC–dextran. Fluorescence intensity was measured using the Envision 2104 Multilabel Plate Reader (Perkin Elmer, Waltham, MA). The permeability coefficient (Papp) was calculated as10 |  | (1) |
where ΔCb is the change in concentration in the recipient (bottom) channel, Vb is the volume of the bottom channel, Ci is the starting concentration in the donor (top) channel, Am is the area of the channel, and Δt is the change in time.
Monocyte adhesion and transmigration assay
HUVECs were cultured in 2D and 3D VitroFlo devices for monocyte adhesion and transmigration assays, respectively. Monocyte adhesion and transmigration assays were performed as previously described.20 Briefly, after 96 hours in static or rocking (10 dyn/cm2) conditions, media was collected, and replaced with media with or without 25 ng/mL TNF-α (PHC3015, Thermo Fisher Scientific) for 4 hours before collecting again. THP-1 cells were pre-labeled with 2 μg/mL Hoechst 33342 (B2261; Sigma-Aldrich) for 30 minutes and seeded on top of the HUVEC cells at a density of 1.0 × 106 cells/mL. For the adhesion assay, THP-1 cells were allowed to attach for 1 hour, then fixed in 100% ice-cold methanol for 10 minutes. For the transmigration assay, 500 ng/mL monocyte chemoattractant protein-1 (MCP-1; PHC1014, Thermo Fisher Scientific) was added to the bottom channel and cells were allowed to transmigrate for 16 hours, then fixed in methanol. Cells were imaged using the Olympus FV3000 confocal laser scanning microscope (Olympus, Tokyo, Japan).
Immunostaining
Cells were fixed with ice-cold 100% methanol for 10 minutes, washed three times with PBS containing Ca2+ and Mg2+, and blocked with 3% BSA (10735086001; Sigma-Aldrich) for 1 hour. Cells were stained with specific antibodies (1
:
100) overnight at 4 °C. After washing thrice with PBS, secondary antibodies (1
:
200) were added for 1 hour. Nuclei were stained with 1
:
1000 DAPI (62
248, Thermo Fisher Scientific) for 15 minutes. The following antibodies were used: rabbit polyclonal VE-cadherin (ab33168; Abcam); mouse monoclonal ZO-1 (33-9100, Thermo Fisher Scientific); rabbit polyclonal GFAP (ab7260; Abcam); mucin-2 (ab11197; Abcam). The following secondary antibodies were used: goat anti-rabbit IgG (H + L) Alexa Fluor 488 (A11008, Thermo Fisher Scientific); goat anti-mouse IgG (H + L) Alexa Fluor 488 (A32723, Thermo Fisher Scientific); goat-anti rabbit IgG (H + L) Alexa Fluor 568 (A11036, Thermo Fisher Scientific). Image acquisition was performed using an Olympus FV3000 confocal laser scanning microscope. Image processing and analysis was performed using Olympus CellSens Software (Olympus, Tokyo, Japan).
Statistical analyses
Statistical analyses were performed using GraphPad Prism 10 Software (La Jolla, California, USA). Normality was determined by D'Agostino–Pearson omnibus test. Parametric and non-parametric analyses were performed as indicated in Figure legends. Data is presented as mean ± SEM.
Data availability
The data that support the findings of this study are available from the corresponding author upon reasonable request.
Conflicts of interest
The authors declare that a patent related to the work described in this manuscript has been awarded and assigned to the University of Toronto. The authors have no additional conflicts to declare.
Acknowledgements
This project was funded by the Natural Sciences and Engineering Research Council of Canada (NSERC DG RGPIN-2022-04375 and CRDPJ 531083-18), the Ontario Centre of Excellence (VIP II 29253), CellScale Biomaterials, and an NSERC CREATE Training Program in Organ-on-a-Chip Engineering and Entrepreneurship fellowship to AU. The graphical abstract figure was created in BioRender.
References
- G. D. Vatine, R. Barrile, M. J. Workman, S. Sances, B. K. Barriga, M. Rahnama, S. Barthakur, M. Kasendra, C. Lucchesi, J. Kerns, N. Wen, W. R. Spivia, Z. Chen, J. Van Eyk and C. N. Svendsen, Cell Stem Cell, 2019, 24, 995–1005.e6 CrossRef CAS PubMed
.
- M. Kasendra, R. Luc, J. Yin, D. V. Manatakis, G. Kulkarni, C. Lucchesi, J. Sliz, A. Apostolou, L. Sunuwar, J. Obrigewitch, K. J. Jang, G. A. Hamilton, M. Donowitz and K. Karalis, eLife, 2020, 9, e50135 CrossRef CAS
.
- A. Sontheimer-Phelps, D. B. Chou, A. Tovaglieri, T. C. Ferrante, T. Duckworth, C. Fadel, V. Frismantas, A. D. Sutherland, S. Jalili-Firoozinezhad, M. Kasendra, E. Stas, J. C. Weaver, C. A. Richmond, O. Levy, R. Prantil-Baun, D. T. Breault and D. E. Ingber, Cell. Mol. Gastroenterol. Hepatol., 2020, 9, 507–526 CrossRef PubMed
.
- M. Bongrazio, C. Baumann, A. Zakrzewicz, A. R. Pries and P. Gaehtgens, Cardiovasc. Res., 2000, 47, 384–393 CrossRef CAS PubMed
.
- F. Vozzi, F. Bianchi, A. Ahluwalia and C. Domenici, Biotechnol. J., 2014, 9, 146–154 CrossRef CAS
.
- S. C. Bischoff, G. Barbara, W. Buurman, T. Ockhuizen, J. Schulzke, M. Serino, H. Tilg, A. Watson and J. M. Wells, BMC Gastroenterol., 2014, 14, 189 CrossRef PubMed
.
- J. Yracheta, W. Muraoka, X. Wu, D. Burmeister, D. Darlington, D. Zhao, Z. Lai, S. Sayyadioskoie, A. P. Cap, J. Bynum and S. E. Nicholson, J. Trauma Acute Care Surg., 2021, 91, 1002–1009 CrossRef CAS PubMed
.
- A. Wallin, K. Blennow, P. Fredman, C. G. Gottfries, I. Karlsson and L. Svenner-holm, Acta Neurol. Scand., 1990, 81, 318–322 CrossRef CAS PubMed
.
- L. Cucullo, M. Hossain, V. Puvenna, N. Marchi and D. Janigro, BMC Neurosci., 2011, 12, 1–15 CrossRef PubMed
.
- J. F. Wong and C. A. Simmons, Lab Chip, 2019, 19, 1060–1070 RSC
.
- D. Ranadewa, J. Wu, V. A. Subramanianbalachandar and R. L. Steward, Cytoskeleton, 2021, 78, 323–334 CrossRef CAS PubMed
.
- M. P. Burns and N. DePaola, Am. J. Physiol., 2005, 288, H194–H204 CAS
.
- S. S. Dhawan, R. P. Avati Nanjundappa, J. R. Branch, W. R. Taylor, A. A. Quyyumi, H. Jo, M. C. Mcdaniel, J. Suo, W. H. Coulter, D. Giddens and H. Samady, Expert Rev. Cardiovasc. Ther., 2010, 8, 545–566 CrossRef PubMed
.
- K. M. Gray and K. M. Stroka, Semin. Cell Dev. Biol., 2017, 71, 106–117 CrossRef CAS PubMed
.
- C. Wang, H. Lu and M. Alexander Schwartz, J. Biomech., 2012, 45, 1212–1218 CrossRef PubMed
.
- J. G. DeStefano, A. Williams, A. Wnorowski, N. Yimam, P. C. Searson and A. D. Wong, Integr. Biol., 2017, 9, 362–374 CrossRef CAS PubMed
.
- Q. Smith, B. Macklin, X. Y. Chan, H. Jones, M. Trempel, M. C. Yoder and S. Gerecht, Cell Rep., 2018, 24, 895–908.e6 CrossRef CAS PubMed
.
- V. Velasco, P. Soucy, R. Keynton and S. J. Williams, Lab Chip, 2022, 22, 4705–4716 RSC
.
- T. E. Park, N. Mustafaoglu, A. Herland, R. Hasselkus, R. Mannix, E. A. FitzGerald, R. Prantil-Baun, A. Watters, O. Henry, M. Benz, H. Sanchez, H. J. McCrea, L. C. Goumnerova, H. W. Song, S. P. Palecek, E. Shusta and D. E. Ingber, Nat. Commun., 2019, 10, 2621 CrossRef PubMed
.
- H. J. Kim, D. Huh, G. Hamilton and D. E. Ingber, Lab Chip, 2012, 12, 2165–2174 RSC
.
- M. W. van der Helm, O. Y. F. Henry, A. Bein, T. Hamkins-Indik, M. J. Cronce, W. D. Leineweber, M. Odijk, A. D. van der Meer, L. I. Segerink and D. E. Ingber, Lab Chip, 2019, 19, 452–463 RSC
.
- J. W. Song, S. P. Cavnar, A. C. Walker, K. E. Luker, M. Gupta, Y. C. Tung, G. D. Luker and S. Takayama, PLoS One, 2009, 4, e5756 CrossRef PubMed
.
- E. W. K. Young and C. A. Simmons, Lab Chip, 2010, 10, 143–160 RSC
.
- S. Srigunapalan, C. Lam, A. R. Wheeler and C. A. Simmons, Biomicrofluidics, 2011, 5, 013409 CrossRef PubMed
.
- M. B. Chen, S. Srigunapalan, A. R. Wheeler and C. A. Simmons, Lab Chip, 2013, 13, 2591–2598 RSC
.
- R. Molteni, E. Bianchi, P. Patete, M. Fabbri, G. Baroni, G. Dubini and R. Pardi, Lab Chip, 2015, 15, 195–207 RSC
.
- M. Cirit and C. L. Stokes, Lab Chip, 2018, 18, 1831–1837 RSC
.
- H. Persson, S. Park, M. Mohan, K. K. Cheung, C. A. Simmons and E. W. K. Young, Sens. Actuators, B, 2022, 356, 131342 CrossRef CAS
.
- H. Ehlers, A. Nicolas, F. Schavemaker, J. P. M. Heijmans, M. Bulst, S. J. Trietsch and L. J. van den Broek, Front. Immunol., 2023, 14, 1–11 Search PubMed
.
- R. Booth and H. Kim, Lab Chip, 2012, 12, 1784–1792 RSC
.
- L. M. Griep, F. Wolbers, B. De Wagenaar, P. M. Ter Braak, B. B. Weksler, I. A. Romero, P. O. Couraud, I. Vermes, A. D. Van Der Meer and A. Van Den Berg, Biomed. Microdevices, 2013, 15, 145–150 CrossRef CAS PubMed
.
- M. Bonakdar, P. M. Graybill and R. V. Davalos, RSC Adv., 2017, 7, 42811–42818 RSC
.
- J. G. Destefano, J. J. Jamieson, R. M. Linville and P. C. Searson, Fluids Barriers CNS, 2018, 15, 32 CrossRef CAS PubMed
.
- I. Matthiesen, D. Voulgaris, P. Nikolakopoulou, T. E. Winkler and A. Herland, Small, 2021, 17, 2101785 CrossRef CAS PubMed
.
- K. Y. Shim, D. Lee, J. Han, N. T. Nguyen, S. Park and J. H. Sung, Biomed. Microdevices, 2017, 19, 37 CrossRef PubMed
.
- M. Kasendra, A. Tovaglieri, A. Sontheimer-Phelps, S. Jalili-Firoozinezhad, A. Bein, A. Chalkiadaki, W. Scholl, C. Zhang, H. Rickner, C. A. Richmond, H. Li, D. T. Breault and D. E. Ingber, Sci. Rep., 2018, 8, 1–14 CAS
.
- C. Beaurivage, E. Naumovska, Y. X. Chang, E. D. Elstak, A. Nicolas, H. Wouters, G. van Moolenbroek, H. L. Lanz, S. J. Trietsch, J. Joore, P. Vulto, R. A. J. Janssen, K. S. Erdmann, J. Stallen and D. Kurek, Int. J. Mol. Sci., 2019, 20, 5661 CrossRef CAS PubMed
.
- A. Tovaglieri, A. Sontheimer-Phelps, A. Geirnaert, R. Prantil-Baun, D. M. Camacho, D. B. Chou, S. Jalili-Firoozinezhad, T. De Wouters, M. Kasendra, M. Super, M. J. Cartwright, C. A. Richmond, D. T. Breault, C. Lacroix and D. E. Ingber, Microbiome, 2019, 7, 43 CrossRef PubMed
.
- S. Jalili-Firoozinezhad, F. S. Gazzaniga, E. L. Calamari, D. M. Camacho, C. W. Fadel, A. Bein, B. Swenor, B. Nestor, M. J. Cronce, A. Tovaglieri, O. Levy, K. E. Gregory, D. T. Breault, J. M. S. Cabral, D. L. Kasper, R. Novak and D. E. Ingber, Nat. Biomed. Eng., 2019, 3, 520–531 CrossRef CAS PubMed
.
- T. E. Winkler, M. Feil, E. F. G. J. Stronkman, I. Matthiesen and A. Herland, Lab Chip, 2020, 20, 1212–1226 RSC
.
- G. Simitian, M. Virumbrales-Muñoz, C. Sánchez-De-Diego, D. J. Beebe and D. Kosoff, Lab Chip, 2022, 22, 3618–3636 RSC
.
- C. M. Leung, P. de Haan, K. Ronaldson-Bouchard, G. A. Kim, J. Ko, H. S. Rho, Z. Chen, P. Habibovic, N. L. Jeon, S. Takayama, M. L. Shuler, G. Vunjak-Novakovic, O. Frey, E. Verpoorte and Y. C. Toh, Nat. Rev. Methods Primers, 2022, 2, 33 CrossRef CAS
.
- A. Ugodnikov, H. Persson and C. A. Simmons, Lab Chip, 2024, 24, 3199–3225 RSC
.
- H. E. Abaci, K. Gledhill, Z. Guo, A. M. Christiano and M. L. Shuler, Lab Chip, 2015, 15, 882–888 RSC
.
- P. G. Miller and M. L. Shuler, Biotechnol. Bioeng., 2016, 113, 2213–2227 CrossRef CAS PubMed
.
- S. J. Trietsch, E. Naumovska, D. Kurek, M. C. Setyawati, M. K. Vormann, K. J. Wilschut, H. L. Lanz, A. Nicolas, C. P. Ng, J. Joore, S. Kustermann, A. Roth, T. Hankemeier, A. Moisan and P. Vulto, Nat. Commun., 2017, 8, 1–7 CrossRef CAS PubMed
.
- H. Lee, D. S. Kim, S. K. Ha, I. Choi, J. M. Lee and J. H. Sung, Biotechnol. Bioeng., 2017, 114, 432–443 CrossRef CAS PubMed
.
- H. J. Chen, P. Miller and M. L. Shuler, Lab Chip, 2018, 18, 2036–2046 RSC
.
- Y. I. Wang and M. L. Shuler, Lab Chip, 2018, 18, 2563–2574 RSC
.
- C. Oleaga, A. Lavado, A. Riu, S. Rothemund, C. A. Carmona-Moran, K. Persaud, A. Yurko, J. Lear, N. S. Narasimhan, C. J. Long, F. Sommerhage, L. R. Bridges, Y. Cai, C. Martin, M. T. Schnepper, A. Goswami, R. Note, J. Langer, S. Teissier, J. Cotovio and J. J. Hickman, Adv. Funct. Mater., 2019, 29, 1–12 Search PubMed
.
- S. Rajasekar, D. S. Y. Lin, L. Abdul, A. Liu, A. Sotra, F. Zhang and B. Zhang, Adv. Mater., 2020, 32, 1–12 Search PubMed
.
- L. C. Delon, A. Nilghaz, E. Cheah, C. Prestidge and B. Thierry, Adv. Healthcare Mater., 2020, 9, 1–9 Search PubMed
.
- P. Fathi, G. Holland, D. Pan and M. B. Esch, ACS Appl. Bio Mater., 2020, 3, 6697–6707 CrossRef CAS PubMed
.
- Z. Chen, J. Zilberberg and W. Lee, Biomed. Microdevices, 2020, 22, 58 CrossRef CAS PubMed
.
- D. J. LaValley, P. G. Miller and M. L. Shuler, Biotechnol. Prog., 2021, 37, 1–15 CrossRef PubMed
.
- S. Y. Lee, H. J. Byun, H. Choi, J. I. Won, J. Han, S. Park, D. Kim and J. H. Sung, Biotechnol. Bioprocess Eng., 2022, 27, 221–233 CrossRef CAS
.
- N. Limjanthong, Y. Tohbaru, T. Okamoto, R. Okajima, Y. Kusama, H. Kojima, A. Fujimura, T. Miyazaki, T. Kanamori, S. Sugiura and K. Ohnuma, J. Biosci. Bioeng., 2023, 135, 151–159 CrossRef CAS PubMed
.
- F. Zhang, D. S. Y. Lin, S. Rajasekar, A. Sotra and B. Zhang, Adv. Healthcare Mater., 2023, 12, 1–12 Search PubMed
.
- D. Tian, Z. Mao, L. Wang, X. Huang, W. Wang, H. Luo, J. Peng and Y. Chen, Lab Chip, 2024, 24, 2561–2574 RSC
.
- Y. Yang, P. Fathi, G. Holland, D. Pan, N. S. Wang and M. B. Esch, Lab Chip, 2019, 19, 3212–3219 RSC
.
- R. Steward, D. Tambe, C. Corey Hardin, R. Krishnan and J. J. Fredberg, Am. J. Physiol., 2015, 308, C657–C664 CrossRef CAS PubMed
.
- J. Shao, L. Wu, J. Wu, Y. Zheng, H. Zhao, Q. Jin and J. Zhao, Lab Chip, 2009, 9, 3118–3125 RSC
.
- Y. S. Chatzizisis, A. U. Coskun, M. Jonas, E. R. Edelman, C. L. Feldman and P. H. Stone, J. Am. Coll. Cardiol., 2007, 49, 2379–2393 CrossRef CAS PubMed
.
- A. Babendreyer, L. Molls, D. Dreymueller, S. Uhlig and A. Ludwig, Mediators Inflammation, 2017, 2017, 1515389 Search PubMed
.
- S. Sheikh, G. E. Rainger, Z. Gale, M. Rahman and G. B. Nash, Blood, 2003, 102, 2828–2834 CrossRef CAS PubMed
.
- A. Reinitz, J. DeStefano, M. Ye, A. D. Wong and P. C. Searson, Microvasc. Res., 2015, 99, 8–18 CrossRef CAS PubMed
.
- N. Choublier, Y. Müller, L. Gomez Baisac, J. Laedermann, C. de Rham, X. Declèves and A. Roux, Appl. Sci., 2021, 11, 5584 CrossRef CAS
.
- N. Choublier, M. Taghi, M. C. Menet, M. Le Gall, J. Bruce, P. Chafey, F. Guillonneau, A. Moreau, C. Denizot, Y. Parmentier, S. Nakib, D. Borderie, H. Bouzinba-Segard, P. O. Couraud, S. Bourdoulous and X. Declèves, Fluids Barriers CNS, 2022, 19, 1–24 CrossRef PubMed
.
- L. Fossati, R. Dechaume, E. Hardillier, D. Chevillon, C. Prevost, S. Bolze and N. Maubon, Int. J. Pharm., 2008, 360, 148–155 CrossRef CAS PubMed
.
- M. Natoli, B. D. Leoni, I. D'Agnano, F. Zucco and A. Felsani, Toxicol. In Vitro, 2012, 26, 1243–1246 CrossRef CAS PubMed
.
- H. J. Kim, H. Li, J. J. Collins and D. E. Ingber, Proc. Natl. Acad. Sci. U. S. A., 2016, 113, E7–E15 CAS
.
-
A. E. Boczula, A. Ly, R. Ebady, J. Cho, Z. Anjum, N. Zlotnikov, H. Persson, T. Odisho, C. A. Simmons and T. J. Moriarty, bioRxiv, 2021, preprint, 2021.05.05.442761, DOI:10.1101/2021.05.05.442761.
- I. Arganda-Carreras, V. Kaynig, C. Rueden, K. W. Eliceiri, J. Schindelin, A. Cardona and H. S. Seung, Bioinformatics, 2017, 33, 2424–2426 CrossRef CAS PubMed
.
|
This journal is © The Royal Society of Chemistry 2025 |
Click here to see how this site uses Cookies. View our privacy policy here.