DOI:
10.1039/D4NH00281D
(Communication)
Nanoscale Horiz., 2025,
10, 124-134
A Blautia producta specific gFET-based aptasensor for quantitative monitoring of microbiome quality
Received
17th June 2024
, Accepted 26th September 2024
First published on 12th October 2024
Abstract
The use of health-relevant bacteria originating from human microbiomes for the control or therapy of diseases, including neurodegenerative disorders or diabetes, is currently gaining increasing importance in medicine. Directed and successful engineering of microbiomes via probiotic supplementation requires subtle, precise as well as, more importantly, easy, fast and convenient monitoring of its success, e.g., in patients’ gut. Based on a previously described polyclonal SELEX aptamer library evolved against the human gut bacterium Blautia producta, we finally isolated three individual aptamers that proved their performance concerning affinity, specificity and robustness in reliably labeling the target bacterium and in combination with “contaminating” control bacteria. Using biofunctionalization molecules on gFETs, we could specifically quantify 101–106 cells per mL, retrace their number in mixtures and determine aptamer Kd-values around 2 nM. These measurements were possible even in the context of a real human stool sample. Our results qualify gFETs in combination with BL2, BL7 and BL8 aptamers as a promising foundation for the construction of respective sensing devices, which will open new avenues towards developing an intended monitoring technique for probiotic therapy and microbiome engineering approaches.
New concepts
A specific gFET-based aptasensor for quantitative monitoring of microbiome quality: we introduce the adaptation of electronic gFET sensors functionalized with binding molecules of high specificity towards biomolecule targets for the precise quantitative measurement of bacterial cells comprising important inhabitants of the human intestinal microbiome. Aptamers in this concept serve as specific binding entities for the quantification of Blautia producta bacterial cells with high sensitivity and specificity in the complex background of stool samples containing up to 1000 different bacterial species. B. producta is only the first example of health-relevant bacteria that can be analysed using this simple and robust technology, and more specific sensors are currently being developed. Specific quantitative monitoring of the bacterial content belonging to individual species in human microbiomes with distinct roles in health and disease using (multiplexed) gFET sensors involving aptamers as nanotechnology-derived binding molecules has the potential to replace conventional sequencing of rRNA genes (next-generation sequencing) for the analysis and quantification of bacterial species in microbiomes. This will help in improving diagnostics and therapy in medicine and allow applications in environmental research.
|
Introduction
Blautia, with B. producta as the most prominent species, is a genus of strictly anaerobic health-relevant bacteria present in the human gut.1–3 This bacterium is highly abundant in the gut microbiome, and it has received increasing attention because it appears to be closely related to the occurrence of various diseases in the case of so-called dysbiosis.4–6 The systematic genus Blautia in general is a group of relatively abundant commensal bacteria in the gut of healthy humans, being in the hitlist of the top 10 most frequent genera as measured via NGS, accounting for 2–8% of bacterial cells in the gut microbiome.7–9 Species belonging to the Blautia genus have been described to play key roles in the digestion of otherwise nondegradable food constituents, such as special carbohydrates from dietary fiber, which metabolise into short-chain fatty acids (SCFAs) as important metabolic mediators between the gut microbiota and their hosts, functioning as fuels for gut epithelial tissues.1,10 Their high abundance in the gut and their physiological roles were important enough to name one of three major (so-called) enterotypes of human microbiomes after Blautia – or by their former genus name – Ruminococcus.8 When administered as a “probiotic”, B. producta was effective in protecting against acute liver damage induced by bacterial lipopolysaccharides (LPSs)11 and alleviating hyperlipidemia induced by a high-fat diet (HFD) through the upregulation of low-density lipoprotein receptor (LDLR) expression in mouse livers.12 Longitudinal studies have further demonstrated that the abundance of B. producta is significantly negatively correlated with the visceral fat area (VFA),13,14 and the relationship between glucose metabolism and gluconeogenesis in obese children was significantly reduced but significantly positively correlated with the abundance of B. producta in the gut.15 Moreover, high abundances of B. producta in the gut can significantly increase the amount of acetate, a free fatty acid receptor 2 (FFAR2) ligand, thereby regulating liver metabolism through the acetate-FFAR2 molecular circuit, which effectively inhibits the non-alcoholic liver disease/non-alcoholic steatohepatitis (NAFLD/NASH) phenotype.16,17 Another protective role of B. producta can be observed in directly or indirectly controlling microbiome composition and thus maintaining healthy and functional bacterial communities. For example, vancomycin-resistant strains of Enterococcus faecium (VRE) are the leading cause of hospital-acquired infections.18B. producta has been shown to effectively inhibit the colonization of VRE in the gut by secreting a lantibiotic.19,20 Thus, a technology allowing fast, easy, and regular quantitative monitoring of B. producta abundance in the gut may represent a diagnostic prerequisite enabling the prevention and treatment of various diseases resulting from dysbiosis in the future (Scheme 1).
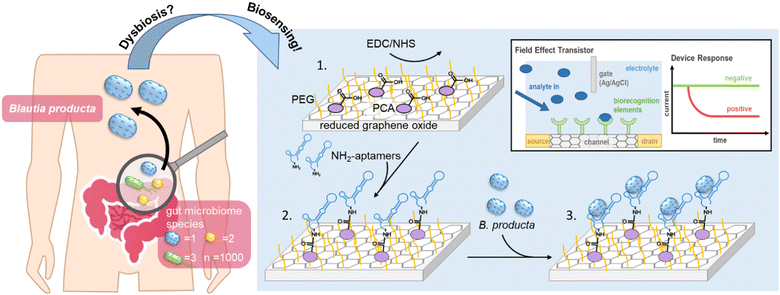 |
| Scheme 1 Human gut microbiome dysbiosis detection using aptamer-based biosensing. Functionalization of rGO-FET with anti-Blautia individual aptamers. (1) Chips were immersed in PyPEG (PEG pre-conjugated with a PBSE) (500 mM) and 1-pyrenecarboxylic acid (PCA, 50 mM, linker) to obtain a 9 : 1 ratio of blocking and linking agents on the reduced graphene surface. (2) 5′-NH2-modified aptamer (BL2, BL7, or BL8) immobilization by first activating the carboxyl groups using EDC (15 mM)/NHS (15 mM) for 30 min, followed by covalent coupling of 5′-NH2-modified aptamers (100 nM in milliQ grade water for 1 h at 25 °C). (3) Specific affinity recognition of B. producta cells. Inlay: typical configuration of an rGO-FET with a response of the device when there is no target applied (green) and when the target analyte is present (red). | |
Currently, the “gold-standard” method for quantitative microbiome analyses is so-called next-generation sequencing (NGS) technologies, which, however, are widely accepted to be laborious, require expensive machineries and chemistry, and are thus relatively slow and normally cost-intensive. Alternative approaches have been suggested, including detection methods based on aptamers as specific binding molecules that recognize bacteria as targets/analytes of high epitopic complexity.21–24 Aptamers are single-stranded oligonucleotides of DNA or RNA that can be enriched and selected in a molecular-directed evolution process called Systematic Evolution of Ligands by Exponential Enrichment (SELEX).25,26 In a variant of this process, we introduce it as the “FluCell-SELEX”27 focused (“polyclonal”) aptamer libraries are generated, which already allow quantitative measurements and effective discrimination of the dedicated target bacteria from other gut microbiome members in mixtures and also in stool samples. Such an aptamer library has been developed over 14 rounds of SELEX for B. producta, and the final library B.p-R14 proved its functionality to specifically label B. producta cells with fluorophores, enabling fluorometric and microscopic assays.28 Libraries of this type not only directly allow initial biotechnological applications but can also serve as a resource for the isolation of high-performance individual aptamers using bioinformatic methods.22,27
The concept of electrolyte-gated field-effect transistors (EG-FETs) in electronic devices has been used previously in biosensing. It has been described that this technique allows for the detection of cancer markers,29 microRNA,30 DNA as a biomarker in certain heart diseases,31,32 and small molecules, such as biotin or urea.33,34 We also developed a highly sensitive method for the detection of the E7-protein for human papillomavirus implicated in carcinogenesis35 and retinol binding protein 4 as a potential novel marker for the onset of type-2-diabetes.36 The key advantage of EG-FET-based sensing devices is their high sensitivity without the need for target modification with redox or fluorescent labels. Both components, the sensor chips and the (electronic) read-out equipment are cheap and readily available, making them ideally suited to further develop them to point-of-care settings potentially even in low socioeconomic areas where expertise and laboratory environments are not readily available. The EG-FET in general is based on the same principle as that known from metal oxide FETs. Two electrodes (source and drain) are separated by a semiconducting channel (Scheme 1). Applying a voltage at the gate electrode leads to changes in channel conductivity by modulating the current between the source and drain electrodes. Molecular binding entities functioning as “biorecognition elements” (antibodies and derivative formats, antigens, protein–ligands or oligonucleotide aptamers) can be used to functionalize the channel. Upon binding of the respective target analyte (molecule), the dielectric layers are structurally modified, changing the charge carrier distribution at the interface between the channel and the electrolyte. This alters the propagation of the gate voltage, resulting in a change in charge carrier mobility.37 By keeping the gate voltage constant, binding events on the channel can be observed as changes in the source–drain current (IDS). As we described previously, already focused aptamer libraries immobilized on reduced graphene oxide field-effect transistors (rGO-FETs, a sub-class of EG-FET devices)38 allowed for the discrimination of empty RBP4 from the loaded form (apo- vs. holo-RBP4) without the need to isolate individual aptamers prior construction of the chip.36 Here, B. producta-specific individual aptamers were isolated by bioinformatic analyses from the previous final library B.p-R1428 after NGS as described before for antibacterial aptamer isolation.22,27 These aptamers were initially characterized biochemically by determining their affinities and specificities to whole bacterial cells. Three of them found to recognize protein-based epitopes on the target cells were selected and used to functionalize rGO-FETs to demonstrate that this concept of aptamer-based electronic sensors is also suitable for quantitatively measuring whole bacterial cells. We show that B. producta can be distinguished from control gut bacteria in designed mixtures with high sensitivity and specificity. Moreover, it was possible to quantify B. producta in the background of real human stool samples.
Materials and methods
Cell lines and culture conditions
All bacterial strains, including A. muciniphila mucT (DSM-22959), A. stercoricanis (DSM-13633), R. intestinalis (DSM-14610), B. producta (DSM-29491), P. distasonis (DSM-29491), and R. microfusus (DSM-15922) were cultured under anaerobic conditions (90% Nitrogen/10% Hydrogen) in Schaedler broth liquid media at 37 °C.
Aptamer library NGS sequencing
Comparative next-generation sequencing and identification of individual aptamer sequences were performed using aptamer libraries R2, R7, and R14 by Eurofins Genomics (Eurofins Genomics Europe Shared Services GmbH, Konstanz, Germany) for Illumina® sequencing. The aptamer library was first elongated during the preparation stage to form sufficient bridges. In this process, the first PCR amplification was performed using the forward primer 5′-ACGATGATACTCG GACTGTAGGGAAGAGAAGGACATATGAT-3′ and the reverse primer 5′-TCTCGTGTTCAAGCGACTCAAGTGGTCATGTACTAGTCA A-3′ to introduce universal primer binding sites. The PCR was carried out using Herculase II Fusion DNA Polymerases (Agilent Technologies, Inc., Santa Clara, California, USA) in a three-step thermal reaction: initial denaturation at 95 °C for 3 min, followed by 9 cycles of denaturation at 95 °C for 30 s, annealing at 56 °C for 30 s, extension at 72 °C for 10 s, and final extension at 72 °C for 2 min. The resulting PCR product was then used as a template for a second elongation, during which index sequences were introduced to allow for parallel sequencing of the aptamer library. Different index sequences were used to discriminate between different aptamer libraries effectively. The second PCR conditions were the same as the first one, except that only 12 cycles were performed. Subsequently, the NGS data were checked using FastQC,39 the sequences were sorted, the primer binding sites were clipped, and the nucleotide distribution was analyzed using the FASTX toolkit. Finally, the sequences were analyzed using the FASTAptamer toolbox.40 The predicted secondary structure of the sequences was then determined using the Mfold server and selected individual aptamers were chemically synthesized by applying Biomers (Biomers.net GmbH, Ulm, Germany).
Fluorescence-based aptamer labeling characterization
First, prior to fluorescence-based aptamer affinity and specificity analyses, B. producta cells were proteinase treated to initially elucidate the chemical nature of binding sites/epitopes on the cell surface potentially protein-based epitopes. Therefore, B. producta was harvested by centrifugation at 7500 × g for 1 min after overnight culturing, washed three times with 1 × DPBS, and adjusted to an optical density at 600 nm (OD600) of 1 in the bacterial suspension (1 mL). Then, they were mixed with 2 μL Proteinase K (20 mg μL−1) (Fermentas International Inc., Waltham, MA, USA) and adjusted to a final concentration of 0.1 mg mL−1 with 1 × DPBS (Dulbecco's Phosphate-Buffered Saline). The mixture was incubated at 37 °C for 30 min to disrupt surface proteins while preserving bacterial binding activity. The control/negative group was treated with 1 × DPBS under the same conditions. After incubation, the mixture was centrifuged at 7500 × g for 1 minute and washed once with 1 × DPBS buffer. The cell pellet was incubated with activated aptamers (95 °C for 5 min, 4 °C for 5 min, and 25 °C for 20 min) at 37 °C for 30 min, followed by centrifugation at 3000 rpm for 2 min, and washed once with 500 μL of 1 × DPBS. The cells were resuspended in 100 μL of 1 × DPBS and incubated at 95 °C for 5 min to elute the bound aptamers, which were collected by centrifugation at 11
000 × g for 1 min, and the fluorescence intensity (excitation: 637 nm and emission: 670 nm) was measured using the Infinite F200 spectrophotometer (TECAN, Männedorf, Switzerland).
For specificity analyses of selected individual aptamers, A. muciniphila mucT, A. stercoricanis, P. distasonis, R. intestinalis, and R. microfusus were mixed in equal proportions to obtain the defined consortium of control bacteria. 5 pmol Cy5-labeled aptamers were either incubated separately or co-incubated with defined cell numbers of B. producta (1 mL OD600 = 1) and/or control bacterial species at 37 °C for 30 min. The supernatants were removed by centrifugation at 3000 rpm for 2 min, and the resulting bacterial pellets were washed and bound aptamers eluted for fluorescence measurement.
To determine the dissociation constant, the binding assay was performed equally using various aptamer concentrations. The binding constant was then derived from a one-site specific binding plot according to the following equation: F = (Fmax × [c]n)/(Kd + [c]n), where F = the measured fluorescence, Fmax = the maximal fluorescence, [c] = concentration of aptamer, n = hill coefficient and Kd = the binding constant.
To determine the aptamer detection limits, 5 pmol Cy5-labeled aptamers were co-incubated with varying cell numbers of B. producta at 37 °C for 30 min, and the detection limit of the aptamers was determined based on the linear correlation between the fluorescence intensity and the logarithm of the bacterial cell count.
Functionalization of rGO-FET for quantitative biosensing
The rGO-FET was fabricated following the procedures outlined in previous reports.41 Subsequently, a mixture of 500 μM PyPEG (polyethylene glycol as a capping agent pre-mixed with PBSE connecting agent) and 50 μM 1-pyrene carboxylic acid (PCA) was dissolved in DMSO. The rGO-FET was then immersed in the mixture and incubated at room temperature for 12 hours. Afterward, the chip was removed, subjected to repeated washing with 1 mL isopropanol, gently dried under a mild nitrogen flow, and subsequently submersed in 0.01 × DPBS containing EDC (15 mM) and NHS (15 mM) for 30 min at room temperature to adequately activate the carboxyl groups. Afterwards, 100 nM 5′-NH2-modified individual aptamers were dissolved in 1 × DPBS and covalently immobilized on the rGO-FET. Finally, after rinsing off the unbound aptamer with 0.01 × DPBS, the aptamer-functionalized rGO-FET was utilized for the specific electric detection of B. producta alone and in defined mixtures with control gut bacteria.
Analysis of B. producta abundance in fecal samples
Fecal samples were obtained from a healthy, lean volunteer recruited from Ulm University, who provided written informed consent. The study was approved by the local ethics committee of Ulm University (reference number 30/20). Furthermore, the design and implementation of the study adhered to regulations for the use of human research participants and strictly followed the standards established by the Helsinki Declaration. Fecal samples were weighed, added to 1 mL extraction buffer (1 × DPBS), and vortexed for 1 min until no fecal particles were visible. Then, the raw fecal extract was filtered, centrifuged at 7500 × g for 1 min, and washed with 1 mL of 1 × DPBS. Then, the fecal bacteria were adjusted to an OD600 = 1 and subjected to gGO-FET biosensing with calculated increasing cell numbers of B. producta (0–40 bacterial cells) in the background of 1000 fecal bacterial cells as calibration measurements where the specific increases correlated linearly with the shift increases for the aptamer sensors. The B. producta content in stool samples was then analyzed using the respective individually derived linear progression.
Results and discussion
In fourteen rounds of whole Cell-SELEX with B. producta as a bacterial target, a polyclonal (or focused) aptamer library was already developed, allowing specific labeling and discrimination of B. producta from other gut bacteria.28 The technological basis for the analysis and subsequent isolation of individual aptamers from the final SELEX round was comparative NGS of round R14 (final) and R2 (early) using Illumina sequencing. An ideal (commercial) synthetic aptamer starter library is expected to contain evenly distributed proportions of the four nucleobases adenosine, thymine, cytosine and guanine in the sequences of the oligonucleotides. Upon selection of sequences with increasing affinities towards the desired target, shifts occur within the distribution of individual nucleotides in the entire sequence space behind the analysis, which is normally accompanied by an increase in the GC content.42 This in turn results in an increase in aptamer melting temperatures when analyzed by applying a novel quantitative PCR method. We recently introduced “IMPATIENT-qPCR”.42 For the B. producta round fourteen libraries (B.p-R14), a final melting temperature of 81 °C was determined previously.28,42 The nucleotide distribution analysis of the B.p-R2 library showed the typical distribution of an early SELEX round, which was still close to the ideal 25% of each distribution but not identical to it (Fig. 1(a), left). In the final library B.p-R14, in contrast, the nucleotide composition showed significant changes, as expected during an SELEX evolution and selection process developing higher affinity aptamers (Fig. 1(a), right).
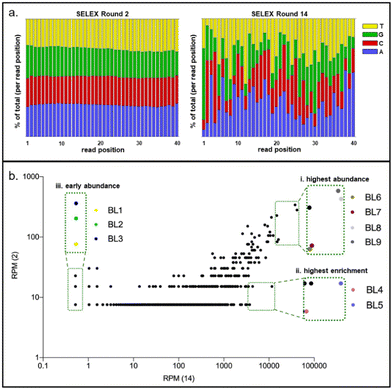 |
| Fig. 1 (a) Bioinformatical analysis of the B.p-R14 aptamer library, showing the nucleotide distribution of the aptamer libraries R2 and R14 (from left to right). The distribution of each nucleotide is presented as a percentage: red = cytosine (C), yellow = thymine (T), blue = adenine (A), and green = guanine (G). (b) Scatter plots comparing the sequence abundances of R2/R14 rounds demonstrate the enrichment of individual sequences as SELEX progresses. In the scatter plot, different colors are used to label and select sequences BL1-BL9 for further evaluation. Each color corresponds to a specific individual aptamer as follows: yellow-BL1, green-BL2, blue-BL3, pink-BL4, purple-BL5, brown-BL6, dark red-BL7, light gray-BL8, and dark gray-BL9. (i) Highest abundance, (ii) highest enrichment and (iii) early occurrence of sequences. | |
Plotting frequencies (reads per million (RPM)) of individual sequences occurring in the total sequence space of NGS sequencing reactions of early and final SELEX rounds delivers a diagram in which individual sequences with the same abundance in both rounds are located on a diagonal. This allows for the identification of sequences with (i) the highest total abundance in the final round, (ii) highest enrichment between early and final rounds, and (iii) sequences with moderate abundance but early occurrence in maintenance during the evolution process. Based on the B. producta frequency plot of B.p-R2 and B.p-R14, the individual aptamer BL6 to BL9 had the highest total abundances (group i), BL4 and BL5 (group ii) had the highest enrichment, and BL1 to BL3 were constantly present in the early group (group iii) B.p-R2 but also in the final round with low abundance (Fig. 1(b)). These sequences were selected for chemical synthesis to enable further in-depth characterization of these binding molecules by the established fluorescence-based assay,21,23,24,28 including labeling of the target cells with Cy5-labeled aptamers, removal of unbound aptamers by centrifugation, resuspension in fresh buffer and subsequent elution with removal of cell debris with a second centrifugation step (Fig. 2(a)). These include predictions of the most probable secondary structure and theoretical stability (folding enthalpy) via the Mfold web server established for the prediction of nucleic acid folding and hybridization by Jerry Zuker more than twenty years ago43 (Fig. 2(b)). The enthalpies were calculated under specific experimental conditions concerning pH and ionic strength (137 nM Na+, pH 7.4, 25 °C), were generally negative, ranging from −2.75 to −6.51 kcal mol−1 and represented the most probable and probably the most stable structure of each sequence, as the output of the algorithm behind Mfold (Fig. 2(b)).
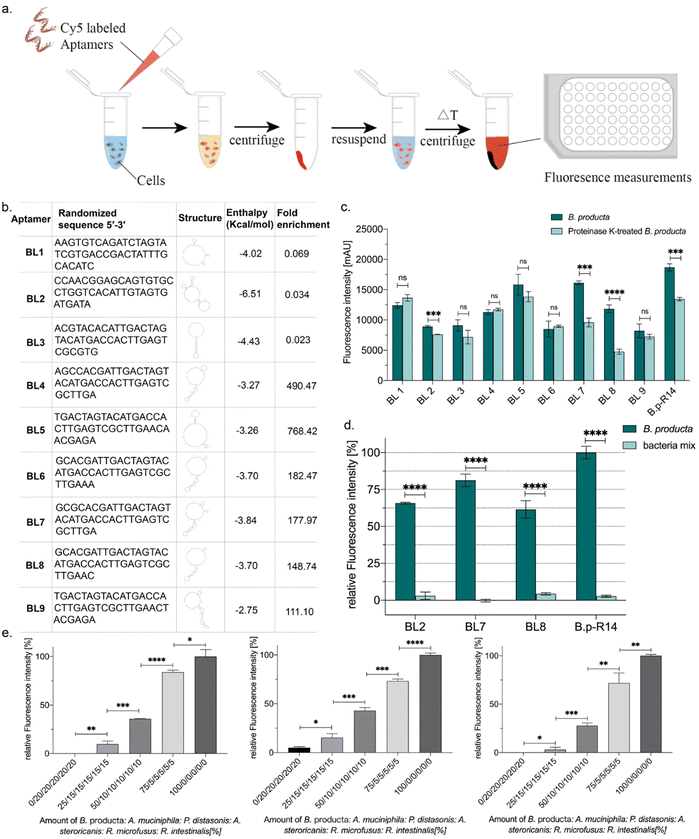 |
| Fig. 2 (a) Characterization of Cy5-labeled individual aptamers using the fluorescence-based assay, as described earlier for FluCell-SELEX. Color code: blue = fresh 1 × PBS buffer, red = Cy-5 labeled aptamers/bacteria, and yellow = unbound labeled aptamers in solution. (b) Sequence, simulated secondary structure, folding enthalpy, and frequency enrichment of selected individual aptamers. (c) Binding assays of individual aptamers BL1-BL9 and aptamer library B.p-R14 to untreated and proteinase K-treated B. producta. Significant differences were observed for individual aptamers BL2, BL7, BL8, and aptamer library B.p-R14. p values <0.05 were considered significant. ***p < 0.001 and **** p < 0.0001. (d) Specificity detection of the individual aptamers BL2, BL7, and BL8 as well as aptamer library B.p-R14. Bacteria mix is a mixture of five other gut bacteria, P. distasonis, A. muciniphila, R. microfusus, A. stercoricanis, and R. intestinalis, at a ratio of 1 : 1 : 1 : 1 : 1. Compared to the bacteria mix, the individual aptamers BL2, BL7, and BL8 and aptamer library B.p-R14 exhibited high specificity in detecting B. producta. Significant differences were observed (****p < 0.0001). (e) Quantitative tracking of B. producta was performed using Cy5-labeled BL2, BL7, and BL8 aptamers (left to right) targeting A. muciniphila, P. distasonis, A. stercoricanis, R. microfusus, and R. intestinalis in a gut bacterial mixture. The optical density of the mixture was adjusted to be equal among all samples, but the relative proportions of the different bacterial strains varied. Statistical significance was determined using a threshold of p < 0.05, with * representing p < 0.05, ** representing p < 0.01, *** representing p < 0.001, and **** representing p < 0.0001. | |
The outer barrier of a Gram-positive bacterium towards the environment is the cell wall, which comprises the lipid bilayer of the cell membrane, including membrane proteins and adjunct cell wall components. These components comprising peptidoglycan and lipoteichoic acids form surface epitopes in which every single molecule can serve as a theoretical epitope recognized and bound by selected aptamers (as reviewed by Lithgow et al. 202344). Among these chemical components, cell wall membrane proteins represent the most distinctive class of cell surface markers because they are represented by their protein sequence in each bacterial species. Theoretically, they allow more specific discrimination of different bacterial species compared to more general metabolism-derived cell wall components, especially the peptidoglycan sacculus with generally low structural variations among Gram-positive bacteria. Proteinase K-treatment of cells has been used to initially evaluate the chemical nature of aptamer binding sites (epitopes) on the cell surface of Bifidobacteria.45 The individual aptamers were fluorescently labeled with Cyanine 5 (Cy5) as described for the binding studies of the B. producta polyclonal aptamer library originating from a FluCell-SELEX.28 Labeling of proteinase K-treated B. producta with these Cy5-aptamers was significantly impaired for aptamers BL2, BL7, and BL8, and also for the original B.p-R14 library, indicating that these individual aptamers and probably more yet undefined individual aptamers in the library bind protein-based epitopes (Fig. 2(c)). These individual aptamers (BL2, BL7, and BL8) were selected for further experimental procedures and analyses. They possessed significant specificity for B. producta when their labeling ability was compared to equal cell numbers of a designed “consortium” of five mixed control bacteria (Fig. 2(d)) residing as natural inhabitants in the human gut, which were used as references in several studies.21–24,28 This was also true in experiments in the presence of this consortium as “contaminating” bacteria, in which increasing numbers of added B. producta cells could be reliably retraced (Fig. 2(e)).
The fluorescence-based preliminary performance characterization of the selected individual aptamers was finalized by determining the (lower) detection limits and the dissociation constants (Kd). However, BL2 and BL8 were able to measure 20
000 cells per mL, and BL7 was more sensitive with a tenfold lower detection limit of 2000 cells per mL (Fig. 3(a)). The Kd-values deduced from the binding experiments with increasing amounts of aptamers ranged from 1.9 nM to 2.0 nM (BL2 < BL8 < BL7) (Fig. 3(b)) and hence represented an almost six-fold improvement compared to the Kd of the original polyclonal library.28
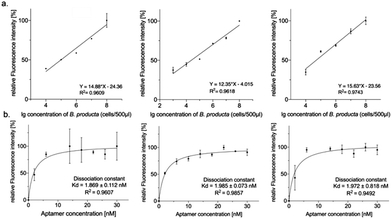 |
| Fig. 3 (a) The lower detection limits of aptamers BL2, BL7, and BL8 (left to right) were analyzed by measuring the decrease in fluorescence intensities with decreasing B. producta cell numbers. A calibration curve of the correlation between cell number and fluorescence intensity was linearly plotted. The cell concentrations ranged from 103 to 108 cells. The detection limits of aptamers BL2, BL7, and BL8 were determined to be 104 (R2 = 0.9609), 103 (R2 = 0.9618), and 104 (R2 = 0.9743) cells, respectively. (b) Site-specific binding of BL2, BL7, and BL8 aptamers (left to right) to B. producta at varying concentrations was determined using the one site-specific binding model in GraphPad Prism 8, with the curves fitted using non-linear regression. The dissociation constants (Kd) of BL2, BL7, and BL8 aptamers were 1.869 ± 0.112 nM (R2 = 0.9607), 1.985 ± 0.073 nM (R2 = 0.9857), and 1.972 ± 0.818 nM (R2 = 0.9492), respectively. | |
Their functionality as specific binding molecules on rGO-FET was evaluated upon EDC-NHS coupling (Scheme 1), as described earlier for other analytes (Kissmann et al. 2022; Reiner-Rozman et al. 2021), implementing the established low-gate-voltage scanning protocol from –0.5 V to +0.5 V.36 The aptamers on the chip captured the target cells in the samples as intended, inducing distinct shifts in the IDsVG curves by altering the charge distribution within the semiconductor structure. Recording IDsVG curves of samples containing increasing numbers of B. producta cells (0–1000
000 cells per mL) revealed increased shift alterations (ΔIDS) depending on the increase in cell numbers measured under constant VG conditions (−0.25 V), fitting distinctive linear progression functions illustrated in Fig. 4 for aptamers BL2, BL7, and BL8 with reasonable determination coefficients (R2) (Fig. 4(a) and (b)). Interestingly, in these experiments, samples adjusted to the calculated numbers of B. producta cells delivered unambiguous signals and were interpreted as reasonably lower detection limits for the three aptamers (Fig. 4(a) and (b)).
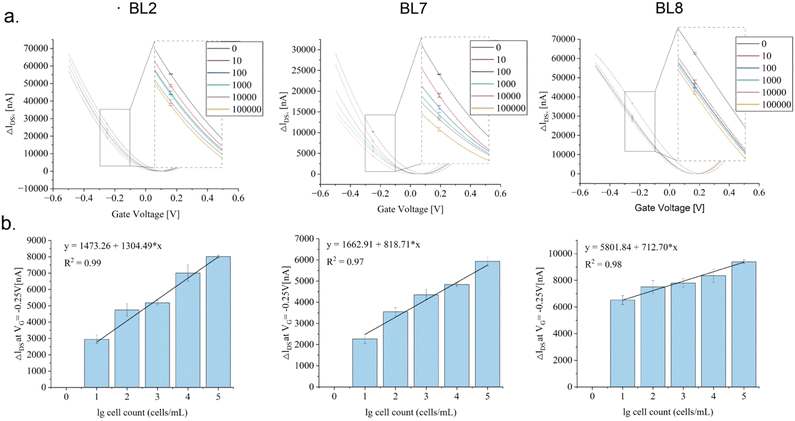 |
| Fig. 4 Sensitivity biosensing of aptamer-functionalized rGO-FET sensor chips. (a) IDVG characterization of sensing devices obtained using sweeping the gate voltage from −0.5 V to 0.5 V. The binding of BL2, BL7, and BL8 aptamers to B. producta ranging 0–1 000 000 cells. (b) Average differences of ΔIDS with varying logarithmic bacterial counts for BL2, BL7, and BL8 in specific binding events at a fixed gate voltage (VG) of −0.25 V. Error bars represent standard deviations by experiments conducted in triplicates. Linear correlation between ΔIDS and logarithm of bacterial count. | |
The intended application of a B. producta-specific rGO-FET exceeds simple quantitative measurements of the pure target bacterium because relevant biotechnological or medical tasks require target quantification in the presence of “contaminating” bacteria or even in the complex environment of microbiomes, such as in samples harvested from the human intestinal tract (e.g. in stool samples collected from patients). The results shown in Fig. 4, indicating a detection limit of 10 cells per mL in total of B. producta, served as the basis for the analysis of the chip specificities (Fig. 5(a)). In defined mixtures consisting of 100 bacterial cells in total, B. producta comprised either 0%, 25%, 50%, 75%, or 100% of all cells, and the remaining portion consisted of an otherwise constant mixture of P. distasonis, A. muciniphila, R. microfusus, A. stercoricanis, and R. intestinalis (1
:
1
:
1
:
1
:
1). Additionally, in this case, retracing of increased B. producta numbers was possible, and linear progression curves were defined accordingly with R2-values above 0.83, 0.91 and 0.96 for aptamers BL8, BL2 and BL7, respectively (Fig. 5(a)). To gain access to the microbiome, free bacteria were released to the planktonic phase from fecal samples (i.e. stool samples) of a healthy male volunteer by removing clotted solid matter from the raw material upon dissolution in 1 × PBS buffer, as illustrated in Fig. 5(b). After photometric estimation of cell densities in these samples, a calculated number of 1000 fecal bacterial cells was used as the base for B. producta specific calibration of the aptamer-based sensor chips. This constant amount of cells was then supplemented by gradually adding B. producta cells at calculated numbers of 0, 10, 20, 30, and 40 per sample, and the specific increases correlated linearly with the shift increases for the BL2, BL7 and BL8 sensors (Fig. 5(c)). Using the respective individually derived linear progression functions, the contents of B. producta cells in the sample could be determined to be 1.03%, 1.12%, and 1.27% for the aptamers BL2, BL7 and BL8, respectively (Fig. 5(c)).
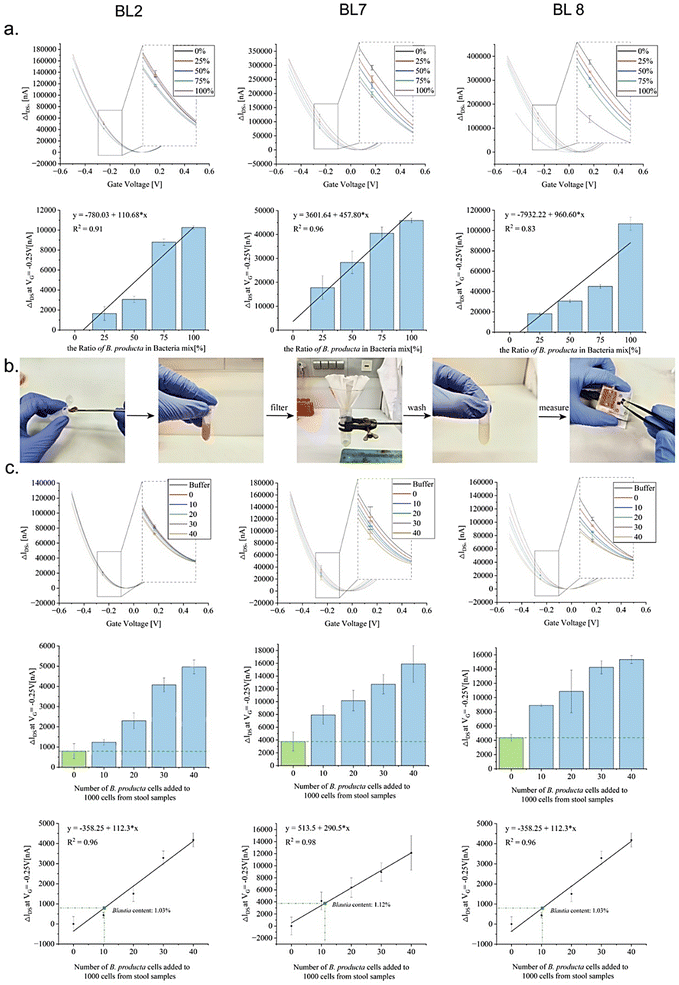 |
| Fig. 5 Specificity biosensing of aptamer-functionalized rGO-FET sensor chips. (a) IDsVG characterization of sensing devices obtained using sweeping the gate voltage from −0.5 V to 0.5 V. Binding of BL2, BL7, and BL8 aptamers to B. producta in defined mixtures with the control bacteria P. distasonis, A. muciniphila, R. microfusus, A. stercoricanis, and R. intestinalis. Under the condition of maintaining a total of calculated 100 bacterial cells per mL, the shifts in IDsVG curves were recorded in the mixed system when B. producta constituted 0%, 25%, 50%, 75%, and 100% of the population. Resulting average differences of ΔIDS with varying bacterial counts in mixtures for BL2, BL7, and BL8 in specific binding events at a fixed gate voltage (VG) of −0.25 V with a linear correlation between ΔIDS and bacterial count. Error bars represent standard deviations by experiments conducted in triplicate. (b) Extraction process of fecal bacteria from stool samples by dissolving human stool in 1 × DPBS buffer, filtration with subsequent centrifugation of the crude extract, followed by gFET-based biosensing. (c) To construct an incremental model of B. producta within fecal bacteria, we fixed the total number of fecal bacteria at 1000 and gradually added 0, 10, 20, 30, and 40 B. producta cells. Analysis of IDsVG curves and corresponding average differences of the ΔIDS at the fixed gate voltage of −0.25 V, showing a significant positive correlation with the additional quantity of added B. producta. The linear regression model depicted in the third row of the chart demonstrates the correlation of ΔIDS with the change in B. producta abundance in the system at a VG of −0.25 V. The amounts of B. producta in the feces were determined to be 1.03%, 1.12%, and 1.27% as detected by the three aptamer-modified rGO-FETs, BL2, BL7, and BL8, respectively. | |
Systematic modification of microbiomes with therapeutic intentions currently develops into a quasi salvation promise for lifestyle and nutrition-dependent civilization diseases, and studies have demonstrated that the addition of B. producta to animal models has clear positive effects on Parkinson's disease and hyperlipidemia as a metabolic disorder.46,47 Supplementing “ill” microbiomes with B. producta as a probiotic bacterium can result in alterations in its final abundance in the low percentage range.47 Intending to develop a monitoring technique to support such directed therapeutic microbiome engineering approaches, this range defines the benchmark of the required sensitivity to measure the success of probiotic intake and colonization in the human gut. In addition, the measurements have to be specific for the desired target bacterium, i.e. the sensor and in turn, the aptamer chosen as a binding entity has to “ignore” contaminating cells being simultaneously present in the (stool) sample to be analyzed. Electronic sensors based on rGO-FETs have been presented against prominent pathogens, including the SARS-Cov-2 virus as well as the Gram-positive and Gram-negative bacteria Stahphylococcus aureus and Acinetobacter baumanii based on biofunctionalization with antibodies or designed peptides.48,49 A biosensor for the detection of Escherichia coli was introduced based on an individual pyrene-modified aptamer.50 However, the aptamer used (E2 from ref. 51) was at best mediocre (>25 nM) compared to BL2, BL7 and BL8. Moreover, the detection limit of this sensor was one order of magnitude higher, and the degree of specificity for the discrimination of E. coli from other (bacterial) cells remained open.50
The individual aptamers BL2, BL7 and BL8 isolated from the B. producta-specific polyclonal aptamer library28 and characterized in this study proved that they can serve as valuable binding molecules for the construction of aptamer-based gFETs and allow for specific detection of the target bacterium not only in the presence of selected species in mixtures but also in the highly complex background of a real stool sample. Based on this result, an in-depth evaluation of this novel concept appears to be promising by involving the validation of the sensor results with comparative deep sequencing-based quantification of B. producta in stool samples of a larger cohort of volunteers/patients (>100 persons). Respective aptamer libraries and selected individual aptamers also exist for other prominent next-generation probiotics, such as A. muciniphila, R. intestinalis, and P. distasonis,21–24,28 which suggest implementing respective gFET sensors for the quantification of these important microbiome members. Individual sensors or multiplexed variants of this detection technology may develop into an easy way for fast but reliable quantification of subsets of human gut microbiome bacteria in general, opening new avenues towards supportive (bedside/home) monitoring of successful probiotic supplementation or future probiotic therapies of important diseases, including neurodegenerative disorders with known dependency from dysbiosis of the gut microbiome composition.
Conclusions
For biofunctionalized rGO-FETs, it has repeatedly been claimed that this technology may serve as precise sensing devices for a range of biotechnological and biomedical applications. Simultaneously, aptamers are claimed to be valuable binding molecules for functionalizing sensor chips. We demonstrate that by bioinformatical analyses, individual aptamers can be easily isolated from polyclonal SELEX aptamer libraries of already proofed applicability. These individual aptamers were characterized with biochemical methods, including fluorescent labeling and performing binding assays to the B. producta target cells in the presence of the defined mixtures of control gut bacteria. Affinities were determined, and Kd values were in the low nanomolar range, qualifying these aptamers as suitable for specific high-affinity binding of their target.
These aptamers were also suited to serve as biofunctionalization elements of gFET sensors, which allowed for the specific quantification and hence discrimination of the desired target cells (B. producta) from “contaminating” control bacteria. The lower detection limit was 10 cells per mL, and specific measurements were possible in mixtures of B. producta with these “contaminating” bacteria. In the background of the human stool, sample alterations in the abundance of B. producta in the range of 1% could easily be retraced. This is sensitive enough to realistically promise the construction of a sensor device for the quantitative supportive monitoring of probiotic supplementation strategies in biomedicine.
Institutional review board statement
Fecal samples were obtained from a healthy, lean volunteer recruited from Ulm University, who provided written informed consent. The study was approved by the local ethics committee of Ulm University (reference number 30/20). Furthermore, the design and implementation of the study adhered to regulations of the German law for the use of human research participants and strictly followed the standards established by the Helsinki Declaration.
Informed consent statement
Written informed consent has been obtained from the patient to publish this paper.
Author contributions
Supervision, A.-K. K. and F. R.; methodology, writing – review and editing, H. X., Y. Z., R. L., H.-M. R., C. H., J. A., A. B., M. H. and R. H.; writing, investigation; H. X., Y. Z., R. L. and A.-K. K.; formal analysis; H. X., Y. Z., R. L., H.-M. R. and A.-K. K., conceptualization, A.-K. K. and F. R.; resources; U. K., C. K., W. G. and F. R. All authors have read and agreed to the published version of the manuscript.
Data svailability
Data generated by NG Sequencing for the analyses and selection of the individual aptamers used in the study are deposited online under the following file name “Xing SELEX Sequencing Blautia producta.gz” and are accessible using this link: https://cloudstore.uni-ulm.de/s/TbPX6C8AERbGnsj.
Conflicts of interest
There are no conflicts to declare.
Acknowledgements
This work was supported by the China Scholarship Council (no.: 202108080084 and 202208080009) and the German Research Society (DFG) project 465229237, and the Gesellschaft für Forschungsförderung (GFF) of Lower Austria as part of the project ‘‘Aptamers and Odorant Binding Proteins – Innovative Receptors for Electronic Small Ligand Sensing’’ (FTI22-G-012). Open Access funding provided by the Max Planck Society.
References
- X. Liu, B. Mao, J. Gu, J. Wu, S. Cui, G. Wang, J. Zhao, H. Zhang and W. Chen, Gut Microbes, 2021, 13, 1–21 CAS.
- W. Zhang, J. Li, S. Lu, N. Han, J. Miao, T. Zhang, Y. Qiang, Y. Kong, H. Wang, T. Gao, Y. Liu, X. Li, X. Peng, X. Chen, X. Zhao, J. Che, L. Zhang, X. Chen, Q. Zhang, M. Hu, Q. Li and B. Kan, Sci. Rep., 2019, 9, 1594 CrossRef PubMed.
- Y. J. Wang, R. Abdugheni, C. Liu, N. Zhou, X. You and S. J. Liu, Int. J. Syst. Evol. Microbiol., 2021, 71, 005371 Search PubMed.
- F. S. Heravi, K. Naseri and H. Hu, Nutrients, 2023, 15(20), 4365 CrossRef CAS PubMed.
- H. Li, W. Guo, S. Li, B. Sun, N. Li, D. Xie, Z. Dong, D. Luo, W. Chen, W. Fu, J. Zheng and J. Zhu, Front. Microbiol., 2023, 14, 1326870 CrossRef PubMed.
- L. Qingbo, Z. Jing, Q. Zhanbo, C. Jian, S. Yifei, W. Yinhang and H. Shuwen, Gut Pathog., 2024, 16, 12 CrossRef PubMed.
- J. L. Maturana and J. P. Cardenas, Front. Microbiol., 2021, 12, 660920 CrossRef PubMed.
- M. Arumugam, J. Raes, E. Pelletier, D. Le Paslier, T. Yamada, D. R. Mende, G. R. Fernandes, J. Tap, T. Bruls, J. M. Batto, M. Bertalan, N. Borruel, F. Casellas, L. Fernandez, L. Gautier, T. Hansen, M. Hattori, T. Hayashi, M. Kleerebezem, K. Kurokawa, M. Leclerc, F. Levenez, C. Manichanh, H. B. Nielsen, T. Nielsen, N. Pons, J. Poulain, J. Qin, T. Sicheritz-Ponten, S. Tims, D. Torrents, E. Ugarte, E. G. Zoetendal, J. Wang, F. Guarner, O. Pedersen, W. M. de Vos, S. Brunak, J. Dore, H. I. T. C. Meta, M. Antolin, F. Artiguenave, H. M. Blottiere, M. Almeida, C. Brechot, C. Cara, C. Chervaux, A. Cultrone, C. Delorme, G. Denariaz, R. Dervyn, K. U. Foerstner, C. Friss, M. van de Guchte, E. Guedon, F. Haimet, W. Huber, J. van Hylckama-Vlieg, A. Jamet, C. Juste, G. Kaci, J. Knol, O. Lakhdari, S. Layec, K. Le Roux, E. Maguin, A. Merieux, R. Melo Minardi, C. M'Rini, J. Muller, R. Oozeer, J. Parkhill, P. Renault, M. Rescigno, N. Sanchez, S. Sunagawa, A. Torrejon, K. Turner, G. Vandemeulebrouck, E. Varela, Y. Winogradsky, G. Zeller, J. Weissenbach, S. D. Ehrlich and P. Bork, Nature, 2011, 473, 174–180 CrossRef CAS PubMed.
- A. M. Eren, M. L. Sogin, H. G. Morrison, J. H. Vineis, J. C. Fisher, R. J. Newton and S. L. McLellan, ISME J., 2015, 9, 90–100 CrossRef CAS PubMed.
- P. O. Sheridan, J. C. Martin, T. D. Lawley, H. P. Browne, H. M. B. Harris, A. Bernalier-Donadille, S. H. Duncan, P. W. O’Toole, P. K. Scott and H. J. Flint, Microb. Genomes, 2016, 2, e000043 Search PubMed.
- B. Mao, W. Guo, X. Liu, S. Cui, Q. Zhang, J. Zhao, X. Tang and H. Zhang, Probiotics Antimicrob. Proteins, 2023, 15(3), 785–796 CrossRef CAS PubMed.
- Y. N. Yang, Q. C. Wang, W. Xu, J. Yu, H. Zhang and C. Wu, Biomed. Pharmacother., 2022, 155, 113749 CrossRef CAS PubMed.
- N. Ozato, T. Yamaguchi, K. Mori, M. Katashima, M. Kumagai, K. Murashita, Y. Katsuragi, Y. Tamada, M. Kakuta, S. Imoto, K. Ihara and S. Nakaji, Biology, 2022, 11, 318 CrossRef CAS PubMed.
- N. Ozato, S. Saito, T. Yamaguchi, M. Katashima, I. Tokuda, K. Sawada, Y. Katsuragi, M. Kakuta, S. Imoto, K. Ihara and S. Nakaji, NPJ Biofilms Microbiomes, 2019, 5, 28 CrossRef PubMed.
- X. Yuan, Y. Zhang, X. Lin, X. Yang and R. Chen, Pediatr. Obes., 2023, 18, e13009 CrossRef PubMed.
- I. Kimura, K. Ozawa, D. Inoue, T. Imamura, K. Kimura, T. Maeda, K. Terasawa, D. Kashihara, K. Hirano, T. Tani, T. Takahashi, S. Miyauchi, G. Shioi, H. Inoue and G. Tsujimoto, Nat. Commun., 2013, 4, 1829 CrossRef.
- I. Kimura, D. Inoue, T. Maeda, T. Hara, A. Ichimura, S. Miyauchi, M. Kobayashi, A. Hirasawa and G. Tsujimoto, Proc. Natl. Acad. Sci. U. S. A., 2011, 108, 8030–8035 CrossRef CAS.
- F. Lebreton, A. L. Manson, J. T. Saavedra, T. J. Straub, A. M. Earl and M. S. Gilmore, Cell, 2017, 169, 849–861 CrossRef CAS.
- S. G. Kim, S. Becattini, T. U. Moody, P. V. Shliaha, E. R. Littmann, R. Seok, M. Gjonbalaj, V. Eaton, E. Fontana, L. Amoretti, R. Wright, S. Caballero, Z. X. Wang, H. J. Jung, S. M. Morjaria, I. M. Leiner, W. Qin, R. Ramos, J. R. Cross, S. Narushima, K. Honda, J. U. Peled, R. C. Hendrickson, Y. Taur, M. R. M. van den Brink and E. G. Pamer, Nature, 2019, 572, 665–669 CrossRef CAS.
- S. Caballero, S. Kim, R. A. Carter, I. M. Leiner, B. Susac, L. Miller, G. J. Kim, L. Ling and E. G. Pamer, Cell Host Microbe, 2017, 21, 592–602 CrossRef CAS.
- H. Xing, A. K. Kissmann, H. F. Raber, M. Kramer, V. Amann, K. Kohn, T. Weil and F. Rosenau, Microorganisms, 2021, 9, 2284 CrossRef CAS PubMed.
- H. F. Raber, D. H. Kubiczek, N. Bodenberger, A. K. Kissmann, D. D’Souza, H. Xing, D. Mayer, P. Xu, U. Knippschild, B. Spellerberg, T. Weil and F. Rosenau, Int. J. Mol. Sci., 2021, 22, 856 CrossRef PubMed.
- Y. Zhang, H. Xing, G. Bolotnikov, M. Kramer, N. Gotzmann, U. Knippschild, A. K. Kissmann and F. Rosenau, Microorganisms, 2023, 11, 2266 CrossRef CAS PubMed.
- H. Xing, Y. Zhang, M. Kramer, A. K. Kissmann, V. Amann, H. F. Raber, T. Weil, K. R. Stieger, U. Knippschild, M. Henkel, J. Andersson and F. Rosenau, Int. J. Mol. Sci., 2022, 23, 7744 CrossRef CAS PubMed.
- A. D. Ellington and J. W. Szostak, Nature, 1990, 346, 818–822 CrossRef CAS PubMed.
- C. Tuerk and L. Gold, Science, 1990, 249, 505–510 CrossRef CAS PubMed.
- D. Kubiczek, H. Raber, N. Bodenberger, T. Oswald, M. Sahan, D. Mayer, S. Wiese, S. Stenger, T. Weil and F. Rosenau, Chemistry, 2020, 26, 14536–14545 CrossRef CAS PubMed.
- H. Xing, Y. Zhang, M. Kramer, A. K. Kissmann, M. Henkel, T. Weil, U. Knippschild and F. Rosenau, Molecules, 2022, 27, 5693 CrossRef CAS PubMed.
- L. Zhou, H. Mao, C. Wu, L. Tang, Z. Wu, H. Sun, H. Zhang, H. Zhou, C. Jia, Q. Jin, X. Chen and J. Zhao, Biosens. Bioelectron., 2017, 87, 701–707 CrossRef CAS.
- B. Cai, L. Huang, H. Zhang, Z. Sun, Z. Zhang and G. J. Zhang, Biosens. Bioelectron., 2015, 74, 329–334 CrossRef CAS PubMed.
- S. Xu, S. Jiang, C. Zhang, W. Yue, Y. Zou, G. Wang, H. Liu, X. Zhang, M. Li, Z. Zhu and J. Wang, Appl. Surf. Sci., 2018, 427, 1114–1119 CrossRef CAS.
- Y. M. Lei, M. M. Xiao, Y. T. Li, L. Xu, H. Zhang, Z. Y. Zhang and G. J. Zhang, Biosens. Bioelectron., 2017, 91, 1–7 CrossRef CAS PubMed.
- S. Wang, M. Z. Hossain, K. Shinozuka, N. Shimizu, S. Kitada, T. Suzuki, R. Ichige, A. Kuwana and H. Kobayashi, Biosens. Bioelectron., 2020, 165, 112363 CrossRef CAS PubMed.
- T. Ono, Y. Kanai, K. Inoue, Y. Watanabe, S. I. Nakakita, T. Kawahara, Y. Suzuki and K. Matsumoto, Nano Lett., 2019, 19, 4004–4009 CrossRef CAS PubMed.
- P. Aspermair, V. Mishyn, J. Bintinger, H. Happy, K. Bagga, P. Subramanian, W. Knoll, R. Boukherroub and S. Szunerits, Anal. Bioanal. Chem., 2021, 413, 779–787 CrossRef CAS PubMed.
- A. K. Kissmann, J. Andersson, A. Bozdogan, V. Amann, M. Kramer, H. Xing, H. F. Raber, D. H. Kubiczek, P. Aspermair, W. Knoll and F. Rosenau, Nanoscale Horiz., 2022, 7, 770–778 RSC.
- C. Reiner-Rozman, M. Larisika, C. Nowak and W. Knoll, Biosens. Bioelectron., 2015, 70, 21–27 CrossRef CAS PubMed.
- P. Aspermair, U. Ramach, C. Reiner-Rozman, S. Fossati, B. Lechner, S. E. Moya, O. Azzaroni, J. Dostalek, S. Szunerits, W. Knoll and J. Bintinger, J. Am. Chem. Soc., 2020, 142, 11709–11716 CrossRef CAS PubMed.
-
S. Andrews, Babraham Bioinformatics, 2010, http://www.bioinformatics.babraham.ac.uk/projects/ Search PubMed.
- K. K. Alam, J. L. Chang and D. H. Burke, Mol. Ther.--Nucleic Acids, 2015, 4, e230 CrossRef CAS PubMed.
- C. Reiner-Rozman, R. Hasler, J. Andersson, T. Rodrigues, A. Bozdogan, J. Bintinger and P. Aspermair, Micro Nano Lett., 2021, 16, 436–442 CrossRef CAS.
- A. K. Kissmann, G. Bolotnikov, R. Li, F. Muller, H. Xing, M. Kramer, K. E. Gottschalk, J. Andersson, T. Weil and F. Rosenau, Appl. Microbiol. Biotechnol., 2024, 108, 284 CrossRef CAS.
- M. Zuker, Nucleic Acids Res., 2003, 31, 3406–3415 CrossRef CAS PubMed.
- T. Lithgow, C. J. Stubenrauch and M. P. H. Stumpf, Nat. Rev. Microbiol., 2023, 21, 502–518 CrossRef CAS PubMed.
- L. Hu, L. Wang, W. Lu, J. Zhao, H. Zhang and W. Chen, Int. J. Mol. Sci., 2017, 18, 2540 CrossRef PubMed.
- J. Liu, X. Lv, T. Ye, M. Zhao, Z. Chen, Y. Zhang, W. Yang, H. Xie, L. Zhan, L. Chen, W. C. Liu, K. P. Su and J. Sun, Brain, Behav., Immun., 2024, 117, 270–282 CrossRef CAS.
- W. Xu, J. Yu, Y. Yang, Z. Li, Y. Zhang, F. Zhang, Q. Wang, Y. Xie, B. Zhao and C. Wu, Gut Microbes, 2023, 15, 2228045 CrossRef.
- L. Lozano-Chamizo, C. Marquez, M. Marciello, J. C. Galdon, E. de la Fuente-Zapico, P. Martinez-Mazon, V. Gonzalez-Rumayor, M. Filice and F. Gamiz, Biosens. Bioelectron., 2024, 250, 116040 CrossRef CAS.
- N. Kumar, W. Wang, J. C. Ortiz-Marquez, M. Catalano, M. Gray, N. Biglari, K. Hikari, X. Ling, J. Gao, T. van Opijnen and K. S. Burch, Biosens. Bioelectron., 2020, 156, 112123 CrossRef CAS PubMed.
- G. Wu, Z. Dai, X. Tang, Z. Lin, P. K. Lo, M. Meyyappan and K. W. C. Lai, Adv. Healthcare Mater., 2017, 6, 1700736 CrossRef.
- Y. S. Kim, M. Y. Song, J. Jurng and B. C. Kim, Anal. Biochem., 2013, 436, 22–28 CrossRef CAS.
Footnote |
† These authors contributed equally to this work. |
|
This journal is © The Royal Society of Chemistry 2025 |
Click here to see how this site uses Cookies. View our privacy policy here.