DOI:
10.1039/D3SD00175J
(Paper)
Sens. Diagn., 2024,
3, 287-294
Discrimination of mycoplasma infection using machine learning models trained on autofluorescence signatures of host cells†
Received
7th July 2023
, Accepted 21st November 2023
First published on 23rd November 2023
Abstract
Cellular autofluorescence signatures are generated by fluorescent metabolites and structural cellular components and are considered to represent the physiological state of individual cells. Since optimal microscopic imaging of cellular autofluorescence signals is rapid, nondestructive, and minimally invasive, the extraction and classification of autofluorescence signatures can be a powerful tool for discriminating different physiological states. Here, we investigated whether changes in the cellular autofluorescence signature of host cells can be used to diagnose mycoplasma infection. Analysis of cellular autofluorescence signals from monkey cells infected with mycoplasma revealed attenuated autofluorescence at several wavelengths. Machine learning models trained on individual cellular autofluorescence signatures achieved more than 70% accuracy in binary classification tasks, demonstrating the utility of autofluorescence signatures in the discrimination of mycoplasma-infected cells. Finally, quantitative measurements of NADH, a major fluorescent metabolite, revealed a ∼20% reduction of cellular NADH in mycoplasma-infected cells that might underlie the attenuation, especially under 405 nm excitation.
Introduction
Prokaryotic and eukaryotic cells exhibit innate fluorescence, called autofluorescence, that is emitted by light-absorbing intracellular biomolecules.1 Diverse biomolecules including tryptophan, collagen, elastin, cyclic ring compounds such as NADH, FAD, riboflavin, and aromatic amino acids, contribute to cellular autofluorescence.2–8 Eukaryotic cellular organelles, mitochondria, and lysosomes also emit specific autofluorescence, which facilitates the dynamic imaging of these organelles at high temporal and spatial resolution.9 Because cellular autofluorescence profiles are primarily determined by cellular properties and physiological status, it is possible to use autofluorescence to distinguish cells with different metabolisms. For example, the wild type Aspergillus nidulans and its nitrogen regulator deletion mutant are successfully identified by their respective cellular autofluorescence signatures.1 Moreover, human breast cancer tissues in different histological grades and tumor sizes, glioma stem cells that exhibit higher stem-like properties, and immune cells in different functional states can be categorized by cellular autofluorescence, especially when using signals corresponding to intracellular tryptophan, NADH and FAD levels, respectively.10–12
To acquire reliable autofluorescence signatures of individual cells from cell clusters, we developed the confocal reflection microscopy-assisted single-cell innate fluorescence (CRIF) system, which combines techniques from reflection confocal microscopy and confocal microspectroscopy.1,13 Additionally, machine learning models that were trained to classify microbial cell populations from autofluorescence images allowed us to distinguish individual cells even from heterogeneous populations under various physiological states.1,13 Notably, the most critical advantage of the CRIF system supported by machine learning models is that cells with different status can be discriminated without quantifying specific fluorescent biomolecules.
Mycoplasmas are the smallest and simplest self-replicating organisms. As obligatory heterotrophs, they require nutrients produced by host cells, and have evolved into obligate parasites of higher eukaryotes animals and plants.14 During infection, almost all mycoplasmas bind to the surface of the host cells and behave as surface parasites.15 Consequently, mycoplasma contamination is difficult to detect, and can give rise to major problems such as recurring and occult cell culture contamination.16 Existing detection methods, such as indirect fluorescent staining of infected cells, direct PCR of mycoplasma DNA, and agar–broth culture of mycoplasma cells,17 are invasive to host cells or are time-intensive. Therefore, rapid and minimally invasive detection methods, such as those that rely on simple imaging techniques, are desirable.
Mycoplasma infection is generally not lethal to host cells. However, infections elicit pathological changes to the host cells, including carcinogenesis and severe immune reactions associated with conditions such as AIDS.18,19 Indeed, mycoplasma infection often activates the major inflammatory responses, including the NF-κB and Nrf2 signaling pathways. Concomitantly, mycoplasma infection inhibits the p53-mediated response that triggers cell cycle arrest and apoptosis.15,20 These observations imply that mycoplasma infection might affect the physiological state of host cells, including signaling and metabolism pathways, and may thereby alter the autofluorescence signatures of host cells.
Here, we acquired cellular autofluorescence signals from intact and mycoplasma-infected living cells. Analyses of autofluorescence signatures revealed that mycoplasma infection resulted in the attenuation of several peaks under short-wavelength excitation. Supervised machine learning models successfully discriminated between healthy and mycoplasma-infected cells.
Results and discussion
Detection of mycoplasma infection of VERO
We hypothesized that mycoplasma infection causes changes in cellular autofluorescence through the modification of signaling and metabolism pathways. To generate mycoplasma-infected cells, we co-cultured Mycoplasmopsis fermentans with VERO cells, a well-known indicator cell line for mycoplasma infection derived from African green monkey kidney tissue. To confirm mycoplasma infection, cellular DNA were stained by Hoechst and imaged (Fig. 1A). Strong fluorescent signals were observed in the cytosol of mycoplasma-infected VERO cells [mycoplasma (+)], indicating infection with M. fermentans, but not healthy VERO cells [mycoplasma (−)]. We further confirmed infection using a PCR-based detection kit that amplifies the mycoplasma 16S rRNA gene (Fig. 1B). A distinct band was detected from supernatant of mycoplasma (+) cells, corresponding to that shown by the positive control (see arrowheads). In contrast, no bands were detected from the supernatant of mycoplasma (−) cells or the negative control (PBS). Having established that the mycoplasma (+) cells were indeed successfully infected with M. fermentans, we performed further analyses using these cells.
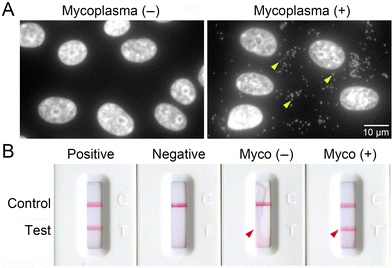 |
| Fig. 1 Detection of mycoplasma infection by (A) Hoechst staining of VERO cells, and (B) isothermal PCR using supernatant from VERO culture. Yellow arrowheads in (A) indicate strong fluorescence from the mycoplasma DNA present in mycoplasma (+) cells, and red arrowheads in (B) indicate the position of the positive band that appears when the cell culture supernatant from mycoplasma (+) cells is tested. | |
Autofluorescence imaging of trypsinized mycoplasma (−) and mycoplasma (+) cells
Compared to adherent cells, which are spread out, trypsinization reduces cell volume and thereby increases the fluorescent signal intensity per cell area in a focal plane. Therefore, trypsinized cells were used in order to acquire sufficient cellular autofluorescence signals from mycoplasma (−) and mycoplasma (+) cells. Trypsinized cells were inoculated into slide dishes and immediately imaged using a confocal microscope equipped with 6 lasers and 32 channel spectral detectors. Fig. 2A shows a representative set of autofluorescence images of mycoplasma (−) and mycoplasma (+) cells excited by 405, 458, 488, 514, 543, and 633 nm lasers, and reflection confocal microscopy (RCM) in the same focal plane. Subsequently, edges of individual cells were defined by the segmentation process (ESI† Fig. S1) and autofluorescence profiles of individual cells were extracted in 192 dimensions (6 lasers × 32 channels) using a custom MATLAB function from at least 3 sets of acquired fluorescence images. The extracted autofluorescence signatures of mycoplasma (−) and mycoplasma (+) cells were compared and visualized as hierarchical clustered heatmaps in which autofluorescence signatures of individual cells were aligned horizontally (Fig. 2B). Together with the fluorescence intensities averaged from more than 80 cell signatures (Fig. 2C), the results clearly indicate that autofluorescence signals of spherical, and thus unattached, VERO cells were attenuated by mycoplasma infection, especially when excited by 405, 458, 488, and 514 nm lasers.
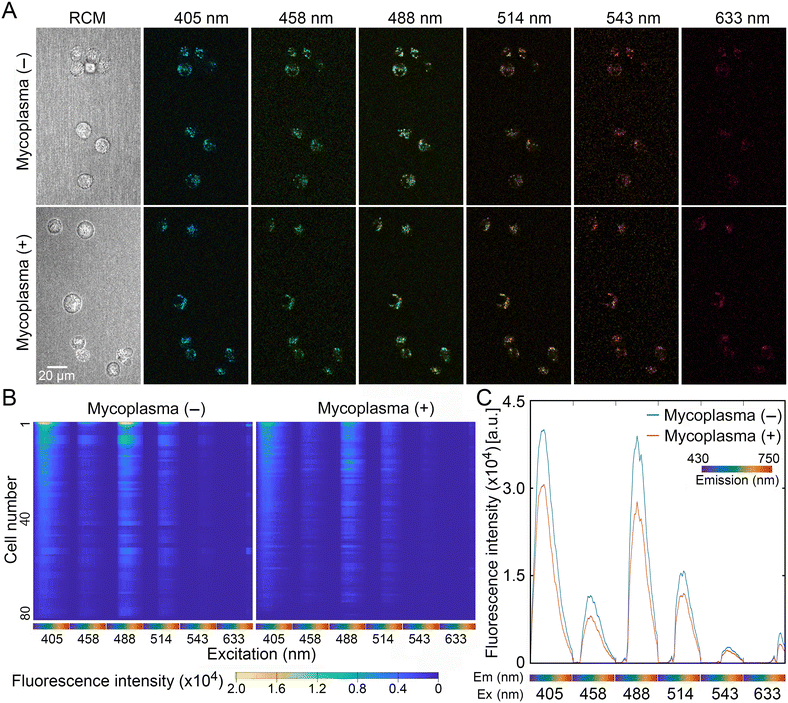 |
| Fig. 2 Autofluorescence signatures of unattached spherical mycoplasma (−) and mycoplasma (+) cells. (A) Representative images of mycoplasma (−) cells and mycoplasma (+) cells excited by 405, 458, 488, 514, 543, and 633 nm lasers. Images from reflection confocal microscopy (RCM) are also shown (left). (B) Heat maps showing hierarchical clustering of autofluorescence signatures of mycoplasma (−) and mycoplasma (+) cells. Autofluorescence signatures of individual 80 cells were aligned on the horizontal axis. (C) A graphical summary of the average autofluorescence signature from more than 80 cells. | |
Discrimination of mycoplasma-infected cells by machine learning models trained using the support vector machine (SVM) algorithm and neural network model
To discriminate mycoplasma (−) and mycoplasma (+) cells using differences in autofluorescence signatures, we used machine learning models trained with the support vector machine (SVM) algorithm and a neural network model (Fig. 3A). In general, the more samples are trained, the better models will be built. However, as a rule of thumb, it is suggested that the number of samples for classification should be at least 10 × n × c (n: input features, c: class) to avoid overfitting.21 Taking into account the sample size (ESI† Table S1), we employed a dimension reduction technique, principal component analysis (PCA), to reduce the features from 192 to 10 prior to training our models. This reduction in features allowed us to train our models effectively without overfitting. The supervisor data consisted of 70% of randomly selected single-cell innate fluorescence signatures. After training, the validation accuracy of binary classification from the SVM-supported model approached 55.6%. In contrast, the neural network-assisted model achieved an accuracy of 70.2%. Confusion matrices of ten-fold validation analyses using these models, shown in Fig. 3B and ESI† Fig. S2, clearly indicate that these models successfully discriminated mycoplasma (−) and mycoplasma (+) cells using their autofluorescence signatures. The neural network-assisted model tended to provide higher accuracy than the SVM-supported model.
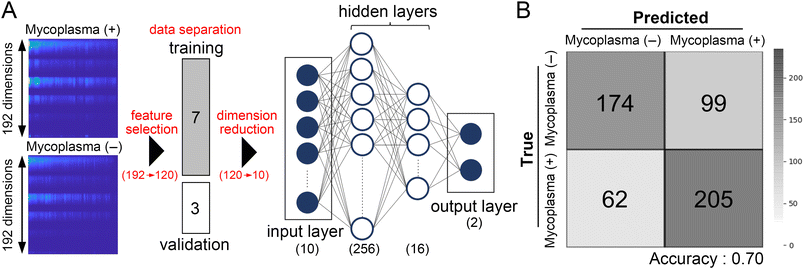 |
| Fig. 3 Discrimination of mycoplasma infection by a neural network-supported machine learning model using individual autofluorescence signatures. (A) A schematic for the machine learning model supported by a simple fully connected neural network model. Prior to training our model, features below the excitation wavelength were excluded (192 to 120), followed by the dimension reduction using PCA (120 to 10). The model includes the input layer (10 dimension), two hidden layers, and the output layer, in which the input and output layers connect directly to 256 nodes and 16 nodes, respectively. The output layer for 8-class classification consists of 8 nodes (see ESI† Fig. S4). (B) The neural network-supported machine learning model performed binary classification of mycoplasma infection with ∼70% accuracy. | |
Imaging and classification of adhered mycoplasma (−) and mycoplasma (+) cells
We next examined whether imaging adhered cells allowed us to distinguish mycoplasma (−) and mycoplasma (+) cells. Cells were independently inoculated into slide dishes and imaged daily for three days. Fig. 4A shows representative images of autofluorescence and RCM of mycoplasma (−) and mycoplasma (+) cells in the same horizontal plane at day 1. The averages of extracted autofluorescence signatures from total cells [mycoplasma (−): 16; mycoplasma (+): 29] revealed differences between cell types when excited with 405, 458, 488, and 514 nm lasers at day 2 (Fig. 4B). However, the differences between peaks from adhered mycoplasma (−) and mycoplasma (+) cells at 488 and 514 nm lasers were smaller than the differences derived from unattached spherical cells (see Fig. 2C). We inferred that the loss of these differences was presumably due to the larger area of adhered cells. Based on these results, we concluded that autofluorescence signals, especially from short-wavelength (405 and 458 nm) excitation, are attenuated by mycoplasma infection.
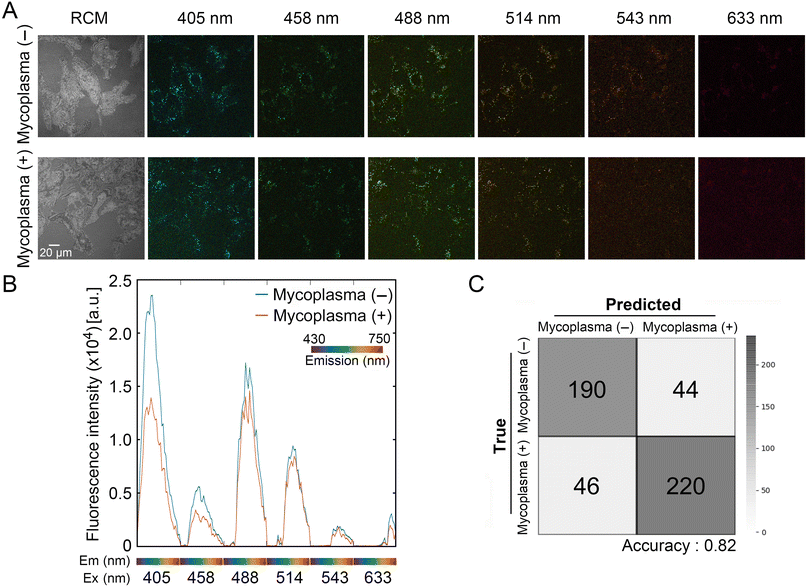 |
| Fig. 4 Autofluorescence signatures of adhered mycoplasma (−) and mycoplasma (+) cells and classification of mycoplasma-infected cells by our machine learning model. (A) Representative images of mycoplasma (−) and mycoplasma (+) cells excited by 405, 458, 488, 514, 543, and 633 nm lasers on day 1. Images of reflection confocal microscopy (RCM) are also shown (left). (B) Graphical summary of the average autofluorescence signatures acquired at day 2. (C) Our neural network-supported machine learning model achieved an accuracy of ∼82% in classifying cells. | |
Using randomly selected fluorescence signatures from all days (0–3 days), we trained SVM- and neural network-assisted models to classify mycoplasma (−) and mycoplasma (+) cells. The accuracy of binary classification from these models approached 77.2% and 82.0%, respectively (Fig. 4C and ESI† Fig. S3). Because the autofluorescence signature of these cells was found to exhibit slight daily variation depending on the stage of culture, we further trained the models with an eight-class dataset (4 days × 2 cells) to examine whether the discrimination ability of our model was stage-agnostic. If this is the case, the autofluorescence signatures acquired at any culture duration could be utilized for classification. As a result, the neural network-assisted model exhibited high validation accuracy (76.5%) in the discrimination task (ESI† Fig. S4). Although the dataset for training our models was limited, these preliminary results implicated that the autofluorescence signature is useful for the identification of mycoplasma-infected VERO cells, independent of culture stage.
Reduction of cellular NADH by mycoplasma infection
Mycoplasma NADH oxidase is reported to be a potential mediator of virulence,22–24 implying that mycoplasma infection causes an increase in the NADH oxidase activity in host cells. NADH is a major cellular autofluorescence molecule with excitation and emission peaks of 330–360 nm and 440–470 nm, respectively.4,25 However, its oxidized form, NAD+, does not exhibit autofluorescence. Although the shortest excitation wavelength used in the present study was 405 nm, we suspected that the attenuated autofluorescence signals observed under 405 nm excitation were driven by changes in cellular NADH levels caused by mycoplasma infection. We therefore measured cellular NADH quantitatively in mycoplasma (−) and mycoplasma (+) cells (ESI† Fig. S5). As expected, NADH levels were reduced by ∼20% by mycoplasma infection, indicating that decreased NADH levels caused by mycoplasma infection could underlie the attenuated autofluorescence signals, especially under 405 nm excitation. However, it cannot be ruled out that the decrease in other fluorescent molecules may also contribute to the attenuation.
Experimental
Strain, cells, and culturing conditions
In this study, VERO (JCRB0111) cells derived from African green monkey kidney tissue, a known indicator cell line for mycoplasma infection, were obtained from the JCRB cell bank. VERO cells were cultured in Eagle's minimum essential medium (EMEM; Thermo Fisher Scientific, Waltham, MA, USA) supplemented with 5% fetal bovine serum (FBS; BioWest, Nuaillé, France). Prior to this study, VERO cells were cryopreserved and stored in Bambanker's freezing medium (GC Lymphotec, Tokyo, Japan).
Mycoplasmopsis fermentans (NBRC 14854) was obtained from the National Institute of Technology and Evaluation (NITE), suspended in 5 mL of mycoplasma negative test medium I (Nissui Pharmaceuticals, Tokyo, Japan) and grown under 37 °C with 5% CO2 supplied.
Mycoplasma infection
To induce mycoplasma infection, 0.5 mL supernatant of 7-day M. fermentans cell culture was added to 5 mL of VERO culture (at ∼70% confluency) and incubated for an additional ∼7 days.
Staining VERO with Hoechst 33258
To visualize mycoplasma infection, mycoplasma (−) and mycoplasma (+) cells were inoculated in Lab-Tek Chamber Slide (Thermo Fisher Scientific) at a density of 1.0 × 105 cells per mL. After a 5-day incubation, cells adhered to coverslips were washed twice in pH 7.4 phosphate-buffered saline (PBS, Thermo Fisher Scientific) and lightly fixed for 15 min in PBS containing 25% ethanol and 8.3% acetic acid, followed by strong fixing in ethanol and acetic acid (3
:
1 ratio) for an additional 5 min. Fixed cells were washed three times in PBS and DNA was stained using Hoechst 33258 (0.5 μg mL−1, Dojindo, Kumamoto, Japan) for 10 min. Subsequently, cells were washed with distilled water, air-dried for approximately 20 min, and imaged using a BZ-X810 microscope with a 64× lens (Keyence, Osaka, Japan).
Detection of mycoplasma infection of host cells by isothermal PCR
To detect mycoplasma infection of VERO cells, a commercially available isothermal PCR-based kit (MycoStrip, InvivoGen, San Diego, CA, USA) was used to detect the mycoplasma 16S rRNA gene from 1 mL culture supernatant. The kit was used according to the manufacturer's instructions.
Acquisition of cellular autofluorescence signatures
To directly observe cultured cells using inverted microscopy, VERO cells cultured in T-25 flasks (Greiner Bio-One Co., Tokyo, Japan) were stripped with 0.25% trypsin–EDTA (0.18 g L−1 EDTA, Nacalai Tesque, Kyoto Japan), washed once in culture medium to remove most of trypsin, and then suspended in culture medium. After counting the cell number in the cell suspension, cells were seeded into μ-Slide VI 0.4 (Ibidi, Gräfelfing, Germany) with thin polymer coverslips at the culture bottom. Note that trypsin is known as a fluorescent protein26 and may interfere with cellular autofluorescence signals. However, each seeded culture could be contaminated with almost the equal amount of trypsin even after the washing step, we speculated that the effect of trypsin on autofluorescence signals in mycoplasma (−) and mycoplasma (+) cells was negligible. After incubation at 37 °C in an incubator supplied with 5% CO2, cellular autofluorescence was imaged using an Axio Observer Z1 microscope with a C-Apochromat 40× water immersion objective lens (421767-9973-000, Carl Zeiss, Oberkochen, Germany). We used the lambda mode of Zen software (Zeiss, Germany). Briefly, in this mode, a lambda stack of 10 nm length steps was obtained using a 32-channel spectral detector in the emission range of 430–750 nm and then the constructed stack was merged to display (see Fig. 2A and 4A).
Reconstruction of single-cell fluorescence signatures
Autofluorescence signatures of individual cells were reconstructed using custom MATLAB code as previously described.1,13 First, VERO cell images obtained by confocal reflection microscopy were processed to identify individual cells. Briefly, the segmentation processes including contrast adjustment, smoothing, edge detection, and edge gap filling were performed to recognize individual cellular regions using a MATLAB software. Then mean signal intensity for each excitation and emission wavelength was calculated for each cell by subtracting background autofluorescence at the cell-free area in each image. Thus, the autofluorescence signature of each cell was recorded as a 192-dimensional vector.
Classification using machine learning models
To confirm whether autofluorescence signatures can be used to discriminate mycoplasma (−) and mycoplasma (+) cells, we employed two machine learning methods: the support vector machine (SVM) algorithm and a neural network model. The SVM used in this study was implemented using the Scikit-learn library,27 whereas the neutral network model was built using the Keras library28 and TensorFlow backend.29 To avoid overfitting, we employed PCA to reduce the features from 192 to 10 before training our model. While, to improve accuracy, the grid search method was used to optimize the hyper-parameter of the neural network model, including the number of hidden layers, neuron (node) size, and batch size. Before training, we randomly divided the autofluorescence signature data into two data sets (70% for training and 30% for validation). The training process and validation of classification performance was executed using the hold-out method. We replicated this routine ten times to evaluate the generalization performance and then calculated the average accuracy of the classification models for each validation data set. The confusion matrix represented the sum of 10 validation results.
NADH measurement
The NAD/NADH assay kit-WST (Dojindo) was used according to the manufacturer's instructions to quantitatively measure cellular NADH levels. Briefly, 10 × 105 mycoplasma (−) and mycoplasma (+) cells were harvested and washed once with PBS. The cell pellets were then suspended in 300 μL of NAD/NADH extraction buffer for cell lysis, and the resulting suspension was passed through a 10 K MWCO filter to remove cell debris. The supernatant was heated at 60 °C for 1 hour for thermal degradation of NAD, mixed with an equal volume of the working solution containing an electron mediator and water-soluble tetrazolium salt (WST), and incubated at 37 °C for additional 1 hour. Colorimetric change due to the reduction of WST by NADH to formazan was measured at 450 nm using a multimode microplate reader (SKAEK 10 M, TEKAN, Männedorf, Switzerland).
Statistics
Statistical analyses were carried out using unpaired Welch's t-tests.
Conclusions
Here, we demonstrated that mycoplasma infection caused changes in cellular autofluorescence of host cells, allowing us to discriminate healthy and infected cells using machine learning models. In addition, we showed that a decrease in cellular NADH level is one of causes for the attenuation of autofluorescence signals under 405 nm excitation, implicating that mycoplasma infection altered cellular signaling and metabolism pathways. The abundant metabolite NADH and its oxidated form NAD+ play essential roles in the maintenance of cellular homeostasis; consistent with the pathological changes caused by mycoplasma infection, the dysregulation of these metabolites is also often associated with defective immune responses and cancer.30 NADH oxidase activity is well-known to be an important virulence factor for bacteria other than mycoplasmas, including Streptococcus species31,32 Accordingly, 405 nm autofluorescence signals may represent a minimally invasive and non-destructive tool for the identification of a wide range of pathogenic infections.
Abbreviations
NADH | Nicotinamide adenine dinucleotide |
FAD | Flavin adenine dinucleotide |
NF-κB | Nuclear factor kappa-light-chain-enhancer of activated B cells |
Nrf2 | Nuclear factor E2-related anti-inflammatory transcription factor 2 |
PBS | Phosphate-buffered saline |
SVM | Support vector machine |
Author contributions
KB, KT, AF, NN, AK, and TK conceived and designed the presented research. KB, KT, HD, and TK wrote and revised the manuscript. KB, YT, AO, MO, and HD cultured mycoplasma and VERO cells and performed mycoplasma infection of VERO cells. KB, KT, and TK acquired autofluorescence images of VERO cells. KT designed the computational models and analyzed the autofluorescence data.
Conflicts of interest
The authors declare no competing interests.
Acknowledgements
We thank Prof. Yutaka Yawata and Dr. Tatsuya Yamamoto for technical supports and helpful comments. NN was financially supported by the Japan Science and Technology Agency JPMJMI21G8. This work was also supported by JSPS KAKENHI Grant Numbers 20K15427 and 23H03542 to KT and TK, respectively. We acknowledge scholarship donations to NN and TK from Bridgestone Corporation.
References
- Y. Yawata, T. Kiyokawa, Y. Kawamura, T. Hirayama, K. Takabe and N. Nomura, Appl. Environ. Microbiol., 2019, 18, e00608–e00619, DOI:10.1128/AEM.00608-19.
- M. S. Islam, M. Honma, T. Nakabayashi, M. Kinjo and N. Ohta, Int. J. Mol. Sci., 2013, 14, 1952–1963, DOI:10.3390/ijms14011952.
- C. Niyangoda, T. Miti, L. Breydo, V. Uversky and M. Muschol, PLoS One, 2017, 12, e0176983, DOI:10.1371/journal.pone.0176983.
- P. M. Schaefer, S. Kalinina, A. Rueck, C. A. F. von Arnim and B. von Einem, Cytometry, Part A, 2019, 1, 34–46, DOI:10.1002/cyto.a.23597.
- V. Prabhu, S. B. S. Rao, E. M. Fernandes, A. C. K. Rao, K. Prasad and K. K. Mahato, PLoS One, 2014, 9, e98609, DOI:10.1371/journal.pone.0098609.
- H. L. Zhao, C. P. Zhang, H. Zhu, Y. F. Jiang and X. B. Fu, Sking Res. Technol., 2017, 23, 588–592, DOI:10.1111/srt.12375.
- L. Shi, L. Lu, G. Harvey, T. Harvey, A. Rodríguez-Contreras and R. R. Alfano, Sci. Rep., 2017, 7, 2599, DOI:10.1038/s41598-017-02673-5.
- S. P. Schantz, H. E. Savage, P. Sacks and R. R. Alfano, Environ. Health Perspect., 1997, 105(Suppl 4), 941–944, DOI:10.1289/ehp.97105s4941.
- J. Miyazaki and Y. Toumon, Biomed. Opt. Express, 2019, 10, 5852–5861, DOI:10.1364/BOE.10.005852.
- Y. Yuan, Z. X. Yan, J. Y. Miao, R. L. Cai, M. S. Zhang, Y. X. Wang, L. H. Wang, W. Q. Dang, D. Wang, D. F. Xiang, Y. Wang, P. Zhang, Y. H. Cui, X. W. Bian and Q. H. Ma, Stem Cell Res. Ther., 2019, 10, 330, DOI:10.1186/s13287-019-1467-7.
- S. Lemire, O. M. Thoma, L. Kreiss, S. Voelkl, O. Friedrich, M. F. Neurath, S. Schuermann and M. J. Waldner, Int. J. Mol. Sci., 2022, 23, 2338, DOI:10.3390/ijms23042338.
- L. A. Sordillo, P. P. Sordillo, Y. Budansky, Y. Pu and R. R. Alfano, J. Biomed. Opt., 2014, 19, 125002, DOI:10.1117/1.JBO.19.12.125002.
- T. Hirayama, K. Takabe, T. Kiyokawa, N. Nomura and Y. Yawata, J. Visualized Exp., 2020, 159, DOI:10.3791/61120.
- S. Rottem, Physiol. Rev., 2003, 83, 417–432, DOI:10.1152/physrev.00030.2002.
- S. N. Borchsenius, I. E. Vishnyakov, O. A. Chernova, V. M. Chernov and N. A. Barlev, Pathogens, 2020, 9, 308, DOI:10.3390/pathogens9040308.
- H. G. Drexler and C. C. Uphoff, Cytotechnology, 2002, 39, 75–90, DOI:10.1023/A:1022913015916.
- L. Young, J. Sung, G. Stacey and J. R. Masters, Nat. Protoc., 2010, 5, 929–934, DOI:10.1038/nprot.2010.43.
- S. Tsai, D. J. Wear, J. W. Shih and S. C. Lo, Proc. Natl. Acad. Sci. U. S. A., 1995, 92, 10197–10201, DOI:10.1073/pnas.92.22.10197.
- S. Huang, J. Y. Li, J. Wu, L. Meng and C. C. Shou, World J. Gastroenterol., 2001, 7, 266–269, DOI:10.3748/wjg.v7.i2.266.
- S. N. Borchsenius, A. Daks, O. Fedorova, O. Chernova and N. A. Barlev, J. Cell. Physiol., 2018, 234, 171–180, DOI:10.1002/jcp.26781.
-
V. Lakshmanan, S. Robinson and M. Munn, Machine Learning Design Patterns, O' Reilly Media Inc., 2020 Search PubMed.
- F. Hao, X. Xie, Z. X. Feng, R. Chen, Y. N. Wei, J. Liu, Q. Y. Xiong, G. Q. Shao and J. Lin, BMC Vet. Res., 2022, 18, 126, DOI:10.1186/s12917-022-03230-7.
- G. Zhao, H. Zhang, X. Chen, X. F. Zhu, Y. S. Guo, C. F. He, F. A. Khan, Y. Y. Chen, C. M. Hu, H. C. Chen and A. Z. Guo, Sci. Rep., 2017, 7, 44, DOI:10.1038/s41598-017-00121-y.
- Z. J. Hu, H. R. Li, Y. X. Zhao, G. J. Wang, Y. B. Shang, Y. T. Chen, S. H. Wang, M. X. Tian, J. J. Qi and S. Q. Yu, BMC Vet. Res., 2022, 18, 455, DOI:10.1186/s12917-022-03556-2.
- I. Kolenc and K. P. Quinn, Antioxid. Redox Signaling, 2019, 30, 875–889, DOI:10.1089/ars.2017.7451.
- Z. Wu, F. Huang, Y. Chen, H. Xu, M. D. Meti, Y. Fan, Q. G. Han, H. Tang, Z. He and Z. Hu, Int. J. Food Prop., 2018, 21, 316–327, DOI:10.1080/10942912.2018.1454944.
- F. Pedregosa, G. Varoquaux, A. Gramfort, V. Michel, B. Thirion, O. Grisel, M. Blondel, P. Prettenhofer, R. Weiss, V. Dubourg, J. Vanderplas, A. Passos, D. Cournapeau, M. Brucher and M. Perrot, Scikit-learn: Machine Learning in Python, J. Mach. Learn. Res., 2011, 12, 2825–2830, DOI:10.48550/arXiv.1201.0490.
-
F. Chollet, Keras, GitHub, 2015, Retrieved from https://github.com/fchollet/keras Search PubMed.
-
M. Abadi, P. Barham, J. Chen, Z. Chen, A. Davis, J. Dean, M. Devin, S. Ghemawat, G. Irving, M. Isard, M. Kudlur, J. Levenberg, R. Monga, S. Moore, D. G. Murray, B. Steiner, P. Tucker, V. Vasudevan, P. Warden, M. Wicke, Y. Yu and X. Zheng, TensorFlow: A system for large-scale machine learning, 12th USENIX Symposium on Operating Systems Design and Implementation, arXiv, 2016, preprint, DOI:10.48550/arXiv.1605.08695.
- L. E. Navas and A. Carnero, Target Ther., 2021, 6, 2, DOI:10.1038/s41392-020-00354-w.
- I. Auzat, S. Chapuy-Regaud, G. Le Bras, D. Dos Santos, A. D. Ogunniyi, I. Le Thomas, J. R. Garel, J. C. Paton and M. C. Trombe, Mol. Microbiol., 1999, 34, 1018–1028, DOI:10.1046/j.1365-2958.1999.01663.x.
- Y. Akeda, Virulence, 2017, 8, 11–12, DOI:10.1080/21505594.2016.1218593.
Footnotes |
† Electronic supplementary information (ESI) available. See DOI: https://doi.org/10.1039/d3sd00175j |
‡ Equal contribution to this study. |
§ Present address: Department of Bacteriology I, National Institute of Infectious Diseases, Toyama, Shinjuku-ku, Tokyo, 162-8640, Japan. |
¶ Present address: Bioproduction Research Institute, National Institute of Advanced Industrial Science and Technology, Higashi, Tsukuba 305-8566, Japan. |
|
This journal is © The Royal Society of Chemistry 2024 |
Click here to see how this site uses Cookies. View our privacy policy here.