DOI:
10.1039/D3AN00670K
(Paper)
Analyst, 2023,
148, 3594-3602
Phosphoproteome analysis of cerebrospinal fluid extracellular vesicles in primary central nervous system lymphoma†
Received
28th April 2023
, Accepted 20th June 2023
First published on 20th June 2023
Abstract
Primary central nervous system lymphoma (PCNSL) is a rare but highly aggressive extra-nodal non-Hodgkin's lymphoma, mostly of the diffuse large B-cell lymphoma (DLBCL) type. The present invasive diagnosis and poor prognosis of PCNSL propose an urgent need to develop molecular markers for early detection, real-time monitoring and treatment evaluation. Cerebrospinal fluid (CSF)-derived extracellular vesicles (EVs) are promising biomarker carriers for liquid biopsy of CNS diseases and brain tumors; however, research remains challenging due to the low concentration of EVs in the limited available volume of CSF from each individual patient and the low efficiency of existing methods for EV enrichment. Here, we introduce functionalized magnetic beads called EVTRAP (extracellular vesicles total recovery and purification) for rapid and efficient EV isolation from CSF. By coupling with high-performance mass spectrometry, over 19
000 peptides representing 1841 proteins were identified from just 30 μL of CSF. Furthermore, up to 3000 phosphopeptides representing over 1000 phosphoproteins were identified from about 2 mL of CSF. Finally, we analyzed the EV phosphoproteomics of CSF samples from PCNSL patients and non-PCNSL controls. Among them, multiple phosphoproteins related to PCNSL, including SPP1, MARCKS, NPM1 and VIM, were shown to be up-regulated in the PCNSL group. These results demonstrated the feasibility of the EVTRAP-based analytical strategy in CSF EV phosphoproteomic analysis of PCNSL molecular markers.
Introduction
Primary central nervous system lymphoma (PCNSL), a rare and aggressive extranodal non-Hodgkin's lymphoma form, is confined to the central nervous system without systemic symptoms and is primarily of the diffuse large B-cell lymphoma (DLBCL) type.1 It is estimated that PCNSL accounts for 4–6% of all extranodal lymphomas, 3% of all CNS tumors and 1% of all lymphomas.2 The diagnosis of PCNSL is usually performed by stereotactic biopsy, which is similar to tumor tissue biopsy and exhibits more influence on lesions compared to plasma/cerebrospinal fluid (CSF) biopsy.3 Moreover, the prognosis of PCNSL is poor due to delayed CNS toxicity.1 Thus, the development of non-invasive and effective detection methods based on liquid biopsy is important for PCNSL treatment.
CSF circulates around the central nervous system and can directly reflect the biochemical changes in the brain, spinal cord, and meninges. Multiple research studies have recently highlighted the importance and influence of CSF in the field of biomarker exploration for early diagnosis, real-time monitoring and prognostic evaluation of CNS diseases and brain tumors.4–8 However, traditional biomarkers such as free proteins and nucleic acids in CSF can be greatly interfered by other abundant contaminant molecules and may undergo some degradation mechanisms in biofluids, which makes biomarker discovery and application difficult.
To address these problems, research priorities have been focused on a new field: profiling of extracellular vesicles (EVs). EVs are lipid bilayer vesicles secreted by most cell types, including tumor cells, and exist in many types of bio-fluids (such as plasma, saliva, urine, and CSF) with a variety of cargos (e.g., proteins, nucleic acids and lipids). Increasing evidence indicates that EVs involve in various cellular processes including tumor progression; therefore, EVs are recognized as potential biomarker carriers for cancer liquid biopsy.9–11
However, existing methods for EV isolation vary from principles to effects and there is still no standardized method. Ultracentrifugation (UC) is currently regarded as the gold-standard technique for EV isolation, but it has a low throughput and is time-consuming; thus, UC cannot be widely used for clinical practice. Several other techniques for EV isolation have been reported and commercialized, including size-exclusion chromatography (SEC), polymer precipitation using commercial EV isolation kits, immunoaffinity-based isolation strategies and microfluidic strategies.12,13 Nevertheless, they have different disadvantages, including but not limited to low productivity, high protein contamination, high expense and irreproducibility. Therefore, establishment of a stable, specific and efficient EV enrichment method has become the key to EV research.
Here, we employed recently introduced functionalized magnetic beads named EVTRAP (extracellular vesicles total recovery and purification) for the isolation of EVs from CSF. EVTRAP has been developed for the proteome and phosphoproteome analysis of urine and plasma EVs,14,15 but its application has not been evaluated for CSF samples which typically have a low volume. Therefore, we attempted to utilize EVTRAP for the EV isolation of CSF. In detail, we first compared the two EV isolation methods, EVTRAP vs. ultra-centrifugation (UC), in aspects of EV concentration, abundance of EV markers, CSF free proteins and protein coverage through western blotting and LC-MS analysis. Then, we applied the EVTRAP approach to analyze the differences in CSF EV phosphoproteomes between patients with PCNSL and non-PCNSL individuals. Protein phosphorylation is one of the most extensive post-translational modifications (PTMs) and is an important regulatory mechanism in cancer and tumor progression,16 but its research and applications are restricted by the low abundance of phosphoproteins and dephosphorylation in biofluids. It is remarkable that EV-based analysis can reduce the interference of free molecules in biofluids to improve the detection of low-abundance proteins and prevent dephosphorylation from the enzyme in biofluids by protecting the EV bilayer membrane.17 Through EVTRAP-based CSF EV phosphoproteome analysis, we obtained an unprecedented dataset of the CSF EV phosphoproteome. Subsequently, using the label-free quantitation method, several phosphoproteins, which have been previously reported to be associated with PCNSL or DLBCL, were identified to be up-regulated in the PCNSL group. These results provide a reference for further discovery and validation of potential biomarkers in PCNSL and also demonstrate the feasibility of EVTRAP for CSF EV proteomic and phosphoproteomic analyses.
Experimental
Detailed experimental materials and methods of UC, western blot, transmission electron microscopy (TEM) imaging, nanoparticle tracking analysis (NTA), LC-MS sample preparation, LC-MS/MS analysis, database search and label-free quantitation analysis are included in the ESI.†
Collection and storage of CSF samples
All CSF samples were collected with ethical approval from Huashan Hospital affiliated to Fudan University (the Huashan Hospital Institutional Review Board (HIRB), approval no. 2018-767), Shanghai, China, and all patients gave their consent before the samples were collected. All frozen samples were thawed and centrifuged at 2500g for 10 min to remove cell debris, apoptotic bodies and other large particles. The supernatant was collected and stored at −80 °C until further use.
Extracellular vesicle isolation by EVTRAP
EVTRAP beads were provided by Tymora Analytical and were used as previously described.14 The CSF samples were diluted four-fold with the diluent buffer and 6 μL of EVTRAP beads were added to 100 μL of CSF. The samples with EVTRAP beads were then incubated by end-over-end rotation for 1 h. After removing the supernatant using a magnet, the beads were washed once with 0.01% Triton X-100/NP-40 PBS solution and three times with PBS. Finally, the EVs were eluted by vortexing for 10 min with 100 mM fresh triethylamine (TEA), and the eluate was collected and freeze-dried.
LC-MS/MS sample preparation
For the proteomics experiment of EVs captured by EVTRAP and UC, a starting sample volume of 100 μL of CSF was used to isolate EVs. After lysis, digestion and desalting, EV proteins from about 30 μL were injected for LC-MS/MS analysis. In the case of phosphoproteome analysis of clinical CSF samples from non-PCNSL controls and patients with PCNSL, the starting sample volume was 3 mL. Phosphopeptides were enriched using PolyMAC by following the provided instructions from Tymora Analytical.
Results and discussion
Assessment of the EVTRAP-based EV isolation strategy
EVs derived from CSF are potential biomarker carriers for nervous system diseases and brain tumors.18,19 However, research studies are always confined by both the limited volume of CSF and the low concentration of EVs in CSF. Here we applied the EVTRAP method for the enrichment of EVs from CSF. Functionalized with hydrophilic and lipophilic groups on the magnetic beads, EVTRAP is highly efficient and specific for EV isolation and has already been utilized for phosphoproteome profiling of urine and plasma EVs.14,15
For the application of EVTRAP in CSF EV isolation, first, EV isolation and characterization were performed using TEM imaging and NTA. After eluting off the beads, EVs were freeze-dried and then redissolved for TEM imaging and NTA, with 100 μL and 1 mL of PBS, respectively. Intact EVs were visualized in the TEM image (Fig. 1A). The size distribution was detected by NTA, revealing a peak diameter of 160 nm and a calculated concentration of 2.2 × 109 particles per milliliter of CSF (Fig. 1B). We further evaluated the EV isolation efficiency of the EVTRAP method using western blotting against three EV markers, TSG101, CD81 and CD9. As shown in Fig. 1C, clear bands of TSG101, CD81 and CD9 could be observed in the EVTRAP lane, while no visible signal was observed in the UC lane, demonstrating a much higher isolation efficiency of EVTRAP than the UC method. Given that western blotting can only provide specific analysis of target EV markers, we then carried out LC-MS analysis of EV proteome samples processed using the EVTRAP and UC methods to further compare their efficiencies. A total of 22
035 and an average of 17
995 peptides were identified among the three repeated mass spectrometric measurements of EVs isolated from CSF by EVTRAP, whereas a total of 13
285 peptides and an average of 11
613 peptides were identified in the three repeated MS analyses of UC processed samples (Fig. 1D and ESI Table S1†).
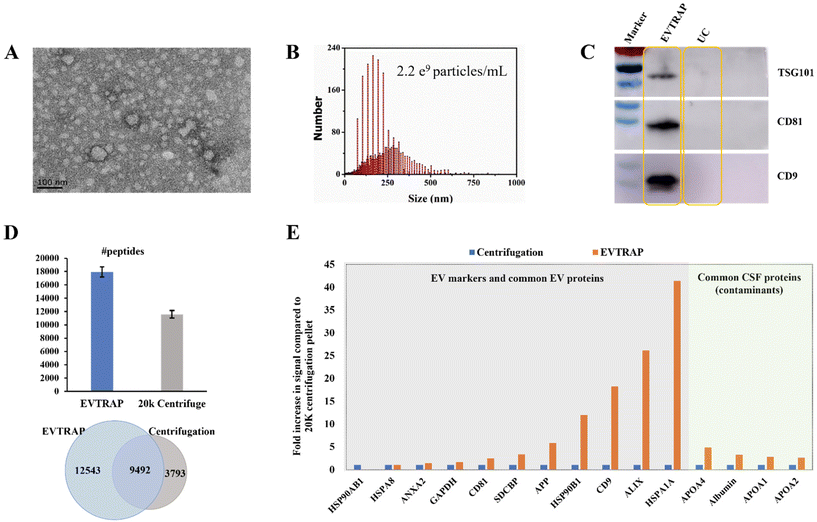 |
| Fig. 1 Assessment of the EVTRAP-based EV capture method. (A) Transmission electron microscopy (TEM) image of EVs captured by EVTRAP from CSF. (B) Nanoparticle tracking analysis of CSF EVs after elution of EVTRAP beads. (C) Detection of the TSG101, CD81, and CD9 EV markers using western blot from EVs enriched by EVTRAP and UC. (D) Number (up) and overlap (bottom) of identified peptides based on LC-MS analysis from EVs isolated by EVTRAP and UC. (E) Fold increase in the total proteome intensity of 11 EV proteins and 4 free CSF proteins from LC-MS data compared to the UC sample. | |
In addition, among all identified peptides, there were 9492 overlapped peptides found in both EVTRAP and UC processed samples, but 12
543 unique peptides were only found in CSF EVs isolated using the EVTRAP method. This indicated a similar but much broader proteome coverage of the EVTRAP method compared to UC. We further evaluated the specificity of the EVTRAP method by comparing the relative intensity of 11 typical EV proteins20 and 4 common CSF proteins21 in EV samples obtained using the EVTRAP and UC methods. As shown in Fig. 1E, the intensity of EV markers and related proteins in EVTRAP processed samples was much higher than that of UC while the intensity increase of CSF free proteins was less remarkable, illustrating good specificity of the EVTRAP method. We also conducted a gene ontology analysis of the whole proteome from CSF EVs isolated by EVTRAP, using DAVID Bioinformatics Resources.22,23 The identified proteins were significantly enriched in Extracellular Exosome, Extracellular Space and Extracellular Region annotated by the DAVID cellular component. Meanwhile, brain-specific proteins accounted for approximately 50% of the total proteins (ESI Fig. S1†).
LC-MS analysis of the EV phosphoproteome of clinical CSF samples
PCNSL is a highly aggressive and rare non-Hodgkin's lymphoma with a poor prognosis but there is still an increased incidence among the aged population.24 While protein phosphorylation is a vital regulatory mechanism for signaling pathways in cancers and diseases, systematic investigation of phosphorylation in PCNSL has been lacking, let alone phosphorylation analyses in CSF EVs. After demonstrating the high efficiency isolation of EVs from CSF samples, we attempted to apply the EVTRAP approach to the analysis of clinical CSF samples. The experimental workflow of proteome and phosphoproteome analyses of CSF EVs from patients diagnosed with PCNSL and non-PCNSL controls (which means patients diagnosed with other diseases or inflammatory reactions of the nervous system) is outlined in Fig. 2. The samples were grouped into PCNSL and control groups according to the clinical diagnosis and both groups included 9 individual samples with a volume of 1 milliliter each. CSF from each of the three individuals (grouped by matching the age and gender composition among groups. Detailed information is provided in ESI Table S2†) was pooled into a biological replicate (n = 3 biological replicates/group × 2 groups = 6). EV proteins were extracted in PTS buffer25 and digested with trypsin, followed by detergent removal and desalting. Afterward, 1% of the peptides was stored for direct proteome analysis and the remaining peptides underwent phosphopeptide enrichment by PolyMAC.26 Both peptides and phosphopeptides were analyzed using a Bruker trapped ion mobility time of flight spectrometer (timsTOF Pro) with a nanoflow LC-MS/MS.
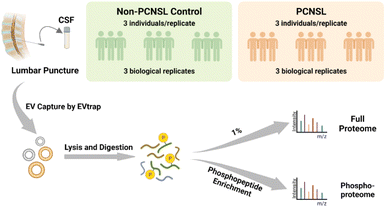 |
| Fig. 2 Workflow of EV phosphoproteomic analysis of CSF samples from non-PCNSL controls and patients diagnosed with primary central nervous system lymphoma. | |
Through the EVTRAP-based approach, over 1500 proteins and 17
000 peptides were identified from EVs of 30 μL of CSF (ESI Fig. S3 and ESI Table S3†), which once again demonstrated the high efficiency of EVTRAP for EV isolation from CSF. The overlap of identified proteins among intra-group biological replicates in the control and PCNSL groups was 71% and 75%, respectively. More importantly, we identified over 800 phosphoproteins and more than 2600 phosphopeptides in a single MS run of each biological replicate. Besides, even up to 3991 unique phosphopeptides corresponding to 1082 phosphoproteins were identified from a replicate of the PCNSL group (Fig. 3A and B and ESI Table S4†). The overlap of identified phosphoproteins among intra-group biological replicates in the control and PCNSL groups was 58% and 65%, respectively (ESI Fig. S2†). In comparison, 517 proteins were only identified in phosphoproteome analysis which indicates the lack of detection of low abundance phosphoproteins through regular shotgun proteomic analysis. Additionally, phosphopeptides with single phosphosite accounted for over 75% of the identified phosphopeptides and a total of 8345 Ser phosphosites were identified (Fig. 3C).
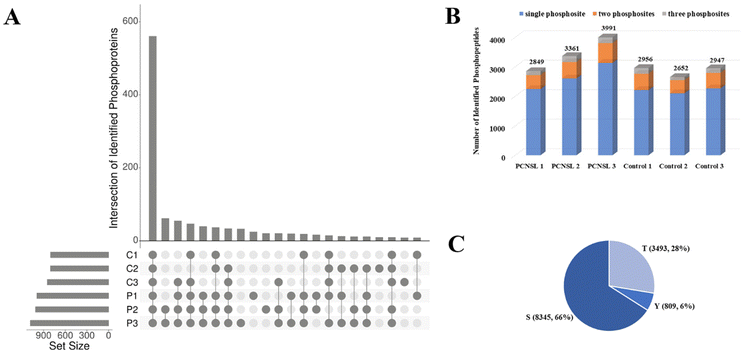 |
| Fig. 3 Number of identified phosphoproteins, phosphopeptides and phosphosites in CSF EV from the PCNSL and control groups. (A) Intersection of identified phosphoproteins. (B) Number of identified phosphopeptides and distribution of peptides with single, two or three phosphosites. (C) Distribution of the Ser/Thr/Tyr phosphosite. | |
Label-free quantitation analysis of the CSF EV phosphoproteome in PCNSL and non-PCNSL control groups
Label-free quantitation analysis of phosphopeptides between the PCNSL and control groups was then performed to identify potential phosphoproteins related to PCNSL. Among all 9225 identified phosphopeptides, we quantified 6179 phosphopeptides and identified 115 significantly changed (p-value < 0.05, |log2fold change| > 2) phosphopeptides representing 84 phosphoproteins in the PCNSL group using the KW test (Fig. 4A and B and ESI Table S5†). Top10 Gene Ontology terms for ‘Molecular Function’, ‘Biological Process’ and ‘Cellular Component’ of changed phosphoproteins are shown using a bubble chart (Fig. 4C). The heatmap of significantly changed phosphoproteins displayed the distribution of their normalized intensity among biological replicates (Fig. 4D). Proteins related to molecular binding and cell adhesion were enriched, and the Extracellular Exosome term was the most abundant annotation. In addition, twenty-eight of all 59 up-regulated phosphoproteins were related to the central nervous system annotated by STRING tissue expression, as shown in the Protein–Protein Interaction Network (Fig. 4E). We then examined these significantly changed phosphoproteins against previous studies. As there have been limited PCNSL investigations on signaling pathways in the literature, we also retrieved the data on DLBCL. Several phosphoproteins, including osteopontin (OSTP, also known as secreted phosphoprotein 1), myristoylated alanine-rich C-kinase substrate (MARCS), human leukocyte antigen A (HLAA), nucleophosmin (NPM), secretogranin-3 (SCG3),27 vimentin (VIME),28 insulin-like growth factor-binding protein 3 (IBP3),29 and brain acid soluble protein 1 (BASP1),30 have been previously reported to be associated with PCNSL or DLBCL. More specifically, OSTP was the most widely reported biomarker of PCNSL,31 which involves the up-regulation of interferon-gamma and interleukin-12 and down-regulation of interleukin-10 and is also essential in the pathway of type I immunity. MARCS, a filamentous (F) actin cross-linking protein, binds calmodulin, actin and synapsin and is closely related to the immunochemotherapy resistance and prognosis of DLBCL.32,33 It is interesting to note that the loss of HLA is a mechanism for immune escape and also the symbol of poor prognosis and high risk of relapse in DLBCL,34 whereas in our results, the relative intensity of HLA-A in CSF EV samples from PCNSL patients is higher than that in the control, indicating stable, even enhanced, T-cell immunity in PCNSL. NPM is also reported as a prognostic predictor of primary DLBCL in the CNS.35 Moreover, the PI3K-Akt signaling pathway, which is known to be correlated with the progress of many cancers including PCNSL,36 was enriched through the analysis of the Kyoto Encyclopedia of Genes and Genomes (KEGG) pathway (Fig. 4F).
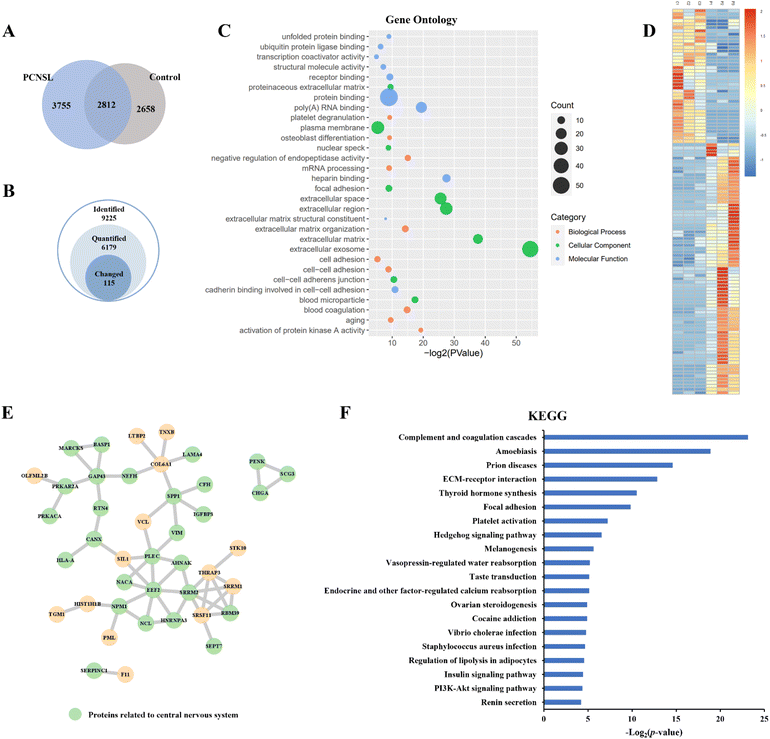 |
| Fig. 4 Label-free quantitation analysis of the phosphoproteome. (A) Venn diagram of the identified phosphopeptides in the PCNSL and control groups. (B) Number of identified, quantified and significantly changed phosphopeptides in label-free quantification. (C) Bubble chart of changed phosphoproteins, showing Top10 Gene Ontology terms for ‘Molecular Function’, ‘Biological Process’ and ‘Cellular Component’ with −log2 (p-value). (D) Heatmap of significantly changed phosphopeptides. (E) Protein–Protein Interaction Network of up-regulated phosphoproteins, green nodes showing proteins related to the central nervous system annotated by STRING tissue expression. (F) Bar graph of the Kyoto Encyclopedia of Genes and Genomes (KEGG) pathway of significantly changed phosphoproteins. | |
We also conducted label-free quantification for proteome data of PCNSL patients and non-PCNSL controls at the protein level (ESI Table S5†). Among 84 phosphoproteins corresponding to 115 differentially expressed phosphopeptides, 61 (73%) were also detected in proteome analysis but not showing any significant changes at the protein level, indicating that a large percentage of these phosphosite changes were due to post-translational regulation in PCNSL patients. These results demonstrated the potential of the EVTRAP method in the phosphoproteome profiling of EVs from CSF and its potential clinical applications in PCNSL.
Conclusions
In-depth phosphoproteome profiling of CSF-derived EVs remains elusive due to limited material and inefficient EV isolation for downstream analyses. Previously, only a small number of phosphopeptides and phosphoproteins were identified from either CSF or CSF EV samples. Here we applied a chemical-affinity-based paramagnetic isolation called EVTRAP for the enrichment of EVs from CSF, which allowed us to achieve in-depth analyses of downstream phosphoproteomics. Through western blot analysis of EV markers and further evidence from LC-MS analysis, we demonstrated the high capture efficiency and specificity of the EVTRAP method compared to the ultracentrifugation approach. In the phosphoproteome analysis of clinical samples, a total of 1232 phosphoproteins and 6567 phosphopeptides were identified from CSF EVs in patients with PCNSL, which is, to our knowledge, the largest dataset of CSF EV phosphoproteomics at present. In addition, 59 phosphoproteins, including some previously reported proteins such as SPP1, MARCKS and NPM1, were shown to be up-regulated in the PCNSL group through label-free quantitation analysis. Furthermore, the PI3K-Akt signalling pathway, involved in the progress of PCNSL and many other tumours, was enriched by the KEGG pathway analysis. Using the EVTRAP method, we demonstrated the ability to profile phosphoproteomes from CSF-derived EVs in PCNSL and we expect that the analytical strategy will be further evaluated with CSF samples to develop less invasive diagnostic methods for PCNS diseases such as PCNSL.
Data availability
The mass spectrometry proteomics data have been deposited to the ProteomeXchange Consortium via the JPOST37 partner repository with the data set identifier JPST002076 or PXD040744.
Author contributions
Yuanyuan Deng: investigation, methodology, validation, data curation, formal analysis, and writing – original draft. Qing Li: resources. Jie Sun: investigation and writing – review & editing. Leyao Ma: software and visualization. Yajie Ding: visualization. Yuhan Cai: investigation. Anton Iliuk: methodology. Bobin Chen: conceptualization. W. Andy Tao: conceptualization, writing – review & editing, supervision, and project administration. Zhuoying Xie: Funding acquisition.
Conflicts of interest
There are no conflicts of interest to declare.
Acknowledgements
This project has been funded in part by the National Key Research and Development Program of China (no. 2017YFA0700404) to Z. X.
References
- A. Korfel and U. Schlegel, Diagnosis and treatment of primary CNS lymphoma, Nat. Rev. Neurol., 2013, 9, 317–327 CrossRef CAS PubMed
.
- K. Hoang-Xuan, E. Bessell, J. Bromberg, A. F. Hottinger, M. Preusser, R. Ruda, U. Schlegel, T. Siegal, C. Soussain, U. Abacioglu, N. Cassoux, M. Deckert, C. M. Dirven, A. J. Ferreri, F. Graus, R. Henriksson, U. Herrlinger, M. Taphoorn, R. Soffietti and M. Weller, Diagnosis and treatment of primary CNS lymphoma in immunocompetent patients: guidelines from the European Association for Neuro-Oncology, Lancet Oncol., 2015, 16, e322–e332 CrossRef PubMed
.
- T. Calimeri, S. Steffanoni, M. Foppoli, M. Ponzoni and A. Ferreri, Implications of recent molecular achievements in early diagnosis and precision treatments for primary CNS lymphoma, Expert Opin. Ther. Targets, 2021, 25, 749–760 CrossRef CAS PubMed
.
- L. De Mattos-Arruda, R. Mayor, C. K. Y. Ng, B. Weigelt, F. Martínez-Ricarte, D. Torrejon, M. Oliveira, A. Arias, C. Raventos, J. Tang, E. Guerini-Rocco, E. Martínez-Sáez, S. Lois, O. Marín, X. de la Cruz, S. Piscuoglio, R. Towers, A. Vivancos, V. Peg, S. Ramon Y Cajal, J. Carles, J. Rodon, M. González-Cao, J. Tabernero, E. Felip, J. Sahuquillo, M. F. Berger, J. Cortes, J. S. Reis-Filho and J. Seoane, Cerebrospinal fluid-derived circulating tumour DNA better represents the genomic alterations of brain tumours than plasma, Nat. Commun., 2015, 6, 8839 CrossRef CAS PubMed
.
- Y. Wang, S. Springer, M. Zhang, K. W. McMahon, I. Kinde, L. Dobbyn, J. Ptak, H. Brem, K. Chaichana, G. L. Gallia, Z. L. Gokaslan, M. L. Groves, G. I. Jallo, M. Lim, A. Olivi, A. Quinones-Hinojosa, D. Rigamonti, G. J. Riggins, D. M. Sciubba, J. D. Weingart, J. Wolinsky, X. Ye, S. M. Oba-Shinjo, S. K. N. Marie, M. Holdhoff, N. Agrawal, L. A. Diaz, N. Papadopoulos, K. W. Kinzler, B. Vogelstein and C. Bettegowda, Detection of tumor-derived DNA in cerebrospinal fluid of patients with primary tumors of the brain and spinal cord, Proc. Natl. Acad. Sci. U. S. A., 2015, 112, 9704–9709 CrossRef CAS PubMed
.
- E. I. Pentsova, R. H. Shah, J. Tang, A. Boire, D. You, S. Briggs, A. Omuro, X. Lin, M. Fleisher, C. Grommes, K. S. Panageas, F. Meng, S. D. Selcuklu, S. Ogilvie, N. Distefano, L. Shagabayeva, M. Rosenblum, L. M. DeAngelis, A. Viale, I. K. Mellinghoff and M. F. Berger, Evaluating Cancer of the Central Nervous System Through Next-Generation Sequencing of Cerebrospinal Fluid, J. Clin. Oncol., 2016, 34, 2404–2415 CrossRef CAS PubMed
.
- A. M. Miller, R. H. Shah, E. I. Pentsova, M. Pourmaleki, S. Briggs, N. Distefano, Y. Zheng, A. Skakodub, S. A. Mehta, C. Campos, W. Hsieh, S. D. Selcuklu, L. Ling, F. Meng, X. Jing, A. Samoila, T. A. Bale, D. W. Y. Tsui, C. Grommes, A. Viale, M. M. Souweidane, V. Tabar, C. W. Brennan, A. S. Reiner, M. Rosenblum, K. S. Panageas, L. M. DeAngelis, R. J. Young, M. F. Berger and I. K. Mellinghoff, Tracking tumour evolution in glioma through liquid biopsies of cerebrospinal fluid, Nature, 2019, 565, 654–658 CrossRef CAS PubMed
.
- O. Karayel, S. Virreira Winter, S. Padmanabhan, Y. I. Kuras, D. T. Vu, I. Tuncali, K. Merchant, A. Wills, C. R. Scherzer and M. Mann, Proteome profiling of cerebrospinal fluid reveals biomarker candidates for Parkinson's disease, Cell Rep. Med., 2022, 3, 100661 CrossRef CAS PubMed
.
- B. Zhou, K. Xu, X. Zheng, T. Chen, J. Wang, Y. Song, Y. Shao and S. Zheng, Application of exosomes as liquid biopsy in clinical diagnosis, Signal Transduction Targeted Ther., 2020, 5, 144 CrossRef CAS PubMed
.
- T. Hu, J. Wolfram and S. Srivastava, Extracellular Vesicles in Cancer Detection: Hopes and Hypes, Trends Cancer, 2021, 7, 122–133 CrossRef CAS PubMed
.
- A. Becker, B. K. Thakur, J. M. Weiss, H. S. Kim, H. Peinado and D. Lyden, Extracellular Vesicles in Cancer: Cell-to-Cell Mediators of Metastasis, Cancer Cell, 2016, 30, 836–848 CrossRef CAS PubMed
.
- Y. Tian, M. Gong, Y. Hu, H. Liu, W. Zhang, M. Zhang, X. Hu, D. Aubert, S. Zhu, L. Wu and X. Yan, Quality and efficiency assessment of six extracellular vesicle isolation methods by nano–flow cytometry, J. Extracell. Vesicles, 2020, 9, 1697028 CrossRef CAS PubMed
.
- S. Gandham, X. Su, J. Wood, A. L. Nocera, S. C. Alli, L. Milane, A. Zimmerman, M. Amiji and A. R. Ivanov, Technologies and Standardization in Research on Extracellular Vesicles, Trends Biotechnol., 2020, 38, 1066–1098 CrossRef CAS PubMed
.
- X. Wu, L. Li, A. Iliuk and W. A. Tao, Highly Efficient Phosphoproteome Capture and Analysis from Urinary Extracellular Vesicles, J. Proteome Res., 2018, 17, 3308–3316 CrossRef CAS PubMed
.
- A. Iliuk, X. Wu, L. Li, J. Sun, M. Hadisurya, R. S. Boris and W. A. Tao, Plasma-Derived Extracellular Vesicle Phosphoproteomics through Chemical Affinity Purification, J. Proteome Res., 2020, 19, 2563–2574 CrossRef CAS PubMed
.
- S. J. Humphrey, D. E. James and M. Mann, Protein Phosphorylation: A Major Switch Mechanism for Metabolic Regulation, Trends Endocrinol. Metab., 2015, 26, 676–687 CrossRef CAS PubMed
.
- I. Chen, L. Xue, C. Hsu, J. S. P. Paez, L. Pan, H. Andaluz, M. K. Wendt, A. B. Iliuk, J. Zhu and W. A. Tao, Phosphoproteins in extracellular vesicles as candidate markers for breast cancer, Proc. Natl. Acad. Sci. U. S. A., 2017, 114, 3175–3180 CrossRef CAS PubMed
.
- S. Muraoka, M. P. Jedrychowski, K. Yanamandra, S. Ikezu, S. P. Gygi and T. Ikezu, Proteomic Profiling of Extracellular Vesicles Derived from Cerebrospinal Fluid of Alzheimer's Disease Patients: A Pilot Study, Cells, 2020, 9, 1959 CrossRef CAS PubMed
.
- S. Herman, R. Djaldetti, B. Mollenhauer and D. Offen, CSF-derived extracellular vesicles from patients with Parkinson’s disease induce symptoms and pathology, Brain, 2023, 146, 209–224 CrossRef PubMed
.
- C. Thery, K. W. Witwer, E. Aikawa, M. J. Alcaraz, J. D. Anderson, R. Andriantsitohaina, A. Antoniou, T. Arab, F. Archer, G. K. Atkin-Smith, D. C. Ayre, J. M. Bach, D. Bachurski, H. Baharvand, L. Balaj, S. Baldacchino, N. N. Bauer, A. A. Baxter, M. Bebawy, C. Beckham, Z. A. Bedina, A. Benmoussa, A. C. Berardi, P. Bergese, E. Bielska, C. Blenkiron, S. Bobis-Wozowicz, E. Boilard, W. Boireau, A. Bongiovanni, F. E. Borras, S. Bosch, C. M. Boulanger, X. Breakefield, A. M. Breglio, M. A. Brennan, D. R. Brigstock, A. Brisson, M. L. Broekman, J. F. Bromberg, P. Bryl-Gorecka, S. Buch, A. H. Buck, D. Burger, S. Busatto, D. Buschmann, B. Bussolati, E. I. Buzas, J. B. Byrd, G. Camussi, D. R. Carter, S. Caruso, L. W. Chamley, Y. T. Chang, C. Chen, S. Chen, L. Cheng, A. R. Chin, A. Clayton, S. P. Clerici, A. Cocks, E. Cocucci, R. J. Coffey, A. Cordeiro-da-Silva, Y. Couch, F. A. Coumans, B. Coyle, R. Crescitelli, M. F. Criado, C. D'Souza-Schorey, S. Das, C. A. Datta, P. de Candia, E. F. De Santana, O. De Wever, P. H. Del, T. Demaret, S. Deville, A. Devitt, B. Dhondt, D. Di Vizio, L. C. Dieterich, V. Dolo, R. A. Dominguez, M. Dominici, M. R. Dourado, T. A. Driedonks, F. V. Duarte, H. M. Duncan, R. M. Eichenberger, K. Ekstrom, A. S. El, C. Elie-Caille, U. Erdbrugger, J. M. Falcon-Perez, F. Fatima, J. E. Fish, M. Flores-Bellver, A. Forsonits, A. Frelet-Barrand, F. Fricke, G. Fuhrmann, S. Gabrielsson, A. Gamez-Valero, C. Gardiner, K. Gartner, R. Gaudin, Y. S. Gho, B. Giebel, C. Gilbert, M. Gimona, I. Giusti, D. C. Goberdhan, A. Gorgens, S. M. Gorski, D. W. Greening, J. C. Gross, A. Gualerzi, G. N. Gupta, D. Gustafson, A. Handberg, R. A. Haraszti, P. Harrison, H. Hegyesi, A. Hendrix, A. F. Hill, F. H. Hochberg, K. F. Hoffmann, B. Holder, H. Holthofer, B. Hosseinkhani, G. Hu, Y. Huang, V. Huber, S. Hunt, A. G. Ibrahim, T. Ikezu, J. M. Inal, M. Isin, A. Ivanova, H. K. Jackson, S. Jacobsen, S. M. Jay, M. Jayachandran, G. Jenster, L. Jiang, S. M. Johnson, J. C. Jones, A. Jong, T. Jovanovic-Talisman, S. Jung, R. Kalluri, S. I. Kano, S. Kaur, Y. Kawamura, E. T. Keller, D. Khamari, E. Khomyakova, A. Khvorova, P. Kierulf, K. P. Kim, T. Kislinger, M. Klingeborn, D. N. Klinke, M. Kornek, M. M. Kosanovic, A. F. Kovacs, E. M. Kramer-Albers, S. Krasemann, M. Krause, I. V. Kurochkin, G. D. Kusuma, S. Kuypers, S. Laitinen, S. M. Langevin, L. R. Languino, J. Lannigan, C. Lasser, L. C. Laurent, G. Lavieu, E. Lazaro-Ibanez, S. Le Lay, M. S. Lee, Y. Lee, D. S. Lemos, M. Lenassi, A. Leszczynska, I. T. Li, K. Liao, S. F. Libregts, E. Ligeti, R. Lim, S. K. Lim, A. Line, K. Linnemannstons, A. Llorente, C. A. Lombard, M. J. Lorenowicz, A. M. Lorincz, J. Lotvall, J. Lovett, M. C. Lowry, X. Loyer, Q. Lu, B. Lukomska, T. R. Lunavat, S. L. Maas, H. Malhi, A. Marcilla, J. Mariani, J. Mariscal, E. S. Martens-Uzunova, L. Martin-Jaular, M. C. Martinez, V. R. Martins, M. Mathieu, S. Mathivanan, M. Maugeri, L. K. McGinnis, M. J. McVey, D. J. Meckes, K. L. Meehan, I. Mertens, V. R. Minciacchi, A. Moller, J. M. Moller, A. Morales-Kastresana, J. Morhayim, F. Mullier, M. Muraca, L. Musante, V. Mussack, D. C. Muth, K. H. Myburgh, T. Najrana, M. Nawaz, I. Nazarenko, P. Nejsum, C. Neri, T. Neri, R. Nieuwland, L. Nimrichter, J. P. Nolan, H. E. Nolte-'T, H. N. Noren, L. O'Driscoll, T. O'Grady, A. O'Loghlen, T. Ochiya, M. Olivier, A. Ortiz, L. A. Ortiz, X. Osteikoetxea, O. Ostergaard, M. Ostrowski, J. Park, D. M. Pegtel, H. Peinado, F. Perut, M. W. Pfaffl, D. G. Phinney, B. C. Pieters, R. C. Pink, D. S. Pisetsky, V. S. E. Pogge, I. Polakovicova, I. K. Poon, B. H. Powell, I. Prada, L. Pulliam, P. Quesenberry, A. Radeghieri, R. L. Raffai, S. Raimondo, J. Rak, M. I. Ramirez, G. Raposo, M. S. Rayyan, N. Regev-Rudzki, F. L. Ricklefs, P. D. Robbins, D. D. Roberts, S. C. Rodrigues, E. Rohde, S. Rome, K. M. Rouschop, A. Rughetti, A. E. Russell, P. Saa, S. Sahoo, E. Salas-Huenuleo, C. Sanchez, J. A. Saugstad, M. J. Saul, R. M. Schiffelers, R. Schneider, T. H. Schoyen, A. Scott, E. Shahaj, S. Sharma, O. Shatnyeva, F. Shekari, G. V. Shelke, A. K. Shetty, K. Shiba, P. R. Siljander, A. M. Silva, A. Skowronek, O. N. Snyder, R. P. Soares, B. W. Sodar, C. Soekmadji, J. Sotillo, P. D. Stahl, W. Stoorvogel, S. L. Stott, E. F. Strasser, S. Swift, H. Tahara, M. Tewari, K. Timms, S. Tiwari, R. Tixeira, M. Tkach, W. S. Toh, R. Tomasini, A. C. Torrecilhas, J. P. Tosar, V. Toxavidis, L. Urbanelli, P. Vader, B. W. van Balkom, S. G. van der Grein, J. Van Deun, M. J. van Herwijnen, K. Van Keuren-Jensen, G. van Niel, M. E. van Royen, A. J. van Wijnen, M. H. Vasconcelos, I. J. Vechetti, T. D. Veit, L. J. Vella, E. Velot, F. J. Verweij, B. Vestad, J. L. Vinas, T. Visnovitz, K. V. Vukman, J. Wahlgren, D. C. Watson, M. H. Wauben, A. Weaver, J. P. Webber, V. Weber, A. M. Wehman, D. J. Weiss, J. A. Welsh, S. Wendt, A. M. Wheelock, Z. Wiener, L. Witte, J. Wolfram, A. Xagorari, P. Xander, J. Xu, X. Yan, M. Yanez-Mo, H. Yin, Y. Yuana, V. Zappulli, J. Zarubova, V. Zekas, J. Y. Zhang, Z. Zhao, L. Zheng, A. R. Zheutlin, A. M. Zickler, P. Zimmermann, A. M. Zivkovic, D. Zocco and E. K. Zuba-Surma, Minimal information for studies of extracellular vesicles 2018 (MISEV2018): a position statement of the International Society for Extracellular Vesicles and update of the MISEV2014 guidelines, J. Extracell. Vesicles, 2018, 7, 1535750 CrossRef PubMed
.
- S. Roche, A. Gabelle and S. Lehmann, Clinical proteomics of the cerebrospinal fluid: Towards the discovery of new biomarkers, Proteomics: Clin. Appl., 2008, 2, 428–436 CAS
.
- B. T. Sherman, M. Hao, J. Qiu, X. Jiao, M. W. Baseler, H. C. Lane, T. Imamichi and W. Chang, DAVID: a web server for functional enrichment analysis and functional annotation of gene lists (2021 update), Nucleic Acids Res., 2022, 50, W216–W221 CrossRef CAS PubMed
.
- D. W. Huang, B. T. Sherman and R. A. Lempicki, Systematic and integrative analysis of large gene lists using DAVID bioinformatics resources, Nat. Protoc., 2009, 4, 44–57 CrossRef CAS PubMed
.
- K. Hoang-Xuan, M. Deckert, A. J. M. Ferreri, J. Furtner, J. Gallego Perez-Larraya, R. Henriksson, A. F. Hottinger, B. Kasenda, F. Lefranc, A. Lossos, C. McBain, M. Preusser, P. Roth, R. Rudà, U. Schlegel, R. Soffietti, C. Soussain, M. J. B. Taphoorn, V. Touitou, M. Weller and J. E. C. Bromberg, European Association of Neuro-Oncology (EANO) guidelines for treatment of primary central nervous system lymphoma (PCNSL), Neuro-Oncology, 2023, 25, 37–53 CrossRef PubMed
.
- T. Masuda, M. Tomita and Y. Ishihama, Phase Transfer Surfactant-Aided Trypsin Digestion for Membrane Proteome Analysis, J. Proteome Res., 2008, 7, 731–740 CrossRef CAS PubMed
.
- A. B. Iliuk, V. A. Martin, B. M. Alicie, R. L. Geahlen and W. A. Tao, In-depth Analyses of Kinase-dependent Tyrosine Phosphoproteomes Based on Metal Ion-functionalized Soluble Nanopolymers, Mol. Cell. Proteomics, 2010, 9, 2162–2172 CrossRef CAS PubMed
.
- B. M. Downs, W. Ding, L. M. Cope, C. B. Umbricht, W. Li, H. He, X. Ke, M. Holdhoff, C. Bettegowda, W. Tao and S. Sukumar, Methylated markers accurately distinguish primary central nervous system lymphomas (PCNSL) from other CNS tumors, Clin. Epigenet., 2021, 13, 104 CrossRef CAS PubMed
.
- M. Montesinos-Rongen, F. G. Purschke, A. Brunn, C. May, E. Nordhoff, K. Marcus and M. Deckert, Primary Central Nervous System (CNS) Lymphoma B Cell Receptors Recognize CNS Proteins, J. Immunol., 2015, 195, 1312–1319 CrossRef CAS PubMed
.
- C. O. Sung, S. C. Kim, S. Karnan, K. Karube, H. J. Shin, D. Nam, Y. Suh, S. Kim, J. Y. Kim, S. J. Kim, W. S. Kim, M. Seto and Y. Ko, Genomic profiling combined with gene expression profiling in primary central nervous system lymphoma, Blood, 2011, 117, 1291–1300 CrossRef CAS PubMed
.
- F. Xiao, S. Lv, Z. Zong, L. Wu, X. Tang, W. Kuang, P. Zhang, X. Li, J. Fu, M. Xiao, M. Wu, L. Wu, X. Zhu, K. Huang and H. Guo, Cerebrospinal fluid biomarkers for brain tumor detection: clinical roles and current progress, Am. J. Transl. Res., 2020, 12, 1379–1396 CAS
.
- H. W. Tun, D. Personett, K. A. Baskerville, D. M. Menke, K. A. Jaeckle, P. Kreinest, B. Edenfield, A. C. Zubair, B. P. O'Neill, W. R. Lai, P. J. Park and M. McKinney, Pathway analysis of primary central nervous system lymphoma (vol 111, pg 3200, 2008), Blood, 2008, 112, 3530 CAS
.
- O. Kooshkaki, Z. Rezaei, M. Rahmati, P. Vahedi, A. Derakhshani, O. Brunetti, A. Baghbanzadeh, B. Mansoori, N. Silvestris and B. Baradaran, MiR-144: A New Possible Therapeutic Target and Diagnostic/Prognostic Tool in Cancers, Int. J. Mol. Sci., 2020, 21, 2578 CrossRef CAS PubMed
.
- I. Hernández-Verdin, K. Labreche, M. Benazra, K. Mokhtari, K. Hoang-Xuan and A. Alentorn, Tracking the Genetic Susceptibility Background of B-Cell Non-Hodgkin's Lymphomas from Genome-Wide Association Studies, Int. J. Mol. Sci., 2021, 22, 122 CrossRef PubMed
.
- M. Alcoceba, E. Sebastián, L. Marín, A. Balanzategui, M. E. Sarasquete, M. C. Chillón, C. Jiménez, N. Puig, R. Corral, E. Pardal, C. Grande, J. L. Bello, C. Albo, F. de la Cruz, C. Panizo, A. Martín, E. González-Barca, M. D. Caballero, J. F. San Miguel, R. García-Sanz and M. González, HLA specificities are related to development and prognosis of diffuse large B-cell lymphoma, Blood, 2013, 122, 1448–1454 CrossRef CAS PubMed
.
- M. Todorovic Balint, J. Jelicic, B. Mihaljevic, J. Kostic, B. Stanic, B. Balint, N. Pejanovic, B. Lucic, N. Tosic, I. Marjanovic, M. Stojiljkovic, T. Karan-Djurasevic, O. Perisic, G. Rakocevic, M. Popovic, S. Raicevic, J. Bila, D. Antic, B. Andjelic and S. Pavlovic, Gene Mutation Profiles in Primary Diffuse Large B Cell Lymphoma of Central Nervous System: Next Generation Sequencing Analyses, Int. J. Mol. Sci., 2016, 17, 683 CrossRef PubMed
.
- Y. Takashima, Y. Sasaki, A. Hayano, J. Homma, J. Fukai, Y. Iwadate, K. Kajiwara, S. Ishizawa, H. Hondoh, T. Tokino and R. Yamanaka, Target amplicon exome-sequencing identifies promising diagnosis and prognostic markers involved in RTK-RAS and PI3K-AKT signaling as central oncopathways in primary central nervous system lymphoma, Oncotarget, 2018, 9, 27471–27486 CrossRef PubMed
.
- S. Okuda, Y. Watanabe, Y. Moriya, S. Kawano, T. Yamamoto, M. Matsumoto, T. Takami, D. Kobayashi, N. Araki, A. C. Yoshizawa, T. Tabata, N. Sugiyama, S. Goto and Y. Ishihama, jPOSTrepo: an international standard data repository for proteomes, Nucleic Acids Res., 2017, 45, D1107–D1111 CrossRef CAS PubMed
.
|
This journal is © The Royal Society of Chemistry 2023 |
Click here to see how this site uses Cookies. View our privacy policy here.