DOI:
10.1039/D4MH00791C
(Communication)
Mater. Horiz., 2024,
11, 5752-5767
Precise diagnosis of tumor cells and hemocytes using ultrasensitive, stable, selective cuprous oxide composite SERS bioprobes assisted with high-efficiency separation microfluidic chips†
Received
20th June 2024
, Accepted 27th August 2024
First published on 12th September 2024
Abstract
Efficient enrichment and accurate diagnosis of cancer cells from biological samples can guide effective treatment strategies. However, the accessibility and accuracy of rapid identification of tumor cells have been hampered due to the overlap of white blood cells (WBCs) and cancer cells in size. Therefore, a diagnosis system for the identification of tumor cells using reliable surface-enhanced Raman spectroscopy (SERS) bioprobes assisted with high-efficiency microfluidic chips for rapid enrichment of cancer cells was developed. According to this, a homogeneous flower-like Cu2O@Ag composite with high SERS performance was constructed. It showed a favorable spectral stability of 5.81% and can detect trace alizarin red (10−9 mol L−1). Finite-difference time-domain (FDTD) simulation of Cu2O, Ag and Cu2O@Ag, decreased the fluorescence lifetime of methylene blue after adsorption on Cu2O@Ag, and surface defects of Cu2O observed using a spherical aberration-corrected transmission electron microscope (AC-TEM) demonstrated that the combined effects of electromagnetic enhancement and promoted charge transfer endowed the Cu2O@Ag with good SERS activity. In addition, the modulation of the absorption properties of flower-like Cu2O@Ag composites significantly improved electromagnetic enhancement and charge transfer effects at 532 nm, providing a reliable basis for the label-free SERS detection. After the cancer cells in blood were separated by a spiral inertial microfluidic chip (purity >80%), machine learning-assisted linear discriminant analysis (LDA) successfully distinguished three types of cancer cells and WBCs with high accuracy (>90%). In conclusion, this study provides a profound reference for the rational design of SERS probes and the efficient diagnosis of malignant tumors.
New concepts
In this study, we first proposed a Cu2O@Ag-based diagnosis system for the identification of tumor cells using reliable surface-enhanced Raman spectroscopy (SERS) bioprobes, assisted with high-efficiency microfluidic chips for the rapid enrichment of cancer cells. The constructed homogeneous flower-like Cu2O@Ag composite showed high SERS activity, with a spectral stability of 5.81% and a limit of detection of 10−9 mol L−1. The mechanism study results, including the highest electromagnetic strength of Cu2O@Ag compared to Cu2O and Ag simulated by the finite difference time domain (FDTD) method, and the decreased fluorescence lifetime of methylene blue after adsorption of Cu2O@Ag demonstrated that the combined electromagnetic enhancement and promoted photo-induced charge transfer endowed the Cu2O@Ag composite with good SERS activity. Moreover, the absorption profile of the composite was modulated to facilitate the electromagnetic enhancement and charge transfer effects at 532 nm. According to the different sizes of cancer cells and blood cells, three types of cancer cells were enriched using an inertial microfluidic chip with high purity (>80%). Machine learning-assisted linear discriminant analysis (LDA) successfully distinguished cancer cells from white blood cells with high accuracy (>90%). It is believed that this study provides a profound reference for the rational design of SERS bioprobes for the efficient diagnosis of cancer.
|
1 Introduction
Accurate diagnosis of cancer can explore the biological characteristics of tumours and guide effective treatment strategies.1 The classic histopathological assay determines the histological type, grade and stage of the tumour relying on obtaining tissue slices and complicated immunohistochemical staining.2 In recent years, an emerging non-invasive liquid biopsy technique allows us to detect and grade cancer simply by collecting blood samples, significantly alleviating patient suffering and enabling long-term monitoring of cancer.3 However, due to the complex composition of blood samples, there is great interference from hemocytes (red and white blood cells) that have similar morphologies to tumour cells. Despite the significant difference in size, these large numbers of blood cells (approximately 5 billion red blood cells and 4000–11
000 white blood cells per millilitre)4 still make it extremely difficult to obtain and detect cancer cells. Therefore, developing efficient separation and enrichment techniques, and accurately identifying cancer cells, is of great necessity.
Microfluidic technology is a highly integrated platform for sample processing that combines physics, mechanical fabrication and biology.5 The separation and enrichment of different components in a sample can be achieved by manipulating the flow of particles in the tailored micro-channels.6 Inertial microfluidic chips use the inertial effects of fluids to focus and separate particles or cells with different sizes.7,8 Because of the rapid separation and high-throughput processing capabilities, no external energy intervention and cost economy, they have become a powerful tool for biological sample processing.9 A spiral-shaped inertial microfluidic chip consists of spiral-shaped channels and liquid entrances, which can achieve the removal of red blood cells (6–8 μm)10,11 and white blood cells (10–15 μm)12 and the enrichment of cancer cells (12–25 μm)13 in a few minutes.14 However, because the size of white blood cells overlaps with that of cancer cells, the cancer cells collected by microfluidic chips are often mixed with interference from some white blood cells.15 Therefore, it is very necessary to develop accurate recognition technology to distinguish cancer cells.
Label-free surface-enhanced Raman spectroscopy (SERS) can reflect the conformation and orientation information of substances by recording the fingerprint formed by the vibration of chemical bonds in substances.16 Owing to the great ability for molecular detection, it has been widely used in life science,17–20 food safety,21 and environmental protection.22 Traditional SERS techniques require the use of signalling molecules or expensive specific antibodies to enhance the signal and identify the target molecule. In contrast, the label-free SERS technique facilitates direct detection of analytes, avoiding the error and interference introduced by markers, which is of great significance for the efficient detection of target objects.23 Semiconductor materials can enhance Raman scattering by promoting charge transfer between the tested molecule and the SERS substrates showing good anti-interference, controllability and spectral stability,24,25 such as cuprous oxide (Cu2O),26,27 zinc oxide (ZnO),28 molybdenum trioxide (MoO3),29 titanium oxide (TiO2)30,31 and Ag2O.32 Despite these advantages, semiconductor-based SERS materials are limited by their inferior sensitivity. Another type of noble metal material is also commonly used as a SERS substrate, which can produce local electromagnetic field enhancement effects, thereby significantly enhancing the Raman scattering signal of target molecules.33,34 However, due to the random distribution of hot spots, the SERS enhancement of noble metals showed poor spectral reproducibility.35 In addition, non-selectivity and the difficulty of surface plasmon resonance (SPR) peaks to resonate with excitation wavelengths also limit their applications.36,37 Therefore, it is urgent to construct a reliable SERS probe with high sensitivity, good stability and selectivity. Cu2O is a good semiconductor that shows wide applications in solar energy conversion, catalysis, and SERS detection.38 Because of the high structural adjustability, it has become an important research object for composite SERS substrates of semiconductors and noble metals. The noble metal nanostructures of Ag can contribute to the formation of abundant hot spots and improve the activity of SERS substrates.39 Therefore, the composite of Cu2O and Ag may be a promising SERS probe.
Due to the diversity of samples and the complexity of spectral data, multivariate statistical methods are essential to analyse, interpret and represent the complex information contained therein.40 Multivariate statistical techniques based on machine learning can process large amounts of Raman spectral data, perform feature extraction, pattern recognition and data modelling, and then build predictive models based on cell recognition.41 To distinguish cell types, machine learning-assisted linear discriminant analysis (LDA) can handle large, high-dimensional data and improve classification accuracy through supervised training and evaluation of models.42,43 The SERS technology shows high specificity at the molecular level, enabling the detection of specific biomarkers within tumour cells. Microfluidic technology facilitates the separation and enrichment of tumour cells from complex samples, enhancing the detection sensitivity, specificity, and accuracy. Therefore, machine learning-assisted SERS technology enables rapid and precise analysis of tumour cells, reducing the detection time and costs, thereby offering significant translational potential.44
In this study, we developed a flower-like Cu2O@Ag composite SERS substrate to efficiently distinguish cancer cells from white blood cells using machine learning-assisted LDA after the separation of cancer cells from blood samples using an inertial microfluidic chip. SERS characterization proved that the composite had good Raman enhancement effect and spectral stability. According to the mechanism study, the highest electromagnetic strength of Cu2O@Ag compared to Cu2O and Ag simulated by the Finite Difference Time domain (FDTD) method and the decreased fluorescence lifetime of methylene blue after the adsorption of Cu2O@Ag endowed good SERS activity to the flower-like Cu2O@Ag composite. In addition, the surface defects of Cu2O were observed by spherical aberration-corrected transmission electron microscopy (AC-TEM). Therefore, the absorption profile of the flower-like Cu2O@Ag composite was modulated to facilitate the electromagnetic enhancement and charge transfer effects at 532 nm. After that, 20 μm particles were successfully enriched by using a spiral inertial microfluidic chip. According to this, cancer cells (purity >80%) were efficiently separated from blood cells. Machine learning-assisted LDA was subsequently used to distinguish the SERS spectra of three types of cancer cells (bladder cancer T24 cells, glioma U251 cells and pancreatic cancer Panc02 cells) and white blood cells. The resulting high accuracy (>90%) revealed that the constructed model had high confidence to identify cancer cells and white blood cells.
2 Experimental methods
2.1 Synthesis of flower-like Cu2O templates
PVP (Mw ≈ 1
300
000, 3 g, Macklin) and CuCl2·2H2O (171 mg) powder were dissolved in 100 mL deionized water. After the solution was totally clear, a NaOH aqueous solution (10.0 mL, 2.0 mol L−1) was slowly dropped in until the reaction colour changed from pale blue to light black. After stirring for 1 h, 10.0 mL 2.0 mol L−1 ascorbic acid aqueous solution was added. The mixture was then subjected to ageing for 3 h. Throughout the process, the solution was continuously stirred and heated in a water bath at 50 °C. The resulting precipitate was centrifuged and washed three times with deionized water and ethanol to remove the remaining polymer and ions. Finally, the product was dried in an oven at 50 °C for further characterization and use.
2.2 Synthesis of flower-like Cu2O@Ag
Flower-like Cu2O powder (4.5 mg) was first dispersed in 3 mL ethanol with ultrasonic vibration to prepare a suspension of Cu2O. The resulting suspension was then dissolved in 20 mL ethanol 80 mL under vigorous stirring. Subsequently, a solution of 1.2 mL of 30 mM anhydrous sodium citrate and 1.5 mL of 100 mM fresh NaBH4 were sequentially added to the solution. After stirring for 5 min, 1.5 mL AgNO3 solution (10 mM) was added dropwise into the mixture. When the colour of the reaction mixture turned bright yellow, it indicated the formation of small Ag nanoparticles. After continuing the reaction for 2 h, the solution was centrifuged and washed with deionized water and ethanol. At last, the collected particles were suspended in ethanol for further use and characterization.
2.3 Preparation of Cu2O@Ag-Raman reporter and Raman detection
Powder of Raman reporter was dissolved in ethanol and diluted to different concentrations. After natural drying at room temperature, the obtained precipitate was dropped onto a cleaned silicon slice (5 × 5 mm) using a micro-pipette for Raman detection. The SERS spectra were recorded using a confocal Raman microscope (Renishaw inVia Reflex) with a 532 nm laser as the excitation light source. Each spectrum was exposed for 5 s at a power of 0.1% w, and the process was repeated three times to accumulate the data.
2.4 Separation of fluorescent polystyrene microspheres on an inertial microfluidic chip
Three types of fluorescent polystyrene microsphere emulsions with diameters of 5 μm (Nile red-labelled fluorescence, 100 μL), 10 μm (DAPI-labelled blue fluorescence, 100 μL) and 20 μm (FITC-labelled green fluorescence, 400 μL) were mixed with 30 mg Tween 20. The mixture was then diluted with a 10 mM phosphate buffer (PBS, 30 mL) and ultrasonicated for 30 min. The prepared suspension was injected into a microfluidic chip with a precision pump (Pump 11 Elite, Harvard) at a speed of 700 μL min−1, and the particles’ flow trajectory was observed using an inverted fluorescence microscope (IX73, Olympus). After particles from central outlet and both side outlets were collected into plastic tubes, respectively, they were subjected to a flow cytometer (LSR Fortessa, Becton Dickinson) for recording the fluorescence intensity.
2.5 Cell culture and separation of blood samples
Human bladder cancer T24 cells transfected with GFP, human glioma U251 cells transfected with RFP, and mouse pancreatic cancer Luci-Panc02 cells transfected with GFP were kindly cultivated. All cells were cultured in DMEM with 10% fetal bovine serum and 1% penicillin–streptomycin in an incubator containing 5% CO2 at 37 °C. Luci-Panc02 cells were induced by potassium luciferase before use. Then, 10 μL anticoagulant rabbit blood was diluted with 10 mL PBS. About 105 fluorescent T24, U251 and Panc02 cells were added to the above sample and drawn into a 10 mL glass syringe. The prepared mixture of blood cells and cancer cells was injected into a microfluidic chip and the liquid from the central outlet and side outlets was collected, respectively. Using a fluorescence microscope, the sizes of fluorescent cancer cells and white blood cells in the central outlet were measured. The data were then analysed using the MedCalc software to plot the distribution pattern of the sizes of fluorescent cancer cells and white blood cells, including calculating the mean values and assessing their normality. The distribution of fluorescent cancer cells was then determined using a flow cytometer.
2.6 Label-free SERS detection of cells and data processing
The Cu2O@Ag ethanol suspension was dripped onto a clean silicon slice measuring 5 × 5 mm. After the liquid completely evaporated, the collected cells from the central outlet were coated onto the surface of silicon. The prepared sample was then placed under a confocal Raman microscope (Horiba, Labram Odyssey) to record label-free SERS data. The excitation source used was a 532 nm laser.
2.7 Raman data processing and statistical analysis
Fifty SERS spectra within the range of 600–1800 cm−1 of each cell line were employed to conduct LDA. The Python 3.6 programming language was used, with the basic code derived from rampy 0.4.6.45 To avoid overfitting, k-fold cross-validation was used. ROC curves were plotted to assess the model's performance. Furthermore, confusion matrices and prediction heatmaps were plotted using the Seaborn Python library code.
3 Results and discussion
3.1 Morphology characterization of flower-like Cu2O@Ag composites
The composite was prepared in two steps (Fig. 1(A)). After obtaining flower-like Cu2O particles, a silver (Ag) nanoparticle layer was reduced on the surface of the template. Flower-like Cu2O particles were synthesizing by the reduction of copper hydroxide using PVP as a templating agent and ascorbic acid as a reducing agent. As shown in Fig. 1(B), multilayer folding structures on the surface of flower Cu2O can be observed using a scanning electron microscope (SEM), which consisted of irregular and randomly arranged protrusions and crevices. These rough structures may provide more sites for hot spots of Ag particles.46 Besides, the prepared flower-like Cu2O was highly uniform in morphology, which laid the foundation for good SERS repeatability and stability (Fig. S1A, ESI†). The synthesized particles also showed clear lattice fringes under a high-resolution transmission electron microscope (HRTEM) (Fig. S2A, ESI†), indicating that the prepared material was a crystal. The lattice spacing was measured to be 0.242 nm, demonstrating a lattice orientation of (111). Accordingly, by selected area electron diffraction (SAED) detection, the crystal diffraction matrix of Cu2O was observed (Fig. S2B, ESI†). Similarly, the resulting characteristic crystal planes were (110), (111) and (220). In order to observe the distribution of copper (Cu) and oxygen (O) elements, the energy spectrum map scanning was performed. As shown in Fig. S3 (ESI†), the O and Cu elements were uniformly distributed in the prepared nanomaterial. After the synthesis of a Cu2O template, a layer of silver nanoparticles was prepared on the surface of flower-like Cu2O by in situ reduction. In order to observe the prepared Cu2O@Ag particles, SEM samples were prepared. It was found that the originally smooth surfaces became rough (Fig. 1(C)). This change can also be found in the transmission electron microscopic (TEM) images (Fig. S1B, ESI† and Fig. 1(D)). In addition, the prepared Cu2O@Ag maintained the high uniformity of the previous template (Fig. S4, ESI†).
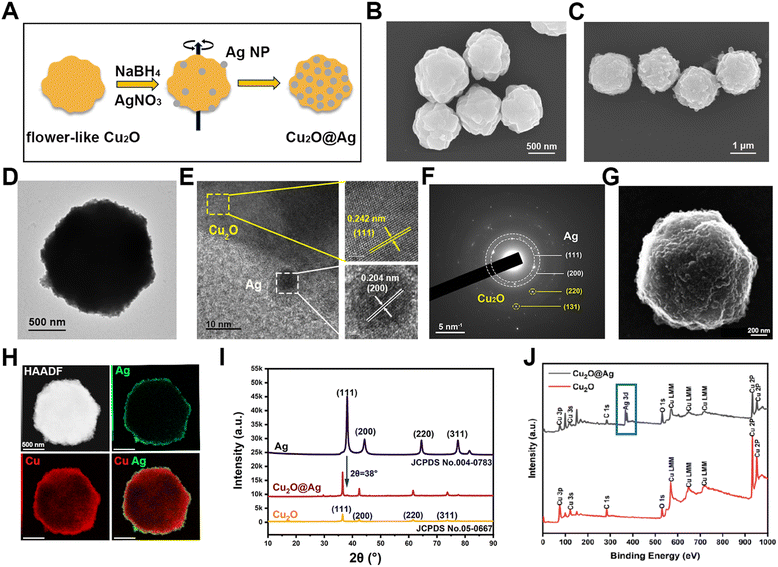 |
| Fig. 1 Morphology and physical property characterization of flower-like Cu2O and Cu2O@Ag composites. Synthesis diagram of the Cu2O@Ag composite (A); SEM image of flower-like Cu2O (B); SEM image (C), TEM image (D), HRTEM (E), SAED (F), SEI (G) and elemental distribution of the Cu2O@Ag composite (H); XRD spectra of Ag nanoparticles, flower-like Cu2O and Cu2O@Ag composites (I); XPS spectra of flower-like Cu2O and Cu2O@Ag composite (J). | |
To validate the formation of composites in a focused area, Cu2O@Ag was broken using an ultrasonic disrupter and characterized by HRTEM. It can be seen that small Ag nanoparticles (Ag NPs) were attached on the outer surface of Cu2O, as the characteristic lattice spacing (0.242 nm, (111)) and plane (0.204 nm, (200)) were recorded at the border of two materials (Fig. 1(E)). Moreover, the crystal diffraction pattern of Cu2O@Ag was acquired. The polycrystalline diffraction rings ((111) and (220)) were assigned to Ag NPs, while the diffraction spots ((220) and (311)) were attributed to Cu2O (Fig. 1(F)). Upon further observation of the material by scanning transmission electron microscopy (STEM), secondary electron imaging (SEI) also depicted the rough and uneven surface morphology of Cu2O@Ag (Fig. 1(G)).47 In addition, we explained the formation of Cu2O@Ag composites from the elemental distribution in the HAADF (high-angle annular dark field) mode (Fig. 1(H)). It is observed that the Ag element was distributed on the surface of the Cu element. This indicated the successful formation of Cu2O@Ag composites with a core–shell structure. Further the crystal property of powder samples of Ag NPs, Cu2O and Cu2O@Ag was measured by X-ray diffraction (XRD).48 The diffraction peaks of Cu2O (JCPDS no. 05-0667) and Ag (JCPDS no. 004-0783) are indexed to the International Centre for Diffraction Data (ICDD). The collected spectra showed that a characteristic peak at 2θ = 38° of Ag appeared in Cu2O@Ag, implying that Cu2O@Ag has been successfully fabricated.49 Another technique for detecting element components on the surface of materials, X-ray photoelectron spectroscopy (XPS), was also used to characterize the composites.50 In the range of binding energy from 1 to 1000 eV, Cu2O and Cu2O@Ag exhibited the same Cu orbital distribution (Fig. 1(J)), which can also be confirmed from the Cu 2p spectra (Fig. S5A, ESI†). The appearance of Ag 3d peak in the 300–400 eV range suggested the formation of the composite (see Fig. S5B for the fine XPS spectrum of Ag, ESI†).51,52
3.2 SERS performance of flower-like Cu2O@Ag composites
SERS performance was evaluated by the enhancement effect and spectral repeatability. By screening various Raman signaling molecules, it was found that the prepared flower-like Cu2O@Ag had good spectral enhancement ability for the alizarin red (AR), crystal violet (CV) and methylene blue (MB) molecules. As shown in Fig. 2(A), the AR molecule presented distinct characteristic peaks at concentrations above the nanomolar range enhanced by Cu2O@Ag. With regard to the limit of detection (LOD), it was found that the composite could detect alizarin red molecules as low as 10−9 mol L−1.
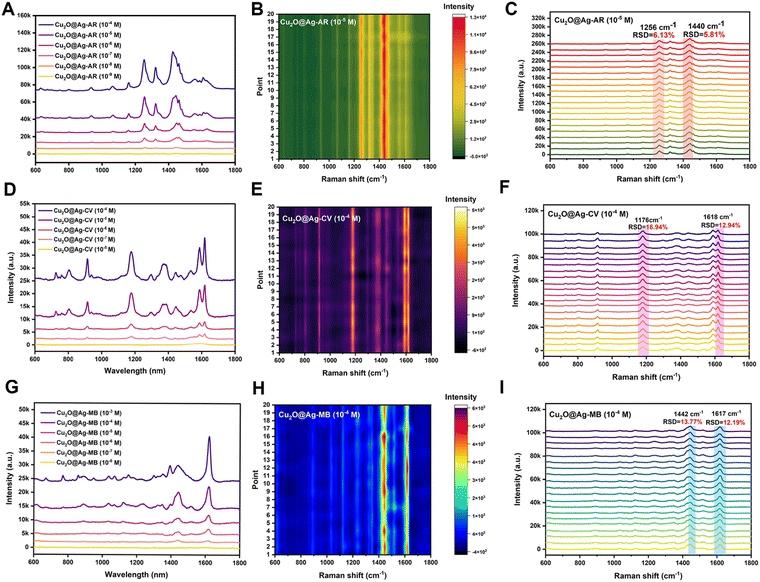 |
| Fig. 2 SERS performance of the flower-like Cu2O@Ag composite. SERS LOD spectra of Cu2O@Ag-AR (A), heat map of 20 spectra of Cu2O@Ag-AR (10−5 mol L−1) (B), SERS spectra with RSD values of Cu2O@Ag-AR (10−5 mol L−1) (C), SERS LOD spectra of Cu2O@Ag-CV (D), heat map of 20 spectra of Cu2O@Ag-CV (10−4 mol L−1) (E), SERS spectra with RSD values of Cu2O@Ag-CV (10−4 mol L−1) (F), SERS LOD spectra of Cu2O@Ag-MB (G), heat map of 20 spectra of Cu2O@Ag-MB (10−5 mol L−1) (H), and SERS spectra with RSD values of Cu2O@Ag-MB (10−4 mol L−1) (I). | |
In addition to the LOD, spectral repeatability is a property to be concerned. The repeatability was achieved by detecting the Raman spectra of several molecules at some specific peaks and calculating the relative standard deviation (RSD) of the intensity. As in Fig. 2(B), 20 Cu2O@Ag-AR (10−5 M) SERS spectra were recorded and plotted as a heatmap. The sharp contrast between the light and dark regions illustrated the general spectral stability, as the signal intensity in the red region was higher than that in the green region. To give a specific explanation of repeatability, the RSD values of the intensities corresponding to two of the peaks were calculated. The RSD is a value that describes the degree of dispersion of the data, ranging from 0 to 100%. In general, the smaller the RSD is, the closer the data is to the mean and the more centralized the data distribution is. The calculation of RSD is based on eqn (1):53
|  | (1) |
where
σ is the standard deviation of SERS intensity and
μ is the average value.
The SERS spectra of AR labelled with peak positions are shown in Fig. 2(C). In particular, the calculated RSD values of Raman shift at 1256 cm−1 and 1440 cm−1 were 6.13% and 5.81%, respectively. This indicated that the prepared materials not only had good signal enhancement ability for AR, but also exhibited high spectral stability. In addition to the AR molecule, the SERS performance of the prepared composite was explored for the CV and MB molecules. The LOD of the CV molecule detected was 10−8 mol L−1. Fig. 2(E) and (F) illustrate the spectral profiles of the CV molecule at a concentration of 10−4 mol L−1. The values at Raman shift of 1176 cm−1 and 1618 cm−1 were 16.94% and 12.94%, respectively. These results indicated the LOD and spectral repeatability of the enhanced spectra of CV were close to those of MB. The LOD of the MB molecule detected was 10−8 mol L−1. Fig. 2(H) and (I) show the spectral profiles of the MB molecule at a concentration of 10−4 mol L−1. The RSD values at Raman shift of 1442 cm−1 and 1617 cm−1 were 13.77% and 12.19%, respectively. Overall, the prepared flower-like Cu2O@Ag showed good generalization for its enhancement effect on various molecules.
Furthermore, in order to illustrate the storage stability of the Cu2O@Ag composite, the morphology and SERS performance were compared on day 1, 3, 5 and 7, respectively. The results (Fig. S6, ESI†) show that the morphology of flower-like Cu2O@Ag showed no significant change in at least one week, and the SERS enhancement effect remains stable.
3.3 Enhancement mechanism of flower-like Cu2O@Ag-based SERS
According to the reported enhancement mechanism, the significant SERS effect mainly comes from the electromagnetic enhancement (EE) dominated by noble metals and the charge transfer (CT) enhancement based on semiconductors.54 EE refers to a strong localized electric field enhancement effect generated by localized surface plasmon resonance (LSPR) when molecules are located near the surface of metal nanostructures. This localized electric field enhancement effect facilitates the excitation of the molecules’ vibration, thus enhancing the Raman signal of the molecule.55,56 The action of surface plasmon resonance (SPR) is related to the energy of the excited light. As can be seen from the absorption profiles (Fig. 3(A)), the optimal absorption peak of the composite was successfully regulated to around 532 nm after the introduction of Ag NPs on the multilayer folding of Cu2O. Given this feature, the SPR effect of Cu2O@Ag acquires a favourable match with the energy of excitation light, showing an accessibility to plasmon resonance in the visible region.57 By testing the same concentration of Raman signal molecules CV and 4 mercaptopyridine (4MPY) using a Raman spectrometer equipped with multiple lasers, it can be seen that the SERS intensity of the two molecules obtained the best enhancement at 532 nm excitation (Fig. 3(B) and (C)). A finite difference time domain (FDTD) method was used to simulate the electromagnetic field enhancement effect of Ag NPs, Cu2O and Cu2O@Ag composite. The results showed that the electric field generated by the Cu2O@Ag composite under excitation at 532 nm showed a higher electric intensity than that of pure Ag and Cu2O (Fig. 3(D)–(F)). To verify the extensibility of the theory, the electric field enhancement under excitation at 514 nm was also simulated. The strong electric field intensity demonstrated that the constructed composite could exert a stable electromagnetic enhancement effect (Fig. S7, ESI†). Further experimental comparison was made between the enhancement effect of Ag NPs and Cu2O@Ag for 4MPY molecules. The resulting SERS spectra is shown in Fig. 3(G). The peaks of Cu2O@Ag-4MPY at the Raman shift of 1019 cm−1, 1216 cm−1, and 1582 cm−1 were assigned to the symmetric vibrations of C–S, CH, and C
C bonds. The intensity of these peaks was significantly improved compared with Ag-4MPY, proving the obvious SRP effect. Therefore, the introduction of multilayer folding structure of Cu2O could effectively promote the hot spot effect between Ag and Ag, produce a strong SPR effect, and improve the EE. In addition, the enhancement of asymmetric C–H vibration at 1061 cm−1 comes from another charge transfer enhancement mechanism, which enhances the Raman signal through the CT between the SERS substrate and the adsorbed molecules.58 To quantify the contribution of CT to SERS, eqn (2) was used: | 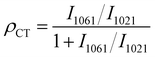 | (2) |
where I1061 is the strength at the Raman shift of 1061 cm−1 and I1021 is the strength at the Raman shift of 1021 cm−1. The calculated contribution of CT effects was 36.75%, which implied the synergistic effect of EE and CT. In addition, it can be found that the Cu2O@Ag composite presented a selective enhancement effect on 4MPY, since the strengths at 1061 cm−1, 1216 cm−1 and 1582 cm−1 were much higher than that of Ag-MB.
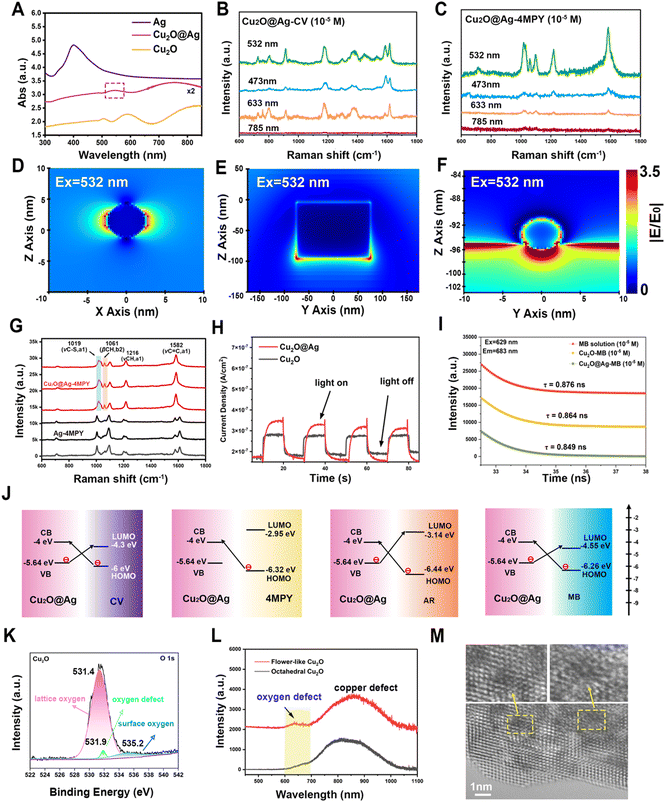 |
| Fig. 3 SERS enhancement mechanism of the flower-like Cu2O@Ag composite. Absorption profiles of prepared Cu2O, Cu2O@Ag and Ag nanoparticles (A), SERS spectra of Cu2O@Ag-CV (10−5 mol L−1) and Cu2O@Ag-4MPY (10−5 mol L−1) at different excitation lasers (B) and (C), simulated electromagnetic field enhancement of Ag nanoparticles, Cu2O and Cu2O@Ag composite at an excitation wavelength of 532 nm (D)–(F), SERS spectra of Cu2O@Ag-4MPY (10−5 mol L−1) and Ag-CV 4MPY (10−5 mol L−1) at an excitation wavelength of 532 nm (G), photocurrent density of Cu2O and Cu2O@Ag (H), time-resolved photoluminescence spectra of MB solution, Cu2O-MB and Cu2O@Ag-MB (10−5 mol L−1) (I), charge transfer diagram between Cu2O@Ag and CV, 4MPY, AR and MB (J), O1s spectrum of flower-like Cu2O (K), PL spectra of flower-like Cu2O and octahedral Cu2O (L), and surface defect of flower-like Cu2O under an AC-TEM (M). | |
The CT phenomenon of the Cu2O@Ag composite can also be confirmed from the improved photoelectric conversion ability.59 The photocurrent density under illumination and without illumination was recorded under the given light source intensity (Fig. 3(H)). The results showed that the CT ability of Cu2O was improved after the introduction of Ag particles.60
The CT between the molecules and the SERS substrate can also be explained by the changed fluorescence lifetime.61 After the fluorescence molecule MB was adsorbed on Cu2O and Cu2O@Ag, the fluorescence spectra (Fig. S8, ESI†) and time-resolved photoluminescence spectra (Fig. 3(I)) were recorded. It can be seen that the lifetime of MB decreased from 0.876 ns to 0.864 ns and 0.849 ns, respectively. The more decrease in Cu2O@Ag-MB implied more CT between MB molecules and the composite. These results indicated that the presence of Ag has promoted the CT of Cu2O-based SERS materials.62 Moreover, by measuring the XPS valence band curves (Fig. S9, ESI†), Mott–Schottky curves (Fig. S10, ESI†) and UV-diffuse reflection spectra (Fig. S11, ESI†) of powder samples, the energy bands of Cu2O and Cu2O@Ag were obtained (Table S1, ESI†).63 The reduced band gap of the Cu2O@Ag (1.64 eV) composite compared to Cu2O (1.97 eV) was favourable to the high-efficiency PICT resonance.30 As a result, the CT pathways between molecules and the composite are shown in Fig. 3(J). It is well documented that surface defects in semiconductor materials can also promote the CT process.64 According to the XPS O 1s spectra (Fig. 3(K)), lattice oxygen (531.4 eV), ambient oxygen (535.2 eV) and oxygen defect (531.9 eV) were detected.65,66 The oxygen defects account for 2.46% of the total oxygen content. Further examination of the photoemission spectra (Fig. 3(L)) showed that flower-like Cu2O had an oxygen defect peak at 640 nm and a copper defect peak at 855 nm. As a comparison, the PL spectra of octahedral Cu2O (Fig. S12, ESI†) were also detected, but no obvious oxygen defect peak was found. This difference suggested that the prepared flower-like material showed potential in facilitating CT. Moreover, the surface defects of flower-like Cu2O observed by spherical corrected aberration electron microscopy (AC-TEM) also provided evidence, as shown in the enlarged area in Fig. 3(M).
3.4 Inertial microfluidic chips for the separation of particles
An inertial microfluidic chip was used for particle sorting according to the force difference of particles with different sizes in the channel. In the spiral channel, the introduction of a periodic expansion structure can produce a stable vortex-induced lift force, and under the joint action of Dean resistance, particles with different sizes can quickly reach the equilibrium position and form a focused flow line. Therefore, a stable and efficient inertial separation microfluidic chip was employed according to a previous report.67 The working diagram of the chip is shown in Fig. 4(A). The 5 μm, 10 μm and 20 μm particles were used to simulate the movement of red blood cells (RBCs), white blood cells (WBCs) and cancer cells in the chip, respectively. After the mixed solution of the three particles passed into the inlet and equilibrate through the spiral channel, the smaller 5 μm and 10 μm particles will flow out of the chip via two side outlets, and the larger 20 μm particles will flow out via the middle outlet. A photograph of the utilized chip is shown in Fig. 4(B), and the chip consists of an inlet, a spiral channel (5 loops) with a periodic expansion structure, and three outlets. The detailed information of the chip is shown in Fig. S13 (ESI†). In order to clearly observe the migration route of particles with different particle sizes in the microfluidic channel, a fluorescently labelled polystyrene microsphere mixed solution was prepared and pumped into the chip at a constant flow rate of 700 μL min−1. A fluorescence microscope with a high-speed camera was used to observe and record the motion trajectories of three types of fluorescent microspheres, including 5 μm (red fluorescence), 10 μm (blue fluorescence) and 20 μm (green fluorescence). As can be seen in Fig. 4(C), the three types of fluorescent particles were in dispersed state and randomly distributed in the channel near the outlet. The 5 μm particles appeared to be dispersed in the first loop, but gradually moved towards both sides of the channel in the third loop. In the fifth loop, the 5 μm particles successfully focused into two streamlines and eventually flowed out through both side outlets. The 10 μm particles showed a similar migration pattern. After five-loop of focusing, most of the particles equilibrate and flowed out through the side outlets. After the initial dispersion, the 20 μm particles quickly focused in the first loop, gradually stabilized in the middle of the channel during the third and fifth loops, and finally flowed out towards the central outlet. These changes indicated that the inertial microfluidic chip can successfully separate the target particles in the mixed sample. In addition, the influence of flow rate on the separation effect was compared by recording the movement trajectories of 5 μm, 10 μm and 20 μm fluorescent microspheres at microfluidic channels and furcation. The results (Fig. S14, ESI†) show that the microfluidic chip has the best performance when the flow rate is 700 μL min−1, as the 20 μm particles can be focused on the center of the flow line. When the flow rate is reduced to 500 μL min−1, the 20 μm particles cannot be focused on the center of the flow line. As a result, some of the larger microspheres cannot be accurately discharged from the central outlet, resulting in reduced separation and enrichment performance. When the flow rate increased to 900 μL min−1, higher flow rates disrupt the microspheres’ movement trajectories within the chip, leading to clogging and subsequently reducing separation and enrichment efficiency.
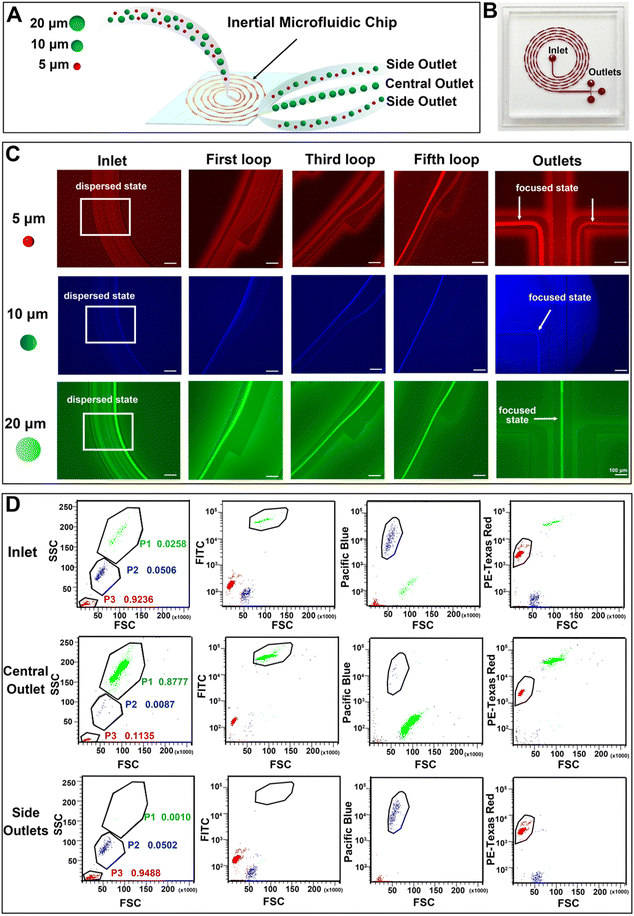 |
| Fig. 4 Separation effect of fluorescent microspheres by inertial microfluidic chip. Separation diagram of mixture of three types of micro-particles with diameter of 5 μm, 10 μm and 20 μm (A); photograph of inertial microfluidic chip with inlet, spiral channel, and outlets (B), fluorescent pictures of particles’ trajectories in outlet, first loop, third loop, fifth loop and outlets (C), comparison of fluorescent intensity and proportion of particles in collected liquid sample from inlet, central outlet and both side outlets (D). | |
The fluorescence intensities of liquid at the inlet (i.e. the unseparated microsphere mixture) and separated liquid collected by both the side outlets and the central outlet were recorded by flow cytometry. By comparing the three groups of scatter plots, it can be seen (Fig. 4(D)) that the number of 5 μm, 10 μm and 20 μm particles at the inlet accounted for 92.36%, 5.06% and 2.58% of the total samples, respectively. After the separation of the inertial microfluidic chip, the proportion of 20 μm particles collected at the central outlet increased to 87.77%, while the proportions of 5 μm and 10 μm particles reduced to 11.35% and 0.87%, respectively, which showed that the chip had a good enrichment ability for large particles. At the same time, the small particle size can be effectively eliminated. The proportions of 5 μm, 10 μm and 20 μm collected in the side outlets were 94.88%, 5.02% and 0.10%, respectively. These low proportions of 20 μm particles in the side outlets suggested the efficient screening effect of the inertial chip. Therefore, the chip can be used for the removal of blood cells and the enrichment of cancer cells.
3.5 Separation of cancer cells and label-free SERS identification of cancer cells and white blood cells
In order to simulate the separation of cancer cells in blood, a rabbit blood diluent mixed with fluorescent tumor cells was injected into a microfluidic chip for separation. Fluorescence and bright field images under a fluorescence microscope (Fig. 5(A)) showed the flow trajectories of fluorescently labelled bladder cancer T24 cells, glioma U251 cells, pancreatic cancer Panc02 cells, tumor cells, and blood cells in the inlet and outlets. After entering the chip, tumor cells and blood cells randomly spread around the inlet, and began to focus on the spiral channel. A large number of fluorescent tumor cells flowed out through the central outlet, while most blood cells flowed out through the side outlets. To further confirm that the chip had a significant enrichment effect on tumor cells, we performed flow cytometry detection of cell suspensions collected at the inlet and different outlets. Scatter plots of the liquid samples of the inlet, central outlet, and side outlets showed that the cell suspension was automatically divided into upper and lower groups of cells, because blood cells and fluorescent tumor cells had different particle sizes and fluorescence intensities. Through comparative analysis of the plots, it can be seen that the number of fluorescent tumor cells at the central outlet was significantly higher than that at the inlet and side outlets, and only a very small number of fluorescent tumor cells appeared to be collected in the side outlets (Fig. 5(B) and Fig. S15, ESI†). In our experiments, the cell separation was repeated, and the processing time was recorded. The calculated average processing time is 16.20 ± 0.06 min. Compared to the study by Bakhshi, M. S.,68 where the separation flux of the microfluidic chip for whole blood was 12.2 mL h−1, our microfluidic chip demonstrated a significantly higher separation flux of 36.1 mL h−1. As a result, the time required for tumor cell separation from whole blood samples in this work was notably reduced. In the subsequent quantitative analysis, it was found that the number of fluorescent tumor cells at the inlet of T24, U251 and Panc02 accounted for 10.80%, 21.10% and 10.90% of the total samples, respectively. The proportion of T24, U251 and Panc02 fluorescent tumor cells collected from the central outlet of the chip increased to 88.70%, 81.40% and 85.70%, respectively, while the proportion of red blood cells (RBCs) and white blood cells (WBCs) reduced to 11.30%, 18.60% and 14.30%, respectively. Meanwhile, the proportion of blood cells collected at side outlets was 97.70%, 97.48% and 94.78%, respectively, while the proportion of three fluorescent tumor cells, T24, U251 and Panc02, was 2.30%, 2.52% and 5.22%, respectively, which proved that the chip had a good separation and enrichment effect on tumor cells. In addition, the purity and recovery rate of the collected tumour cells were calculated according to eqn (3) and (4):69 |  | (3) |
|  | (4) |
The average purity of T24, U251 and Panc02 at the central outlet was 87.36%, 82.35% and 80.51%, respectively, and the average recovery rate was 92.95%, 88.01% and 82.79%, respectively (Fig. 5(C)). Compared to other related research, the purity was higher than that of cascaded sinusoidal channels (70.41%)69 and the recovery rate was consistent with another curved expansion-contraction array (CEA) microchannel (80.31%).70 In addition, the presence of serum in blood could potentially impact the purity and recovery rate of blood cells and cancer cells during separation using the microfluidic chip. To address this concern, we conducted an experiment where fluorescent tumor cells were injected into both serums with a consistent dilution ratio and serum-free samples. Microfluidic screening was then performed on both sets of samples. The cell suspensions collected from the central outlet were analyzed by cytometry. The results showed no statistically significant difference in the average fluorescence intensity of fluorescent tumor cells between the two groups (Fig. S16, ESI†), indicating that the number of tumor cells sorted by the microfluidic chip was comparable in both cases. At this dilution concentration, the influence of serum on the sorting efficiency of the microfluidic chip is minimal.
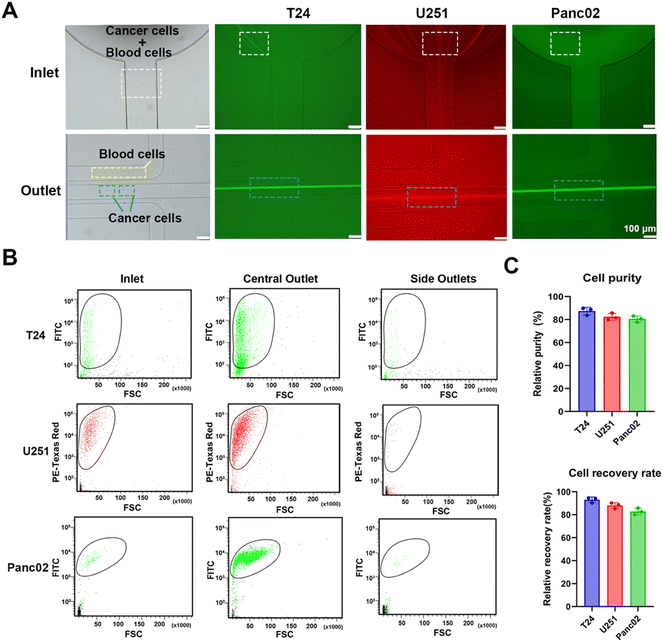 |
| Fig. 5 Separation of fluorescently labelled cancer cells from the blood sample by an inertial microfluidic chip. Bright-field image of a mixture of cancer cells in the blood sample at chip inlet and fluorescence images of three cancer cells (T24, U251, and Panc02) separated from the blood mixture at the central outlet, respectively (A); flow cytometry scatter plots of fluorescent cells collected at the inlet, central outlet, and side outlets of the chip (B); and purity and recovery rate of cancer cells collected from the central outlet of the microfluidic chip (C). | |
However, it should be noticed that about 13–20% of blood cells were collected. It was found by fluorescence microscopy that most of these blood cells were WBCs with a diameter greater than 10 μm, which was also consistent with a large number of microfluidic chip screening results.71,72 The presence of WBCs greatly interfered with the accurate detection of label-free SERS detection of subsequent tumour cells and restricted the establishment of machine learning prediction model. To tackle with this issue, the diameter of 200 WBCs and 200 fluorescent tumour cells collected at the central outlet was randomly obtained and their normality was evaluated. The results showed that the mean diameter of WBCs was 15.8 μm, while the mean diameter of T24, U251 and Panc02 fluorescent tumour cells was 22.22 μm, 22.79 μm and 20.92 μm, respectively, and the cell sizes of all types were in line with normal distribution (Fig. S17, ESI†). Therefore, the size difference between tumour cells and WBCs was the basis for the accurate acquisition of subsequent label-free SERS detection. Cells with a diameter less than 15 μm were regarded as WBCs, while those with a diameter greater than 20 μm were regarded as tumour cells. Subsequently, tumour cells and WBCs were subjected to Cu2O@Ag-based SERS detection and machine learning-assisted efficient identification.
Previous studies have shown that the combination of machine learning and SERS detection can accurately distinguish nucleic acids,73 exosomes74 and proteins.75 LDA algorithm has the advantages of insensitivity to outliers, good dimensional flexibility, fast computation speed and strong adaptability to multiple classification tasks.76 LDA enhances class separation by maximizing the ratio of between-class variance to within-class variance, making it particularly effective in handling the multidimensional spectral data typical of SERS. By identifying the optimal linear combinations of features, LDA can reduce the impact of certain types of noise, thereby improving stability and generalization, especially in high-dimensional datasets. Moreover, LDA's low computational complexity allows for the rapid processing of data, making it an ideal choice for situations where quick and reliable classification is required without the overhead of more complex models. LDA performs particularly well in scenarios where the assumptions of linear separability and similar covariance structures among classes hold true, consistently providing robust and interpretable results.77 Therefore, a binary classification model based on the LDA algorithm was used, and 5-fold cross-validation was applied to prevent the occurrence of overfitting. Each of the 50 SERS spectra of WBCs and T24, U251 and Panc02 cells was randomly collected, and the average spectra are shown in Fig. S18 (ESI†). The data set was composed of each tumour cell and white blood cell spectra, and the training set and test set were divided in a ratio of 7/3. The results showed that the accuracy of this classification model in distinguishing between T24 and WBCs, U251 and WBCs, and Panc02 and WBCs reached 96.48%, 90.23% and 93.33%, respectively, indicating that the established classification model had excellent robustness and generalization ability. Subsequently, the overall performance of the classification model was evaluated in detail through the confusion matrix. The results showed that the overall accuracy of the classification model for T24, U251 and Panc02 cells reached 94%, 94% and 100% (as shown in Fig. 6(A)), and the F1-score was 0.88, 0.87 and 1.00, respectively. The receiver operating characteristic (ROC) curve is a graph used to represent the performance (robustness and reliability) of a machine learning classifier by plotting the relationship between the true positive rate (TPR) and the false positive rate (FPR). The area under the curve (AUC) of the ROC curve, which varies from 0 to 1, is a quantitative indicator of the performance of the classifier, and a larger AUC indicates better reliability.78 The ROC curves (Fig. 6(B)) demonstrated the excellent overall performance of the model in multiple mixed samples with the classification model showing a sensitivity of 94.44% and a specificity of 94.10% and an AUC of 0.97 when distinguishing the mixed samples of T24 cells and WBCs. In the mixed samples of U251 cells and WBCs, the sensitivity of the classification model reached 94.44%, the specificity reached 100%, and the AUC value also reached the optimal 1.00. For a mixture of Panc02 cells and WBCs, the model also showed 100% sensitivity and specificity, with an AUC value of 1.00, reflecting its ability to consistently and accurately identify different types of tumour cells and WBCs. These results demonstrated the high-efficiency and reliability of the classification model in distinguishing between tumour cells and WBCs. In order to evaluate the prediction accuracy of the classification model for an unknown single-sample, we drew the prediction heat map and score map for a single-sample according to the analysis results of the previously obtained data, ROC curve and confusion matrix. The prediction heat map (Fig. 6(C)) and score map (Fig. 6(D)) represent the confidence of each sample classification by the depth of colour, where the darker the colour, the higher the classification confidence. Specifically, in predicting a single mixed sample, the model achieved 89% and 91% accuracy in identifying T24 and WBCs, 94% and 96% accuracy in identifying U251 and WBCs, respectively, while the model predicted Panc02 and WBCs with 100% accuracy. These data further confirmed the excellent performance of the classification model in single-sample prediction. The heat map showed the model's performance in predicting three types of tumours from a single sample, as well as the fluctuations that may occur when observing multiple single-sample predictions. The classification model developed in this study outperforms the model proposed by Lee79 in predicting bladder cancer cells. This result highlighted that the adoption of machine learning algorithms can significantly improve the predictive accuracy of models. In addition, this experimental model showed better reliability than Carmicheal80 with regard to distinguishing pancreatic cancer cells. This difference may be due to the fact that the SERS spectra of Panc02 cells were directly recorded in this study, rather than the spectrum of exosomes of pancreatic cancer cells. This makes the obtained spectral data not only more accurate, but also more fully reflect the inherent characteristics and heterogeneity of Panc02 cells, thus making the prediction model more effective in distinguishing Panc02 cells from leukocytes. Therefore, the classification model provided a solid foundation for the follow-up clinical individual sample testing.
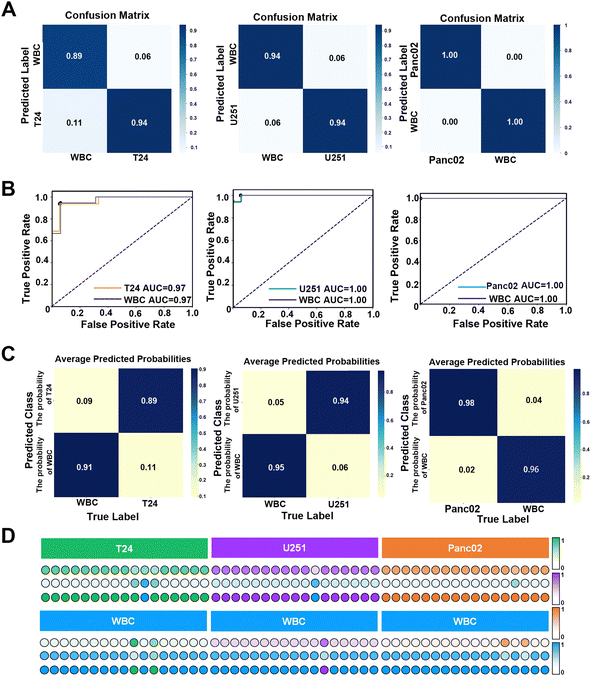 |
| Fig. 6 Prediction results of LDA classification between cancer cells and WBCs. Confusion matrixes (A, columns represent the actual label and rows show the predicted label), ROC curves (B), prediction heat maps (C, columns represent the predicted class and rows show the actual class) and score maps (D) of the LDA classification model in accurately classifying three types of cancer cells (T24, U251 and Panc02) and WBCs in the blood sample. | |
4 Conclusions
To realize efficient enrichment and accurate diagnosis of cancer cells in blood samples, a diagnosis system for the identification of tumor cells using reliable SERS probes assisted with high-efficiency microfluidic chips for rapid enrichment of cancer cells was developed. According to this, a homogeneous flower-like Cu2O@Ag composite was developed. The SERS performance results proved that the composite had a good Raman enhancement effect and stable spectral repeatability. It was demonstrated that the combined effects of EE and promoted CT endowed Cu2O@Ag with good SERS activity. In addition, the presence of defect states detected by photoluminescence spectroscopy and spherical aberration-corrected transmission electron microscopy (AC-TEM) also promoted charge transfer. A spiral inertial microfluidic chip was used for separating cancer cells from blood with a purity greater than 80%. Machine learning-assisted LDA was employed to distinguish the SERS spectra of three types of cancer cells and white blood cells, the high accuracy (>90%) revealed that the constructed classification model had high confidence and reliability. This study can give a forward-looking guide for the rational design of SERS probes and the efficient diagnosis of malignant tumors.
Data availablity
The data supporting this article have been included as part of the ESI.†
Conflicts of interest
There are no conflicts to declare.
Acknowledgements
This work is financially supported by National Natural Science Foundation of China (no. 32025021, 12374390, 31971292), National Key Research and Development Program (2023ZD0500902), the member of Youth Innovation Promotion Association Foundation of CAS, China (2023310), and the Key Scientific and Technological Special Project of Ningbo City (2023Z209, 2023Z189).
References
- A. Pulumati, A. Pulumati and B. S. Dwarakanath,
et al., Technological advancements in cancer diagnostics: Improvements and limitations, Cancer Rep., 2023, 6(2), e1764, DOI:10.1002/cnr2.1764.
- C. Dunn, D. Brettle and M. Cockroft,
et al., Quantitative assessment of H&E staining for pathology: development and clinical evaluation of a novel system, Diagn. Pathol., 2024, 19(1), 42, DOI:10.1186/s13000-024-01461-w.
- Z. Li, J. Shu and B. Yang,
et al., Emerging non-invasive detection methodologies for lung cancer, Oncol. Lett., 2020, 19(5), 3389–3399, DOI:10.3892/ol.2020.11460.
- A. Farahinia, W. J. Zhang and I. Badea, Novel microfluidic approaches to circulating tumor cell separation and sorting of blood cells: A review, J. Sci.: Adv. Mater. Devices, 2021, 6(3), 303–320, DOI:10.1016/j.jsamd.2021.03.005.
- A. G. Niculescu, C. Chircov, A. C. Bîrcă and A. M. Grumezescu, Fabrication and Applications of Microfluidic Devices: A Review, Int. J. Mol. Sci., 2021, 22(4), 2011, DOI:10.3390/ijms22042011.
- S. F. Berlanda, M. Breitfeld, C. L. Dietsche and P. S. Dittrich, Recent Advances in Microfluidic Technology for Bioanalysis and Diagnostics, Anal. Chem., 2021, 93(1), 311–331, DOI:10.1021/acs.analchem.0c04366.
- Z. Zhu, H. Ren and D. Wu,
et al., High-throughput and simultaneous inertial separation of tumor cells and clusters from malignant effusions using spiral-contraction-expansion channels, Microsyst. Nanoeng., 2024, 10(1), 36, DOI:10.1038/s41378-024-00661-0.
- W. Tang, S. Zhu and D. Jiang,
et al., Channel innovations for inertial microfluidics, Lab Chip, 2020, 20(19), 3485–3502, 10.1039/D0LC00714E.
- D. Huang, J. Man and D. Jiang,
et al., Inertial microfluidics: Recent advances, Electrophoresis, 2020, 41(24), 2166–2187, DOI:10.1002/elps.202000134.
- M. Kinnunen, A. Kauppila, A. Karmenyan and R. Myllylä, Effect of the size and shape of a red blood cell on elastic light scattering properties at the single-cell level, Biomed. Opt. Express, 2011, 2(7), 1803–1814, DOI:10.1364/boe.2.001803.
-
L. Dean and L. Dean, Blood groups and red cell antigens, NCBI Bethesda, 2005 Search PubMed.
- J. Prinyakupt and C. Pluempitiwiriyawej, Segmentation of white blood cells and comparison of cell morphology by linear and naïve Bayes classifiers, Biomed. Eng. Online, 2015, 14, 63, DOI:10.1186/s12938-015-0037-1.
- S.-J. Hao, Y. Wan and Y.-Q. Xia,
et al., Size-based separation methods of circulating tumor cells, Adv. Drug Delivery Rev., 2018, 125, 3–20, DOI:10.1016/j.addr.2018.01.002.
- M. E. Warkiani, B. L. Khoo and D. S. Tan,
et al., An ultra-high-throughput spiral microfluidic biochip for the enrichment of circulating tumor cells, Analyst, 2014, 139(13), 3245–3255, 10.1039/c4an00355a.
- C. Ni, Y. Chen and Y. Zhou,
et al., Inertia-magnetic microfluidics for rapid and high-purity separation of malignant tumor cells, Sens. Actuators, B, 2023, 397, 134619, DOI:10.1016/j.snb.2023.134619.
- L. A. Lane, X. Qian and S. Nie, SERS Nanoparticles in Medicine: From Label-Free Detection to Spectroscopic Tagging, Chem. Rev., 2015, 115(19), 10489–10529, DOI:10.1021/acs.chemrev.5b00265.
- X.-S. Zheng, I. J. Jahn and K. Weber,
et al., Label-free SERS in biological and biomedical applications: Recent progress, current challenges and opportunities, Spectrochim. Acta, Part A, 2018, 197, 56–77, DOI:10.1016/j.saa.2018.01.063.
- J. Lin, J. Zheng and A. Wu, An efficient strategy for circulating tumor cell detection: surface-enhanced Raman spectroscopy, J. Mater. Chem. B, 2020, 8(16), 3316–3326, 10.1039/C9TB02327E.
- S. Sun, Q. Chen and Y. Li,
et al., Tumor-specific and photothermal-augmented chemodynamic therapy by ferrocene-carbon dot-crosslinked nanoparticles, SmartMat, 2022, 3(2), 311–322, DOI:10.1002/smm2.1119.
- J. Jiang, X. Cui and Y. Huang,
et al., Advances and Prospects in Integrated Nano-oncology, Nano Biomed. Eng., 2024, 16(2), 152–187, DOI:10.26599/NBE.2024.9290060.
- H. Li, S. A. Haruna and W. Sheng,
et al., SERS-activated platforms for chemical contaminants in food: Probes, encoding methods, and detection, TrAC, Trends Anal. Chem., 2023, 169, 117365, DOI:10.1016/j.trac.2023.117365.
- Q. Zou, X. Ai and T. Xue, Synthesis of environment-friendly and label-free SERS probe for Iron(III) detection in integrated circuit cleaning solution waste, Microchem. J., 2021, 169, 106549, DOI:10.1016/j.microc.2021.106549.
- Y. Liu, H. Zhou and Z. Hu,
et al., Label and label-free based surface-enhanced Raman scattering for pathogen bacteria detection: A review, Biosens. Bioelectron., 2017, 94, 131–140, DOI:10.1016/j.bios.2017.02.032.
- L. Chen, J. Tang and H. Ma,
et al., High-efficiency charge transfer on SERS-active semiconducting K2Ti6O13 nanowires enables direct transition of photoinduced electrons to protein redox centers, Biosens. Bioelectron., 2021, 191, 113452, DOI:10.1016/j.bios.2021.113452.
- Q. Wang, K. Chang, Q. Yang and W. Wu, Semiconductor-based surface-enhanced Raman scattering sensing platforms: State of the art, applications and prospects in food safety, Trends Food Sci. Technol., 2024, 147, 104460, DOI:10.1016/j.tifs.2024.104460.
- J. Lin, Y. Shang and X. Li,
et al., Ultrasensitive SERS Detection by Defect Engineering on Single Cu2O Superstructure Particle, Adv. Mater., 2017, 29(5), 1604797, DOI:10.1002/adma.201604797.
- J. Lin, W. Hao and Y. Shang,
et al., Direct Experimental Observation of Facet-Dependent SERS of Cu2O Polyhedra, Small, 2018, 14(8), 1703274, DOI:10.1002/smll.201703274.
- L. Yang, Y. Yang and Y. Ma,
et al., Fabrication of Semiconductor ZnO Nanostructures for Versatile SERS Application, Nanomaterials, 2017, 7(11), 398 CrossRef PubMed.
- J. Zhang, Y. Pan, Y. Chen and H. Lu, Plasmonic molybdenum trioxide quantum dots with noble metal-comparable surface enhanced Raman scattering, J. Mater. Chem. C, 2018, 6(9), 2216–2220, 10.1039/C7TC04807F.
- X. Wang, W. Shi and S. Wang,
et al., Two-Dimensional Amorphous TiO2 Nanosheets Enabling High-Efficiency Photoinduced Charge Transfer for Excellent SERS Activity, J. Am. Chem. Soc., 2019, 141(14), 5856–5862, DOI:10.1021/jacs.9b00029.
-
J. Lin and A. Wu, Surface-Enhanced Raman Spectrum of TiO2 Nanoparticle for Biosensing (TiO2 Nanoparticle Served as SERS Sensing Substrate), TiO2 Nanoparticles, 2020, pp. 133–152 DOI:10.1002/9783527825431.ch4.
- M. He, J. Lin and O. U. Akakuru,
et al., Octahedral silver oxide nanoparticles enabling remarkable SERS activity for detecting circulating tumor cells, Sci. China: Life Sci., 2022, 65(3), 561–571, DOI:10.1007/s11427-020-1931-9.
- B. Sharma, R. R. Frontiera and A.-I. Henry,
et al., SERS: Materials, applications, and the future, Mater. Today, 2012, 15(1), 16–25, DOI:10.1016/S1369-7021(12)70017-2.
- T. Lan, D. Cui and T. Liu,
et al., Gold NanoStars: Synthesis, Modification and Application, Nano Biomed. Eng., 2023, 15(3), 330–341, DOI:10.26599/NBE.2023.9290025.
- J. Yu, C. Chen and Q. Zhang,
et al., Au Atoms Anchored on Amorphous C3N4 for Single-Site Raman Enhancement, J. Am. Chem. Soc., 2022, 144(48), 21908–21915, DOI:10.1021/jacs.2c07413.
- Y. Xie, C. Chen and C. Zhang,
et al., Synergistic enhancement of ultrahigh SERS activity via Cu2O@Ag Core-Shell structure for accurate label-free identification of breast tumor subtypes, Nano Today, 2024, 54, 102140, DOI:10.1016/j.nantod.2023.102140.
- X. Wang, G. Ma and A. Li,
et al., Composition-adjustable Ag–Au substitutional alloy microcages enabling tunable plasmon resonance for ultrasensitive SERS, Chem. Sci., 2018, 9(16), 4009–4015, 10.1039/C8SC00915E.
- S. Sheng, Y. Ren and S. Yang,
et al., Remarkable SERS Detection by Hybrid Cu2O/Ag Nanospheres, ACS Omega, 2020, 5(28), 17703–17714, DOI:10.1021/acsomega.0c02301.
- D. Cheng, Y. Zhou and Q. Wang,
et al., Ag/ZIF-8 Substrate with Enhanced SERS via the Plasmonic Nanogap and MOF-Enabled Molecular Preconcentration Effect, J. Phys. Chem. C, 2023, 127(7), 3542–3550, DOI:10.1021/acs.jpcc.2c07974.
- M. E. Keating, H. Nawaz, F. Bonnier and H. J. Byrne, Multivariate statistical methodologies applied in biomedical Raman spectroscopy: assessing the validity of partial least squares regression using simulated model datasets, Analyst, 2015, 140(7), 2482–2492, 10.1039/C4AN02167C.
- D. P. dos Santos, M. M. Sena and M. R. Almeida,
et al., Unraveling surface-enhanced Raman spectroscopy results through chemometrics and machine learning: principles, progress, and trends, Anal. Bioanal. Chem., 2023, 415(18), 3945–3966, DOI:10.1007/s00216-023-04620-y.
- F. Lussier, V. Thibault and B. Charron,
et al., Deep learning and artificial intelligence methods for Raman and surface-enhanced Raman scattering, TrAC, Trends Anal. Chem., 2020, 124, 115796, DOI:10.1016/j.trac.2019.115796.
- L. M. Wurm, B. Fischer and V. Neuschmelting,
et al., Rapid, label-free classification of glioblastoma differentiation status combining confocal Raman spectroscopy and machine learning, Analyst, 2023, 148(23), 6109–6119, 10.1039/D3AN01303K.
- S. Peng, D. Lu and B. Zhang,
et al., Machine learning–assisted internal standard calibration label-free SERS strategy for colon cancer detection, Anal. Bioanal. Chem., 2023, 415(9), 1699–1707, DOI:10.1007/s00216-023-04566-1.
-
C. L. Losq, Rampy: a Python library for processing spectroscopic (IR, Raman, XAS.) data, 2018, https://zenodo.org/records/4715040.
- H. Liang, Z. Li and W. Wang,
et al., Highly Surface-roughened “Flower-like” Silver Nanoparticles for Extremely Sensitive Substrates of Surface-enhanced Raman Scattering, Adv. Mater., 2009, 21(45), 4614–4618, DOI:10.1002/adma.200901139.
- O. Dyck, J. Almutlaq and D. Lingerfelt,
et al., Direct imaging of electron density with a scanning transmission electron microscope, Nat. Commun., 2023, 14(1), 7550, DOI:10.1038/s41467-023-42256-9.
- Z.-F. Yao, Q.-Y. Li and H.-T. Wu,
et al., Building crystal structures of conjugated polymers through X-ray diffraction and molecular modeling, SmartMat, 2021, 2(3), 378–387, DOI:10.1002/smm2.1053.
- S. C. Abeyweera, M. Simukaitis, Q. Wei and Y. Sun, Interfaced Ag/Cu nanostructures derived from metal thiolate nanoplates: A highly selective catalyst for electrochemical reduction of CO2 to ethanol, SmartMat, 2022, 3(1), 173–182, DOI:10.1002/smm2.1096.
- M. A. Isaacs, J. Davies-Jones and P. R. Davies,
et al., Advanced XPS characterization: XPS-based multi-technique analyses for comprehensive understanding of functional materials, Mater. Chem. Front., 2021, 5(22), 7931–7963, 10.1039/D1QM00969A.
- P. F. Cai, J. Li and X. B. Wu,
et al., ALD-induced TiO(2)/Ag nanofilm for rapid surface photodynamic ion sterilization, Rare Met., 2022, 41(12), 4138–4148, DOI:10.1007/s12598-022-02096-w.
- B. Niu, S. Yang, Y. Yang and T. Hua, Highly conductive fiber with design of dual conductive Ag/CB layers for ultrasensitive and wide-range strain sensing, SmartMat, 2023, 4(6), e1178, DOI:10.1002/smm2.1178.
- C. A. Smyth, I. Mirza, J. G. Lunney and E. M. McCabe, Surface-enhanced Raman spectroscopy (SERS) using Ag nanoparticle films produced by pulsed laser deposition, Appl. Surf. Sci., 2013, 264, 31–35, DOI:10.1016/j.apsusc.2012.09.078.
- B. Yang, S. Jin and S. Guo,
et al., Recent Development of SERS Technology: Semiconductor-Based Study, ACS Omega, 2019, 4(23), 20101–20108, DOI:10.1021/acsomega.9b03154.
- J. Langer, D. Jimenez de Aberasturi and J. Aizpurua,
et al., Present and Future of
Surface-Enhanced Raman Scattering, ACS Nano, 2020, 14(1), 28–117, DOI:10.1021/acsnano.9b04224.
- X. Meng, L. Qiu and G. Xi,
et al., Smart design of high-performance surface-enhanced Raman scattering substrates, SmartMat, 2021, 2(4), 466–487, DOI:10.1002/smm2.1058.
- S. Cong, X. Liu and Y. Jiang,
et al., Surface Enhanced Raman Scattering Revealed by Interfacial Charge-Transfer Transitions, Innovation, 2020, 1(3), 100051, DOI:10.1016/j.xinn.2020.100051.
- A. Musumeci, D. Gosztola and T. Schiller,
et al., SERS of Semiconducting Nanoparticles (TiO2 Hybrid Composites), J. Am. Chem. Soc., 2009, 131(17), 6040–6041, DOI:10.1021/ja808277u.
- F. E. Bedoya-Lora, M. E. Valencia-García and A. Hankin,
et al., Determination of photon-driven charge transfer efficiency: Drawbacks, accuracy and precision of different methods using Hematite as case of study, Electrochim. Acta, 2022, 402, 139559, DOI:10.1016/j.electacta.2021.139559.
- X. Zhang, Z. Yu and W. Ji,
et al., Charge-Transfer Effect on Surface-Enhanced Raman Scattering (SERS) in an Ordered Ag NPs/4-Mercaptobenzoic Acid/TiO2 System, J. Phys. Chem. C, 2015, 119(39), 22439–22444, DOI:10.1021/acs.jpcc.5b06001.
- G. Song, W. Gong, S. Cong and Z. Zhao, Ultrathin Two-Dimensional Nanostructures: Surface Defects for Morphology-Driven Enhanced Semiconductor SERS, Angew. Chem., Int. Ed., 2021, 60(10), 5505–5511, DOI:10.1002/anie.202015306.
- M. M. Zalduendo, J. Langer and J. J. Giner-Casares,
et al., Au Nanoparticles–Mesoporous TiO2 Thin Films Composites as SERS Sensors: A Systematic Performance Analysis, J. Phys. Chem. C, 2018, 122(24), 13095–13105, DOI:10.1021/acs.jpcc.8b01444.
- X. Mo, B. Bouchet Fabre, N. Herlin-Boime and E. C. M. Tse, Rapid laser synthesis of surfactantless tantalum-based nanomaterials as bifunctional catalysts for direct peroxide–peroxide fuel cells, SmartMat, 2023, 4(6), e1181, DOI:10.1002/smm2.1181.
- C. Li, C. Wu and K. Zhang,
et al., The charge transfer effect on SERS in a gold-decorated surface defect anatase nanosheet/methylene blue (MB) system, New J. Chem., 2021, 45(42), 19775–19786, 10.1039/D1NJ03941E.
- M. Singh, D. Jampaiah and A. E. Kandjani,
et al., Oxygen-deficient photostable Cu2O for enhanced visible light photocatalytic activity, Nanoscale, 2018, 10(13), 6039–6050, 10.1039/C7NR08388B.
- J. Wang, Q. Huang and W. Zhao,
et al., Defect-induced plasmon resonance of SERS-active MoO2-x/Mo composite films by pulsed laser irradiation, Vacuum, 2023, 218, 112632, DOI:10.1016/j.vacuum.2023.112632.
- Y. Gou, S. Zhang and C. Sun,
et al., Sheathless Inertial Focusing Chip Combining a Spiral Channel with Periodic Expansion Structures for Efficient and Stable Particle Sorting, Anal. Chem., 2020, 92(2), 1833–1841, DOI:10.1021/acs.analchem.9b03692.
- M. S. Bakhshi, M. Rizwan and G. J. Khan,
et al., Design of a novel integrated microfluidic chip for continuous separation of circulating tumor cells from peripheral blood cells, Sci. Rep., 2022, 12(1), 17016, DOI:10.1038/s41598-022-20886-1.
- S. Ebrahimi, M. Alishiri and E. Pishbin,
et al., A curved expansion-contraction microfluidic structure for inertial based separation of circulating tumor cells from blood samples, J. Chromatogr. A, 2023, 1705, 464200, DOI:10.1016/j.chroma.2023.464200.
- H. Cha, H. Fallahi and Y. Dai,
et al., Tuning particle inertial separation in sinusoidal channels by embedding periodic obstacle microstructures, Lab Chip, 2022, 22(15), 2789–2800, 10.1039/D2LC00197G.
- H. Fallahi, S. Yadav and H.-P. Phan,
et al., Size-tuneable isolation of cancer cells using stretchable inertial microfluidics, Lab Chip, 2021, 21(10), 2008–2018, 10.1039/D1LC00082A.
- H. Jeon, B. Jundi and K. Choi,
et al., Fully-automated and field-deployable blood leukocyte separation platform using multi-dimensional double spiral (MDDS) inertial microfluidics, Lab Chip, 2020, 20(19), 3612–3624, 10.1039/D0LC00675K.
- M. G. Kim, M. Jue and K. H. Lee,
et al., Deep Learning Assisted Surface-Enhanced Raman Spectroscopy (SERS) for Rapid and Direct Nucleic Acid Amplification and Detection: Toward Enhanced Molecular Diagnostics, ACS Nano, 2023, 17(18), 18332–18345, DOI:10.1021/acsnano.3c05633.
- Y. Xie, X. Su and Y. Wen,
et al., Artificial Intelligent Label-Free SERS Profiling of Serum Exosomes for Breast Cancer Diagnosis and Postoperative Assessment, Nano Lett., 2022, 22(19), 7910–7918, DOI:10.1021/acs.nanolett.2c02928.
- M. Peng, Z. Wang and X. Sun,
et al., Deep Learning-Based Label-Free Surface-Enhanced Raman Scattering Screening and Recognition of Small-Molecule Binding Sites in Proteins, Anal. Chem., 2022, 94(33), 11483–11491, DOI:10.1021/acs.analchem.2c01158.
- Y. Liu, M. Li and H. Liu,
et al., Cancer diagnosis using label-free SERS-based exosome analysis, Theranostics, 2024, 14(5), 1966–1981, DOI:10.7150/thno.92621.
- R. Graf, M. Zeldovich and S. Friedrich, Comparing linear discriminant analysis and supervised learning algorithms for binary classification—A method comparison study, Biom. J., 2024, 66(1), 2200098, DOI:10.1002/bimj.202200098.
- J. N. Mandrekar, Receiver operating characteristic curve in diagnostic test assessment, J. Thorac. Oncol., 2010, 5(9), 1315–1316, DOI:10.1097/JTO.0b013e3181ec173d.
- S. Lee, M. Jue and K. Lee,
et al., Early-stage diagnosis of bladder cancer using surface-enhanced Raman spectroscopy combined with machine learning algorithms in a rat model, Biosens. Bioelectron., 2024, 246, 115915, DOI:10.1016/j.bios.2023.115915.
- J. Carmicheal, C. Hayashi and X. Huang,
et al., Label-free characterization of exosome via surface enhanced Raman spectroscopy for the early detection of pancreatic cancer, Nanomedicine, 2019, 16, 88–96, DOI:10.1016/j.nano.2018.11.008.
|
This journal is © The Royal Society of Chemistry 2024 |
Click here to see how this site uses Cookies. View our privacy policy here.