DOI:
10.1039/D3CS00972F
(Tutorial Review)
Chem. Soc. Rev., 2024,
53, 2851-2862
Strategies for designing biocatalysts with new functions
Received
17th November 2023
First published on 14th February 2024
Abstract
The engineering of natural enzymes has led to the availability of a broad range of biocatalysts that can be used for the sustainable manufacturing of a variety of chemicals and pharmaceuticals. However, for many important chemical transformations there are no known enzymes that can serve as starting templates for biocatalyst development. These limitations have fuelled efforts to build entirely new catalytic sites into proteins in order to generate enzymes with functions beyond those found in Nature. This bottom-up approach to enzyme development can also reveal new fundamental insights into the molecular origins of efficient protein catalysis. In this tutorial review, we will survey the different strategies that have been explored for designing new protein catalysts. These methods will be illustrated through key selected examples, which demonstrate how highly proficient and selective biocatalysts can be developed through experimental protein engineering and/or computational design. Given the rapid pace of development in the field, we are optimistic that designer enzymes will begin to play an increasingly prominent role as industrial biocatalysts in the coming years.
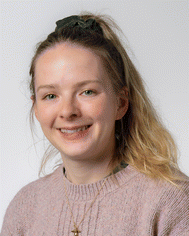
Elizabeth L. Bell
| Dr. Elizabeth L. Bell is a Postdoctoral researcher at the National Renewable Energy Laboratory (NREL) in Colorado, USA. After completing a BA in Natural Sciences and MPhil at St Catharine's College, University of Cambridge, UK, Elizabeth obtained her PhD in the group of Prof. Anthony P. Green, at the University of Manchester, UK, conducting research into the directed evolution of PET plastic degrading enzymes. Following on from a post-doc with A. P. Green engineering carbon fixing enzymes, Elizabeth's current research at NREL focusses on discovering and engineering enzymes for the deconstruction of nylons and polyurethanes. |
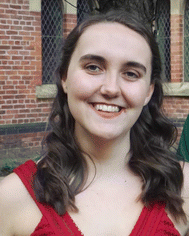
Amy E. Hutton
| Dr. Amy E. Hutton is a Postdoctoral researcher at the University of Manchester. After a MChem in Chemistry with Medicinal Chemistry at the University of Manchester, Amy obtained her PhD under the supervision of Prof. Anthony Green, conducting research into the directed evolution of enzymes with an expanded genetic code. Amy's current research with Prof. Anthony Green and Prof. Nicholas Turner focusses on the directed evolution of enzymes for the biocatalytic production of pharmaceuticals. |
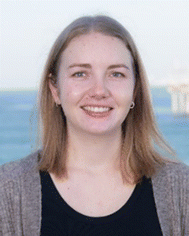
Ashleigh J. Burke
| Dr Ashleigh J. Burke is a Postdoctoral researcher at the Scripps Institution of Oceanography (SIO) in San Diego, CA, USA. Ashleigh obtained her PhD in the group of Prof. Anthony P. Green, at the University of Manchester conducting research into the design and evolution of enzymes with noncanonical organocatalytic mechanisms. Following on from a post-doc with Prof. A. P. Green and Prof. Nicholas Turner engineering enzymes for biocatalytic production of pharmaceuticals, Ashleigh's current research at SIO focusses on discovering and engineering enzymes for the production of marine natural products. |
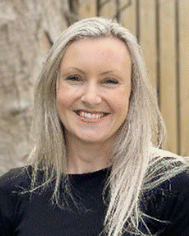
Elaine O’Reilly
| Elaine O’Reilly obtained her PhD in Chemistry from University College Dublin under the supervision of Prof. Francesca Paradisi. From 2010–2014 she carried out postdoctoral re-search at the University of Manchester before joining Manchester Metropolitan University as a Lecturer. Elaine was an Assistant Professor, then Associate Professor in Chemical Biology at the University of Nottingham between 2015 and 2018, before returning to University College Dublin as Associate Professor of Chemical Biology in 2019. Her research focuses on the development and application of biocatalysts in organic chemistry. |
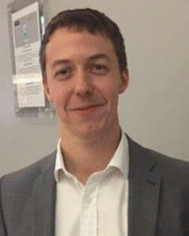
Anthony P. Green
| Following his PhD in synthetic organic chemistry under the supervision of Professor E. J. Thomas, Dr Anthony Green began postdoctoral research with Professor Nicholas Turner and Professor Sabine Flitsch based in the Manchester Institute of Biotechnology (MIB), working in the field of industrial biocatalysis. Professor Green worked as a postdoctoral research associate in the group of Professor Donald Hilvert at ETH (Zurich), before starting his independent research career in 2016 based in the MIB (Department of Chemistry) at the University of Manchester where he is a BBSRC David Phillips research fellow, a Professor in organic & biological chemistry and holder of an ERC starter grant. |
Key learning points
(1) New enzymes can be designed computationally based on fundamental principles of transition state stabilization.
(2) The activities of designed enzymes can be optimized by directed evolution.
(3) The range of chemistries accessible in designed enzymes can be greatly expanded by introducing new functional elements into proteins.
(4) Emerging deep learning algorithms could greatly increase the speed and accuracy of enzyme design.
|
1. Introduction
Enzymes are powerful biological catalysts, that use well-defined active sites to achieve complex chemical conversions with remarkable efficiencies and selectivities. As a result of their catalytic prowess, enzymes are now routinely deployed as biocatalysts for diverse applications across the chemical industry.1,2 For example, enzymes are commonly used to manufacture high-value molecules such as pharmaceuticals or for deconstructing anthropogenic contaminants including plastics.3–6 An important feature of enzymes that has contributed to their broad utility is their high degree of engineerability. Thanks to advanced enzyme engineering methodologies, we are no longer restricted to natural enzymes when developing biocatalytic processes. Instead, enzyme properties can now be adapted to meet the specific requirements of a target application.7 For example, enzymes can be engineered to broaden substrate range, alter selectivity, improve kinetic parameters, or enhance stability under process conditions. This engineering is commonly achieved through directed evolution, which has proven to be an exceptionally powerful and versatile strategy for tailoring enzyme properties.8,9 In some cases, directed evolution is used in conjunction with computational tools, to navigate sequence space more efficiently, and to reduce the screening burden during the enzyme engineering process.10,11
Although a wide variety of enzymes are now available for synthetic applications, for many important chemical transformations there are no natural enzymes known that can serve as suitable starting templates for evolutionary optimization. As a result, there is a long-standing interest in developing enzymes that operate through new reactivity modes which could enable the expansion of the biocatalytic repertoire to chemical processes not currently known in Nature. One approach to achieve this goal is to uncover any mechanistic promiscuity of natural enzymes, that can subsequently be engineered to optimize efficiency (‘top-down approach’).12–15 An attractive and potentially more versatile strategy is to build entirely new catalytic sites into proteins (Fig. 1). As well as providing a gateway to new biocatalytic functions, this ‘bottom-up’ approach to enzyme development provides a critical test of our understanding of the molecular determinants of efficient protein catalysis. In this tutorial review, we will summarize different strategies that have been used to design new enzymes and illustrate these approaches through selected key examples.
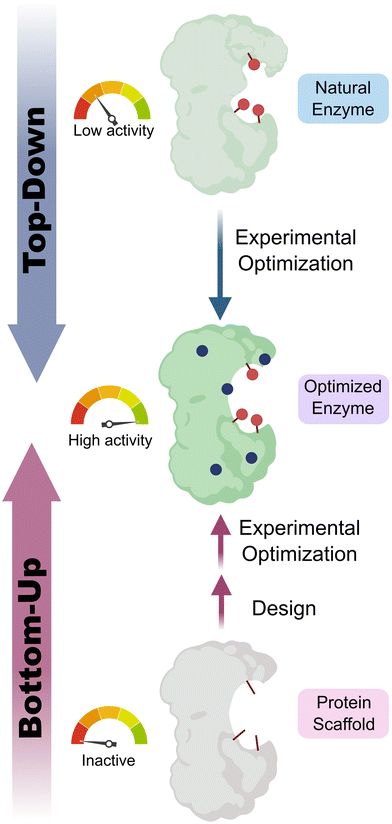 |
| Fig. 1 Top-down vs. bottom-up engineering of enzymes. Top-down: natural enzymes with desired catalytic activities are identified and their properties optimized experimentally, for example, using directed evolution.7 Bottom-up: new catalytic sites are built into protein scaffolds to promote a target transformation. These designer enzymes can be optimized experimentally to achieve high efficiencies. Blue circles represent mutations of amino acid residues, red sticks/circles represent catalytically important positions. | |
2. Stabilization of rate-limiting transition states
As first proposed by Linus Pauling, enzymes accelerate chemical transformations by preferentially stabilizing the reaction transition state relative to the reactant or product states, thus reducing the activation energy.16 Several enzyme design strategies have been developed that seek to capitalize on this mechanistic framework by developing proteins with high affinity for a target transition state or transition state analogue. One approach is to exploit the mammalian immune system to raise antibodies towards stable transition state analogues (or haptens).17 To date over 100 distinct reactions have been accelerated by catalytic antibodies including amide and ester hydrolysis, aldol condensations, oxidations and reductions.18–21 Catalytic antibody technology also gave rise to the first known protein catalysts for bimolecular Diels–Alder cycloadditions, which are valuable transformations that generate two new carbon–carbon bonds and up to four stereocentres.22,23 In one example, the antibody catalyst IE9 was generated for the conversion of tetrachlorothiophene dioxide (1) and N-ethylmaleimide (2) to a dihydrophthalimide product (3) using the stable bicyclic hapten (5), that resembles the boat-like conformation of the cycloaddition transition state leading to intermediate (4) (Fig. 2).22,24 In this case, tetrachlorothiophene dioxide was selected as the diene, as extrusion of sulphur dioxide from the Diels–Alder adduct minimizes product inhibition that is likely to occur due to the close resemblance of Diels–Alder products and the transition state.
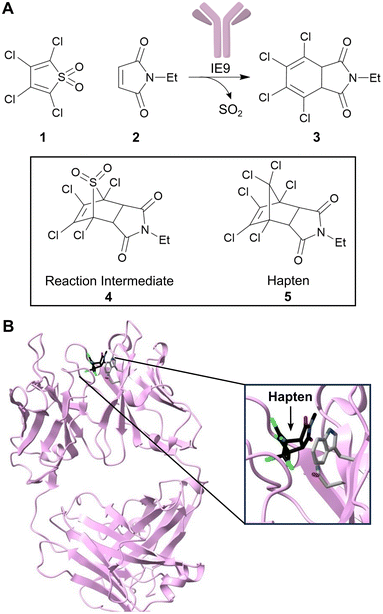 |
| Fig. 2 Development of a catalytic antibody for the Diels–Alder reaction. (A) Catalytic antibody IE9 catalyzes the Diels–Alder reaction between (1) and (2). IE9 was elicited using the stable bicyclic hapten (5). (B) Crystal structure of IE9 (PDB: 1C1E)24 with hapten (5) bound (shown as atom coloured sticks with carbons in black). Residues TrpH50 and AsnH35 which are involved in hapten binding, are shown as atom coloured sticks with grey carbons. | |
Despite their early promise, many catalytic antibodies suffered from low catalytic efficiencies compared with natural enzymes. Moreover, their rigid structures and a limited understanding of their catalytic mechanisms made further optimization through rational engineering or directed evolution challenging. In recent years, computational enzyme design has emerged as an attractive strategy to develop new protein catalysts that overcome some of the limitations of catalytic antibody technology. This approach is not reliant on the availability of imperfect transition state analogues and is also not restricted to the rigid antibody fold.10,11
Computational enzyme design involves initial development of an idealised active site model known as a ‘theozyme’, which comprises a quantum mechanically calculated transition state, alongside key functional residues required for its stabilization (Fig. 3A). The theozyme is then docked into structurally characterized proteins from the Protein Data Bank (PDB), using programs such as RosettaMatch,25,26 ORBIT27 or ScaffoldSelection,28 to identify promising scaffolds based on their steric complementarity to the theozyme. Finally, residues around the enzyme active site are redesigned computationally to optimize packing of the theozyme.11 The most promising designs are then produced and characterized experimentally.
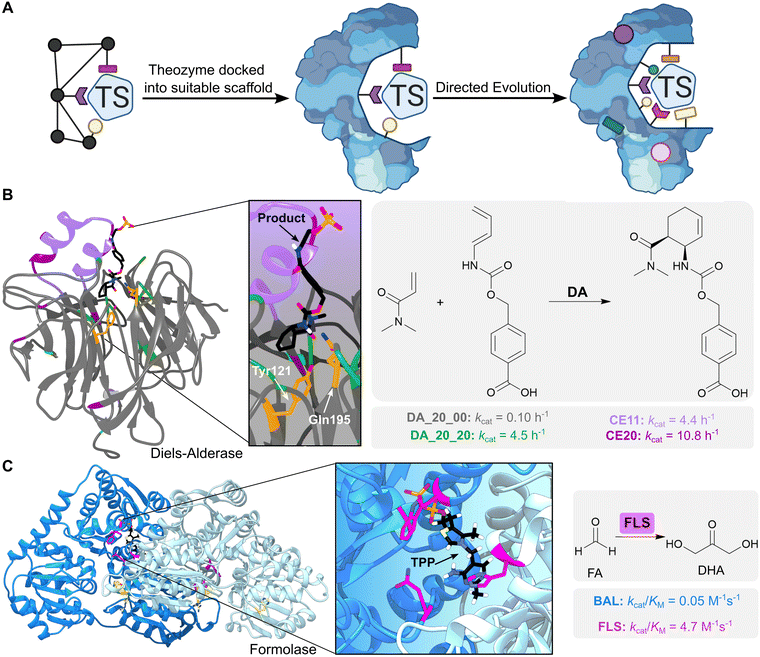 |
| Fig. 3 Computational enzyme design using theozymes. (A) Computational enzyme design first involves creating an active site model known as a ‘theozyme’. The theozyme is docked into proteins from the PDB to find scaffolds with suitable geometry, alongside computationally optimizing the active site by modifying nearby residues. The designed enzyme can then be further optimized by directed evolution. Coloured shapes represent mutations introduced by computational design and directed evolution to develop new enzymes. TS = transition state. (B) A Diels–Alderase was generated by in silico design to catalyse the Diels–Alder cycloaddition of 4-carboxybenzyl-trans-1,3-butadiene-1-carbamate with N,N-dimethyl acrylamide (reaction product is shown as atom coloured sticks with black carbons). The theozyme contained amino acid side chains which could act as hydrogen bond donors (Tyr121 and Gln195, shown as orange-coloured sticks) and was docked into a protein scaffold DA_20_00 (grey ribbon PDB: 3U0S). The enzyme was optimized by directed evolution (residue positions highlighted in green), the addition of a computationally designed lid element (residues highlighted in purple), and error-prone PCR (residues highlighted in dark purple). (C) A formolase (FLS) was created to promote the carboligation of formaldehyde (FA) to produce dihydroxyacetone (DHA), by computationally optimizing the active site of a benzaldehyde lyase (BAL). FLS is active as a dimer (blue ribbons, PDB: 4QQ8) and uses a thiamine pyrophosphate (TPP) cofactor (atom coloured sticks with black carbons). FLS was first optimized computationally (residues highlighted in pink), followed by improvement of activity via error-prone PCR (residues highlighted in yellow). | |
Thus far, computational enzyme design has given rise to protein catalysts for a variety of reactions, however their efficiencies are typically modest. Nevertheless, in many cases these systems have proven amenable to evolutionary optimization, producing more efficient biocatalysts, which, in some instances, have activities in line with natural enzymes.29,30
One notable example is the in silico design and experimental optimization of a proficient biocatalyst for the Diels–Alder cycloaddition of 4-carboxybenzyl-trans-1,3-butadiene-1-carbamate with N,N-dimethyl acrylamide (Fig. 3B).31 Initial computational designs were generated using a theozyme that incorporated amino acid side chains as hydrogen bond donors and acceptors to activate the substrates by lowering the energy gap between the highest occupied and lowest unoccupied molecular orbitals (the HOMO and LUMO). Following evaluation of 84 designs, the most active enzyme identified was DA_20_00, that used Tyr121 and Gln195 embedded within a rigid beta-propeller protein as the key hydrogen-bonding residues. Subsequent optimization of DA_20_00 was achieved through targeted mutagenesis, introduction of an additional lid element to shield the active site from solvent, and directed evolution.32,33 These engineering efforts gave rise to the Diels–Alderase CE20 which displays a 10
000-fold improvement in efficiency compared with the original DA_20_00 design (kcat/(Kdiene·Kdienophile) of 0.06 M−2 s−1 and 540 M−2 s−1 for DA_20_00 and CE20, respectively) and shows a high degree of selectivity for production of the RR-endo product. Structural analysis of CE20 and DA_20_00 reveals that orientation of the bound product and conformations of the Tyr and Gln residues closely match the original design model and changed minimally across the evolutionary trajectory. In this instance, activity gains were likely achieved through reshaping of the active site pocket to allow more effective pre-organization of the substrates into productive conformations for cycloaddition.
The combination of design and evolution has also given rise to efficient biocatalysts for other transformations, including Kemp elimination, retro-aldol, and Morita–Baylis–Hillman reactions.34–38 In these cases, evolution resulted in the emergence of new catalytic features that were not present in the original design models. For example, during evolutionary optimization of the designed retro-aldolase RA95, the catalytic Lys210 was abandoned in favour of Lys83 which formed part of a catalytic tetrad in the most highly engineered variant.39 Similarly, during the development of the Morita–Baylis–Hillmanase, BH32.14, a flexible Arg124 emerged during evolution to stabilize oxyanion intermediates formed along the reaction coordinate.38
Computational design can also be used to create new enzymes by dramatically reshaping the active site of natural systems whilst preserving some important catalytic elements. One notable example is the development of a formolase (FLS) enzyme that uses a thiamine pyrophosphate (TPP) cofactor to promote the carboligation of three molecules of formaldehyde (FA) into dihydroxyacetone (DHA) (Fig. 3C).40 The TPP-dependent enzyme benzaldehyde lyase (BAL), which catalyses the ligation of two benzaldehyde molecules to form benzoin, was selected as the template for computational re-engineering. The substrate binding pocket of BAL was optimized to accommodate the smaller formaldehyde substrates using RosettaDesign,41 a computational tool that allows identification of protein sequences with improved predicted affinity for small molecule ligands and transition states, followed by rational mutagenesis and error-prone PCR to further improve formolase activity. This combination of computational and experimental engineering afforded an FLS enzyme with a 100-fold increase in formose activity compared with the starting enzyme, and no detectable benzoin activity. The FLS enzyme has subsequently led to the development of new efficient carbon fixation pathways. For example, a further engineered FLS enzyme was a key component of an 11-step chemoenzymatic pathway for converting CO2 into starch.42
3. Designing and engineering of de novo metalloenzymes
The computationally designed enzymes described above have relied on the repurposing of natural protein folds. However, natural proteins are often only marginally stable and contain structural features such as long flexible loops that can be difficult to model computationally. As a result, introducing large numbers of designed mutations into natural scaffolds can result in poorly stable or insoluble proteins. These mutations often also result in unintended structural changes, leading to discrepancies between the initial design models and the experimentally characterized proteins. An attractive approach to overcome these challenges is to create de novo proteins, where in principle, we have a more complete understanding and control over sequence–structure relationships (Fig. 4A). This approach also enables us to create entirely new protein architectures, in theory allowing the development of protein backbones that are specifically tailored to accommodate complex theozyme arrangements.
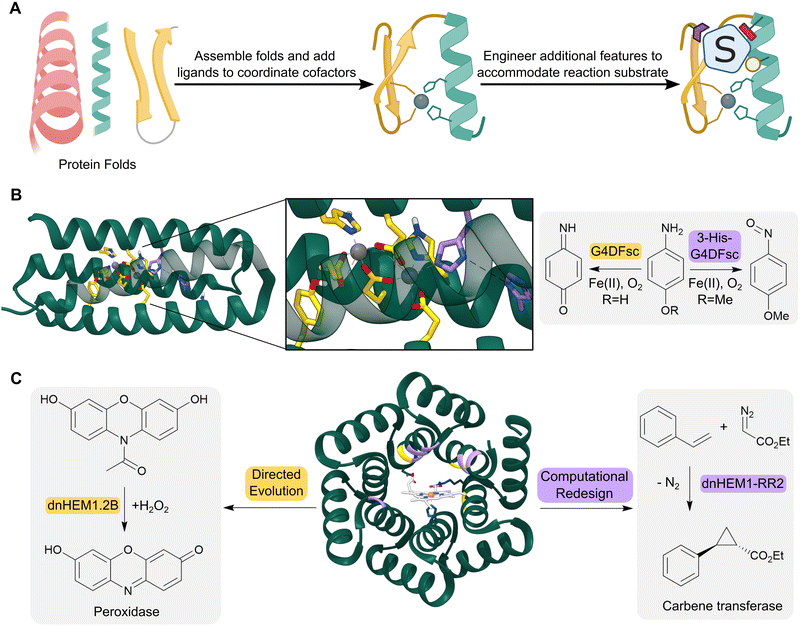 |
| Fig. 4 Design of de novo metalloenzymes. (A) The design of de novo metalloenzymes can be achieved by first selecting simple stable protein scaffolds. Ligands can then be introduced computationally to act as binding sites for metal-ion cofactors. Additional features can then be engineered to tailor substrate specificity and reactivity. (B) Helical bundles can act as highly evolvable scaffolds for enzyme design. For example, a computationally designed Due Ferri protein (green ribbon, PDB: 2LFD) utilizes binuclear non-heme iron centres, and has been engineered to catalyze two alternative chemical reactions, acting as either a 4-aminophenol oxidase (residues mutated highlighted as yellow sticks) or an N-hydroxylase (residues mutated highlighted as purple sticks). (C) Advances in computational design have led to increasingly elaborate de novo protein folds, such as the alpha-helical solenoid scaffold, which was computationally engineered to bind heme (shown as atom coloured sticks with white carbons) to give dnHEM1 (green ribbon, PDB: 8C3W). The protein was then redesigned both via directed evolution and additional computational design to produce enzymes with alternative reactivity, acting as either a peroxidase (directed evolution: dnHEM1.2B, mutated residues highlighted in yellow) or a carbene transferase (computational design: dnHEM1-RR2, mutated residues highlighted in purple). | |
Simple four-helix bundles have commonly served as protein scaffolds for de novo enzyme design due to their designability and customizability.43 By introducing binding sites for metal ion cofactors, de novo metalloenzymes have been developed for redox processes, hydrolytic reactions and carbene transfer chemistry.44–46 A notable example is the creation of a family of Due Ferri (DF) proteins that use carboxylate-bridged diiron centres to catalyse a range oxygen-dependent reactions (Fig. 4B).47–49
Enzymes have also been developed starting from designed peptides, using metal ions to template the assembly of higher order structures. For example, a homodimeric peptide containing two interfacial zinc binding sites was elaborated into a highly active and enantioselective Zn-hydrolase (MID1sc10) by fusing the N and C termini of the dimer subunits, removal of one of the zinc binding sites, and laboratory evolution.50 MID1sc was subsequently engineered into a highly active and stereoselective Diels–Alderase for the conversion of azachalcone and 3-vinylindole to the Diels Alder products (4S,6S)- and (4R,6R)-3,4-dihydro-2H-pyran.51 Through a combination of RosettaDesign and directed evolution, 12 mutations were introduced into MID1sc to afford DA7, which promotes the production of the optically pure endo-hetero-Diels–Alder product (4R,6R)-3,4-dihydro-2H-pyran with a kcat of 10 s−1. Activity increases observed during evolution were attributed to a significant conformational change that reduced the crossover angle of the two helix-turn-helix motifs to create a more enclosed hydrophobic pocket for substrate binding. Interestingly, evolution also resulted in an altered coordination environment around the Lewis acid's zinc centre, with one of the original coordinating His ligands (His39) replaced by a Cys35 ligand.
More recent advancements in computational protein design have led to the creation of a broader range of de novo protein folds that can be used as templates for designer enzymes. For example, a closed alpha-helical solenoid scaffold has recently been redesigned using RosettaMatch and RosettaDesign, into a high affinity heme binding protein (dnHEM1, KD <10 nM) (Fig. 4C).52 Structural characterization shows excellent agreement between the design model and experimental structure: His148 serves as the axial ligand to the heme iron, and a large reconfigurable distal pocket is available for substrate binding. The metalloenzyme dnHEM1 was subsequently engineered into a proficient peroxidase dnHEM1.2B (kcat = 130 s−1) through directed evolution. In parallel, dnHEM1 was reengineered computationally to afford an enantio-complementary carbene transferase biocatalyst, highlighting the versatility and engineerability of these de novo heme proteins.
4. Embedding new, non-canonical, functional elements into proteins
The range of chemistries achievable with designed enzymes can be greatly expanded by recruiting new functional elements that are not typically found in natural enzymes. One approach to achieve this goal is to introduce noble metal cofactors into proteins to generate artificial metalloenzymes.53,54 For example, replacing the iron porphyrin cofactor of heme proteins by a non-biological iridium porphyrin has given rise to efficient and selective catalysts for carbene insertions into C–H bonds.55–57 An alternative strategy to develop artificial metalloenzymes is to anchor preassembled transition metal complexes into selected protein scaffolds. This is commonly achieved by tethering the metal complex to a biotin handle to guide its incorporation into streptavidin (SVA) (Fig. 5A). Using this approach, artificial metalloenzymes have been developed for a variety of chemical transformations including transfer hydrogenations and alkene metathesis.58,59 A notable example is the design of a benzannulase, that uses a rhodium complex and a Glu121 base to promote enantioselective coupling of benzamides and alkenes to dihydroisoquinolones with a ca. 100-fold rate acceleration compared to the isolated metal complex (Fig. 5B).60
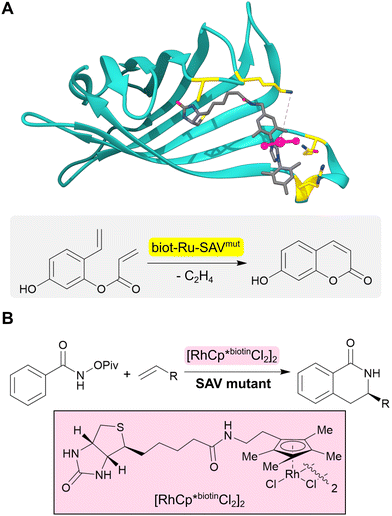 |
| Fig. 5 Design of artificial metalloenzymes. (A) Development of an artificial metalloenzyme by tethering a biotinylated ruthenium complex (grey sticks and pink ball and stick) to streptavidin (turquoise ribbon, PDB: 5F2B). Subsequent engineering of this protein revealed biot-Ru-SAVmut (mutated residues highlighted in yellow) which can catalyze an abiological olefin metathesis reaction. (B) Benzannulase (also based on streptavidin) uses a rhodium complex (pink box) to catalyse a benzannulation reaction for the synthesis of enantioenriched dihydroisoquinolones. | |
An alternative approach for adding new functional elements is by harnessing the novel reactivities achievable by non-natural amino acids. Catalytic sites in proteins are often made up from amino acid side chains. However, the range of functional groups found within the 20 canonical amino acids is limited (Fig. 6A). A powerful approach for introducing new chemistries into proteins is to embed new functional groups as non-canonical amino acid side chains.61–63 This can be achieved using genetic code expansion technology, where orthogonal aminoacyl-tRNA synthetase (aaRS)–tRNA pairs are used to selectively install non-canonical amino acids (ncAAs) in response to an unassigned codon (typically the UAG stop codon). This approach has been used to develop metalloenzymes with non-standard coordination environments and to develop enzymes that employ ncAAs as non-canonical catalytic nuclophiles.64–66
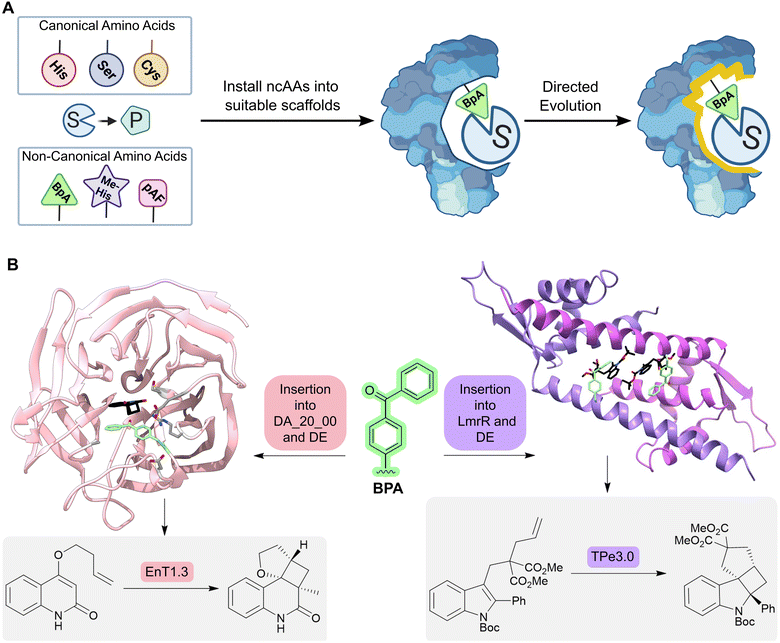 |
| Fig. 6 Designing enzymes with noncanonical amino acids. (A) For some reactions, there may not be a natural amino acid which can act as a catalytic residue to conduct the desired chemical transformation from substrate (S) to product (P). However, some non-canonical amino acids (ncAAs) may be able to catalyze the desired transformation; these residues can be embedded into a protein scaffold to access new chemistries. However, ncAA-containing enzymes often need to be optimized further by directed evolution. (B) Using an expanded genetic code enabled the creation of enzymes for triplet energy transfer photocatalysis, unlocking new reactivity in organic synthesis. By introducing a 4-benzoylphenylalanine (BpA, green sticks) as a noncanonical photosensitizer into the Diels–Alderase DA_20_00, an enantioselective photoenzyme for thermally forbidden [2 + 2]-cycloadditions was developed (EnT1.0). The enzyme was further optimized by directed evolution to give EnT1.3 (pink ribbon, PDB: 7ZP7, product of reaction represented as atom coloured sticks with black carbons, mutations highlighted as light grey sticks). Embedding of the same ncAA (BPA, green sticks) into a different scaffold, LmrR, allowed the development of a photoenzyme for intramolecular [2 + 2] photocycloadditions of N-substituted indole derivatives (TPe). TPe was further engineered by directed evolution to produce the most active variant TPe3.0 (PDB: 7XUQ, shown as a purple ribbon, with the product of the reaction represented as atom coloured sticks with black carbons). | |
More recently, an expanded genetic code has been used to create enzymes for triplet energy transfer photocatalysis, a versatile mode of reactivity in organic synthesis that was previously inaccessible to biocatalysts. Introduction of a 4-benzoylphenylalanine (BpA) photosensitizer into a previously designed Diels–Alderase scaffold, DA_20_00, gave rise to a modestly selective photoenzyme for thermally forbidden [2 + 2]-cyclizations (Fig. 6B).67 Subsequent optimization through laboratory evolution afforded the efficient and enantioselective photoenzyme EnT1.3 that selectively promotes a range of intramolecular and bimolecular cycloadditions, including those that have proved challenging for small-molecule photocatalysts. Structural characterization of an EnT1.3-product complex shows that the ligand sits in close proximity to the BpA photosensitizer and forms complementary hydrogen bonding interactions with Tyr121 and Gln195 in the active site pocket. In a simultaneous report from Sun et. al., photoenzymes were developed for intramolecular [2 + 2] photocycloadditions of N-substituted indole derivatives by embedding BpA into the transcriptional regulator protein LmrR (Fig. 6B).68 These studies highlight how the combination of genetic code expansion and directed evolution can afford enzymes that operate through new and valuable modes of catalysis.
5. The emergence of deep learning for protein design
Deep learning (DL) algorithms are able to efficiently analyse and extract valuable information from large, complex datasets and offer exciting new opportunities in enzyme design and engineering research.69 For example, DL has been used to distinguish catalytic and non-catalytic metal cofactors in proteins, improve protein stability and solubility, and to accelerate directed evolution campaigns.70–72 One of the major successes of DL lies in accurate protein structure prediction from primary amino acid sequences, with powerful tools such as AlphaFold2 having a transformative impact on the field.73 More recently, DL algorithms (e.g. Protein MPNN71) have been developed to address the inverse challenge, by predicting protein sequences that fold to generate a given protein backbone. Such methods can be used in conjunction with protein scaffold design tools (e.g. hallucination74 and RF diffusion75) to generate new functional proteins. For example, de novo metal and small ligand binding proteins have been developed by generating sequences and structures that scaffold a pre-defined functional site.75 The recent development of a de novo luciferase highlights the potential to apply these deep learning methods to enzyme design challenges. A family wide hallucination strategy was used to generate a panel of small, stable Nuclear Transport Factor 2 (NTF2)-like folds suitable for binding the synthetic luciferin substrate diphenylterazine (DTZ) (Fig. 7).76 Multiple conformations of DTZ were docked into the designed NTF2s using RifDock, followed by further optimization of the binding pocket using RosettaDesign. Following experimental testing of a panel of 7648 promising designs, three active sequences were identified. The most active of these, LuxSit, was subsequently optimized through targeted mutagenesis to generate a triple mutant of LuxSit, LuxSit-i, with a catalytic efficiency approaching that of natural luciferases (kcat/KM of 106 M−1 s−1).
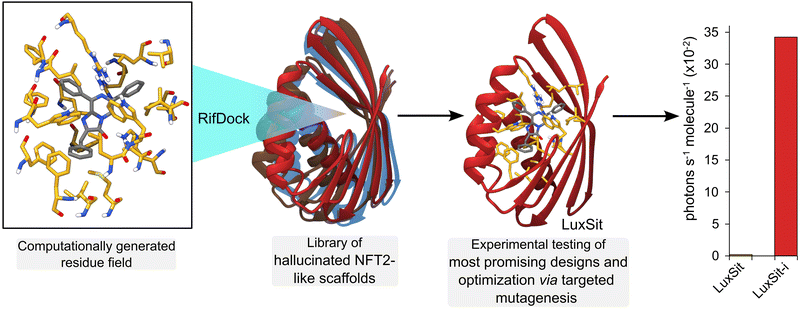 |
| Fig. 7 Deep learning for computational protein design. A de novo luciferase was created by computationally “hallucinating” a family of protein scaffolds based on Nuclear Transport Factor 2 (NFT2, multi-coloured ribbons). Into these scaffolds, a computationally generated residue interaction field (atom coloured sticks with yellow carbons) was docked with RifDock software that stabilized the reaction substrate diphenylterazine (DTZ, atom coloured sticks with grey carbons). Expression and testing of the most promising designs, followed by additional optimization by targeted mutagenesis led to the most active enzyme LuxSit-i (red ribbon, AlphaFold2 predicted structure), which was substantially more efficient than the starting scaffold (LuxSit). | |
6. Conclusions and outlook
As illustrated in this review, great progress has been made in the field of enzyme design and engineering over the past few decades. Using a combination of computational and experimental methodologies, enzymes have been developed for a range of non-biological transformations, thus expanding the toolbox of biocatalysts available for chemical synthesis. Remarkably in some cases, the enzymes developed display catalytic efficiencies in line with highly evolved natural systems.
Despite this impressive progress, there are several remaining challenges that must be addressed if enzyme design is to achieve the same level of practical utility as top-down engineering of natural enzymes. For instance, even with the latest methods, design success rates remain stubbornly low, meaning that large numbers of designs must be experimentally tested to identify active catalysts. This is, in part, due to a significant proportion of designed sequences being difficult to express in soluble form, coupled with our incomplete understanding of enzyme structure–function relationships. Even active designs tend to have low activity, and considerable experimental engineering is required to achieve the high efficiencies required for synthetic applications. These limitations mean that the development of new enzymes is both time consuming and expensive. Moving forward, it is likely that computational tools powered by deep learning will help to address some of these issues,71,74,75,77,78 by greatly improving design speed and model accuracy. The recent development of the de novo luciferase, LuxSit, highlights the enormous potential of deep learning-based design approaches, and it will be fascinating to see how these methods develop to achieve more complex chemical transformations in the future. In this regard, using such methods in conjunction with genetic code reprogramming techniques, to expand the range of catalytic sites that can be embedded into proteins, will likely prove a fruitful avenue for addressing more complex biochemical challenges.
In addition to overcoming the described hurdles, an important and complementary next step in the field is to move beyond model transformations and develop biocatalysts specifically tailored to produce high-value synthetic targets. Towards this goal, it is important to foster close collaborations with industry partners to identify suitable targets where enzyme design can have a major positive impact on manufacturing routes. The demonstration that designed enzymes can be developed and implemented in large-scale biomanufacturing would provide new impetus and attract greater investment into the field. Taking these challenges and opportunities into account, in our view we are about to embark on an exciting new era in enzyme design, where the latest tools for computational and experimental protein engineering will come together to deliver a step change in speed and scope of biocatalyst development. With these tools in hand, we are optimistic that the accurate and reliable design of enzymes will be achievable in the future to deliver tailored biocatalysts to meet diverse societal needs.
Author contributions
ELB, AEH, AJB and APG conceived and wrote the article. EO’R, AO’C and AB contributed towards ideas for content and provided critical commentary during manuscript preparation.
Conflicts of interest
There are no conflicts to declare.
Acknowledgements
We acknowledge the European Research Council (ERC Starting Grant no. 757991 to APG), the Biotechnology and Biological Sciences Research Council (David Phillips Fellowship BB/M027023/1 to APG and grants BB/W014483/1 and BB/X000974/1), the Human Frontier Science Program research grant (RGP0004/2022). ELB was funded by the U.S. Department of Energy, Office of Energy Efficiency and Renewable Energy and Bioenergy Technologies Office (BETO). This work was supported by AMMTO and BETO under contract no. DE-AC36-08GO28308 with the National Renewable Energy Laboratory, operated by Alliance for Sustainable Energy, LLC. ELB also acknowledges funding from the U.S. Department of Energy, Office of Science, Biological and Environmental Research Office. AJB was funded by the Scripps Institutional Postdoctoral Program. Any opinions, findings and conclusions or recommendations expressed in this material are those of the authors and do not necessarily reflect the views of the NSF. The views expressed in the article do not necessarily represent the views of the DOE or the U.S. Government. The U.S. Government retains and the publisher, by accepting the article for publication, acknowledges that the U.S. Government retains a nonexclusive, paid up, irrevocable, worldwide license to publish or reproduce the published form of this work, or allow others to do so, for U.S. Government purposes. AEH was supported by a BBSRC Industrial CASE PhD studentship (BB/S507040/1) supported by GSK. EO’R, AO’C and AB acknowledge financial support from the Science Foundation Ireland (SFI) Frontiers for the Future Project (19/FFP/6469). EO’R, AO’C and AB acknowledge financial support from the A2P-Centre for Doctoral Training, which is supported by Science Foundation Ireland (SFI) and the Engineering and Physical Sciences Research Council (EPSRC) (18/EPSRC-CDT/3582). BiOrbic is funded under the Science Foundation Ireland Research Centres Programme (16/RC/3889).
References
- N. J. Turner, Nat. Chem. Biol., 2009, 5, 567–573 CrossRef CAS PubMed.
- U. T. Bornscheuer, G. W. Huisman, R. J. Kazlauskas, S. Lutz, J. C. Moore and K. Robins, Nature, 2012, 485, 185–194 CrossRef CAS PubMed.
- C. K. Savile, J. M. Janey, E. C. Mundorff, J. C. Moore, S. Tam, W. R. Jarvis, J. C. Colbeck, A. Krebber, F. J. Fleitz, J. Brands, P. N. Devine, G. W. Huisman and G. J. Hughes, Science, 2010, 329, 305–309 CrossRef CAS PubMed.
- M. A. Huffman, A. Fryszkowska, O. Alvizo, M. Borra-Garske, K. R. Campos, K. A. Canada, P. N. Devine, D. Duan, J. H. Forstater, S. T. Grosser, H. M. Halsey, G. J. Hughes, J. Jo, L. A. Joyce, J. N. Kolev, J. Liang, K. M. Maloney, B. F. Mann, N. M. Marshall, M. McLaughlin, J. C. Moore, G. S. Murphy, C. C. Nawrat, J. Nazor, S. Novick, N. R. Patel, A. Rodriguez-Granillo, S. A. Robaire, E. C. Sherer, M. D. Truppo, A. M. Whittaker, D. Verma, L. Xiao, Y. Xu and H. Yang, Science, 2019, 366, 1255–1259 CrossRef CAS PubMed.
- E. L. Bell, R. Smithson, S. Kilbride, J. Foster, F. J. Hardy, S. Ramachandran, A. A. Tedstone, S. J. Haigh, A. A. Garforth, P. J. R. Day, C. Levy, M. P. Shaver and A. P. Green, Nat. Catal., 2022, 5, 673–681 CrossRef CAS.
- E. Radley, J. Davidson, J. Foster, R. Obexer, E. L. Bell and A. P. Green, Angew. Chem., Int. Ed., 2023, e202309305 CAS.
- A. O’Connell, A. Barry, A. J. Burke, A. E. Hutton, E. L. Bell, A. P. Green and E. O’Reilly, Chem. Soc. Rev., 2024 Search PubMed , submitted.
- C. Zeymer and D. Hilvert, Annu. Rev. Biochem., 2018, 87, 131–157 CrossRef CAS PubMed.
- E. L. Bell, W. Finnigan, S. P. France, A. P. Green, M. A. Hayes, L. J. Hepworth, S. L. Lovelock, H. Niikura, S. Osuna, E. Romero, K. S. Ryan, N. J. Turner and S. L. Flitsch, Nat. Rev. Methods Primers, 2021, 1, 46 CrossRef CAS.
- G. Kiss, N. Çelebi-Ölçüm, R. Moretti, D. Baker and K. N. Houk, Angew. Chem., Int. Ed., 2013, 52, 5700–5725 CrossRef CAS PubMed.
- D. Hilvert, Annu. Rev. Biochem., 2013, 82, 447–470 CrossRef CAS PubMed.
- P. J. Almhjell, C. E. Boville and F. H. Arnold, Chem. Soc. Rev., 2018, 47, 8980–8997 RSC.
- F. H. Arnold, Angew. Chem., Int. Ed., 2018, 57, 4143–4148 CrossRef CAS PubMed.
- R. K. Zhang, K. Chen, X. Huang, L. Wohlschlager, H. Renata and F. H. Arnold, Nature, 2019, 565, 67–72 CrossRef CAS PubMed.
- K. F. Biegasiewicz, S. J. Cooper, X. Gao, D. G. Oblinsky, J. H. Kim, S. E. Garfinkle, L. A. Joyce, B. A. Sandoval, G. D. Scholes and T. K. Hyster, Science, 2019, 364, 1166–1169 CrossRef CAS PubMed.
- L. Pauling, Nature, 1948, 161, 707–709 CrossRef CAS PubMed.
- A. Tramontano, K. D. Janda and R. A. Lerner, Science, 1986, 234, 1566–1570 CrossRef CAS PubMed.
- K. D. Janda, D. Schloeder, S. J. Benkovic and R. A. Lerner, Science, 1988, 241, 1188–1191 CrossRef CAS PubMed.
- L. C. Hsieh, S. Yonkovich, L. Kochersperger and P. G. Schultz, Science, 1993, 260, 337–339 CrossRef CAS PubMed.
- J. Wagner, R. A. Lerner and C. F. Barbas, Science, 1995, 270, 1797–1800 CrossRef CAS PubMed.
- P. Wentworth, L. H. Jones, A. D. Wentworth, X. Zhu, N. A. Larsen, I. A. Wilson, X. Xu, W. A. Goddard, K. D. Janda, A. Eschenmoser and R. A. Lerner, Science, 2001, 293, 1806–1811 CrossRef CAS PubMed.
- D. Hilvert, K. W. Hill, K. D. Nared and M. T. M. Auditor, J. Am. Chem. Soc., 1989, 111, 9261–9262 CrossRef CAS.
- A. C. Braisted and P. G. Schultz, J. Am. Chem. Soc., 1990, 112, 7430–7431 CrossRef CAS.
- J. Xu, Q. Deng, J. Chen, K. N. Houk, J. Bartek, D. Hilvert and I. A. Wilson, Science, 1999, 286, 2345–2348 CrossRef CAS PubMed.
- A. Zanghellini, L. Jiang, A. M. Wollacott, G. Cheng, J. Meiler, E. A. Althoff, D. Röthlisberger and D. Baker, Protein Sci., 2006, 15, 2785–2794 CrossRef CAS PubMed.
- J. K. Leman, B. D. Weitzner and S. M. Lewis,
et al.
, Nat. Methods, 2020, 17, 665–680 CrossRef CAS PubMed.
- D. N. Bolon and S. L. Mayo, Proc. Natl. Acad. Sci. U. S. A., 2001, 98, 14274–14279 CrossRef CAS PubMed.
- C. Malisi, O. Kohlbacher and B. Höcker, Proteins, 2009, 77, 74–83 CrossRef CAS PubMed.
- D. Röthlisberger, O. Khersonsky, A. M. Wollacott, L. Jiang, J. DeChancie, J. Betker, J. L. Gallaher, E. A. Althoff, A. Zanghellini, O. Dym, S. Albeck, K. N. Houk, D. S. Tawfik and D. Baker, Nature, 2008, 453, 190–195 CrossRef PubMed.
- L. Jiang, E. A. Althoff, F. R. Clemente, L. Doyle, D. Röthlisberger, A. Zanghellini, J. L. Gallaher, J. L. Betker, F. Tanaka, C. F. Barbas, D. Hilvert, K. N. Houk, B. L. Stoddard and D. Baker, Science, 2008, 319, 1387–1391 CrossRef CAS PubMed.
- J. B. Siegel, A. Zanghellini, H. M. Lovick, G. Kiss, A. R. Lambert, J. L. S. Clair, J. L. Gallaher, D. Hilvert, M. H. Gelb, B. L. Stoddard, K. N. Houk, F. E. Michael and D. Baker, Science, 2010, 329, 309–313 CrossRef CAS PubMed.
- C. B. Eiben, J. B. Siegel, J. B. Bale, S. Cooper, F. Khatib, B. W. Shen, F. Players, B. L. Stoddard, Z. Popovic and D. Baker, Nat. Biotechnol., 2012, 30, 190–192 CrossRef CAS PubMed.
- N. Preiswerk, T. Beck, J. D. Schulz, P. Milovník, C. Mayer, J. B. Siegel, D. Baker and D. Hilvert, Proc. Natl. Acad. Sci. U. S. A., 2014, 111, 8013–8018 CrossRef CAS PubMed.
- H. K. Privett, G. Kiss, T. M. Lee, R. Blomberg, R. A. Chica, L. M. Thomas, D. Hilvert, K. N. Houk and S. L. Mayo, Proc. Natl. Acad. Sci. U. S. A., 2012, 109, 3790–3795 CrossRef CAS PubMed.
- R. Blomberg, H. Kries, D. M. Pinkas, P. R. E. Mittl, M. G. Grütter, H. K. Privett, S. L. Mayo and D. Hilvert, Nature, 2013, 503, 418–421 CrossRef CAS PubMed.
- E. A. Althoff, L. Wang, L. Jiang, L. Giger, J. K. Lassila, Z. Wang, M. Smith, S. Hari, P. Kast, D. Herschlag, D. Hilvert and D. Baker, Protein Sci., 2012, 21, 717–726 CrossRef CAS PubMed.
- S. Bjelic, L. G. Nivón, N. Çelebi-Ölçüm, G. Kiss, C. F. Rosewall, H. M. Lovick, E. L. Ingalls, J. L. Gallaher, J. Seetharaman, S. Lew, G. T. Montelione, J. F. Hunt, F. E. Michael, K. N. Houk and D. Baker, ACS Chem. Biol., 2013, 8, 749–757 CrossRef CAS PubMed.
- R. Crawshaw, A. E. Crossley, L. Johannissen, A. J. Burke, S. Hay, C. Levy, D. Baker, S. L. Lovelock and A. P. Green, Nat. Chem., 2022, 14, 313–320 CrossRef CAS PubMed.
- R. Obexer, A. Godina, X. Garrabou, P. R. E. Mittl, D. Baker, A. D. Griffiths and D. Hilvert, Nat. Chem., 2017, 9, 50–56 CrossRef CAS PubMed.
- J. B. Siegel, A. L. Smith, S. Poust, A. J. Wargacki, A. Bar-Even, C. Louw, B. W. Shen, C. B. Eiben, H. M. Tran, E. Noor, J. L. Gallaher, J. Bale, Y. Yoshikuni, M. H. Gelb, J. D. Keasling, B. L. Stoddard, M. E. Lidstrom and D. Baker, Proc. Natl. Acad. Sci. U. S. A., 2015, 112, 3704–3709 CrossRef CAS PubMed.
- J. Meiler and D. Baker, Proteins, 2006, 65, 538–548 CrossRef CAS PubMed.
- T. Cai, H. Sun, J. Qiao, L. Zhu, F. Zhang, J. Zhang, Z. Tang, X. Wei, J. Yang, Q. Yuan, W. Wang, X. Yang, H. Chu, Q. Wang, C. You, H. Ma, Y. Sun, Y. Li, C. Li, H. Jiang, Q. Wang and Y. Ma, Science, 2021, 373, 1523–1527 CrossRef CAS PubMed.
- A. Lombardi, F. Pirro, O. Maglio, M. Chino and W. F. DeGrado, Acc. Chem. Res., 2019, 52, 1148–1159 CrossRef CAS PubMed.
- M. Faiella, C. Andreozzi, R. T. M. de Rosales, V. Pavone, O. Maglio, F. Nastri, W. F. DeGrado and A. Lombardi, Nat. Chem. Biol., 2009, 5, 882–884 CrossRef CAS PubMed.
- M. L. Zastrow, A. F.
A. Peacock, J. A. Stuckey and V. L. Pecoraro, Nat. Chem., 2012, 4, 118–123 CrossRef CAS PubMed.
- R. Stenner, J. W. Steventon, A. Seddon and J. L. R. Anderson, Proc. Natl. Acad. Sci. U. S. A., 2020, 117, 1419–1428 CrossRef CAS PubMed.
- O. Maglio, F. Nastri, V. Pavone, A. Lombardi and W. F. DeGrado, Proc. Natl. Acad. Sci. U. S. A., 2003, 100, 3772–3777 CrossRef CAS PubMed.
- A. J. Reig, M. M. Pires, R. A. Snyder, Y. Wu, H. Jo, D. W. Kulp, S. E. Butch, J. R. Calhoun, T. Szyperski, E. I. Solomon and W. F. DeGrado, Nat. Chem., 2012, 4, 900–906 CrossRef CAS PubMed.
- M. Chino, L. Leone, O. Maglio, D. D’Alonzo, F. Pirro, V. Pavone, F. Nastri and A. Lombardi, Angew. Chem., Int. Ed., 2017, 56, 15580–15583 CrossRef CAS PubMed.
- S. Studer, D. A. Hansen, Z. L. Pianowski, P. R. E. Mittl, A. Debon, S. L. Guffy, B. S. Der, B. Kuhlman and D. Hilvert, Science, 2018, 362, 1285–1288 CrossRef CAS PubMed.
- S. Basler, S. Studer, Y. Zou, T. Mori, Y. Ota, A. Camus, H. A. Bunzel, R. C. Helgeson, K. N. Houk, G. Jiménez-Osés and D. Hilvert, Nat. Chem., 2021, 13, 231–235 CrossRef CAS PubMed.
- I. Kalvet, M. Ortmayer, J. Zhao, R. Crawshaw, N. M. Ennist, C. Levy, A. Roy, A. P. Green and D. Baker, J. Am. Chem. Soc., 2023, 145, 14307–14315 CrossRef CAS PubMed.
- S. N. Natoli and J. F. Hartwig, Acc. Chem. Res., 2019, 52, 326–335 CrossRef CAS PubMed.
- A. S. Klein and C. Zeymer, Protein Eng., Des. Sel., 2021, 34, gzab003 CrossRef PubMed.
- P. Dydio, H. M. Key, A. Nazarenko, J. Y.-E. Rha, V. Seyedkazemi, D. S. Clark and J. F. Hartwig, Science, 2016, 354, 102–106 CrossRef CAS PubMed.
- S. A. Kerns, A. Biswas, N. M. Minnetian and A. S. Borovik, JACS Au, 2022, 2, 1252–1265 CrossRef CAS PubMed.
- H. M. Key, P. Dydio, D. S. Clark and J. F. Hartwig, Nature, 2016, 534, 534–537 CrossRef CAS PubMed.
- J. Zhao, J. G. Rebelein, H. Mallin, C. Trindler, M. M. Pellizzoni and T. R. Ward, J. Am. Chem. Soc., 2018, 140, 13171–13175 CrossRef CAS PubMed.
- M. Jeschek, R. Reuter, T. Heinisch, C. Trindler, J. Klehr, S. Panke and T. R. Ward, Nature, 2016, 537, 661–665 CrossRef CAS PubMed.
- T. K. Hyster, L. Knörr, T. R. Ward and T. Rovis, Science, 2012, 338, 500–503 CrossRef CAS PubMed.
- C. C. Liu and P. G. Schultz, Annu. Rev. Biochem., 2010, 79, 413–444 CrossRef CAS PubMed.
- J. W. Chin, Nature, 2017, 550, 53–60 CrossRef CAS PubMed.
- J. Zhao, A. J. Burke and A. P. Green, Curr. Opin. Chem. Biol., 2020, 55, 136–144 CrossRef CAS PubMed.
- A. J. Burke, S. L. Lovelock, A. Frese, R. Crawshaw, M. Ortmayer, M. Dunstan, C. Levy and A. P. Green, Nature, 2019, 570, 219–223 CrossRef CAS PubMed.
- I. Drienovská, C. Mayer, C. Dulson and G. Roelfes, Nat. Chem., 2018, 10, 946–952 CrossRef PubMed.
- C. Mayer, C. Dulson, E. Reddem, A. W. H. Thunnissen and G. Roelfes, Angew. Chem., Int. Ed., 2019, 58, 2083–2087 CrossRef CAS PubMed.
- J. S. Trimble, R. Crawshaw, F. J. Hardy, C. W. Levy, M. J. B. Brown, D. E. Fuerst, D. J. Heyes, R. Obexer and A. P. Green, Nature, 2022, 611, 709–714 CrossRef CAS PubMed.
- N. Sun, J. Huang, J. Qian, T.-P. Zhou, J. Guo, L. Tang, W. Zhang, Y. Deng, W. Zhao, G. Wu, R.-Z. Liao, X. Chen, F. Zhong and Y. Wu, Nature, 2022, 611, 715–720 CrossRef CAS PubMed.
- R. Feehan, D. Montezano and J. S. G. Slusky, Protein Eng., Des. Sel., 2021, 34, 1–10 CAS.
- R. Feehan, M. W. Franklin and J. S. G. Slusky, Nat. Commun., 2021, 12, 3712 CrossRef CAS PubMed.
- J. Dauparas, I. Anishchenko, N. Bennett, H. Bai, R. J. Ragotte, L. F. Milles, B. I. M. Wicky, A. Courbet, R. J. de Haas, N. Bethel, P. J. Y. Leung, T. F. Huddy, S. Pellock, D. Tischer, F. Chan, B. Koepnick, H. Nguyen, A. Kang, B. Sankaran, A. K. Bera, N. P. King and D. Baker, Science, 2022, 378, 49–56 CrossRef CAS PubMed.
- K. K. Yang, Z. Wu and F. H. Arnold, Nat. Methods, 2019, 16, 687–694 CrossRef CAS PubMed.
- J. Jumper, R. Evans, A. Pritzel, T. Green, M. Figurnov, O. Ronneberger, K. Tunyasuvunakool, R. Bates, A. Žídek, A. Potapenko, A. Bridgland, C. Meyer, A. A. A. Kohl, A. J. Ballard, A. Cowie, B. Romera-Paredes, S. Nikolov, R. Jain, J. Adler, T. Back, S. Petersen, D. Reiman, E. Clancy, M. Zielinski, M. Steinegger, M. Pacholska, T. Berghammer, S. Bodenstein, D. Silver, O. Vinyals, A. W. Senior, K. Kavukcuoglu, P. Kohli and D. Hassabis, Nature, 2021, 596, 583–589 CrossRef CAS PubMed.
- I. Anishchenko, S. J. Pellock, T. M. Chidyausiku, T. A. Ramelot, S. Ovchinnikov, J. Hao, K. Bafna, C. Norn, A. Kang, A. K. Bera, F. DiMaio, L. Carter, C. M. Chow, G. T. Montelione and D. Baker, Nature, 2021, 600, 547–552 CrossRef CAS PubMed.
- J. L. Watson, D. Juergens, N. R. Bennett, B. L. Trippe, J. Yim, H. E. Eisenach, W. Ahern, A. J. Borst, R. J. Ragotte, L. F. Milles, B. I. M. Wicky, N. Hanikel, S. J. Pellock, A. Courbet, W. Sheffler, J. Wang, P. Venkatesh, I. Sappington, S. V. Torres, A. Lauko, V. De Bortoli, E. Mathieu, S. Ovchinnikov, R. Barzilay, T. S. Jaakkola, F. DiMaio, M. Baek and D. Baker, Nature, 2023, 620, 1089–1100 CrossRef CAS PubMed.
- A. Yeh, C. Norn, Y. Kipnis, D. Tischer, S. Pellock, D. Evans, M. Pengchen, G. Lee, J. Zhang, I. Anishchenko, B. Coventry, L. Cao, J. Dauparas, S. Halabiya, M. DeWitt, L. Carter, K. Houk and D. Baker, Nature, 2023, 614, 774–780 CrossRef CAS PubMed.
-
R. Krishna, J. Wang and W. Ahern, et al., bioRxiv, 2023 DOI:10.1101/2023.10.09.561603.
-
J. Dauparas, G. R. Lee, R. Pecoraro and L. An, et al., bioRxiv, 2023 DOI:10.1101/2023.12.22.573103.
Footnote |
† These authors contributed equally. |
|
This journal is © The Royal Society of Chemistry 2024 |
Click here to see how this site uses Cookies. View our privacy policy here.