DOI:
10.1039/D4TA08475F
(Paper)
J. Mater. Chem. A, 2025,
13, 12938-12948
Multifunctional and highly stretchable gold-leaf/WPU/TPU fibers by a rolling adhesive method†
Received
29th November 2024
, Accepted 4th February 2025
First published on 17th February 2025
Abstract
Flexible conductive fibers have attracted significant attention due to their excellent mechanical and electrical properties. In this study, a new rolling adhesive technique was introduced to fabricate flexible conductive fibers with a nano-gold leaf on thermoplastic polyurethane (TPU) fiber using waterborne polyurethane (WPU) as an inner adhesive layer. The gold-leaf/WPU/TPU (GLWT) fiber with a single gold sheath layer can be applied as a resistive strain sensor with high sensitivity (GF = 130) at large strain (80%) to detect human motion. In addition, we have successfully woven GLWT fibers into gloves by taking advantage of the knittability of the fibers to enable the identification of various hand gestures. The GLWT fibers can be thermoformed into helices to achieve excellent electrical properties (Q = 123) over a wide range of strains (1600%). It is worth noting that GLWT fibers can be prepared into capacitive pressure sensing arrays by staggered placement, which have the ability to identify the shape of objects. We also fabricated a GLWT fiber with two gold-leaf sheath layers, which shows a capacitive response with good linearity (0.999) up to 60% strain and GF of 0.8. Overall, GLWT fibers have considerable potential in the field of flexible electronic devices due to their simple manufacturing process and multifunctional applications.
1. Introduction
Flexible electronics are emerging as promising candidates for wearable and smart devices,1–5 owing to their vast potential in a variety of applications, including stretchable circuits,6–11 electronic skins,12 and smart fabrics.13–15 Compared to traditional planar-type flexible electronics,16–18 flexible conductive fibers have attracted considerable attention in flexible electronics because they can be easily integrated into various textile structures, providing diverse deformations, wearability, and comfort, as well as offering distinct advantages such as lightweight properties and excellent breathability.19–21
The core-sheath structure is a typical configuration for flexible conductive fibers,22–26 with the sheath made of conductive materials and the core composed of stretchable materials. As a high concentration of conductive fillers can considerably reduce the tensile properties of fibers,25 conductive fibers with a core-sheath structure offer a distinct advantage over those formed using composite elastic matrices with conductive fillers, allowing for both high electrical conductivity and excellent tensile strength.27–31 The core of flexible conductive fibers provides stretchability, while the conductive sheath ensures electrical conductivity, with the two layers working synergistically to optimize the fiber's overall performance. The fiber's core is typically composed of stretchable materials, such as polydimethylsiloxane (PDMS),32,33 styrene–butadiene–styrene (SBS),34–36 or thermoplastic polyurethane (TPU).37–40 As a thermoplastic material, TPU offers additional advantages, such as thermoplasticity and recyclability, expanding the potential applications of the fiber.22 Various methods have been employed to prepare the conductive sheath layers of fibers, including dip-coating,41 magnetron sputtering,42 electrochemical deposition,43 and in situ polymerization,44–46 among others.47–50 Compared to chemical methods, nanometal films prepared by physical methods have advantages such as high electrical conductivity and low defects;51,52 however, their relatively poor adhesion on the polymer surface and high cost are serious problems.25,31
Among conductive metals, gold is commonly used in the preparation of fiber's conductive sheath layer because of its superior electrical conductivity and inoxidizability.51,53–55 Liu et al. developed a fiber-based stretchable strain sensor by depositing gold films onto modified elastic microfibers with intrinsic microbeads.56 Li et al. demonstrated a waterproof core-sheath structure strain sensor with a relatively wide strain sensing range (50%) fabricated by a simple approach. This core-sheath structure is composed of a super elastic core material polyurethane (PU) yarn and a highly conductive multilayer sheath layer with graphene nanosheets/thin gold film/graphene nanosheets.54 Xiao et al. developed a fiber-core-sheathed fiber optic strain sensor featuring a conductive layer made of a porous elastic conductive material and fractured metal mesh.40 However, the majority of gold conductive layers in the reports are deposited using methods such as electron beam evaporation and ion beam sputtering, which require specialized equipment and are at a high cost, so a simpler and more cost-efficient method for fabricating nanometal films on the surface of fiber is urgently needed. Traditional nano-gold leaves are economically viable alternatives as commercially available and low-cost thin metal films for decorative purposes commonly.57–59 Many studies have employed nano-gold leaves to fabricate planar electronics for electrochemical sensing, which exhibit excellent electrical properties, and their manufacturing process is simple and fast and does not require specialized equipment.60–62 However, as a possible solution for the above issue, integrating nano-gold leaves onto the surface of stretchable fibers is a considerable challenge aimed towards green and sustainable goals.
In this study, a new rolling adhesive method was introduced to fabricate flexible conductive fibers by adhering a nano-gold leaf onto TPU fibers, with waterborne polyurethane (WPU) serving as the inner adhesive layer. The fiber can be mass-produced by adhering multiple sheets of the nano-gold leaf, achieving high initial conductivity (σ = 6 × 106 S m−1) and excellent strain response (GF is 130 at 80% strain), making it suitable for applications such as human motion detection. At an appropriate thermoplastic temperature, the fiber can be easily molded into a helical electrode, which maintains electrical signal stability under ultra-large strain when the helical index C is adjusted. Additionally, these conductive fibers can be employed as capacitive strain sensors with favorable electrical properties. Notably, the fibers can also be arranged in a staggered configuration to form capacitive pressure-sensing arrays, capable of differentiating between object shapes.
2. Experimental
2.1 Materials
TPU wires of 400 μm diameter were acquired from Guangzhou Xinshijie Jewellery Co. The WPU precursor (model: F0409) was purchased from Shenzhen Jitian Chemical Co. The nano-gold leaf (thickness: 0.12 μm, purity: 99.0%) was provided by Nanjing Jinling nano-gold leaf Group Co.
2.2 Preparation of GLWT fibers
GLWT fibers were fabricated as shown in Fig. 1a. First, the TPU fibers were cut to the desired length and then washed with an alcohol solution to remove surface pollutants. The cleaned TPU fiber was then immersed in the WPU precursor for 1 minute, and then removal at a rate of 120 mm min−1 was performed to generate a smooth WPU coating on the surface. After drying at room temperature (humidity: 60%) for 10 minutes, a semi-cured WPU adhesive layer was obtained. Next, the TPU fibers were rolled on the nano-gold leaf, and the nano-gold leaf was adhered to the surface of the fibers. Subsequently, the excess gold leaf on the fiber surface was removed using a soft wool brush after drying at 50 °C for 30 minutes. To finish, copper wires were wrapped around both ends, and the electrodes were coated with liquid metal to guarantee good electrical contact between the nano-gold leaf and the copper wires, followed by encapsulation with a layer of WPU for waterproofing (thickness: 20 μm). The gold-leaf/WPU/TPU(GLWT) fibers obtained are shown in Fig. S1.† In order to show the production cost, we have made a table (Table S1†). It can be observed from the table that the preparation cost of a single GLWT fiber is under 1 RMB, with each centimeter costing no more than 0.2 RMB.
 |
| Fig. 1 Preparation of GLWT fibers. (a) Schematic diagram of the GLWT fiber manufacturing process. (b) The GLWT fiber was fixed at the index finger joint to monitor the electrical resistance changes caused by bending. (c) The thermoplastic spiral fiber was used as a stretchable electrode to monitor the brightness changes of the LED. (d) The fibers were arranged in an array and the pressure of the weights is measured. | |
Notably, GLWT fibers can be attached to the finger to detect joint movement (Fig. 1b). As shown in Fig. 1c, the light intensity from the LED remains almost unchanged between the original and stretched configurations of the helical fibers, demonstrating the excellent stability of the helical conducting fibers. In addition, GLWT fibers can be developed into capacitive pressure sensing arrays where mechanical sensing units are formed at each contact point (Fig. 1d).
2.3 Characterization and measurements
SEM was used to observe both the surface and cross section of the fibers (JSMIT500HR, Japan Electronics). A high-precision uniaxial testing device for tensile measurements (ZQ-990B, Zhiqu Precision Instrument Co., Ltd) was used to evaluate the tensile properties. The FTIR spectra were acquired by using a Spectrum Two FTIR spectrometer (PerkinElmer) with an attenuated total reflectance (ATR) attachment. An optical microscope (GP-660V, Kunshan Gaopin Precision Instrument Co., Ltd) was used to photograph the surface morphology of fiber. Three objects (materials: polylactic acid) for monitoring pressure distribution were manufactured using an Ender-3 S1 Pro 3D printer (Creative 3D multi-creation Yunbo technology studio).
2.4 Consent statement
All experiments were conducted in accordance with relevant guidelines and approved by the School of Optoelectronics and Computer Engineering, University of Shanghai for Science and Technology, Shanghai 200093, China. Related written informed consent was obtained for each participant according to institutional guidelines.
3. Results and discussion
3.1 Seamless rolling adhesion technology
It is worth noting that most commercially available nano-gold leaves are limited to a length of no more than 10 cm, which restricts the length of the fiber and significantly limits its potential applications. In contrast, our GLWT fibers can continuously adhere to multiple sheets of nano-gold leaf, thereby extending the fiber length (Fig. 2a). Fig. 2b shows the process of removing the excess gold leaf from the seams of the nano-gold leaf. Among them, Fig. 2b1 presents an optical image of the junction between two nano-gold leaves with excess gold leaves attached to them. A soft-bristled brush is first used to remove the excess broken nano-gold leaf from the joints (Fig. 2b2). After brushing back and forth about 20 times, the surface of GLWT fibers becomes smooth and even, with the seam barely visible (Fig. 2b3 and Movie 1†). To evaluate the seamless adhering effect of multiple nano-gold leaves, the resistance of a 20 cm long fiber was measured at 1 cm intervals. As shown in Fig. 2c, the resistance increases approximately linearly with GLWT fiber length (with the nano-gold leaf seams marked using red boxes). Notably, there is almost no abrupt change in resistance at the seams (6.2–6.3 Ω cm−1), indicating that GLWT fibers adhered to multiple layers of the nano-gold leaf exhibit excellent electrical properties.
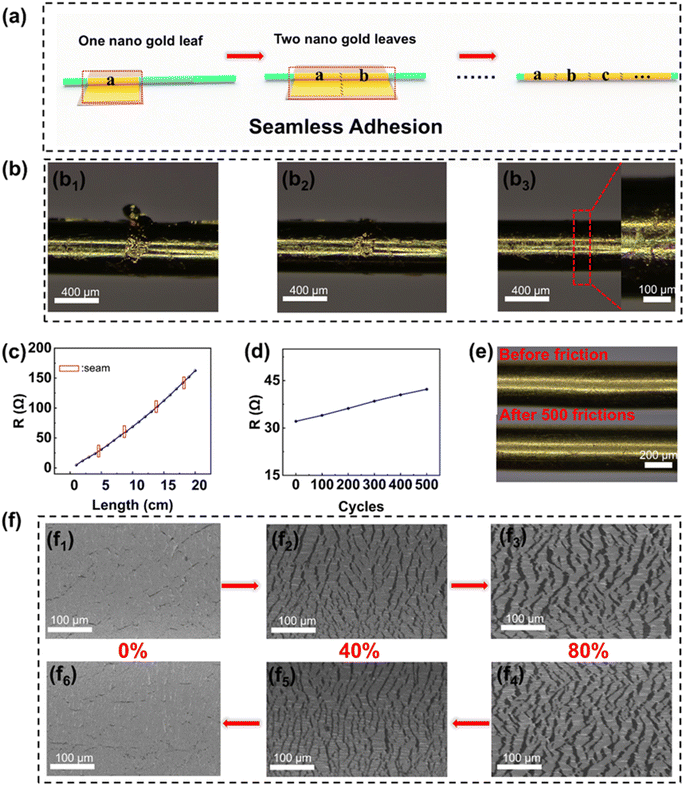 |
| Fig. 2 Seamless rolling adhesion technology. (a) Seamless adhesion technology. (b) Optical image at the nano-gold leaf seam. (c) Curve of initial resistance of GLWT fibers versus length. (d) Curve of resistance of fibers versus the number of friction times. (e) Optical microscope images of GLWT fibers before and after friction. (f) SEM image of the surface of the fibers with strain-recovery of 0–80% fibers. | |
The physical adhesion of the gold leaf to the TPU surface is driven by electrostatic forces and van der Waals forces. Electrostatic forces act over short ranges near the material surface, limiting the effective contact area; moreover, the van der Waals forces are relatively weak compared to chemical bonds, resulting in weaker adhesion. Therefore, the adhesion of the gold leaf to the TPU surface through physical adhesion is usually poor, so the gold leaf after physical adhesion onto TPU fiber can be easily peeled off only once with 3M tape as shown in Fig. S2.† However, the gold leaf can be adhered onto TPU fiber through semi-cured WPU using a rolling adhesive method, ensuring in situ curing of the WPU prepolymer on the gold leaf to ensure maximum contact area. During curing, a stable hydrogen bond network is formed on the interface between the hydrophilic gold leaf63 and WPU adhesion layer, resulting in good adhesion.52 Our new rolling adhesion method ensured the good adhesion of the gold leaf on the WPU adhesion layer, so the gold leaf remained good without damage on the TPU fiber's WPU layer after 100-cycle peeling off, as illustrated in Fig. S3.† Through the optical microscope observation, it was found that the nano-gold leaf on the surface of the GLWT fiber was almost undamaged. Fig. S4† illustrates the change in resistance of GLWT fibers before and after 100 adhesions, with the resistance increasing only slightly from 29.54 Ω to 30.13 Ω. A 4 cm GLWT fiber was subjected to 500 cycles of friction (Fig. 2d), showing a slight increase in resistance from 8.02 Ω cm−1 to 10.56 Ω cm−1. Optical microscope images of the GLWT fibers before and after friction are shown in Fig. 2e. No significant shedding of the nano-gold leaf was observed, further demonstrating the good adhesion of the nano-gold leaf to the WPU.
In order to ensure the stability of GLWT fiber in the strain test, aging cycle processes were carried out. As shown in Fig. S5,† the fibers were stretched at 20%, 40%, 60% and 80% strains, and the recovery resistance was recorded every 30 cycles for a total of 300 cycles. In the initial stage of aging, as the strain increases, new cracks form, leading to an increase in recovery resistance. With further training, no new cracks appear on the fiber surface, resulting in the formation of a stable crack structure, and the resistance changes stabilize.40 The recovery resistance stabilizes after about 150 cycles, indicating that the aging process is complete. The following GLWT fibers used in subsequent experiments were all subjected to complete aging training at 80% strain for 150 cycles. The morphology of the nano-gold leaf on the GLWT fibers during stretching and release was observed using scanning electron microscopy (Fig. 2f). After stretching, visible network cracks appear on the surface of the fibers. Fig. 2f2 and f3 show the network crack structures of the GLWT fibers under 40% and 80% strain, respectively. It is evident that as the strain increases, the network cracks gradually widen. Additionally, Fig. 2f6 demonstrates that GLWT fiber retains good recovery ability even after being stretched to 80%.
3.2 Strain sensing performance of GLWT fibers
GLWT fibers exhibit excellent elasticity properties, as demonstrated in Fig. 3a, which presents the stress–strain curves of TPU and GLWT fibers. Both fibers reach a maximum strain of 466%, with the curves nearly overlapping. Fig. S6† shows the stretch-release curve of GLWT fiber at 80% strain. The curves almost overlap, indicating its low hysteresis and good mechanical response.
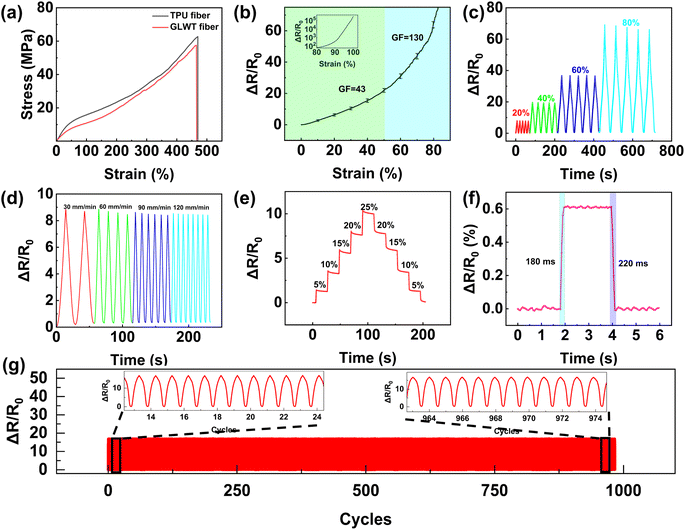 |
| Fig. 3 Strain sensing performance of GLWT fibers. (a) Stress–strain curves of TPU fiber and GLWT fiber. (b) Variation of relative resistance with strain. (c) Variation of relative resistance at different cyclic strains. (d) Variation of relative resistance under stretch-release cycles with a strain of 20% at 30, 60, 90, and 120 mm min−1 strain rates. (e) The relative resistance response during a single cycle, ranging from 0% to 25% and back, with 5% increments. (f) Response and recovery time at 1% strain. (g) Long-term performance stability over 1000 stretching cycles at 40% strain. | |
The strain sensing of GLWT fibers was measured through a stress–strain experiment (Fig. 3b). The gauge factor (GF) is commonly used to evaluate stretchable strain sensors, and it is defined as the ratio of the change in resistance to the change in strain:
|  | (1) |
In
eqn (1),
R0 represents the resistance, while
ε denotes the applied force. From
Fig. 3b, under strain, the resistance of GLWT fibers increases in a monotonically increasing manner, with two distinct segments: a GF value of 43 in the range of 0–50% strain and a GF value of 130 in the range of 50–80% strain. GLWT fibers produce a segmented linearly fitted resistance response. It is hypothesized that the resistance response curve in the initial stage (0–50% strain) primarily reflects the early process of the microcrack transition from closure to opening. During this stage, cracks are partially open, causing a moderate increase in resistance and resulting in a low level of GF. In the second linear range (50–80%), the resistance of the GLWT fibers increases very rapidly due to the continuous increase in strain and the widening of the crack gaps resulting in a reduction of the current path. As shown in Fig. S7,
† the current–voltage characteristics of GLWT fibers depend on strain. According to Ohm's law, current and voltage demonstrate a linear relationship, unaffected by the applied strain, highlighting the stable and reliable performance of the fibers under varying strain conditions.
As shown in Fig. 3c, the relative resistance changes are depicted when GLWT fibers were stretched to 20%, 40%, 60%, and 80% of maximum strain, resulting in a continuous and stable response to multi-cycle loading. Fig. 3d shows the resistance change of GLWT fibers at a constant 20% strain at different strain rates, showing how the relative resistance of GLWT fibers changes across different applied strain rates (30–120 mm min−1) is almost frequency independent and it suggests that GLWT fibers have good response stability, which is essential for long-term practical applications of strain sensing.
Fig. 3e illustrates that the GLWT fiber stretched ranging from 0% to 25%, followed by a return to 0% strain in 5% increments and held for 5 seconds at each step. With an increase in strain from 0% to 25%, the relative resistance gradually increases due to the cracking of the nano-gold leaf under strain. During the residence period, the sensor response stays stable. As the strain decreases from 25% to 0%, the relative resistance decreases as the cracks close, thanks to the excellent elasticity of the TPU matrix, the resistance almost returns to its initial value. The strain response of GLWT fibers at 1% strain was evaluated, and it was found that they responded within 180 ms and recovered within 220 ms, as illustrated in Fig. 3f, suggesting excellent load-unload behavior. A critical factor in evaluating the potential of GLWT fibers as strain sensors is their ability to recover their initial resistance after strain release. Fig. S8† illustrates the hysteresis behavior of GLWT fibers during a single stretch-release cycle over 0–20% strain. The nearly identical stretch and release curves demonstrated that the fiber had minimal hysteresis and insignificant structural damage during deformation and recovery.
Furthermore, stability of the GLWT fibers is vital for real-world applications. Therefore, multiple strain cycling experiments were carried out to assess their operational stability and long-term durability. As shown in Fig. 3g, 1000 stretch-and-release cycles were performed at 60 mm min−1 under 40% strain. It can be seen that GLWT fibers exhibit good durability and stability. As a gold-based conductive fiber, our GLWT fiber demonstrates high sensitivity over a wide strain range, outperforming previously reported gold-based strain sensors (Fig. S9†).56,64–70 Furthermore, to investigate the sensing mechanism, the resistive properties of the cracked mechanism were examined with the help of a simple equivalent model (Fig. S10†).71
3.3 Thermoplasticity of GLWT fibers
TPU is a multiblock copolymer that can be processed as a thermoplastic. Fig. S11† illustrates the FTIR spectral transmission curve of the TPU material employed in this study. The thermoplasticity of TPU is attributed to the presence of hydrogen bonding, as confirmed by the FTIR data.
The process for making helical GLWT fibers is shown in Fig. 4a. The GLWT fibers were uniformly wrapped around a glass rod (with a line spacing λ = d, where d is the diameter of the GLWT fibers), secured with tape at both ends, and the sample was placed in a drying oven for 40 minutes to allow the shape to set. The fibers were taken off the glass rod after cooling to room temperature, resulting in the helical fibers. Fig. 4b shows the optical image of the helical fibers at C = 8. In order to determine the optimum thermoplastic temperature, the thermoplastic treatment was carried out at a temperature of 150 °C, 160 °C, and 170 °C and the three cases are shown in Fig. S12.† At 150 °C, the GLWT fibers did not fully reach the desired thermoplastic shape. At 160 °C, a good thermoplastic shape was achieved, but at 170 °C, parts of the GLWT fibers melted due to excessive heat, damaging the helical structure. Therefore, a thermoplastic temperature of 160 °C was selected as the optimal treatment temperature.
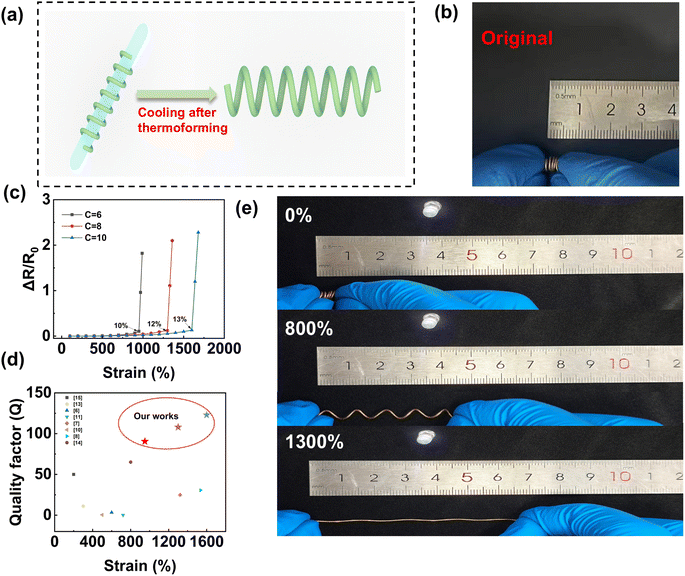 |
| Fig. 4 Thermoplasticity of GLWT fibers. (a) Schematic diagram of the method for imparting a helical structure on the GLWT fiber. (b) Photograph of the original helical GLWT fiber. (c) Variation of relative resistance with strain for fibers with different helical indices. (d) The Q-values of fibers with different helix configurations are compared with the Q-values of previously reported stretchable conductors. (e) Photograph of a wire with a helical index C of 8 stretched while connected to a light-emitting diode (LED). | |
By using glass rods with different diameters, the helical index C (the ratio between the helical diameter D and the fiber diameter d of GLWT) was modified. Fig. 4c illustrates the strain-relative resistance changes of helical GLWT fibers at various helix indices (6, 8 and 10). Resistance change is nearly imperceptible until the critical strain (defined as the strain where resistance changes abruptly) is reached. Beyond this point, the relative resistance change increases drastically. For helical fibers with helix indices of 6, 8, and 10, the critical strains were 950%, 1300%, and 1600%, with corresponding resistance changes of 10%, 12%, and 13%, respectively. The quality factor Q, which represents the ratio of the strain percentage to the percentage change in resistance, was utilized to assess the electrical performance of the helical fibers at the maximum strain. Fig. 4d compares the Q value of the helical fiber with the Q value of previously reported stretchable conductors.6–8,10,11,13–15 The helical GLWT fibers have a large strain range with Q values up to 123 (1600%), which outperforms most of the studies shown in Fig. 4d. To illustrate the application potential of the helical GLWT fiber, a conductor with a helix index of 8 was connected as a wire in an LED circuit. As shown in Fig. 4e, the LED maintained stable brightness at all strain levels, demonstrating the exceptional electrical stability of the helical GLWT fiber. In addition, the shape retention ability of the helical fibers under cyclic stretch loadings under large strains is very important for the long-term reliability of the electrodes. The results of the cyclic tensile test at 1000% strain show that the shape of helical GLWT fiber is almost the same as that of the initial state after 500 stretches, and it is difficult to observe deformation, which indicates that the helical electrode has a good shape retention ability (Fig. S13†).
3.4 Strain sensing applications of GLWT fibers
The GLWT fibers were attached to various locations on the volunteer's body, including the knees, wrists, and carotid arteries, to monitor deformation in different states. Fig. 5a presents the variation in relative resistance of the strain sensor while the volunteer was walking and running, with the sensor displaying excellent sensitivity and repeatability when the knee was bent to different degrees. Fig. 5b illustrates strain sensors' ability to detect wrist bending, showing stable and consistent resistance changes. A good strain sensor should be able to detect not only the generation of large strains but also small strains. When the GLWT fiber was attached to the carotid artery, the sensor successfully detected small-scale deformations associated with the pulse, providing key cardiovascular information. The signals were accurately recorded and provided key cardiac physiological data with three well-defined peaks, the first wave is the percussion wave (P-wave), the second is the tidal wave (T-wave), and the third is the diastolic wave (D-wave) (Fig. 5c). GLWT fibers are waterproof due to the protective WPU layer covering the surface of the fibers. As shown in Fig. 5d, the GLWT fibers were mounted on the index finger of a volunteer to simulate the resistance response at two frequencies (1 Hz and 2 Hz) by bending the finger to produce a change in resistance. The results show that the GLWT fibers perform reliably underwater and exhibit excellent stability.
 |
| Fig. 5 Strain sensing applications of GLWT fibers. (a) Monitoring of knee flexion during walking and running. (b) Monitoring of wrist flexion. (c) Detection of carotid artery changes. (d) Finger flexion changes at different frequencies in water. (e) Physical picture of a smart glove. (f) Gesture recognition of the smart glove. | |
A simple smart sensing glove can be manufactured by sewing 5 GLWT fibers to the joints of 5 fingers (Fig. 5e). When making different gestures, the sensors will output different signals. Here we demonstrated four simple gestures (OK, STOP, YES, and GOOD), and the results are shown in Fig. 5f. Different gestures have different signal strengths, allowing them to be distinguished based on these intensity changes. By capturing these gesture signals and integrating them with current artificial intelligence (AI) recognition technologies, there is significant potential for applications in human motion detection, sign language recognition, and AI-driven systems.
3.5 Capacitive strain sensor
The capacitive strain sensor was fabricated by adding a second layer of the nano-gold leaf on top of an existing layer of the nano-gold leaf (Fig. 6a). As shown in Fig. 6b, the cross-sectional energy dispersive X-ray spectroscopy (EDS) image of the capacitive sensor reveals that the upper and lower nano-gold leaf electrodes are uniformly adhered to the WPU interlayer, with the middle WPU serving as the dielectric layer.
 |
| Fig. 6 Capacitive strain sensor. (a) Capacitive strain sensor fabrication process. (b) Cross-sectional SEM image and the EDS image of the capacitive strain sensor. (c) Variation of relative capacitance as a function of strain. (d) Recovery time of response at 1% strain. (e) Variation of relative capacitance for different cyclic strains. (f) Cycling for 200 times at 20% strain. (g) Variation of the relative capacitance of the fiber for different angles of finger bending. | |
The sensor exhibits a linear gauge factor (GF) ranging from 0.8 to 60% strain (Fig. 6c), though the theoretical GF value of 1 was not reached due to cracking issues at the electrode interfaces. The sensor has a high linear correlation (R2 = 0.999). The capacitive sensor exhibited a response time of around 200 ms and a recovery time of approximately 220 ms when the fiber was stretched to 1% strain, held for 1 second, and then released, as shown in Fig. 6d. Meanwhile, the sensing performance of the capacitive sensor under different strains was investigated. The sensor exhibits good repeatability and reliable strain recognition for strains ranging from 10% to 50% (Fig. 6e). Additionally, 200 strain release cycles were performed at 20% strain, demonstrating excellent long-term durability and stability (Fig. 6f). The sensor was also successfully used to monitor finger flexion. As shown in Fig. 6g, capacitance changes correlate with finger bending, and the sensor could accurately indicate the degree of bending based on these capacitance variations, providing fast and precise feedback.
3.6 Pressure sensor array
In addition, a 5 × 5 pressure sensing array was integrated by placing 10 individual GLWT fibers onto an acrylic plate (10 cm × 10 cm), with WPU used to adhere to the fibers at their contact points. Each GLWT fiber was spaced 15 mm apart. The sensor array uses a single point measurement mode, and each measurement unit operates independently. Therefore, in the test process, only the two electrodes of the tested unit need to be separately connected to the circuit. Since each sensor in the array operates independently, we selected one of them for testing. Fig. S14† shows the response curve of its relative capacitance as a function of pressure. Fig. 7a illustrates the operating principle of a single sensor, where capacitance changes occur as pressure reduces the thickness of the dielectric layer. To minimize interference between the electrode arrays and facilitate testing, the electrodes on both sides were bonded with insulating tape. The letters “U”, “S”, and “T” (weight: 2.1 g, 2.8 g, and 1.6 g and density: 1.24 g cm−3) were printed using a 3D printer. These three letters were placed on the sensor array, and the capacitive signals were collected at each point. Fig. 7b–d show histograms of the pressure response, which correspond to the shape of the three letters. This observation indicates that the integrated sensor array can perform multi-point measurements and recognize patterns.
 |
| Fig. 7 (a) Physical diagram of the pressure sensing array and the operating principle of the single sensing units. (b)–(d) Response of the letters U, S, and T pressed on the sensor array. (e) Pressure response of the earphone charging box. (f) Pressure response of a plastic key-shaped object. (g) Pressure response of a loop of PI tape. | |
Next, the 5 × 5 sensor array was used to monitor the pressure distribution of everyday objects and measure changes in capacitance signals after loading. An earphone charging box (46.6 g), a plastic key-shape object (3.2 g), and a loop of PI tape (6.1 g) were placed on the sensor array, and the capacitance changes at each point were recorded and plotted as histograms, as shown in Fig. 7e, f, and g, respectively. The results demonstrate that the shape of each item can be clearly distinguished based on the relative capacitance change.
4. Conclusions
In summary, our new rolling adhesive method was successfully proposed for preparing flexible conductive fibers with conductive sheath layers of nano-gold leaves, which may be applied for adhering other kinds of metal leaves onto the surface of fiber. The GLWT fibers can be prepared on a large scale through seamless rolling adhesion technology. We had developed GLWT fibers using the rolling adhesive method, which had high sensitivity (GF = 130) at high strain (80%). We also fabricated a GLWT fiber with two gold-leaf sheath layers, which can effectively monitor the movement of various body parts. The GLWT fibers can be thermoformed molded into C-10 helical structures with excellent electrical properties (Q = 123 at 1600% strain) and be used as stretchable electrodes. Additionally, the GLWT fibers can be integrated into glove fabrics for smart gesture recognition. Furthermore, multiple GLWT fibers can be combined to form capacitive pressure sensing arrays recognizing the shape of objects. The results show that the rolling adhesive method is environmentally friendly and sustainable and shows great application potential in wearable electronic devices.
Data availability
The data that support the findings of this study are available upon request from the first and corresponding author upon reasonable request. Requests may be sent to E-mail: bsheng@usst.edu.cn.
Conflicts of interest
The authors declare that they have no known competing financial interests or personal relationships that could have appeared to influence the work reported in this paper.
Acknowledgements
This work was supported by the Natural Science Foundation of Shanghai (19ZR1436100) and the National Natural Science Foundation of China (11105149).
References
- J. W. Chen, Y. T. Zhu, X. H. Chang, D. Pan, G. Song, Z. H. Guo and N. Naik, Adv. Funct. Mater., 2021, 31, 2104686 CrossRef CAS
.
- W. Gao, S. Emaminejad, H. Y. Y. Nyein, S. Challa, K. V. Chen, A. Peck, H. M. Fahad, H. Ota, H. Shiraki, D. Kiriya, D. H. Lien, G. A. Brooks, R. W. Davis and A. Javey, Nature, 2016, 529, 509–514 CrossRef CAS PubMed
.
- T. Q. Trung and N. E. Lee, Adv. Mater., 2016, 28, 4338–4372 CrossRef CAS PubMed
.
- M. Y. Li, Y. T. Wang, S. H. Sun, C. B. Wan, Y. L. Wang, R. K. Li, A. C. Tang and X. Ju, J. Mater. Chem. A, 2024, 12, 29241–29253 RSC
.
- X. Y. Jiang, Y. Cheng, L. J. Shi, J. Sun and R. R. Wang, Angew. Chem., Int. Ed., 2024, 63, e202411418 CAS
.
- C. Choi, J. H. Kim, H. J. Sim, J. Di, R. H. Baughman and S. J. Kim, Adv. Energy Mater., 2017, 7, 1602021 CrossRef
.
- Z. F. Liu, S. Fang, F. A. Moura, J. N. Ding, N. Jiang, J. Di, M. Zhang, X. Lepró, D. S. Galvao, C. S. Haines, N. Y. Yuan, S. G. Yin, D. W. Lee, R. Wang, H. Y. Wang, W. Lv, C. Dong, R. C. Zhang, M. J. Chen, Q. Yin, Y. T. Chong, R. Zhang, X. Wang, M. D. Lima, R. Ovalle-Robles, D. Qian, H. Lu and R. H. Baughman, Science, 2015, 349, 400–404 CrossRef CAS PubMed
.
- J. Woo, H. Lee, C. Yi, J. Lee, C. Won, S. Oh, J. Jekal, C. Kwon, S. Lee, J. Song, B. Choi, K. I. Jang and T. Lee, Adv. Funct. Mater., 2020, 30, 1910026 CrossRef CAS
.
- S. S. Zhao, J. Chen, J. Y. Zhou, S. X. Shi, M. Hou and B. Sheng, Adv. Mater. Technol., 2023, 8, 2201951 CrossRef CAS
.
- X. Zhao, F. Chen, Y. H. Li, H. Lu, N. Zhang and M. M. Ma, Nat. Commun., 2018, 9, 3579 CrossRef PubMed
.
- S. Zhu, J. H. So, R. Mays, S. Desai, W. R. Barnes, B. Pourdeyhimi and M. D. Dickey, Adv. Funct. Mater., 2013, 23, 2308–2314 CrossRef CAS
.
- Y. N. Hao, Q. Y. Yan, H. J. Liu, X. Y. He, P. H. Zhang, X. H. Qin, R. R. Wang, J. Sun, L. M. Wang and Y. Cheng, Adv. Funct. Mater., 2023, 33, 2303881 Search PubMed
.
- F. Q. Sun, M. W. Tian, X. T. Sun, T. L. Xu, X. Q. Liu, S. F. Zhu, X. J. Zhang and L. J. Qu, Nano Lett., 2019, 19, 6592–6599 Search PubMed
.
- H. Wang, Z. Liu, J. Ding, X. Lepro, S. Fang, N. Jiang, N. Yuan, R. Wang, Q. Yin, W. Lv, Z. Liu, M. Zhang, R. Ovalle-Robles, K. Inoue, S. Yin and R. H. Baughman, Adv. Mater., 2016, 28, 4946 CrossRef CAS PubMed
.
- L. J. Zheng, M. M. Zhu, B. H. Wu, Z. L. Li, S. T. Sun and P. Y. Wu, Sci. Adv., 2021, 7, eabg4041 CrossRef CAS PubMed
.
- Q. Liu, J. Chen, Y. R. Li and G. Q. Shi, ACS Nano, 2016, 10, 7901–7906 CrossRef CAS PubMed
.
- T. Sekitani, H. Nakajima, H. Maeda, T. Fukushima, T. Aida, K. Hata and T. Someya, Nat. Mater., 2009, 8, 494–499 CrossRef CAS PubMed
.
- T. Sekitani and T. Someya, Adv. Mater., 2010, 22, 2228–2246 CrossRef CAS PubMed
.
- F. Huang, J. Y. Hu and X. Yan, Textiles, 2022, 2, 81–111 CrossRef
.
- Z. H. Yang, Z. R. Zhai, Z. M. Song, Y. Z. Wu, J. H. Liang, Y. F. Shan, J. R. Zheng, H. C. Liang and H. Q. Jiang, Adv. Mater., 2020, 32, 1907495 CrossRef CAS PubMed
.
- Y. Cheng, R. R. Wang, J. Sun and L. Gao, Adv. Mater., 2015, 27, 7365–7371 CrossRef CAS PubMed
.
- Z. Q. Wen, J. Y. Zhou, S. S. Zhao, S. B. Chen, D. W. Zhang and B. Sheng, J. Mater. Chem. C, 2023, 11, 12163–12173 RSC
.
- B. Y. Liu, B. X. Lan, L. J. Shi, Y. Cheng, J. Sun and R. R. Wang, ACS Appl. Mater. Interfaces, 2024, 16, 36821–36831 CrossRef CAS PubMed
.
- X. Y. Yue, Y. Y. Jia, X. Z. Wang, K. K. Zhou, W. Zhai, G. Q. Zheng, K. Dai, L. W. Mi, C. T. Liu and C. Y. Shen, Compos. Sci. Technol., 2020, 189, 108038 CrossRef CAS
.
- X. H. Liu, J. L. Miao, Q. Fan, W. X. Zhang, X. W. Zuo, M. W. Tian, S. F. Zhu, X. J. Zhang and L. J. Qu, Adv. Fiber Mater., 2022, 4, 361–389 CrossRef CAS
.
- G. J. Zhu, P. G. Ren, J. Wang, Q. Duan, F. Ren, W. M. Xia and D. X. Yan, ACS Appl. Mater. Interfaces, 2020, 12, 19988–19999 CrossRef CAS PubMed
.
- L. H. Wang, M. W. Tian, Y. Y. Zhang, F. Q. Sun, X. J. Qi, Y. M. Liu and L. J. Qu, J. Mater. Sci., 2020, 55, 6187–6194 CrossRef CAS
.
- Y. M. Xu, X. X. Xie, H. Huang, Y. Wang, J. R. Yu and Z. M. Hu, J. Mater. Sci., 2021, 56, 2296–2310 CrossRef CAS
.
- J. J. Pan, B. W. Hao, W. F. Song, S. X. Chen, D. Q. Li, L. Luo, Z. G. Xia, D. S. Cheng, A. C. Xu, G. M. Cai and X. Wang, Composites, Part B, 2020, 183, 107683 CrossRef CAS
.
- Y. H. Li, B. Zhou, G. Q. Zheng, X. H. Liu, T. X. Li, C. Yan, C. B. Cheng, K. Dai, C. T. Liu, C. Y. Shen and Z. H. Guo, J. Mater. Chem. C, 2018, 6, 2258–2269 RSC
.
- Z. Wang, Z. Wang and Z. J. Pan, Curr. Opin. Solid State Mater. Sci., 2018, 22, 213–228 CrossRef
.
- C. L. He, F. C. Liang, L. Veeramuthu, C. J. Cho, J. S. Benas, Y. R. Tzeng, Y. L. Tseng, W. C. Chen, A. Rwei and C. C. Kuo, Adv. Sci., 2021, 8, 2102275 CrossRef CAS PubMed
.
- J. H. Lee, T. Lim, K. Seo, J. Park, J. Yang, S. M. Jeong and S. Ju, NPG Asia Mater., 2022, 14, 13 CrossRef CAS
.
- S. Lee, S. Shin, S. Lee, J. Seo, J. Lee, S. Son, H. J. Cho, H. Algadi, S. Al-Sayari, D. E. Kim and T. Lee, Adv. Funct. Mater., 2015, 25, 3114–3121 CrossRef CAS
.
- M. Park, J. Im, M. Shin, Y. Min, J. Park, H. Cho, S. Park, M. B. Shim, S. Jeon, D. Y. Chung, J. Bae, J. Park, U. Jeong and K. Kim, Nat. Nanotechnol., 2012, 7, 803–809 CrossRef CAS
.
- L. Veeramuthu, C. J. Cho, M. Venkatesan, G. R. Kumar, H. Y. Hsu, B. X. Zhuo, L. J. Kau, M. A. Chung, W. Y. Lee and C. C. Kuo, Nano Energy, 2022, 101, 107592 CrossRef CAS
.
- J. F. Gao, M. J. Hu and R. K. Y. Li, J. Mater. Chem., 2012, 22, 10867–10872 RSC
.
- Y. F. Yu, G. C. Zheng, K. Dai, W. Zhai, K. K. Zhou, Y. Y. Jia, G. Q. Zheng, Z. C. Zhang, C. T. Liu and C. Y. Shen, Mater. Horiz., 2021, 8, 1037–1046 RSC
.
- J. Y. Zhou, S. S. Zhao, L. Tang, D. W. Zhang and B. Sheng, ACS Appl. Mater. Interfaces, 2023, 15, 57533–57544 CAS
.
- J. Y. Xiao, Y. Xiong, J. Chen, S. S. Zhao, S. B. Chen, B. L. Xu and B. Sheng, Nanoscale, 2022, 14, 1962–1970 RSC
.
- S. Y. Kim, H. E. Gang, G. T. Park, H. B. Jeon and Y. G. Jeong, Adv. Eng. Mater., 2021, 23, 2100548 CrossRef CAS
.
- A. B. Appiagyei, J. Banua and J. I. Han, J. Ind. Eng. Chem., 2021, 100, 372–382 CrossRef CAS
.
- X. Lu, W. H. Shang, G. K. Chen, H. F. Wang, P. Tan, X. B. Deng, H. F. Song, Z. Y. Xu, J. Q. Huang and X. C. Zhou, ACS Appl. Electron. Mater., 2021, 3, 1477–1488 CrossRef CAS
.
- X. J. Du, M. W. Tian, G. S. Sun, Z. Q. Li, X. J. Qi, H. T. Zhao, S. F. Zhu and L. J. Qu, ACS Appl. Mater. Interfaces, 2020, 12, 55876–55883 CrossRef CAS PubMed
.
- Y. M. Kim and H. C. Moon, Adv. Funct. Mater., 2020, 30, 1907290 CrossRef CAS
.
- H. G. Wei, D. S. Kong, T. Li, Q. Z. Xue, S. Y. Wang, D. P. Cui, Y. D. Huang, L. Wang, S. M. Hu, T. Wan and G. Yang, ACS Sens., 2021, 6, 2938–2951 CrossRef CAS PubMed
.
- B. Z. Zhou, Z. X. Liu, C. C. Li, M. S. Liu, L. Jiang, Y. F. Zhou, F. L. Zhou, S. J. Chen, S. Jerrams and J. Y. Yu, Adv. Electron. Mater., 2021, 7, 2100233 CrossRef CAS
.
- S. Jang, J. Y. Choi, E. S. Yoo, D. Y. Lim, J. Y. Lee, J. K. Kim and C. Pang, Composites, Part B, 2021, 210, 108674 CrossRef CAS
.
- M. S. Sadi, M. Y. Yang, L. Luo, D. S. Cheng, G. M. Cai and X. Wang, Cellulose, 2019, 26, 6179–6188 CrossRef CAS
.
- R. Yin, S. Y. Yang, Q. M. Li, S. D. Zhang, H. Liu, J. Han, C. T. Liu and C. Y. Shen, Sci. Bull., 2020, 65, 899–908 CrossRef CAS PubMed
.
- S. Béfahy, S. Yunus, T. Pardoen, P. Bertrand and M. Troosters, Appl. Phys. Lett., 2007, 91, 141911 CrossRef
.
- J. Cao, X. S. Liu, J. Qiu, Z. F. Yue, Y. Li, Q. Xu, Y. Chen, J. W. Chen, H. F. Cheng, G. Z. Xing, E. M. Song, M. Wang, Q. Liu and M. Liu, Nat. Commun., 2024, 15, 1116 CrossRef CAS PubMed
.
- T. Q. Tran, J. K. Y. Lee, A. Chinnappan, N. H. Loc, L. T. Tran, D. X. Ji, W. Jayathilaka, V. V. Kumar and S. Ramakrishna, J. Mater. Sci. Technol., 2020, 42, 46–53 CrossRef CAS
.
- X. T. Li, K. H. Koh, M. Farhan and K. W. C. Lai, Nanoscale, 2020, 12, 4110–4118 RSC
.
- B. W. Zhu, S. Gong and W. L. Cheng, Chem. Soc. Rev., 2019, 48, 1668–1711 RSC
.
- Z. Y. Liu, D. P. Qi, G. Y. Hu, H. Wang, Y. Jiang, G. Chen, Y. F. Luo, X. J. Loh, B. Liedberg and X. D. Chen, Adv. Mater., 2018, 30, 1704229 CrossRef PubMed
.
-
H. Jeong and J. M. Seo, IEEE, Berlin, Germany, 2019, pp. 1286–1289 Search PubMed
.
- P. Prasertying, N. Jantawong, T. Sonsa-ard, T. Wongpakdee, N. Khoonrueng, S. Buking and D. Nacapricha, Analyst, 2021, 146, 1579–1589 RSC
.
- M. S. F. Santos, W. A. Ameku, I. G. R. Gutz and T. Paixao, Talanta, 2018, 179, 507–511 CrossRef CAS PubMed
.
- I. Podunavac, M. Kukkar, V. Leguillier, F. Rizzotto, Z. Pavlovic, L. Janjusevic, V. Costache, V. Radonic and J. Vidic, Talanta, 2023, 259, 124557 CrossRef CAS PubMed
.
- J. A. Hondred, Z. T. Johnson and J. C. Claussen, J. Mater. Chem. C, 2020, 8, 11376–11388 RSC
.
- Y. K. Wang, Z. X. Pei, M. S. Zhu, Z. X. Liu, Y. Huang, Z. H. Ruan, Y. Huang, Y. Zhao, S. Y. Du and C. Y. Zhi, ACS Appl. Mater. Interfaces, 2018, 10, 21297–21305 CrossRef CAS PubMed
.
- T. Smith, J. Colloid Interface Sci., 1980, 75, 51–55 CrossRef CAS
.
- J.
F. Shu, R. R. Yang, Y. Q. Chang, X. Q. Guo and X. Yang, J. Alloys Compd., 2021, 879, 160466 CrossRef CAS
.
- Y. T. Chen, Y. Y. Wu, S. S. Mechael and T. B. Carmichael, Chem. Mater., 2019, 31, 1920–1927 CrossRef CAS
.
- G. H. Lim, N. E. Lee and B. Lim, J. Mater. Chem. C, 2016, 4, 5642–5647 RSC
.
- Y. Z. Bai, L. T. Yin, C. Hou, Y. L. Zhou, F. Zhang, Z. Y. Xu, K. Li and Y. A. Huang, Adv. Funct. Mater., 2023, 33, 2214119 CrossRef CAS
.
- H. L. Filiatrault, R. S. Carmichael, R. A. Boutette and T. B. Carmichael, ACS Appl. Mater. Interfaces, 2015, 7, 20745–20752 CrossRef CAS PubMed
.
- C. H. Kim, D. H. Lee, J. Youn, H. Lee and J. Jeong, Sci. Rep., 2021, 11, 14823 CrossRef CAS PubMed
.
- B. Zhang, J. Lei, D. P. Qi, Z. Y. Liu, Y. Wang, G. W. Xiao, J. S. Wu, W. N. Zhang, F. W. Huo and X. D. Chen, Adv. Funct. Mater., 2018, 28, 1801683 CrossRef
.
- X. Y. Qu, Y. C. Wu, P. Ji, B. X. Wang, Q. Q. Liang, Z. L. Han, J. Li, Z. T. Wu, S. Y. Chen, G. L. Zhang and H. P. Wang, ACS Appl. Mater. Interfaces, 2022, 14, 29167–29175 CrossRef CAS PubMed
.
|
This journal is © The Royal Society of Chemistry 2025 |
Click here to see how this site uses Cookies. View our privacy policy here.