DOI:
10.1039/D1RA01809D
(Paper)
RSC Adv., 2021,
11, 16026-16033
In silico identification of potential SARS COV-2 2′-O-methyltransferase inhibitor: fragment-based screening approach and MM-PBSA calculations†
Received
7th March 2021
, Accepted 12th April 2021
First published on 29th April 2021
Abstract
In the present era, there are many efforts trying to face the emerging and successive waves of the COVID-19 pandemic. This has led to considering new and unusual targets for SARS CoV-2. 2′-O-Methyltransferase (nsp16) is a key and attractive target in the SARS CoV-2 life cycle since it is responsible for the viral RNA protection via a cap formation process. In this study, we propose a new potential inhibitor for SARS COV-2 2′-O-methyltransferase (nsp16). A fragment library was screened against the co-crystal structure of the SARS COV-2 2′-O-methyltransferase complexed with Sinefungin (nsp16 – PDB ID: 6WKQ), and consequently the best proposed fragments were linked via a de novo approach to build molecule AP-20. Molecule AP-20 displayed a superior docking score to Sinefungin and reproduced the key interactions in the binding site of 2′-O-methyltransferase. Three molecular dynamic simulations of the 2′-O-methyltransferase apo structure and its complexed forms with AP-20 and Sinefungin were performed for 150 nano-seconds to provide insights on the dynamic nature of such setups and to assess the stability of the proposed AP-20/enzyme complex. AP-20/enzyme complex demonstrated better stability for the ligand–enzyme complex compared to Sinefungin in a respective setup. Furthermore, MM-PBSA binding free energy calculations showed a better profile for AP-20/enzyme complex compared to Sinefungin/enzyme complex emphasizing the potential inhibitory effect of AP-20 on SARS COV-2 2′-O-methyltransferase. We endorse our designed molecule AP-20 to be further explored via experimental evaluations to confront the spread of the emerging COVID-19. Also, in silico ADME profiling has ascribed to AP-20 an excellent safety and metabolic stability profile.
1. Introduction
SARS-CoV-2 is positive-sense, single-stranded RNA betacoronavirus, responsible for the outbreak of the respiratory disease known as Coronavirus disease-2019 (COVID-19).1,2 In March 2020, COVID-19 was declared a pandemic threat by the World Health Organization (WHO).3 The outbreak triggered the scientific community to respond promptly by focusing their efforts on accelerating drug discovery using computational methods, as seen in the enormous amount of recent literature.4–29 Currently, due to successive pandemic waves of COVID-19, under-represented targets are to be studied further to minimize resistance mechanisms and to tackle the evolving pandemic. Among these targets, 2′-O-methyltransferase (nsp16) stands out as a promising one.
The SARS COV-2 2′-O-methyltransferase (nsp16) has a main function in protecting the viral RNA from the cellular innate immunity through participation in the formation of a specific arrangement at the 5′ end of the RNA molecule. This arrangement is referred to as RNA cap, resembling the native mRNA of the host cells. This leads to stabilizing the RNA and secures its translation. The cap formation starts when 5′-RNA triphosphatase removes a γ-phosphate from a 5′-triphosphate end of the nascent RNA. Then, to the formed 5′-diphosphate end of RNA, guanylyltransferase attaches a guanosine monophosphate (GMP). Two phases of methylation are accomplished by two different enzymes, nsp14 which inserts a methyl group at N-7 of the GTP nucleobase (N-7 methyltransferase) and nsp16 which inserts a methyl group at C2′-O of the next nucleotide.30,31 This procedure is vital for RNA solidity, avoiding its degradation by the host.32 The procedure and significance of cap formation emphasizing that SARS COV-2 2′-O-methyltransferase (nsp16) is an encouraging target.
The inhibition of the viral nsp16 activity, and the resulting creation of an incompletely capped RNA, may possibly stimulate the recognition of viral RNA by pathogen-associated molecular patterns (PAMPs) and stimulate a host antiviral response.3 Therefore, its targeting can be an augmenting strategy for developing a remedy for COVID-19 infection, as reported in different studies.33–35 As a continuation to our efforts in computational discovering novel entities as potential inhibitors for SARS COV-2 2′-O-methyltransferase,36 we introduce in this study a Fragment-based approach to design a potential inhibitor for nsp16. Our approach is supplemented by molecular docking study and supported by three medium-range molecular dynamics simulations for 150 nano-seconds. The designed compound AP-20 is a fruitful template that is worth experimental validation via other studies.
2. Results and discussion
2.1. Fragment based drug design (FBDD)
FBDD is a technique aiming in the identification of potential fragments that strongly bind the target active site prior utilizing those fragments in the design of novel inhibitors. The technique relies on three main strategies; (a) fragment linking; where the retrieved fragment from the fragment search are linked together to yield single compound. This technique is applied when the fragments are bound to different regions in the active site apart from each other, (b) fragment growing; where a central fragment that matches the role of three is increased in size for enhanced binding strength and interactions with the required target, and (c) fragment merging; where fragments that bound to the same region in the active site, are joined to give a superior interacting fragment.37
FBDD has many advantages in the discovery of drug candidates because it provides full and efficient exploration of the binding site, and always generates fragments of low complexity. Those fragments are always ideal to be a start for identifying compounds with desired interaction pattern and possible synthetic feasibility. Despite our previous success in identifying potential inhibitor for SARS COV-2 2′-O-methyl transferase from screening of ZINC database, the mentioned advantages of FBBD encouraged us to utilize it in this study.
In the current work of designing a novel SARS COV-2 2′-O-methyltransferase inhibitor, linking strategy was the most suitable technique using the de novo receptor approach available in DS software. By default, the constructed cavity around the binding of the co-crystalized Sinefungin was subdivided by the technique, allowing full and efficient screening of the fragment database through the entire binding site. The DS software contains Ludi database (as fragments source) that consists of 1053 diverse fragments. All the fragments in the database obey the rule of three with molecular weight less than (300 Da) and are mainly derived from the standard amino acids. Although the Ludi library is small in size, it contains highly diverse fragments, which are capable of engaging different interactions in every site in the binding pocket. Firstly, the binding of the screened fragments was evaluated using the “Energy estimate 3” algorithm as a scoring function. The algorithm allows the passing only for fragments that induce negative change in the receptor free energy after binding, as this highlights their ability to induce favorable binding with target. The previous step resulted into the identification of 831 potential fragments with high binding affinity to the receptor. Further confirmation for the fragments potentiality was conducted by docking each fragment into the receptor cavity using the MCSS algorithm.38 After visual inspection of the binding of each retrieved fragment, the best six fragments having the strongest binding interactions with the enzyme active site have been selected (Fig. 1A). Finally, the selected fragments were then linked together to construct the designed compound AP-20 (Fig. 1B). Furthermore, another five potential inhibitors (W1–5) were designed from the less active fragments (ESI†).
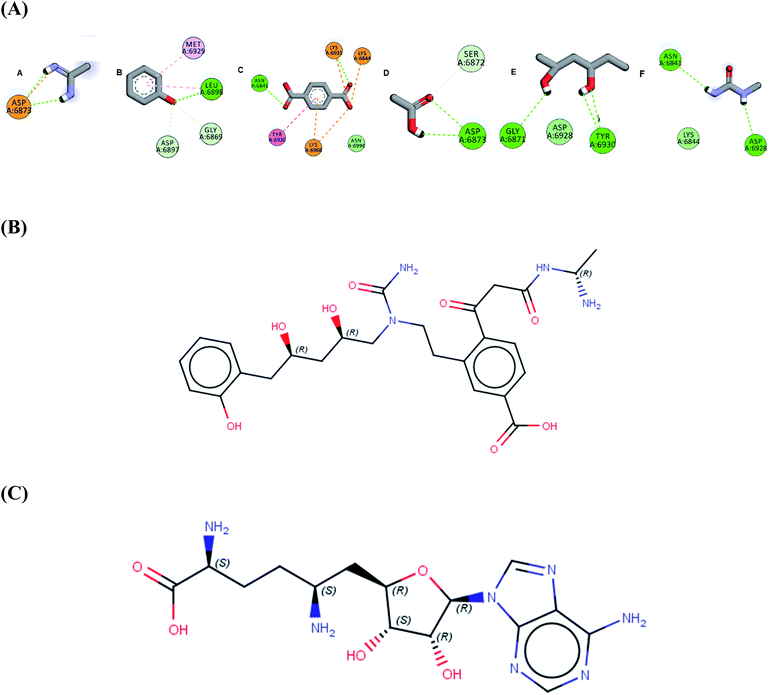 |
| Fig. 1 (A) The six selected fragments after de novo FBDD (B) the 2D structure of AP-20 after linking the fragments (C) the 2D structure of Sinefungin. | |
2.2. Docking
Docking strategy is one of the most valuable techniques in CADD studies, providing many useful applications including prediction of the binding mode between a ligand and its target, ranking a library of compounds based on their docking scores, and correlating those scores with potential activity. Also, docking has an important role in characterizing the most significant amino acids controlling the activity of the ligands. Moreover, visualizing the interaction images resulting from docking software gives insights and guides for the optimization of the existing ligands to yield compounds with better affinity. Accordingly, we aimed to determine the binding mode of designed compounds with SARS-COV-2 methyl transferase active site by applying a docking simulation experiment using Vina Autodock Software. Vina achieves more accurate binding predictions with twice-speed compared to the regular Autodock.39 Pose retrieval validation step was conducted through re-docking the co-crystalized ligand (Sinefungin) to the SARS-COV-2 methyl transferase which resulted in a calculated RMSD between the docked and co-crystalized poses of Sinefungin of 1.1 Å (Fig. 2).
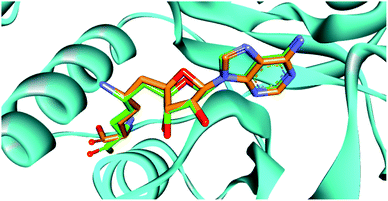 |
| Fig. 2 The superimposition between the re-docked pose (green) and the co-crystalized ligand (orange), showing similar orientation in the binding site. | |
The docking results predicted favorable binding scores for both Sinefungin and the designed compounds. AP-20, W-1, W-2, W-3, W-4 and W-5 achieved a score of −11.1, −9.5, −8.8, −8.1, −8.0 and −7.7 kcal per mole, respectively, vs. −7.5 kcal per mole for Sinefungin. This highlights the good potentiality of the designed compounds to inhibit the SARS-COV-2 methyl transferase, even better than Sinefungin. A deep look at the predicted binding mode of AP-20 within the active site, as shown in Fig. 3, reveals the ability of AP-20 to engage in a large number of diverse interaction types within the active site. For example, AP-20 was able to interact via hydrogen-bonding with residues ASP6873, ASP6897, ASP6928, TYR6930, GLY6869, GLY6871, ASN6841, LYS6935 and LYS6968, as well as, ionic interactions with residues LYS6935, LYS6968, and LYS6844. Moreover, AP-20 was involved in many non-polar interactions with residues MET6929, LEU6898, LYS6968 and TYR456. The detailed interactions of AP-20 within SARS-COV-2 methyl transferase active site were summarized in Table 1. The predicted binding modes for compounds W1–5 have been provided in the ESI.†
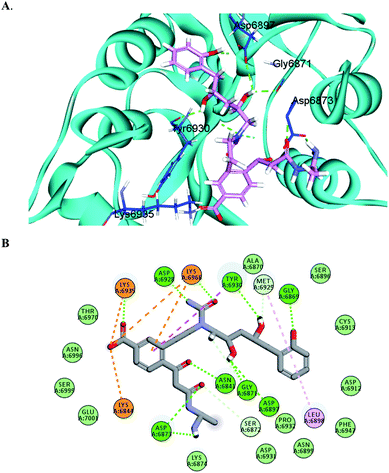 |
| Fig. 3 3D (A) and 2D (B) interaction diagrams between AP-20 and methyl transferase enzyme; the ligand is shown in stick presentation and relevant residues at the active site are shown in line presentations. Bond lengths between the ligand and interacting residues are shown in the 3D diagram. | |
Table 1 The types and distances of the formed interactions between AP-20 and the methyl transferase active site
Bond type |
Distance Å |
Bond type |
Distance Å |
Hydrogen bond with TYR6930 |
2.15 |
Ionic interaction with LYS6844 |
4.83 |
Hydrogen bond with ASP6928 |
2.93 |
Ionic interaction with LYS6935 |
4.36 |
Hydrogen bond with ASP6873 |
1.17 |
Ionic interaction with LYS6968 |
5.26 |
Hydrogen bond with ASP6873 |
2.28 |
Pi-cation interaction with LYS6968 |
4.69 |
Hydrogen bond with ASP6897 |
2.60 |
Pi–Pi interaction with TYR6930 |
5.69 |
Hydrogen bond with LYS6968 |
1.92 |
Pi-alkyl interaction with MET6929 |
4.57 |
Hydrogen bond with ASN6841 |
2.07 |
Non-classical hydrogen bond with MET6929 |
2.54 |
Hydrogen bond with GLY6869 |
2.83 |
Pi-alkyl interaction with LEU6898 |
4.52 |
Hydrogen bond with LYS6935 |
2.02 |
Non-classical hydrogen bond with GLY6871 |
3.07 |
Hydrogen bond with GLY6871 |
2.10 |
Non-classical hydrogen bond with ASP6897 |
2.08 |
Non-classical hydrogen bond with SER6872 |
2.48 |
Non-classical hydrogen bond with GLY6869 |
2.27 |
2.3. In silico ADME profiling
Compounds are considered potential drugs when they gather acceptable pharmacodynamic and pharmacokinetic profiles. Considering the promising potential inhibitory activity of AP-20, which is attributable to its strong binding interactions, it was worthy to assess its physico-chemical properties using the online Swiss ADME server. The server predicted that AP-20 has a high metabolic stability with no interaction with any hepatic cytochrome enzymes. Accordingly, AP-20 may have no hepatotoxicity and could be safely used concurrently with other COVID-19 drugs. AP-20 is a polar compound with log
P and total polar surface area (TPSA) equal 0.19 and 216.51 A2, respectively. Also, it has a molecular weight of 530 dalton. These physico-chemical properties make the penetration of AP-20 to the BBB or the placenta is very difficult, and thus it could have excellent safety profile. Other parameters are provided in Table S1 in the (ESI†).
2.4. Molecular dynamics (MD) simulations
Molecular dynamic simulations have been inevitable in many computational studies of drug discovery. For instance, the discovery of novel compounds for drug targets, investigating the nature of macromolecules and explaining the effect of certain mutations on drug resistances40–43. To support our protocol so far, and to provide insights on the stability of the predicted binding mode of AP-20 in the binding site of COVID-19 methyl transferase, we conducted three molecular dynamic simulation experiments.
Also, we aimed from the MD simulations to identify and study the dynamic nature of the SARS COV-2 2′-O-methyltransferase (nsp16) and correlate this to its key biological role in the virus life cycle.
2.4.1. RMSD analysis and hydrogen bond monitoring. The ultimate endeavor for SARS COV-2 2′-O-methyltransferase (nsp16) is to prevent the degradation of the viral RNA through the process of cap formation as above mentioned in the introduction section. Thus, the enzyme must have sufficient degree of flexibility and dynamicity, to deliver its intended function.44,45 So, the performed simulation experiment for the free SARS COV-2 2′-O-methyltransferase provided a mean for comparison with the two enzyme-ligand simulation experiments. The SARS COV-2 2′-O-methyltransferase (nsp16) was found to be highly dynamic as proven from the calculated RMSD that reached 4.8 Å for all the residues of the free enzyme, Fig. 4.
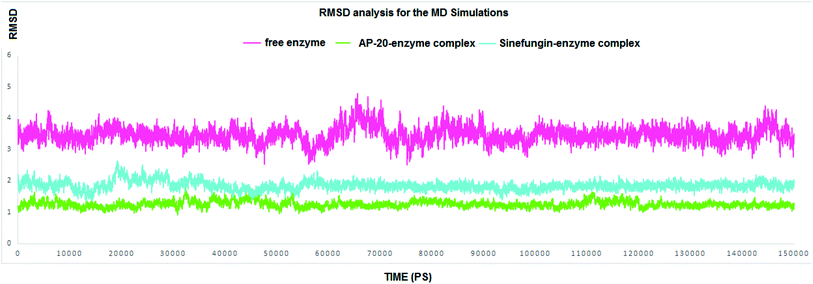 |
| Fig. 4 The RMSD for all the residues in the entire MD simulations. SARS COV-2 2′-O-methyltransferase without a ligand is in pink line; Sinefungin-SARS COV-2 2′-O-methyltransferase complex is in blue line, and AP-20-SARS COV-2 2′-O-methyltransferase complex is in green line. | |
In this section, our prime goal was to provide the evidence on the binding stability of the designed compound to COVID-19 methyl transferase, and to give an account to the proven degrees of flexibility of the binding site of nsp16. Accordingly, simulating the enzyme–inhibitor complex could provide a very consistent and reliable parameter to evaluate its stability after the binding. Accordingly, we executed two MD simulations to monitor the dynamic behavior for both SARS COV-2 2′-O-methyltransferase (nsp16) complex with Sinefungin, and SARS COV-2 2′-O-methyltransferase (nsp16) complex with AP-20 through measuring the RMSD for both complexes.
RMSD values for both AP-20 and Sinefungin reached at their maximum dynamicity peaks 1.82 Å and 2.64 Å, respectively (Fig. 4). This indicates that AP-20 shows stronger binding to SARS COV-2 2′-O-methyltransferase (nsp16) even more than Sinefungin. Also, it was necessary to determine the stability of the interactions formed between AP-20 and SARS COV-2 2′-O-methyltransferase (nsp16) through the entire MD experiment. Various built-in commands in GROMACS were implemented to measure the stability of the formed hydrogen bonds between AP-20 and SARS COV-2 2′-O-methyltransferase (nsp16), as hydrogen bond should always maintain a distance between the hydrogen bond acceptor and the donor less than 3.5 Å. This distance criterion was maintained in all the formed hydrogen bonds between AP-20 and SARS COV-2 2′-O-methyltransferase (nsp16) indicating a stable binding mode, Table 2.
Table 2 The average distances of all the hydrogen bonds formed between AP-20 and its target through the entire MD simulation
Hydrogen bond name |
Average distance (Å) ± SD |
Hydrogen bond with TYR6930 |
2.27 ± 0.18 |
Hydrogen bond with ASP6928 |
2.28 ± 0.15 |
Hydrogen bond with ASP6873 |
1.5 ± 0.07 |
Hydrogen bond with ASP6873 |
2.37 ± 0.10 |
Hydrogen bond with ASP6897 |
2.52 ± 0.16 |
Hydrogen bond with LYS6968 |
1.90 ± 0.05 |
Hydrogen bond with ASN6841 |
2.14 ± 0.15 |
Hydrogen bond with GLY6869 |
2.72 ± 0.16 |
Hydrogen bond with LYS6935 |
2.04 ± 0.07 |
Hydrogen bond with GLY6871 |
2.5 ± 0.09 |
2.4.2. MM-PBSA binding free energy calculations. Another important indicator that gives account for the potential affinity of a ligand with its target is the binding free energy calculated using MM-PBSA and MD simulations. In general, complexes that have lower binding free energy can be considered to be more stable and their ligands are expected to have high activity and potency. In MD simulation, the binding free energies are calculated for every conformation saved in the trajectory. Accordingly, the binding free energy for both AP-20 and Sinefungin in complex with SARS COV-2 2′-O-methyltransferase enzyme was calculated using MmPbSaStat.py python script available in g_mmpbsa package. Running the script allows the package to calculate the total free energy for the complex, receptor and the ligand.Moreover, each component (complex, receptor and the ligand) of free energy could be calculated by the cumulative sum of its molecular mechanics potential energy in a vacuum and the free energy of solvation. The last one is the sum of electrostatic energy and non-electrostatic energy that is commonly calculated by the solvent accessible surface area (SASA) model. All those types of energies were calculated using g_mmpbsa package alongeside with the standard deviation before summed together to give the average total free energy of each component. At last, subtracting the total free energy of the receptor and the total free energy of the ligand from the total free energy of the complex yielded the binding free energy. Table 3 summarizes the interaction energies and the binding free energy for the two complexes.
Table 3 MM-PBSA calculations of the binding free energy for the two complexes; AP-20 and Sinefungin
Complex |
ΔEbinding (kJ mol−1) |
ΔEelectrostatic (kJ mol−1) |
ΔEvan der Waal (kJ mol−1) |
ΔEpolar solvation (kJ mol−1) |
SASA (kJ mol−1) |
AP-20 |
−301.23 ± 18.55 |
−109.13 ± 18.51 |
−284.76 ± 22.17 |
117.97 ± 13.99 |
−25.31 ± 1.01 |
Sinefungin |
−256.01 ± 17.54 |
−98.27 ± 18.64 |
−215.91 ± 20.07 |
80.12 ± 12.89 |
−21.95 ± 1.08 |
Generally, AP-20-SARS COV-2 2′-O-methyltransferase complex was superior in all the forms of the calculated energy over Sinefungin-SARS COV-2 2′-O-methyltransferase complex except (polar solvation energy). The average binding free energy reached −301.23 kJ mol−1, and −256.01 kJ mol−1 for AP-20 and Sinefungin complexes, respectively. Overall, the results of the MD simulations support the design of AP-20; also, they emphasize the potential ability of AP-20 to inhibit SARS COV-2 2′-O-methyltransferase.
3. Material and methods
3.1. Fragment based drug design (FBDD)
The steps of FBDD have been implemented in the same manner as in our previously published work.36,46 Firstly, the SARS COV-2 2′-O-methyltransferase structure co-crystalized with Sinefungin was retrieved from the protein data bank (PDB ID: 6wkq). Then, an expanded co-cavity surrounding the binding site of Sinefungin was constructed by Discovery Studio (DS) software.47 After that, the de novo receptor server and the default Ludi fragment database of the DS software were used to conduct the fragment-based drug design (FBDD) approach in order to find fragments that strongly bind to the 2′-O-methyltransferase binding site.48 Multiple Copy Simultaneous Search (MCSS) algorithm implemented in the same software was used to evaluate the strength of binding for each fragment from the previous step was further evaluated after docking into the 2′-O-methyltransferase binding site.38,49 Finally, after filtering the generated fragments, based on their rank and binding mode, the selected fragments were linked together using suitable carbon linkers to produce AP-20 that entered a docking simulation process to elucidate its initial binding mode in addition to its strength of binding.
3.2. Docking
To provide a rough validation of the docking protocol used, we performed a pose-retrieval docking experiments for the X-ray coordinates of Sinefungin in the binding site of nsp16 and then RMSD values between the docked and the co-crystalized poses were calculated. AutoDock Vina software was used to conduct the previous step in addition to the docking of the designed inhibitor. MGL tools 1.5.7 was implemented to prepare the equilibrated nsp16, Sinefungin, W1–5 and AP-20 by conversion into pdbqt format; a prerequisite for Vina Autodock software.39,50 The active site was determined by generating a grid box sized 24 × 24 × 24 Å surrounding the binding site of Sinefungin. The docking was performed using the default genetic algorithm with exhaustiveness value equals 64 (ESI†).39 All the docking results were visually inspected and analyzed using the interaction diagram generated by Discovery Studio Visualizer.51
3.3. In silico ADME predictions
The Swiss ADME server available online at (http://www.swissadme.ch/index.php) was used to model the AP-20 physicochemical properties.
3.4. Molecular dynamics (MD)
To validate the results obtained from the docking step, three molecular dynamic simulation experiments were conducted, one for ligand-free methyl transferase, while the other two were for the enzyme in complex with the AP-20 and the co-crystal reference Sinefungin. All the three molecular dynamic simulations were performed according to the standard published methodology of GROningen MAchine for chemical simulations GROMACS 2020.3 software,52 starting by generating and joining the enzyme and the ligand topologies into one complex.53 After that, the three systems were solvated using single point charge (SPC) water model to add water molecules to cubic simulation boxes. After adding suitable counter-ions to neutralize the entire system, steepest descent minimization algorithm with a maximum of 50
000 steps and <10.0 kJ mol−1 force was implemented for energy minimization under GROMOS96 43a1 force field.54 Two consecutive equilibrations steps were performed on the energy minimized structures, beginning with 2 ns of NVT ensemble simulation with constant number of particles, volume and temperature (310 K) then 8 ns of NPT ensemble with constant number of particles, pressure and temperature for 8 ns. The long range electrostatic was maintained by the use of Particle Mesh Ewald (PME) method with a 12 Å cut-off and 12 Å Fourier spacing.55 Finally, equilibrated systems were subjected without any restrains to a production stage of 150 ns. The time step was set to 2 fs, and the structural coordinates were saved every 10 ps into the trajectories. The generated trajectories retrieved from production step were then used to calculate the root means square deviation (RMSD) of the entire system residues in addition to the distances of the formed hydrogen bonds between the receptor and the ligand by various scripts of GROMACS.
3.4.1. MM-PBSA calculation. The standard equation for calculating binding free energy using MM-PBSA approach was applied, which states the following:
ΔG(Binding) = G(Complex) − G(Receptor) − G(Ligand) |
where, G(Complex), G(Receptor) and G(Ligand) are the total free energy of the protein–ligand complex, free enzyme and ligand in solvent, respectively. All trajectories retrieved from the MD simulations were processed by the g_mmpbsa package implemented in GROMACS software to calculate the total free energy for the three mentioned entities (complex, receptor and ligand) that compose both molecular mechanics potential energy as well as the energy of solvation.56 These calculations were done for the two complexes of methyl transferase – AP-20 and methyl transferase – Sinefungin.
4. Conclusion
2′-O-Methyltransferase is an attractive target for SARS CoV-2 which is responsible for the viral RNA protection via a cap formation process. In this study, we considered 2′-O-methyltransferase (nsp16) for a FBDD approach to propose new potential inhibitor. The potential inhibitor AP-20 was built via linking fragments from Ludi database (1053 diverse fragments Mw < 300 kDa). AP-20 docking pose showed superior score to the co-crystal ligand (Sinefungin) with −11.1 kcal per mole and −7.5 kcal per mole, respectively. AP-20 docking pose exhibited favorable interactions with the key residues of the binding site of 2′-O-methyltransferase. To support the established protocol, three molecular dynamic simulations of 2′-O-methyltransferase of the apo structure and its complexed formed with AP-20 and Sinefungin were performed for 150 nano-seconds. RMSD values for complexes with AP-20 and Sinefungin reached at their maximum dynamicity peaks 1.82 Å and 2.64 Å, respectively, while the maximum RMSD value for the apo structure reached 4.8 Å. These data suggest that AP-20 possesses stronger binding to SARS COV-2 2′-O-methyltransferase (nsp16) even more than Sinefungin. Furthermore, H-bonding interactions between AP-20 and the binding site residues always less than 3.5 Å throughout the MD simulation indicating a stable and a valid binding mode to 2′-O-methyltransferase. Finally, we recommend our designed molecule AP-20 to be further investigated via in vitro and in vivo experiments to tackle the spread of the emerging COVID-19. Also, the in silico ADME predictions have ascribed to AP-20 an excellent safety and metabolic stability profile. Our future plan includes further optimization of AP-20 to develop a promising candidate suitable for the clinic use.
Conflicts of interest
The authors declare no conflict of interest.
Funding
The authors would like to extend their sincere appreciation to the Deanship of Scientific Research at King Saud University for its funding of this research through the Research Group Project no. RG-1439-065.
References
- F. A. Rabi, M. S. Al Zoubi, G. A. Kasasbeh, D. M. Salameh and A. D. Al-Nasser, Pathogens, 2020, 9(3), 231 CrossRef CAS PubMed
. - X. Li, M. Geng, Y. Peng, L. Meng and S. Lu, J. Pharm. Anal., 2020, 10(2), 102–108 CrossRef PubMed
. - M. Romano, A. Ruggiero, F. Squeglia, G. Maga and R. Berisio, Cells, 2020, 9(5), 1267 CrossRef CAS PubMed
. - R. Alexpandi, J. F. De Mesquita, S. K. Pandian and A. V. Ravi, Front. Microbiol., 2020, 11, 1796 CrossRef PubMed
. - L. Zhang, D. Lin, X. Sun, U. Curth, C. Drosten, L. Sauerhering, S. Becker, K. Rox and R. Hilgenfeld, Science, 2020, 368(6489), 409–412 CrossRef CAS PubMed
. - W. R. Ferraz, R. A. Gomes, A. L. S. Novaes and G. H. G. Trossini, Future Med. Chem., 2020, 12, 1815–1828 CrossRef CAS PubMed
. - D. Gentile, V. Patamia, A. Scala, M. T. Sciortino, A. Piperno and A. Rescifina, Mar. Drugs, 2020, 18(4), 225 CrossRef CAS PubMed
. - O. O. Olubiyi, M. Olagunju, M. Keutmann, J. Loschwitz and B. Strodel, Molecules, 2020, 25(14), 3193 CrossRef CAS PubMed
. - J. Wang, J. Chem. Inf. Model., 2020, 60, 3277–3286 CrossRef CAS PubMed
. - R. Banerjee, L. Perera and L. M. Viranga Tillekeratne, Drug Discovery Today, 2021, 26(3), 804–816, DOI:10.1016/j.drudis.2020.12.005
. - W. Cui, K. Yang and H. Yang, Front. Mol. Biosci., 2020, 7, 616341 CrossRef CAS PubMed
. - C. C. Chen, X. Yu, C. J. Kuo, J. Min, S. Chen, S. Wu, L. Ma, K. Liu and R. T. Guo, FEBS J., 2021 DOI:10.1111/febs.15696
. - E. Singh, R. J. Khan, R. K. Jha, G. M. Amera, M. Jain, R. P. Singh, J. Muthukumaran and A. K. Singh, J. Genet. Eng. Biotechnol., 2020, 18, 69 CrossRef PubMed
. - Q. Li and C. Kang, Microorganisms, 2020, 8(8), 1250 CrossRef CAS PubMed
. - S. A. Amin, K. Ghosh, S. Gayen and T. Jha, J. Biomol. Struct. Dyn., 2020 Jun, 18, 1–10 Search PubMed
. - M. I. Ismail, H. M. Ragab, A. A. Bekhit and T. M. Ibrahim, Comput. Biol. Med., 2021 Apr 1, 131, 104295 Search PubMed
. - S. De Vita, M. G. Chini, G. Lauro and G. Bifulco, RSC Adv., 2020, 10, 40867–40875 RSC
. - P. Delre, F. Caporuscio, M. Saviano and G. F. Mangiatordi, Front. Chem., 2020, 8, 594009 CrossRef CAS PubMed
. - M. Kandeel, A. H. M. Abdelrahman, K. Oh-Hashi, A. Ibrahim, K. N. Venugopala, M. A. Morsy and M. A. A. Ibrahim, J. Biomol. Struct. Dyn., 2020, 1–8 CrossRef PubMed
. - C. N. Cavasotto and J. I. Di Filippo, Mol. Inf., 2021, 40(1), 2000115 CrossRef CAS PubMed
. - T. M. Ibrahim, M. I. Ismail, M. R. Bauer, A. A. Bekhit and F. M. Boeckler, Front. Chem., 2020, 8, 592289 CrossRef CAS PubMed
. - R. Alexpandi, J. F. De Mesquita, S. K. Pandian and A. V. Ravi, Front. Microbiol., 2020, 11, 1796 CrossRef PubMed
. - W. Ribeiro Ferraz, R. Augusto Gomes, A. Luis S Novaes and G. Henrique Goulart Trossini, Future Med. Chem., 2020, 12(20), 1815–1828, DOI:10.4155/fmc-2020-0165
. - A. K. Ghosh, M. Brindisi, D. Shahabi, M. E. Chapman and A. D. Mesecar, ChemMedChem, 2020, 15, 907, DOI:10.1002/cmdc.202000223
. - S. T. Ngo, N. Quynh Anh Pham, L. Thi Le, D. H. Pham and V. V. Vu, J. Chem. Inf. Model., 2020 Dec 28, 60(12), 5771–5780, DOI:10.1021/acs.jcim.0c00491
. - S. Shahinshavali, K. A. Hossain, A. V. D. N. Kumar, A. G. Reddy, D. Kolli, A. Nakhi, M. V. B. Rao and M. Pal, Tetrahedron Lett., 2020, 61(40), 152336 CrossRef PubMed
. - M. Hagar, H. A. Ahmed, G. Aljohani and O. A. Alhaddad, Int. J. Mol. Sci., 2020, 21(11), 3922 CrossRef CAS PubMed
. - D. Gentile, V. Patamia, A. Scala, M. T. Sciortino, A. Piperno and A. Rescifina, Mar. Drugs, 2020, 18(4), 225 CrossRef CAS PubMed
. - R. Chemboli, R. Kapavarapu, K. Deepti, K. R. S. Prasad, A. G. Reddy, A. N. Kumar, M. V. B. Rao and M. Pal, J. Mol. Struct., 2021, 1230, 129868 CrossRef CAS PubMed
. - Y. Chen and D. Guo, Molecular mechanisms of coronavirus RNA capping and methylation, Virol. Sin., 2016, 31, 3–11 CrossRef CAS PubMed
. - P. Krafcikova, J. Silhan, R. Nencka and E. Boura, Nat. Commun., 2020, 11, 3717 CrossRef CAS PubMed
. - A. Ramanathan, G. B. Robb and S. H. Chan, Nucleic Acids Res., 2016, 44, 7511–7526 CrossRef PubMed
. - S. Daffis, K. J. Szretter, J. Schriewer, J. Li, S. Youn, J. Errett, T. Y. Lin, S. Schneller, R. Zust, H. Dong, V. Thiel, G. C. Sen, V. Fensterl, W. B. Klimstra, T. C. Pierson, R. M. Buller, M. Gale, Jr., P. Y. Shi and M. S. Diamond, Nature, 2010, 468, 452–456 CrossRef CAS PubMed
. - P. Morales, N. Curtis, S. Zárate, A. Bastida and V. Bolanos-Garcia, Catalysts, 2020, 10(9), 1023, DOI:10.3390/catal10091023
. - A. Paramasivam, Cell Death Discovery, 2020, 6, 118, DOI:10.1038/s41420-020-00358-z
. - M. A. El Hassab, T. M. Ibrahim, S. T. Al-Rashood, A. Alharbi, R. O. Eskandrani and W. M. Eldehna, J. Enzyme Inhib. Med. Chem., 2021, 36(1), 727–736 CrossRef CAS PubMed
. - P. Kirsch, A. M. Hartman, A. K. Hirsch and M. Empting, Molecules, 2019 Jan, 24(23), 4309 Search PubMed
. - E. Evensen, D. Joseph-McCarthy,and M. Karplus. MCSS version 2.1. Harvard University, Cambridge, MA, USA. 1997 Search PubMed
. - O. Trott and A. J. Olson, J. Comput. Chem., 2010, 31, 455–461, DOI:10.1002/jcc.21334
. - M. A. Alamri, M. Tahir ul Qamar, M. U. Mirza, R. Bhadane, S. M. Alqahtani, I. Muneer, M. Froeyen and O. M. Salo-Ahen, J. Biomol. Struct. Dyn., 2020 Jun 23, 1–3 Search PubMed
. - M. A. El-Hasab, E. E. El-Bastawissy and T. El-Moselhy, J. Biomol. Struct. Dyn., 2018 May 19, 36(7), 1713–1727 Search PubMed
. - M. A. El-Hassab, E. E. El-Bastawissy and T. F. El-Moselhy, J. Biomol. Struct. Dyn., 2019, 6, 1–5 Search PubMed
. - H. Nagarajan, S. Narayanaswamy and U. Vetrivel, Mutat. Res., Fundam. Mol. Mech. Mutagen., 2020, 1, 111687 CrossRef PubMed
. - S. Chen, R. P. Wiewiora, F. Meng, N. Babault, A. Ma, W. Yu, K. Qian, H. Hu, H. Zou, J. Wang and S. Fan, eLife, 2019, 8, e45403 CrossRef PubMed
. - B. Xiao, C. Jing, G. Kelly, P. A. Walker, F. W. Muskett, T. A. Frenkiel, S. R. Martin, K. Sarma, D. Reinberg, S. J. Gamblin and J. R. Wilson, Genes Dev., 2005, 19(12), 1444–1454, DOI:10.1101/gad.1315905
. - M. A. El Hassab, A. A. Shoun, S. T. Al-Rashood, T. Al-Warhi and W. M. Eldehna, Front. Chem., 2020, 8, 584894, DOI:10.3389/fchem.2020.584894
. - Dassault Systèmes BIOVIA, BIOVIA Workbook, Release 2016; BIOVIA Pipeline Pilot, Release 2016: Dassault Systèmes, San Diego, 2016 Search PubMed
. - H. J. Böhm, J. Comput.-Aided Mol. Des., 1992 Feb, 6(1), 61–78, DOI:10.1007/BF00124387
. - A. Caflisch, A. Miranker and M. Karplus, J. Med. Chem., 1993, 23;36(15), 2142–2167, DOI:10.1021/jm00067a013
. - G. M. Morris, R. Huey, W. Lindstrom, M. F. Sanner, R. K. Belew, D. S. Goodsell and A. J. J. Olson, Comput.Chem., 2009, 16, 2785–2791 CrossRef PubMed
. - https://3dsbiovia.com/resource-center/downloads/.
- M. J. Abraham, T. Murtola, R. Schulz, S. Pall, J. C. Smith, B. Hess and E. Lindahl, SoftwareX, 2015, 1–2, 19–25, DOI:10.1016/j.softx.2015.06.001
. - J. C. Phillips, R. Braun, W. Wang, J. Gumbart, E. Tajkhorshid, E. Villa, C. Chipot, R. D. Skeel, L. Kale and K. Schulten, J. Comput. Chem, 2005 Dec, 26(16), 1781–1802 Search PubMed
. - S. W. Chiu, S. A. Pandit, H. L. Scott and E. Jakobsson, J. Phys. Chem. B, 2009, 113(9), 2748–2763 CrossRef CAS PubMed
. - V. K. Bhardwaj, R. Singh, J. Sharma, V. Rajendran, R. Purohit and S. Kumar, J. Biomol. Struct. Dyn., 2020, 8, p1–13 CrossRef PubMed
. - R. Kumari and R. C. Kumar, J. Chem. Inf. Model., 2014, 54, 1951–1962 CrossRef CAS PubMed
.
Footnote |
† Electronic supplementary information (ESI) available. See DOI: 10.1039/d1ra01809d |
|
This journal is © The Royal Society of Chemistry 2021 |
Click here to see how this site uses Cookies. View our privacy policy here.