Quantum DFT analysis and molecular docking investigation of various potential breast cancer drugs
Received
11th August 2024
, Accepted 6th November 2024
First published on 9th November 2024
Abstract
Breast cancer is among the deadliest cancers worldwide, highlighting the urgent need for effective treatments. This study employs density functional theory (DFT) and molecular docking analyses to evaluate the anti-cancer efficacy and specificity of drug molecules lapatinib, tucatinib, neratinib, anastrozole, and letrozole. DFT analysis provides comprehensive insights into the structural, electronic, optical, and vibrational properties of these drugs, helping to elucidate their molecular stability and reactivity through global reactivity descriptors. Additionally, molecular docking simulations reveal the binding conformations and interaction profiles of these drugs with key breast cancer targets, underscoring their therapeutic potential. Docking results indicate that lapatinib, tucatinib, and neratinib have high binding affinities for HER2, with lapatinib exhibiting the strongest overall binding, particularly with PDK1 (PDB ID: 1UU7), PAK4 (PDB ID: 2X4Z), GSK3 (PDB ID: 1GNG), and HER2 (PDB ID: 2IOK). The stable hydrogen bonding and other interactions observed with lapatinib support its effectiveness in treating HER2-positive breast cancers, tucatinib's selective HER2 binding reduces off-target effects, while neratinib's irreversible binding provides prolonged inhibition, making it useful for overcoming resistance in HER2-positive cases. In contrast, anastrozole and letrozole show lower binding affinities for HER2 and EGFR due to their simpler structures but are potent aromatase inhibitors, making them effective in treating estrogen receptor-positive (ER-positive) breast cancers. In conclusion, DFT and molecular docking studies affirm the suitability of lapatinib, tucatinib, and neratinib for HER2-positive cancers, while anastrozole and letrozole are effective in ER-positive cancers, emphasizing the role of molecular structure and binding affinity in optimizing cancer treatment strategies.
Introduction
Breast cancer has a long and storied past. Over 3500 years ago, the sickness was originally reported by the ancient Egyptians.1,2 When compared to males, women experienced breast cancer (BC) symptoms in a unique way.3 Many risk factors related to biomolecular dynamics contribute to the under-recognized nature of breast carcinogenesis. Breast cancer (BC) is a prevalent malignancy that impacts women globally.4 Cancer is produced by genetic damage in cells, which results in abnormal division and mutation. Breast cancer is a specific type of cancer that is influenced by hormones. The breast's glandular tissues are vulnerable to fluctuations in the body's hormone levels.5 Based on the genetic and histologic evidence, three types of breast cancer (BC) may be distinguished: those that express human epidermal receptor 2 (HER2+), those that express hormone receptor (PR+ or ER+), and triple-negative breast cancer (TNBC) (ER−, PR−, HER2−).6,7 The pathways leading to breast cancer are still unclear.8 The most common kind of BC is HR-expressing BC, which accounts for 60–70% of occurrences in affluent nations and is solely found in premenopausal women. Consequently, hormonotherapy is the most prevalent therapeutic method.9 There is some evidence that these sex hormones play a role in the emergence of breast cancer risk factors.10 In contrast, pregnancy or breastfeeding lowers the risk of developing cancer. A higher risk of developing breast cancer is associated with a greater breast mass, according to the research.11
Breast cancer treatment targets many receptors, including estrogen receptor alpha (Erα), progesterone receptor (PR), and epidermal growth factor receptor (EGFR). Studies show that estrogen, namely 17 β-estradiol promotes cell cycle progression from G1 to the S phase in mammary gland epithelial cells by upregulating c-Myc and cyclin D1 expression and function. As the first targeted treatment for human breast cancer, anti-estrogen therapy shows great promise in the treatment of ER-positive breast cancer.12 An active progesterone receptor (PR) pathway is associated with an overactive estrogen receptor (ER) in breast cancer since PR is generated in response to estrogenic stimulation in target tissues. Breast cancer patients with PR-positive tumours have a better chance of responding to hormone treatment if their PR and ER genes are overexpressed.13 Before the development of anti-HER2 medications, aggressive behaviour, treatment resistance, and a poor prognosis were all associated with the overexpression of human epidermal growth factor receptor-2 (HER2), which is present in around 20–30% of BC patients.14–17 Overexpression of HER2 in breast cancer often leads to involvement of the central nervous system (CNS).18–21 Breast cancer brain metastasis (BCBM) is a leading cause of morbidity and mortality among metastatic breast cancer (MBC) patients.22,23 Three main obstacles to effective drug delivery in the central nervous system are the blood–brain barrier (BBB), the blood-tumour barrier (BTB), and the blood–cerebrospinal fluid barrier (CSF).24
The monoclonal antibody trastuzumab has greatly enhanced the prognosis for patients with both early and advanced HER2-positive breast cancer.16,25–27 Trastuzumab, a macromolecule, cannot cross the blood–brain barrier. Tyrosine kinase inhibitors (TKIs) are usually small compounds that can enter the central nervous system (CNS). Over the last decade, various drugs, notably tyrosine kinase inhibitors (TKIs) and therapeutic approaches have been developed to address trastuzumab resistance.28,29 Due to their varied targeting choices and limited toxicity, small-molecule TKIs may be a better choice than systemic chemotherapy and monoclonal antibodies for treating CNS metastases. The second or third line of treatment usually involves the use of small-molecule pan-HER-targeted medications. lapatinib is a well-known HER2 inhibitor that has been extensively studied and widely used in clinical settings for the treatment of HER2-positive breast cancer. It works by inhibiting the tyrosine kinase activity associated with both the HER2 and EGFR pathways, effectively blocking downstream signaling processes that promote tumour growth and survival.30 It blocks downstream pathways and suppresses tumour growth by reversibly binding to the ATP-binding site in the tyrosine kinase region of HER1 (EGFR) and HER2 cells,31,32 In 2007, the US Food and Drug Administration authorized lapatinib plus letrozole as the initial line of treatment for HR-positive HER2-overexpressing breast cancer (MBC) in postmenopausal women.33
In 2017, FDA, USA approved neratinib, an oral small-molecule pan-human TKI.34–37 Neratinib binds to EGFRs HER1, HER2, and HER4 in an irreversible manner.37–39 In patients with HER2-positive, hormone receptor (HR)-negative breast cancer, the I-SPY2 neoadjuvant trial discovered that the pathologic complete response (pCR) rate was improved when neratinib was administered in combination with trastuzumab.40,41 In April 2020, the FDA authorized tucatinib, an oral, potent, and exclusive HER2-specific targeted medicine, for the treatment of metastatic or advanced, inoperable breast cancer. Both in preclinical and phase I clinical trials, trastuzumab demonstrated promise as a monotherapy and improved effectiveness when combined with trastuzumab or chemotherapy.42–45 Unlike other TKIs, such as lapatinib and neratinib, which operate as dual inhibitors for both EGFR and HER2, tucatinib selectively and reversibly inhibits the protein tyrosine kinase (PTK) activity of HER2. It also shows minimal inhibition of EGFR.45–47 EGFR has various cellular functions but aberration of the receptor results in tumour development. About half of breast cancer cases overexpress EGFR, making it an appropriate target for the discovery of molecular-based targeted therapeutics.48 Letrozole binds to the estrogen production pathway's rate-limiting enzyme P450 aromatase; it is a non-steroidal, highly selective oral aromatase inhibitor (AI). It prevents androstenedione and testosterone from converting to estradiol and estrone, respectively.49 The pituitary gland secretes more follicle-stimulating hormone (FSH), which triggers ovulation, when estrogen levels are downregulated. Letrozole is a popular choice for ovulating females to increase their follicle count or for an ovulatory infertile patient who is unable to trigger an egg release. Intrauterine insemination is enhanced with letrozole as well.50 Anastrozole, exemestane, and letrozole are the aromatase inhibitors (AIs) that are initially prescribed to postmenopausal women who have estrogen-dependent breast cancer.51–54 As adjuvant treatment for early-stage breast cancer, anastrozole, exemestane, and letrozole are considered interchangeable and are similarly effective. Two large phase III clinical trials provided the data necessary to support this claim. Anastrozole and exemestane were the two treatments given to 7576 postmenopausal women in the MA.27 study. All three measures of survival-event-free, distant disease-free, and disease-specific-had the same results.54
The computational quantum mechanical method known as density-functional theory (DFT) is applied in the field of pharmaceutical chemistry to provide accurate predictions about the ground state energies of electronic three-dimensional atoms as well as molecules.55 When compared to other current ab initio methods, DFT computations outperform them in terms of accuracy, speed, and cost.56 Although DFT is typically accurate, it is quite expensive to calculate. To create machine learning (ML) potentials, DFT has lately been integrated with ML methods, especially graph neural networks. For big systems in particular, these graph neural networks provide a computationally far more inexpensive alternative to DFT that is just as accurate. They learn from a pre-existing dataset of molecules whose DFT-calculated properties are well-known. Researchers have been trying to use machine learning to estimate DFT for a while now, but only recently have they found decent estimators.57,58
At that time, numerous studies described the chemical route and chemical properties of these anticancer drugs, but very few theoretical studies examined their physicochemical properties, such as electronic band gap, MEP, reactivity of drugs, total energy and global descriptors such as chemical potential (μ), hardness (η), electrophilic index (ω), and softness (S) and chemical potential. In this study, we employed DFT calculations to theoretically examine the electronic properties. Molecular docking analysis was employed to analyze the ligand interactions with the target protein and to study the binding affinity of protein–ligand interactions. This may provide reliable data for further in vitro and in vivo studies with a holy-grail of developing therapeutics for breast cancer.
Material and methods
The Gaussian 16 software59 is used to perform DFT calculations for breast cancer drugs, namely anastrozole, letrozole, lapatinib, neratinib, and tucatinib. These five compounds' geometric optimization was carried out at the DFT level using a hybrid functional B3LYP (Becke's three-parameter hybrid functional employing the LYP correlation functional) 6–311G (d,p).60 The optimized molecular structure and MEP surface were visualized using the visualizer tool GaussView 06.61 We examined the electronic characteristics of these molecules, including their electronic band gap (Egap), total energy (ET), dipole moment (a), and the highest occupied and lowest unoccupied molecular orbitals (EHOMO and ELUMO, respectively). Based on these relationships, we were able to determine the electronic band gap.62Eqn (3) and (4) may be used to represent the ionization energy (I) and electron affinity (A) in terms of the HOMO and LUMO orbital energies following Koopmans' theorem.The study also incorporates the computation of theoretical physicochemical characteristics such as energy gap (ΔE), chemical softness (S), chemical hardness (η), electronegativity (χ), electrophilicity index (ω), and chemical potential (μ). Quantum chemical descriptors suggested by Parr and colleagues are widely used by computational chemists for predicting a molecule's chemical behaviour.63,64 These descriptors can be calculated using equations: | 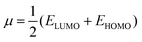 | (4) |
| 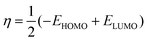 | (5) |
|  | (6) |
| χ = −(EHOMO + ELUMO)/2 | (7) |
|  | (8) |
Molecular docking simulation
Target proteins were sourced from the RCSB online Protein Data Bank (PDB) website at https://www.rcsb.org/. Eight breast cancer-associated proteins—HER-2, BCL2, EGFR, ERα, PR, PAK4, GSK3, and PDK1 (PDB IDs: 2IOK, 4AQ3, 2J6M, 6WOK, 4OAR, 2X4Z, 1GNG, and 1UU7) – were retrieved. The active site regions of these proteins were then analyzed using the AutoDock VINA tool (https://vina.scripps.edu/). Each protein was processed in BIOVIA Discovery Studio 202165 by removing ligands, hetatms, and water molecules, then saved in “.pdb” format. A grid box was generated around the core of each protein, and docking simulations were performed. The resulting docking scores were analyzed and saved as a “.csv” file.
Analysis of protein–ligand interaction
Molecular docking output file was used to generate visual representations of protein–ligand interactions. BIOVIA Discovery Studio 2021 was employed to display these interactions, enabling analysis of bond types, bond distances, and the amino acid residues involved. By loading both the protein and ligand into the software's graphical interface, the interactions were visualized, with ligands positioned within the protein's receptor region. The resulting 2D and 3D interaction visuals were then saved in ‘.png’ format.
Results and discussions
Energies and global descriptors
Five potential breast cancer drugs: anastrozole, letrozole, lapatinib, neratinib, and tucatinib were optimized utilizing the DFT method at B3LYP/6-311G++(d,p) level. The optimized structures are shown in Fig. 1. Frontier molecular orbital (FMO) analysis was used to assess how the molecule interacted with other species. The FMO consists of the HOMO, the highest occupied molecular orbital, and LUMO, the lowest unoccupied molecular orbital. HOMO refers to a nucleophile that donates electrons, whereas LUMO represents an electrophile that accepts.62
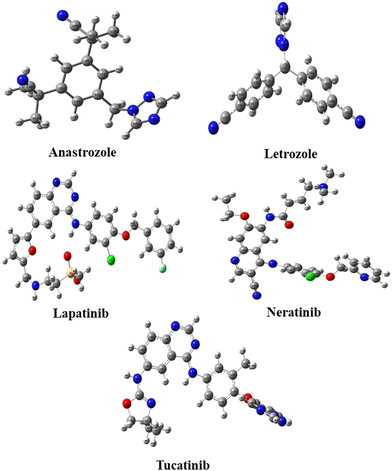 |
| Fig. 1 The geometrical optimization of the anticancer drugs considered in this study. | |
The different electronic characteristics of each of these molecules were then calculated using this method. Once all of these anticancer drugs were optimized, we concluded that this method is appropriate for providing all of the factors mentioned above because they are consistent with earlier theoretical and experimental research. This was followed by computing the electronic characteristics of each of these compounds. Initially, the total energy of each drug was calculated to examine the stability. Table 1 summarizes the calculated dipole moments (α), and frontier molecular orbital energies, all optimized for gaseous conditions. Fig. 2 illustrates the energies of the frontier molecular orbitals (HOMO and LUMO) and their corresponding three-dimensional plots for each anti-cancer drug molecule taken in the study. As shown in Table 1, lapatinib drug is more stable and less reactive than other molecules since its total energy value is larger.66 The dipole moment is another important characteristic that has been used to explain the structure and reactivity of numerous chemical systems. A significant component influencing a drug's solubility is its dipole moment. To maximize the drug's effectiveness, its polarity and solubility must be matched. For drug administration in biological systems, a high dipole moment value is a desirable characteristic.67 The dipole moments of the studied drug were around 3.9772–9.5206 Debye. Consequently, we found that in comparison to other molecules, neratinib has a larger dipole moment value. The drug with a large dipole moment allows for high polarity and hydrophilic interactions, leading to increased activity. The Koopmans' theorem states that the negative electron affinity (A = –ELUMO) and negative ionization potential (I = –EHOMO) can be represented by the HOMO and LUMO, respectively. As a result, we noticed that all compounds have a higher electron affinity and a lower ionization potential (Table 1). Then, these molecules needed to lower energy to donate/accept an electron to become a cation/anion. The energy difference between the HOMO and the LUMO is a key indicator of a molecule's chemical stability and reactivity. Previous computational studies on bioactive molecule glycine-tyrosine dipeptide have reported a HOMO–LUMO gap of 0.054 eV, calculated using the DFT/B3LYP method with the 6-31++G(d) basis set.68 In another study (Sefa et al.69), the energy gap of the caprolactam-glycin cluster was reported to be about 9.931 eV. Another computational study on bioactive molecule bidentate and tetradentate-type Schiff base has reported a HOMO–LUMO gap of 3.51 eV and 2.03 eV.70 V. Rani et al. performed the HOMO–LUMO study on bioactive compound 3,4-difluoroacetophenone thiosemicarbazone (DFAT) with band gap of 3.9726 eV.71 The HOMO–LUMO gap of the bioactive breast cancer drug biochanin-A has been reported to be 4.13 eV in previous computational investigations.72 The current study aims to investigate the quantum descriptors of the five anticancer drugs used in the study. A molecule that is most stable and more reactive has a smaller energy gap. Table 1 illustrates that lapatinib is the most reactive and stable drug due to its smaller ΔE values of 3.822 eV, and anastrozole is the least reactive molecule with the highest energy gap value of 6.113 eV. Hardness is correlated with the energy gap and is a useful indicator of chemical stability and reactivity. Band gap energies are high in hard compounds. Table 1 shows that anastrozole has the maximum hardness with a large band gap, whereas lapatinib has the minimum hardness with a lower band gap. The order of increasing molecular hardness was in order: anastrozole > letrozole > tucatinib > neratinib > lapatinib. Softness is the inverse of hardness and possesses a similar relation. A drug's electrophilicity index value corresponds to its capacity to take electrons. The electrophilic character of a molecule is indicated by an electrophilic index value greater than 1.5 eV.73 Each drug molecule has an electrophilicity index value greater than 1.5 eV, as illustrated in Table 1. It follows that each molecule under study has an electrophilic nature. Since all the drugs used in the study have the ability to accept electrons, this property may enhance their interaction with the target protein, resulting in better therapeutic potential of the studied drugs.
Table 1 The global chemical indexes of the four anticancer drugs; the ionization potential (I), electron affinity (A), electronic band gap (ΔE), chemical hardness (η), chemical softness (S), chemical potential (μ), electrophilicity index (ω) and dipole moment (α)
Global Chemical Indexes |
Anastrozole |
Letrozole |
Lapatinib |
Neratinib |
Tucatinib |
I (eV) |
7.540 |
7.667 |
5.535 |
5.938 |
5.856 |
A (eV) |
1.428 |
2.376 |
1.712 |
1.985 |
1.839 |
ΔE (eV) |
6.113 |
5.290 |
3.822 |
3.953 |
4.017 |
η (eV) |
3.056 |
2.645 |
1.911 |
1.977 |
2.009 |
S (eV) |
0.164 |
0.189 |
0.262 |
0.253 |
0.249 |
μ (eV) |
−4.484 |
−5.021 |
−3.623 |
−3.961 |
−3.848 |
χ (eV) |
4.484 |
5.021 |
3.623 |
3.961 |
3.848 |
ω (eV) |
3.289 |
4.767 |
3.435 |
3.970 |
3.686 |
α (Debye) |
5.205 |
3.977 |
7.394 |
9.521 |
6.513 |
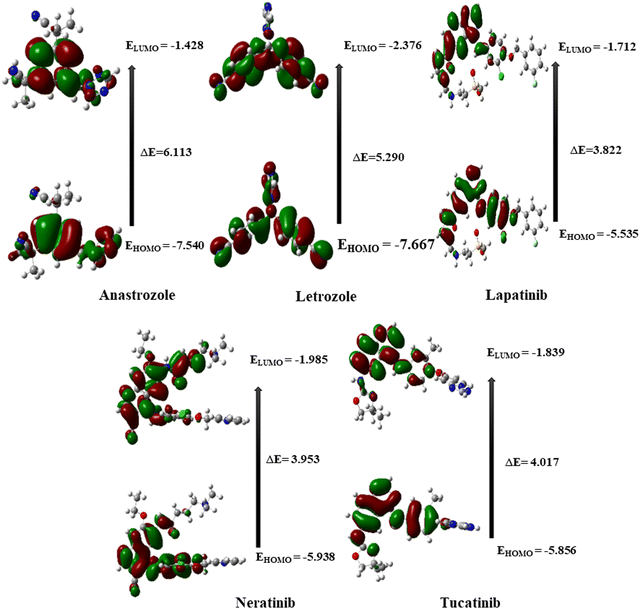 |
| Fig. 2 Frontier molecular orbitals and their energy gaps of studied anticancer drugs. | |
Molecular electrostatic potential (MEP)
MEP plots74 provide a 3D visualization of the charge distribution across atoms within a molecule. MEP helps in pinpointing electrophilic and nucleophilic regions within biomolecules and pharmaceutical compounds.75 MEP surfaces are color-coded: green for neutral, blue for highly positive (nucleophilic sites), yellow for slightly electron-rich, light blue for slightly electron-deficient, and red for highly negative (electrophilic sites). The MEP plots of the drug molecules are presented in Fig. 3.
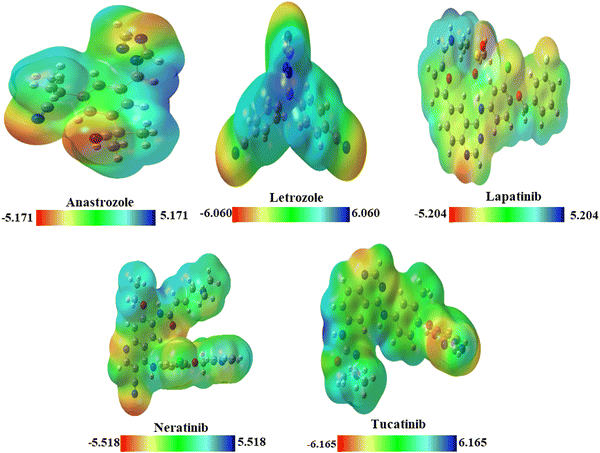 |
| Fig. 3 The molecular electrostatic potential maps of the studied anticancer drugs. | |
MEP surfaces of the drug molecule are shown in Fig. 3. For anastrozole and letrozole, high electron density near nitrogen atoms favors electrophilic attacks, while low electron density around hydrogen atoms favors nucleophilic attacks. In lapatinib, nitrogen atoms attached to benzene and oxygen are prime sites for electrophilic attacks, while hydrogen atoms, with minimal electron density, are susceptible to nucleophilic interactions. The yellow color near chlorine and sulfur indicates a slight electron-rich density. In neratinib, negative potential is located around oxygen and nitrogen atoms on the benzene ring, with positive potential on other atoms. Chlorine shows a slight electron-rich density and hydrogen atoms serve as nucleophilic sites. Tucatinib's high electron density near oxygen and nitrogen atoms favors electrophilic attacks, while low density near hydrogen atoms supports nucleophilic activity. These molecules exhibit both electrophilic and nucleophilic reactivity, indicating overall chemical reactivity.
Optical properties
Time-dependent DFT (TD-DFT) calculations were performed using the basis set B3LYP/6-311G(d,p) to determine the optical properties of the five anticancer drugs. The computed UV-visible absorption spectra of all five anticancer drugs are shown in Fig. 4. We determined the optical transition energies, absorption wavelengths, oscillator strengths, and types of electronic transitions for these anticancer drugs using the method outlined above. It was observed that all anticancer drugs exhibit light absorption in the UV region, with each drug displaying distinct optical absorption characteristics. These unique features can be leveraged in various biological and pharmaceutical applications.
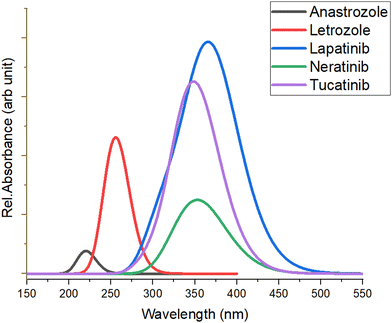 |
| Fig. 4 The UV-visible absorption spectra of the studied anticancer drugs computed at B3LYP/6-311G(d,p) level. | |
Table 2 presents the optical transition energies (Eopt), absorption wavelengths (λabs), oscillator strengths (f), and electronic transition descriptions derived from the calculated UV-visible absorption spectra of the four anticancer drugs using the TD-DFT method. The absorption maxima of all five drugs result from the convolution of adjacent transitions, primarily from the HOMO → LUMO transition, corresponding mainly to bonding (π) to anti-bonding (π*) orbitals. Notably, lapatinib shows the highest absorption at ∼369.4 nm, while anastrozole exhibits the lowest, with absorption maxima at ∼216.5 nm.
Table 2 Optical transition energies (Eopt), absorption wavelengths (λabs), oscillator strengths (f), and description of electronic transitions obtained from the calculated UV-visible absorption spectra of the four anticancer drugs using the TD-DFT method
Drug Molecules |
State |
E
opt (eV) |
λ
abs (nm) |
Oscillator strengths (f) |
Electronic transitions |
Transitions |
Contribution % |
Type of transition |
Anastrozole |
S1 |
5.13 |
241.84 |
0.0003 |
HOMO → LUMO |
48 |
π → π* |
S2 |
5.60 |
221.41 |
0.0375 |
HOMO−1 → LUMO |
24 |
HOMO → LUMO+1 |
27 |
HOMO−2 → LUMO |
58 |
S3 |
5.73 |
216.49 |
0.0110 |
HOMO−1 → LUMO |
61 |
Letrozole |
S1 |
4.80 |
258.15 |
0.2059 |
HOMO → LUMO |
61 |
π → π* |
S2 |
4.95 |
250.59 |
0.0140 |
HOMO → LUMO+3 |
25 |
HOMO−3 → LUMO |
33 |
HOMO−2 → LUMO |
23 |
S3 |
4.96 |
249.97 |
0.0749 |
HOMO−2 → LUMO |
30 |
HOMO → LUMO+2 |
27 |
HOMO → LUMO |
26 |
HOMO → LUMO+1 |
22 |
Lapatinib |
S1 |
3.36 |
369.39 |
0.4550 |
HOMO → LUMO |
70 |
π → π* |
S2 |
3.73 |
332.26 |
0.0422 |
HOMO → LUMO+1 |
63 |
S3 |
3.97 |
312.09 |
0.1172 |
HOMO−1 → LUMO |
55 |
HOMO → LUMO+1 |
22 |
Neratinib |
S1 |
3.41 |
363.12 |
0.0998 |
HOMO → LUMO |
69 |
π → π* |
S2 |
3.65 |
339.32 |
0.0628 |
HOMO → LUMO+1 |
61 |
S3 |
3.75 |
330.90 |
0.0076 |
HOMO−1 → LUMO |
58 |
HOMO−1 → LUMO+1 |
36 |
Tucatinib |
S1 |
3.55 |
349.46 |
0.3960 |
HOMO → LUMO |
69 |
π → π* |
S2 |
4.09 |
303.36 |
0.0380 |
HOMO → LUMO+1 |
56 |
S3 |
4.11 |
301.98 |
0.0056 |
HOMO−3 → LUMO |
55 |
Vibrational analysis
The calculated IR spectra of the five drugs, obtained using the B3LYP/6-311++G(d,p) level of theory, are shown in Fig. 5. Vibrational assignments were made based on Potential Energy Distributions (PED) using the vibrational energy distribution analysis (VEDA) program.76 The vibrational assignments of the prominent IR active vibrations are presented in Table 3. The vibrational modes of the peptide group, including C
O, C–N, C–H, N–H, O–H, and C–C, are crucial for analyzing the structural stability and bonding characteristics of peptides, especially in the context of hydrogen bonding. Therefore, this study focused on the vibrational bands related to atoms involved in hydrogen bonds. In particular, the N–H stretching vibration in peptides and proteins typically appears in the 3300–3500 cm−1 range in infrared spectroscopy, corresponding to the N–H bond in amide groups, which plays a key role in hydrogen bonding.77 The NH stretching vibrations in lapatinib, neratinib, and tucatinib are observed at ∼3576 cm−1, ∼3618 cm−1, and ∼3634 cm−1, respectively. The N–H in-plane bending vibration, along with C–N stretching (Amide II band), is typically observed in the range of 1500–1600 cm−1.78 The N–H in-plane bending vibration is observed at ∼1600 cm−1 in lapatinib, ∼1608 cm−1 in tucatinib drug, and a distinct peak at ∼1574 cm−1 in neratinib. The second intense peak in lapatinib appearing at ∼1536 cm−1 corresponds to C–H in-plane bending vibration. The C–H stretching vibrations in aromatic rings typically occur above 3000 cm−1, usually in the range of 3000–3100 cm−1. These vibrations often manifest as multiple weak to moderate bands, in contrast to the stronger C–H stretches of aliphatic chains, which are found below 3000 cm−1 (commonly around 2850–2950 cm−1).79
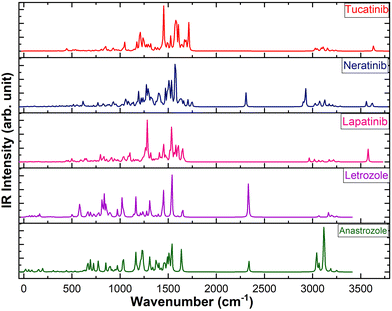 |
| Fig. 5 Computed IR spectra of all five anticancer drugs at B3LYP/6-311++G(d,p) level of theory. | |
Table 3 Calculated vibrational frequencies in cm−1 and the potential energy distribution (PED) calculated for studied five anticancer drugs
Wavenumber |
IR intensity |
Vibrational assignments (PED %) |
Wavenumber |
IR intensity |
Vibrational assignments (PED %) |
Here, ν = stretching, δ = bending, and τ = torsion. |
Anastrozole |
Letrozole |
3188 |
21 |
93 ν(C–H) |
3065 |
31 |
100 ν(C–H) |
3116 |
258 |
87 ν(C–H) |
2334 |
218 |
14 ν(C N) |
3068 |
52 |
31 ν(C–H) |
1651 |
45 |
17 ν(C C) |
3044 |
111 |
83 ν(C–H) |
1540 |
276 |
11 δ(HCC) |
2340 |
62 |
18 ν(C N) |
1538 |
39 |
12 ν(C N) |
1638 |
131 |
29 ν(C C)) |
1536 |
40 |
18 ν(C–N), 10 δ(HCN) |
1537 |
162 |
16 ν(C N) |
1451 |
176 |
20 ν(C–N), 27 τ(HCCC) |
1508 |
110 |
38 δ(HNC), 31 δ (HCH) |
1318 |
51 |
14 ν(C–C) |
1494 |
88 |
27 τ(HCCC) |
1307 |
30 |
13 δ(NCN), 26 δ(HCN), 21 δ(CNN) |
1465 |
54 |
14 τ(HCCC) |
1276 |
40 |
16 ν(C–C), 20 δ(HCC) |
1403 |
52 |
29 τ(HCCC) |
1239 |
37 |
20 ν(N–N), 14 ν(C–N) |
1375 |
67 |
33 δ(HCH), 18 δ (HCH) |
1214 |
29 |
12 ν(C–N), 21 δ(HCN) |
1338 |
28 |
12 ν(C–C), 20 δ (HCC), 31 δ (HCN) |
1164 |
133 |
26 δ(HCN) |
1306 |
88 |
19 ν(C–N), 23 δ (HCH), 35 δ (CNN) |
1022 |
128 |
40 ν(C–N), 40 ν(N–N), 14 δ (CNN) |
1228 |
125 |
11 ν(C–N), 21 δ(HCN) |
972 |
52 |
13 ν(N–N), 49 δ(NCN), 16 δ(CNN) |
1166 |
114 |
31 δ(HCN) |
852 |
87 |
74 τ(HCNC), 15 τ(NCNN) |
1032 |
72 |
42 ν(C–N), 33 ν(N–N), 12 δ(CNN) |
835 |
153 |
16 τ(HCCC) |
963 |
38 |
13 ν(N–N), 14 δ(CNN) |
665 |
36 |
15 τ(HCNC), 62 τ(NCNN) |
899 |
32 |
74 τ(HCNN) |
584 |
84 |
14 τ (NCCC), 11 δ(HCCC) |
852 |
53 |
62 τ(HCNN), |
Lapatinib |
724 |
50 |
12 τ(HCCC) |
3576 |
586 |
100 ν(N–H) |
668 |
50 |
19 τ(HCNN), 61τ(NCNN) |
3018 |
125 |
52 ν(C–H) |
Neratinib |
2965 |
184 |
97 ν(C–H) |
3618 |
121 |
100 ν(N–H) |
1654 |
605 |
25 ν(C C) |
3559 |
187 |
100 ν(N–H) |
1649 |
119 |
12 ν(C C) |
3125 |
235 |
66 ν(C–H), 15 ν(C–H) |
1606 |
753 |
11 ν(C C) |
3075 |
196 |
47 ν(C–H) |
1600 |
612 |
17 ν(C–N) |
2927 |
624 |
54 ν(C–H) |
1536 |
1591 |
12 δ(HCC), 11 δ(HCC) |
2308 |
485 |
89 ν(C N), 11 ν(C–C) |
1453 |
811 |
68 δ(HCH) |
1746 |
162 |
53 ν(C O) |
1317 |
418 |
13 δ(HCC) |
1704 |
253 |
25 ν(C O), 48 ν(C C) |
1295 |
17 |
18 δ(HCN) |
1629 |
275 |
32 ν(C–N), 12 ν(C C) |
1283 |
1905 |
16 ν(C–C), 30 ν(C–O) |
1574 |
1458 |
29 δ(HNC), 11 δ(HCC) |
1261 |
661 |
26 τ(HCCN) |
1536 |
908 |
10 ν(C O), 15 δ(HCC), 12 δ(CCC) |
1137 |
132 |
47 ν(C–N), 29 ν(C–N) |
1528 |
904 |
73 δ(HCH) |
909 |
203 |
62 τ(HCCC) |
1472 |
642 |
13 δ(HCH) |
Tucatinib |
1470 |
114 |
18 δ(HCC) |
3634 |
240 |
98 ν(N–H) |
1395 |
467 |
26 τ(HCCC), 20 τ(HCCC) |
3154 |
142 |
100 ν(C–H) |
1315 |
334 |
12 ν(C–N), 10 ν(C–O) |
1711 |
1328 |
57 ν(C N) |
1293 |
622 |
12 ν(C–O), 21 δ(HCC) |
1668 |
534 |
21 ν(C C) |
1271 |
765 |
10 ν(C–C), 11 δ(HCC) |
1608 |
1235 |
37 ν(C C) |
1190 |
535 |
22 ν(C–N), 15 ν(C–O), 20 δ(HCC) |
1583 |
1430 |
10 ν(C C) |
1056 |
266 |
27 ν(C–O), 20 δ(CCC) |
1484 |
393 |
71 δ(HCH) |
929 |
171 |
10 ν(C–C), 27 τ(HCCN) |
1451 |
2096 |
76 ν(C N) |
771 |
146 |
17 τ(HCCC), 21 τ(HCCC), 42 τ(HCCN) |
1318 |
342 |
33 ν(C–C) |
617 |
193 |
65 τ(HNCC) |
1250 |
510 |
20 δ(HCC), 20 ν(C–C) |
|
|
|
1215 |
398 |
11 δ(HCC), 12 ν(C–O) |
|
|
|
1210 |
874 |
12 δ(HCO), 12 ν(C–N) |
|
|
|
842 |
215 |
11 τ(HCCN), 18 τ(HNCN), 15 τ(HCCC) |
The C–H stretching vibrations of anastrozole, letrozole, lapatinib, neratinib, and tucatinib fall within the expected range for aromatic and heteroaromatic compounds, as shown in Table 3. These drugs contain aromatic rings and various functional groups, resulting in C–H stretches in the 3000–3300 cm−1 region. In contrast, aliphatic C–N bonds, typically found in simple amines, usually exhibit stretching vibrations in the range of 1000–1350 cm−1. Aromatic C–N bonds typically appear in a slightly higher range, approximately 1250–1350 cm−1. Meanwhile, C
N stretching generally occurs in the range of 1600–1690 cm−1.80 The C–N and C
N stretching vibrations for anastrozole, letrozole, lapatinib, neratinib, and tucatinib are observed within the expected ranges (see Table 3). The peak ∼2308 cm−1, ∼2334 cm−1, and ∼2340 cm−1 in neratinib, letrozole, and anastrozole, respectively, correspond to the C
N stretching vibration. The infrared (IR) absorption of C–O stretching is typically intense, occurring in the range of 1000 to 1300 cm−1.81 As shown in Table 3, C–O stretching is observed in lapatinib at 1283 cm−1, while in neratinib, it appears at approximately 1056 cm−1. For tucatinib, the C–O stretching modes are more narrowly distributed around 1215 cm−1. The C–C stretching and C
C stretching vibrations appear typically in the range 800–1300 cm−1 and 1620–1680 cm−1, respectively. The prominent vibrations corresponding to C–C and C
C stretching vibrations in all five drugs are presented in Table 3.
Toxicity profile/toxicological endpoints
Toxicological analysis plays an important role in the drug design process, helping to assess the adverse effects of chemicals on humans, animals, plants, and the environment. Traditional approaches for evaluating a compound's toxicity typically involve in vivo animal testing, which can be expensive, time-consuming, and raises ethical concerns.82 Therefore, the computer-aided in silico toxicity assessments of chemical compounds are advantageous for the drug design process. In this study, the ProTox-II web server was utilized to computationally evaluate the toxicity of the compounds. This approach is efficient, cost-effective, and avoids ethical dilemmas associated with traditional methods. We assessed the hepatotoxicity, neurotoxicity, nephrotoxicity, respiratory toxicity, cardiotoxicity, carcinogenicity, immunotoxicity, mutagenicity, cytotoxicity, and the ability to cross the blood–brain barrier (BBB) for five breast cancer drugs, along with their toxicological endpoints as detailed in Table 4. The results indicated that none of the drugs exhibited hepatotoxicity, mutagenicity, or cardiotoxicity. Furthermore, according to Edith et al., forty-four clinical studies reported low levels of cardiotoxicity associated with lapatinib.83 Except for lapatinib and neratinib, none of the drugs were found to be nephrotoxic. Anastrozole, letrozole, and tucatinib were identified as carcinogenic. Patients with breast cancer frequently encounter psychosocial stress, which can exacerbate inflammation and contribute to neurotoxic symptoms.84
Table 4 Pro Tox II predicted organ toxicity and toxicological and acute toxicity of anticancer drugs
Toxicity profile |
Anticancer drugs |
Anastrozole |
Letrozole |
Lapatinib |
Neratinib |
Tucatinib |
Hepatotoxicity |
Prediction |
Inactive |
Inactive |
Inactive |
Inactive |
Inactive |
Neurotoxicity |
Prediction |
Active |
Active |
Active |
Active |
Active |
Nephrotoxicity |
Prediction |
Inactive |
Inactive |
Active |
Active |
Inactive |
Respiratory toxicity |
Prediction |
Active |
Active |
Active |
Active |
Active |
Cardiotoxicity |
Prediction |
Inactive |
Inactive |
Inactive |
Inactive |
Inactive |
Carcinogenicity |
Prediction |
Active |
Active |
Inactive |
Inactive |
Active |
Immunotoxicity |
Prediction |
Inactive |
Inactive |
Active |
Active |
Active |
Mutagenicity |
Prediction |
Inactive |
Inactive |
Inactive |
Inactive |
Inactive |
Cytotoxicity |
Prediction |
Inactive |
Inactive |
Active |
Inactive |
Inactive |
BBB permeability |
Active |
Active |
Active |
Active |
Active |
Active |
LD50 (mg kg−1) |
2900 |
1463 |
1500 |
4000 |
3160 |
Acute toxicity class |
5 |
4 |
4 |
5 |
5 |
Additionally, one of the most prevalent non-hematologic side effects of breast cancer treatment is drug-induced pulmonary toxicity, particularly when high-dose chemotherapy is administered alongside breast cancer medications.85 Previous studies have emphasized the cytotoxic effects of lapatinib when used in conjunction with other breast cancer medications against MCF-7 cells.86 Several studies have particularly noted the enhanced cytotoxicity of lapatinib when combined with agents like sorafenib, paclitaxel, and fulvestrant.87 Consistent with these findings, our current study also demonstrates the cytotoxic effects of lapatinib under similar experimental conditions. All the drugs analyzed in this study exhibit associated neurotoxicity, which directly results from their application in radiation therapy (RT) and chemotherapy (CT). This observation is supported by numerous studies examining RT and CT for cancer treatment, as well as various epidemiological studies. Liao et al.88 observed that cancer therapies may trigger a myasthenic-like syndrome impacting the neuromuscular junction. This condition is characterized by symptoms such as ptosis, double vision, muscle weakness, and respiratory issues, often exacerbated by fatigue or repetitive movements. Neurotoxic effects from cancer treatments can arise due to an intensified immune response and unintended cross-reactivity with nervous system cells. Radiation therapy, which targets proliferating cells, can also harm neural tissues either directly or indirectly through mechanisms like vascular injury, hormonal imbalances, and fibrosis of neural structures.89
Plotkin et al.90 found that neurotoxicity can arise from direct harm to neurons or glial cells, or indirectly by affecting the surrounding microenvironment, such as causing localized vascular damage. Additionally, certain chemotherapy drugs that can cross the BBB may negatively impact cognitive functions, leading to issues like memory impairment and depression. Yang et al.91 observed that the anticancer drug methotrexate induces depression-like behaviors in mice, both one and seven days post-treatment, which was associated with decreased hippocampal neurogenesis, lower cell viability, and increased levels of cytotoxicity and apoptosis. Chemotherapy has been shown to alter ion channels in dorsal root ganglia and dorsal horn neurons, leading to secondary effects that manifest as neuropathic pain.92 Crew et al.93 noted that neurotoxic substances and neurodegenerative conditions can impact synaptic plasticity and neurogenesis within the adult hippocampus.
Epidemiological studies94 have highlighted neurological impairments and psychological distress linked to chemotherapy in patients with various types of peripheral cancers, such as breast cancer, colorectal cancer, and lymphoma, as well as in those with brain tumors, including gliomas, glioblastomas, and primary central nervous system lymphoma. The BBB serves as the primary protective gateway for the CNS. As a specialized neurovascular unit composed of tightly connected, non-porous blood vessels, the BBB meticulously controls the movement of ions, molecules, and cells between the bloodstream and the brain.95 It interacts with various cell types, including endothelial, immune, glial, and neuronal cells, providing crucial defense against pathogens, toxins, injury, inflammation, and diseases. However, this barrier also presents a challenge for the delivery of therapeutic drugs to the brain. As indicated in Table 4, all five anticancer drugs possess the ability to cross the blood–brain barrier, potentially impacting healthy brain tissue and leading to neurotoxicity. Chemotherapy is known for its toxic effects, which include multiple side effects, and it can significantly weaken the immune system by impairing bone marrow function, making patients more susceptible to other diseases.96 In our study, we observed that lapatinib, neratinib, and tucatinib exhibit immunotoxic effects.
The median lethal dosage (LD50) values were reported between 1463 mg kg−1 for letrozole and 4000 mg kg−1 for neratinib (Table 4). Acute classifications describe drugs depending on their toxicity levels. These classes are numbered from 1 to 5, with 1 being the most poisonous and 5 being the least harmful. Since the studied five anticancer drugs typically fall into acute toxicity class 4 and 5, this indicates that they pose moderate to low toxicity risks, meaning they are less hazardous and their potential for severe harm is lower.
Molecular docking analysis
Molecular docking analysis was performed using AutoDock Vina tool,97 which is a part of MGL Tools package. The program was used to evaluate the target proteins' active site area. The docking analysis subsequently utilized these binding sites of the breast cancer proteins.
Interpretation of protein–ligand interaction (active site)
In AutoDock, the active site is determined by creating a grid box around the predicted binding region. This tool assists in choosing the grid's size and position. The protocol requires setting up the grid box while ensuring that both the protein and ligand files are properly prepared in the PDBQT format. The next step involves configuring the grid box to define the region of interest, such as the active site or potential binding site, where ligand docking will take place using AutoDock tools. The grid center can be adjusted by either manually entering the X, Y, and Z coordinates or by dragging the box to the desired location. In this study, the grid center was set at coordinates (x = 100, y = 94, and z = 90) with a grid spacing of 0.785 Å. The grid box needs to be sufficiently large to cover the active site and provide enough room for ligand movement.98 Proper grid box configuration ensures that the docking is focused on the appropriate protein region, enhancing the precision and relevance of the docking outcomes. The interactions between breast cancer drugs and their target proteins were examined using BIOVIA Discovery Studio 2021 software,65 where bond types and bond distances were analyzed during visualization.
Tamoxifen's inhibitory effects on breast cancer have been previously documented.99 Lapatinib, neratinib, and tucatinib are small-molecule tyrosine kinase inhibitors that have demonstrated positive outcomes in phase 3 trials for patients with HER2-positive breast cancer.100,101 Prior studies have compared neratinib as a monotherapy to lapatinib in combination therapy, but these trials were inconclusive in determining whether neratinib was superior or noninferior. While overall survival was slightly higher for lapatinib, the difference was not statistically significant.102 Treatment with tucatinib led to a complete inhibition of HER2 autophosphorylation within 30 minutes in HER2-positive breast cancer cells, which was also linked to a reduction in EGFR, AKT signaling, and S6 phosphorylation.45 Previous studies103 identified lapatinib as a small, hydrophobic molecule classified as a tyrosine kinase inhibitor. It functions by inhibiting the phosphorylation of the human epidermal growth factor receptor 2 (HER2) and the epidermal growth factor receptor (EGFR), thereby suppressing their abnormal activity. Despite its therapeutic role, lapatinib is 99% bound to human serum albumin (HSA), the primary transporter of many drugs in the bloodstream. M. Priya et al.104 conducted docking studies on the breast cancer drug biochanin-A, targeting proteins such as HER-2, BCL2, ERα, EGFR, GSK3, PDK1, PR, and PAK4. Their findings showed the highest binding affinity for HER-2 (PDB ID: 2IOK) with a docking score of −9.2 kcal mol−1.
Here we investigate the docking of drugs (anastrozole, letrozole, lapatinib, neratinib, and tucatinib) against the target proteins HER-2 (PDB ID:2IOK), BCL2 (PDB ID:4AQ3), EGFR (PDB ID:2J6M), Era (PDB ID:6WOK), PR (PDB ID:4OAR), PAK4 (PDB ID:2X4Z), GSK3 (PDB ID:1GNG), and PDK1 (PDB ID:1UU7). The docking scores of anticancer drugs against target proteins are presented in Table 5.
Table 5 The docking scores of anticancer drugs against target proteins
S. No. |
Protein |
PDB ID |
Anastrozole |
Letrozole |
Lapatinib |
Neratinib |
Tucatinib |
1 |
HER2 |
2IOK
|
−7.2 |
−8.3 |
−9.8 |
−9.3 |
−9.7 |
2 |
BCL2 |
4AQ3
|
−8.0 |
−8.1 |
−11.1 |
−10.8 |
−10.7 |
3 |
EGFR |
2J6M
|
−8.1 |
−9.1 |
−10 |
−10 |
−9.1 |
4 |
Erα |
6WOK
|
−6.6 |
−8.5 |
−9.6 |
−9.2 |
−8.8 |
5 |
PR |
4OAR
|
−6.6 |
−5.2 |
−10.2 |
−7.8 |
−9.9 |
6 |
PAK4 |
2X4Z
|
−7.3 |
−11.4 |
−11.5 |
−10.0 |
−9.8 |
7 |
GSK3 |
1GNG
|
−7.6 |
−8.1 |
−11.4 |
−10.3 |
−10.0 |
8 |
PDK1 |
1UU7
|
−7.8 |
−7.5 |
−12.2 |
−9.8 |
−10.9 |
The Interaction of anastrozole, letrozole, lapatinib, neratinib and tucatinib with HER2 (PDB ID:2IOK)
The interaction between the above-studied drugs and the target protein HER2 is shown in Fig. 6, and the binding affinities in terms of docking scores are shown in Table 5. The free energy of binding for the complex HER2-anastrozole was found to be −7.2 kcal mol−1. Anastrozole was found to interact with HER2 receptors through pi–pi T-shaped and pi-alkyl hydrophobic interaction with the amino acid (aa) residue Trp 1393, Ile 1326, Arg 1394 and Pro 1324 with the bond distance of 5.06 Å, 5.47 Å, 5.01 Å and 4.78 Å respectively. The binding affinity of HER2-letrozole was calculated to be −8.3 kcal mol−1, with interactions including a carbon–hydrogen bond with Leu (distances of 4.88 Å and 5.21 Å) and hydrophobic interactions (pi-Sigma, pi-alkyl, and pi-sulfur) with Ala 350, Leu 525, Leu 387, and Met 343, at bond distances of 3.84 Å, 3.95 Å, 5.00 Å, and 4.75 Å, respectively. HER2-lapatinib exhibited the highest binding affinity at −9.8 kcal mol−1, forming three hydrogen bonds with Cys 381 (3.56 Å), Glu 380 (2.52 Å), and Lys 1250 (2.52 Å), as well as pi–pi stacked hydrophobic interactions with Tyr 537 involving three benzene rings of lapatinib (bond lengths of 3.76 Å, 4.81 Å, and 5.08 Å), along with a pi-alkyl interaction with Met 522 (4.84 Å).
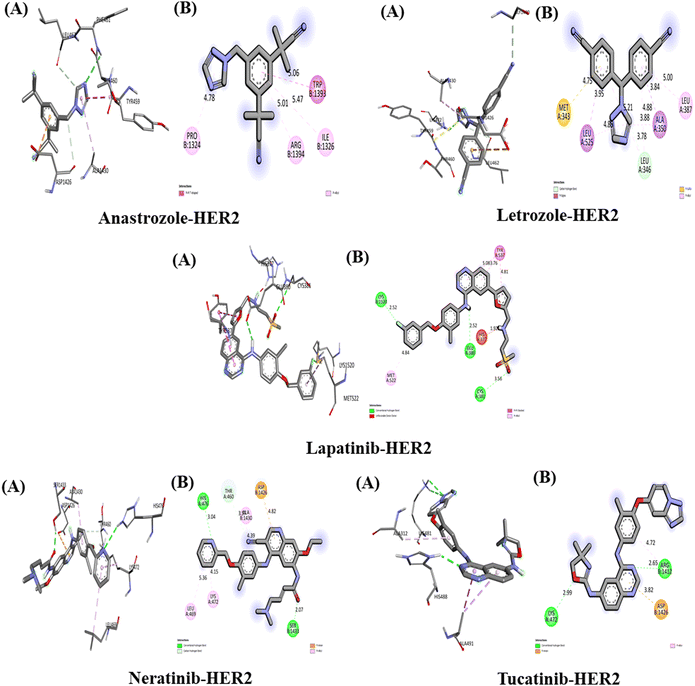 |
| Fig. 6 Interaction of five anti-cancerous drugs with HER2. (A)3D interaction of anti-cancer drug at the active site of the target protein. (B) 2D interaction of anticancer drugs with HER2, including type of bond. | |
HER2-neratinib showed a binding affinity of −9.3 kcal mol−1, involving two conventional hydrogen bonds with Ser 1433 (2.07 Å) and His 476 (3.04 Å), one carbon–hydrogen bond with Thr 460 (3.57 Å), and hydrophobic interactions with Lys 472 (4.15 Å), Leu 469 (5.36 Å), Ala 1430 (4.39 Å), and Asp 1426 (4.82 Å). Tucatinib bound to HER2 with a binding affinity of −9.7 kcal mol−1, through two hydrogen bonds with Lys 472 (2.99 Å) and Arg 1412 (2.65 Å), a pi-anion interaction with Asp 1426 (5.98 Å), and a pi-alkyl interaction with Arg 1412 (4.72 Å). Among all the drugs, lapatinib demonstrated the strongest interaction with HER2, forming three hydrogen bonds and achieving the highest binding affinity.
The interaction of anastrozole, letrozole, lapatinib, neratinib and tucatinib with BCL2 (PDB ID: 4AQ3)
The interactions between the five studied drugs and the target protein BCL2 are illustrated in Fig. 7, and the binding affinities in terms of docking scores are listed in Table 5. Anastrozole binds to BCL2 with a binding affinity of −8.0 kcal mol−1, forming one conventional hydrogen bond with Glu 94 at a distance of 2.84 Å, as well as a pi-anion and pi-alkyl hydrophobic interaction with Glu 94 and Ala 90, at bond distances of 3.34 Å and 4.31 Å, respectively, as shown in Fig. 7. Letrozole binds to BCL2 with a binding affinity of −8.3 kcal mol−1, forming one hydrogen bond with Ser 1433 (2.07 Å) and His 476 (3.04 Å), one carbon–hydrogen bond with Gly 162 (2.39 Å), and pi–pi T-shaped and pi-alkyl hydrophobic interactions with Phe 63 (5.50 Å), Ala 108 (5.12 Å, 4.69 Å), and Arg 105 (5.25 Å). Lapatinib binds to BCL2 with a binding affinity of −11.1 kcal mol−1, forming one hydrogen bond with Arg 65 (4.37 Å), one carbon hydrogen bond with Asp 61 (3.22 Å), a pi-cation interaction with Asp 150 (3.87 Å), a pi-anion interaction with Asp 61 (4.49 Å and 4.73 Å), and pi-alkyl interactions with Arg 65 and Arg 68 at distances of 4.50 Å and 5.41 Å, respectively. Neratinib binds to BCL2 with a binding affinity of −10.8 kcal mol−1, forming one hydrogen bond with Arg (2.35 Å), two carbon–hydrogen bonds with His 143 (3.36 Å), and Trp 147 (3.63 Å), and pi-anion and pi-alkyl hydrophobic interactions with Glu 119 (3.82 Å) and Ala 72 (4.72 Å).
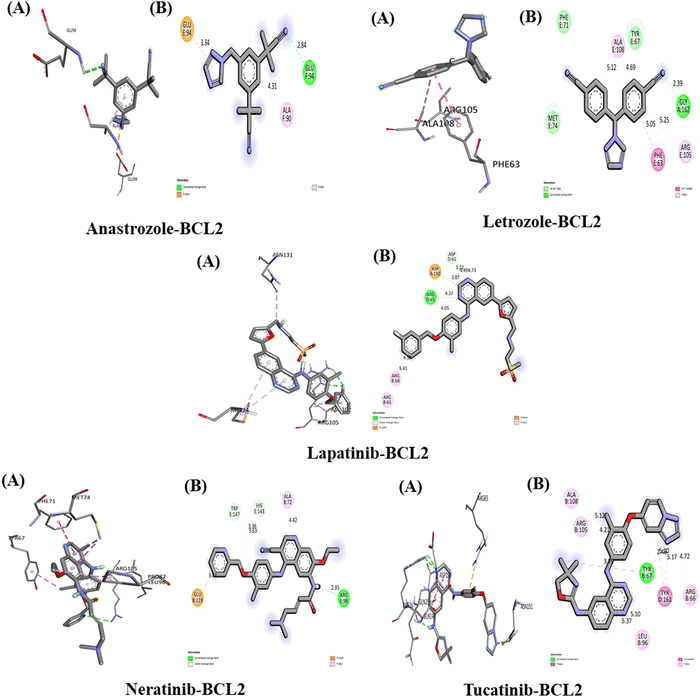 |
| Fig. 7 Interaction of five anti-cancerous drugs with BCL2. (A) 3D interaction of anti-cancer drug at the active site of the target protein. (B) 2D interaction of anticancer drugs with BCL2, including type of bond. | |
Tucatinib interact with BCL2 with binding affinity −10.7 kcal mol−1 forming one conventional H-bond with aa residue Tyr 67 (2.41 Å) and Pi-akyl hydrophobic interaction with aa residue Arg 105 (4.21 Å), Ala 108 (5.12 Å), Arg 66 (4.72 Å) and Leu 96 (5.10 Å, 5.37 Å), one pi–pi stacked with aa residue Tyr 161 at 5.60 Å and 5.17 Å and Pi-Sigma hydrophobic interaction with aa residue Tyr 67 at distance 3.79 Å. Our result showed that among all five drugs, lapatinib has the strongest interaction with target protein BCL2 with one H-bond and maximum binding affinity.
The Interaction of anastrozole, letrozole, lapatinib, neratinib and tucatinib with EGFR (PDB ID: 2J6M)
The interactions between the five studied drugs and the target protein EGFR are depicted in Fig. 8, and the binding affinities in terms of docking scores are presented in Table 5. Anastrozole binds to EGFR with a binding affinity of −8.1 kcal mol−1, forming one hydrogen bond with Thr 854 at a distance of 2.33 Å, as well as pi-alkyl and pi-Sigma hydrophobic interactions with Val 726 and Leu 844 at distances of 5.24 Å and 3.81 Å, respectively. Letrozole shows a binding affinity of −9.1 kcal mol−1 with EGFR, forming pi-alkyl interactions with Ala 743, Leu 844, Val 726, and Lys 745 at bond distances of 4.90 Å, 4.98 Å, 5.17 Å, and 5.09 Å, along with van der Waals interactions with Met 793 and Phe 723. Lapatinib binds to EGFR with a binding affinity of −10 kcal mol−1, forming a carbon–hydrogen bond with Asp 800 at 3.29 Å, as well as pi-alkyl interactions with Ala 743 (4.53 Å, 3.73 Å), Leu 844 (5.32 Å), Leu 718 (4.98 Å), and Val 726 (5.18 Å), a pi-Sigma interaction with Leu 718 (3.66 Å), and a pi-sulfur interaction with Phe 723 (5.20 Å).
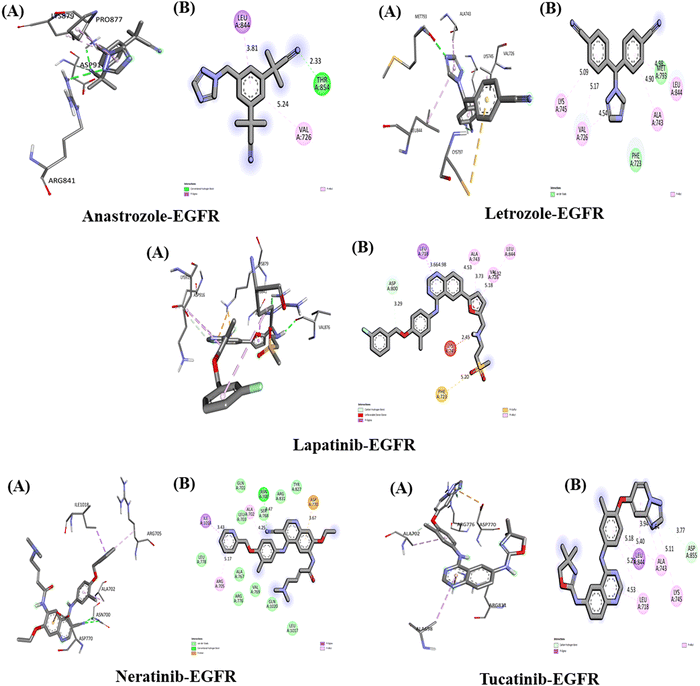 |
| Fig. 8 Interaction of five anti-cancerous drugs with EGFR. (A) 3D interaction of anti-cancer drug at the active site of the target protein. (B) 2D interaction of anticancer drugs with EGFR, including the type of bond. | |
Similarly, neratinib shows a binding affinity of −10 kcal mol−1 with EGFR, forming a hydrogen bond with Asn 700 at 4.77 Å, a pi-anion interaction with Asp 770 (3.67 Å), pi-alkyl interactions with Ala 702 (4.25 Å) and Arg 705 (5.17 Å), a pi-Sigma interaction with Ile 1018 (3.43 Å), and several van der Waals interactions. Tucatinib binds to EGFR with a binding affinity of −9 kcal mol−1, forming a hydrogen bond with Asp 855 (3.77 Å), pi-alkyl interactions with Ala 743 (4.64 Å, 5.18 Å), Lys 745 (5.11 Å), and Leu (5.29 Å, 4.53 Å), and a pi-Sigma interaction with Leu 844 (3.94 Å). Among the five drugs, lapatinib and neratinib showed the strongest interactions with EGFR, exhibiting the highest binding affinities.
The Interaction of anastrozole, letrozole, lapatinib, neratinib and tucatinib with ERa (PDB ID: 6WOK)
The interactions between the five studied drugs and the target protein ERα are depicted in Fig. 9, and the binding affinities in terms of docking scores are presented in Table 5. Anastrozole binds to ERα with a binding affinity of −6.6 kcal mol−1, forming two conventional hydrogen bonds with Thr 347 (2.46 Å) and Pro 535 (2.19 Å), as well as a pi-Sigma hydrophobic interaction with Leu 525 (3.40 Å), as shown in Fig. 9. Letrozole binds to ERα with a higher binding affinity of −8.5 kcal mol−1, exhibiting pi-alkyl hydrophobic interactions with Leu 525 (5.08 Å), Leu 349 (4.77 Å), and Leu 346 (4.89 Å and 5.43 Å). It also forms a pi-sulfur interaction with Met 421 (5.62 Å) and a pi-Sigma interaction with Ala 350 (3.69 Å). Lapatinib binds to the ERα target protein with a binding affinity of −9.6 kcal mol−1. It forms one conventional hydrogen bond with Gly 442 at 2.40 Å, a carbon–hydrogen bond with Glu 397 at 3.75 Å, and a pi-donor hydrogen bond with Gly 442 at 2.89 Å. Additionally, lapatinib establishes three pi-alkyl interactions with Leu 403, Pro 406, and Met 396 at bond distances of 4.94 Å, 5.00 Å, and 4.97 Å, respectively, along with a pi-Sigma hydrophobic interaction with Trp 393 at 5.16 Å. Neratinib binds to ERα with a binding affinity of −9.2 kcal mol−1, forming two conventional hydrogen bonds with Arg 412 at 2.39 Å and Asp 538 at 2.74 Å. It also exhibits pi-alkyl interactions with Leu 429 and Arg 412 at 5.27 Å and 5.30 Å, a pi-cation interaction with His 373 at 3.03 Å and 4.53 Å, and a pi–pi T-shaped interaction with His 377 at 5.25 Å. Tucatinib binds to ERα with a binding affinity of −8.8 kcal mol−1, forming one conventional hydrogen bond with Asp 374 at 1.82 Å, a pi-alkyl interaction with Met 543 at 4.88 Å, and both pi-cation and pi-anion interactions with His 373 and Asp 538 at 3.20 Å and 3.74 Å, respectively. Among the studied drugs, lapatinib and neratinib demonstrated the strongest interactions with ERα, exhibiting the highest binding affinities.
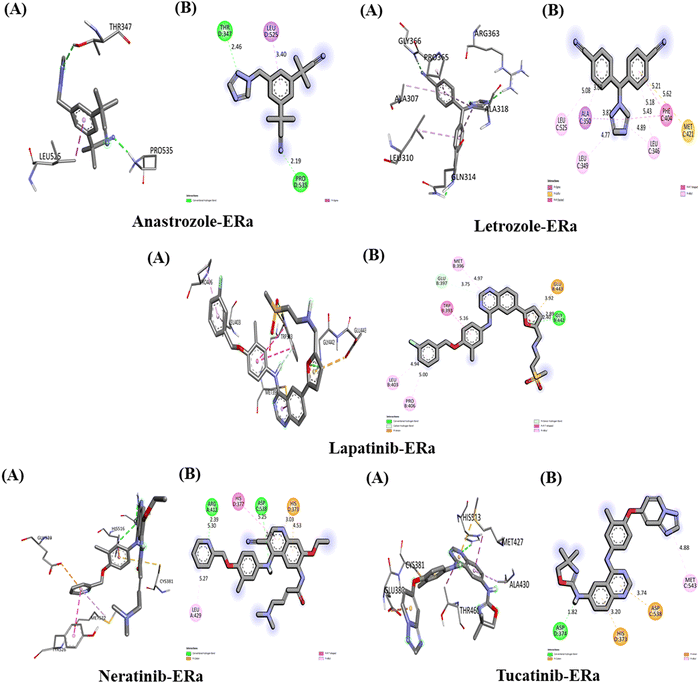 |
| Fig. 9 Interaction of five anti-cancerous drug with ERα. (A) 3D interaction of anti-cancer drug at the active site if the target protein. (B) 2D interaction of anticancer drugs with ERα, including type of bond. | |
The interaction of anastrozole, letrozole, lapatinib, neratinib and tucatinib with PR (PDB ID: 4OAR)
The interactions of the five studied drugs with the target protein are illustrated in Fig. 10, and the binding affinities in terms of docking scores are detailed in Table 5. Anastrozole forms one acceptor carbon–hydrogen bond and one pi-donor hydrogen bond with the amino acids Asn 719 and Cys 891 at distances of 2.60 Å and 4.02 Å, respectively, resulting in a weak binding affinity of −6.6 kcal mol−1 with the target protein. Letrozole exhibits a lower binding affinity of −5.2 kcal mol−1 when interacting with the PR target protein, forming a carbon–hydrogen bond with Glu 791 at 3.58 Å and 3.53 Å, as well as a pi-anion and a pi-cation interaction with Glu 791 and Arg 788 at bond distances of 3.55 Å and 4.35 Å, respectively. In contrast, lapatinib demonstrates the strongest binding affinity of −10.2 kcal mol−1 with the PR target protein, forming two conventional hydrogen bonds with Asn 719 and Cys 891 at distances of 2.65 Å and 3.67 Å. Additionally, it establishes a carbon–hydrogen bond with Leu 718 at 3.61 Å, a pi-anion hydrophobic interaction with Glu 723 at 3.54 Å and 4.32 Å, a pi-alkyl interaction with Leu 726 at 5.12 Å, a pi–pi T-shaped interaction with Trp 755 at 5.96 Å, and a pi-Sigma interaction with Leu 350 at 3.58 Å and 3.97 Å.
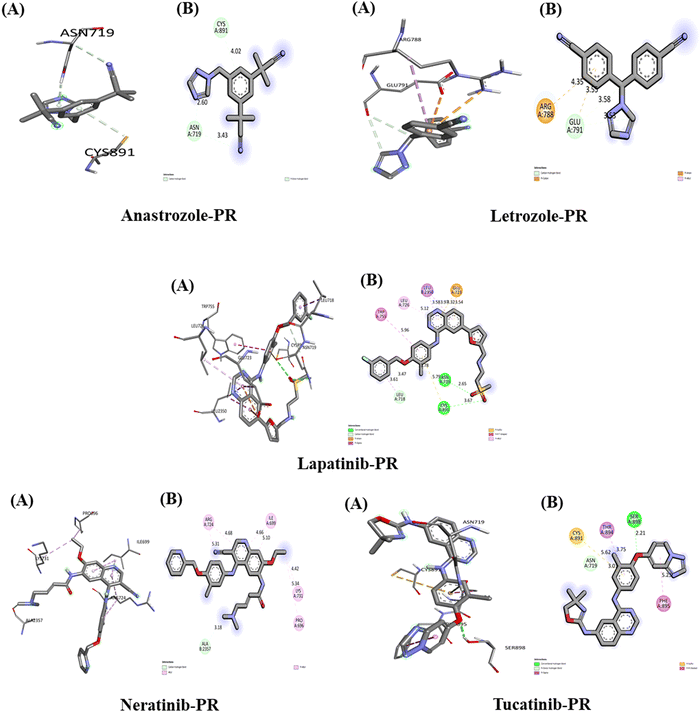 |
| Fig. 10 Interaction of five anti-cancerous drug with PR. (A) 3D interaction of anti-cancer drug at the active site if the target protein. (B) 2D interaction of anticancer drugs with PR, including type of bond. | |
Neratinib interacts with the PR target protein, exhibiting a binding affinity of −7.8 kcal mol−1. It forms a carbon–hydrogen bond with the amino acid Ala 2357 at a distance of 3.18 Å and establishes pi-alkyl hydrophobic interactions with Arg 724 (5.31 Å, 4.68 Å), Ile 699 (4.66 Å, 5.10 Å), Lys 731 (4.42 Å), and Pro 696 (5.34 Å). In contrast, tucatinib displays a stronger binding affinity of −9.9 kcal mol−1 with PR, forming a conventional hydrogen bond with Ser 898 at 2.21 Å, a pi-donor hydrogen bond with Asn 719 at 3.01 Å, a pi-sulfur interaction with Cys 891 at 5.62 Å, a pi-Sigma interaction with Thr 894 at 3.75 Å, and a pi-stacked interaction with Phe 895 at 5.23 Å. Our results indicate that lapatinib demonstrates the best interaction with the PR target protein, achieving the highest binding affinity among the five drugs studied.
The interaction of anastrozole, letrozole, lapatinib, neratinib and tucatinib with PAK4 (PDB ID: 2X4Z)
The interactions between the five studied drugs and the target protein are illustrated in Fig. 11, with corresponding docking scores representing the binding affinities presented in Table 5. The binding affinity of anastrozole with PAK4 was measured at −7.3 kcal mol−1, involving a conventional hydrogen bond and a pi-Sigma hydrophobic interaction with Asp 444 and Leu 447, with bond distances of 3.51 Å and 5.69 Å, respectively. In contrast, lapatinib demonstrated a significantly stronger binding affinity of −11.5 kcal mol−1, forming a carbon–hydrogen bond with Gly 330 at 3.53 Å and a pi-Sigma hydrophobic interaction with Thr 404 at 3.75 Å. Additionally, lapatinib established five pi-alkyl hydrophobic interactions with Lys 350, Val 335, Ala 348, Leu 447, and Ala 402, at bond distances of 4.90 Å, 5.04 Å, 5.16 Å, 4.98 Å, and 4.69 Å, respectively, along with several van der Waals interactions with Ser 331 and Gly 330. Neratinib exhibited a binding affinity of −10.0 kcal mol−1, forming two carbon–hydrogen bonds with Gly 330 and Asp 444 at 3.52 Å and 3.60 Å, respectively, alongside a pi-anion hydrophobic interaction with Asp 458 at 3.84 Å and 4.16 Å. It also included two pi-alkyl hydrophobic interactions with Lys 350 and Val 335 at bond distances of 4.98 Å (5.39 Å) and 5.31 Å, respectively, along with van der Waals interactions with Ala 348 and Ala 402.
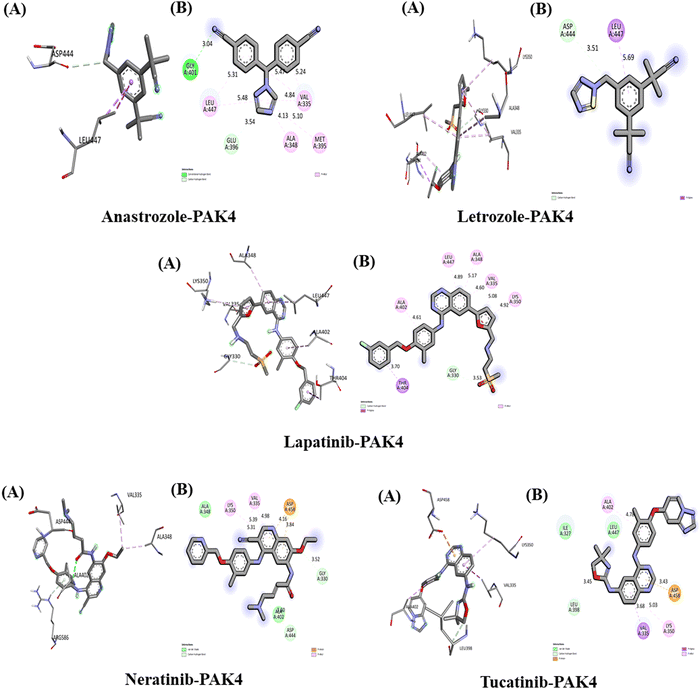 |
| Fig. 11 Interaction of five anti-cancerous drug with PAK4. (A) 3D interaction of anti-cancer drug at the active site if the target protein. (B) 2D interaction of anticancer drugs with PAK4, including type of bond. | |
Tucatinib demonstrated a binding affinity of −9.8 kcal mol−1, forming a carbon–hydrogen bond with Leu 398 at 3.45 Å and a pi-anion hydrophobic interaction with Asp 458 at 3.43 Å. It also established two pi-alkyl hydrophobic interactions with Lys 350 and Ala 402 at bond distances of 5.03 Å and 4.78 Å, respectively, along with van der Waals interactions with Ala 348 and Ala 402, and a pi-Sigma interaction with Val 335 at a bond distance of 3.68 Å. Our results indicate that among the five drugs studied, lapatinib exhibited the strongest interaction with the target protein PAK4, achieving the highest binding affinity.
The interaction of anastrozole, letrozole, lapatinib, neratinib and tucatinib with GSK3 (PDB ID: 1GNG)
The interactions between the five studied drugs and the target protein are depicted in Fig. 12, with the docking scores representing the binding their affinities are presented in Table 5. The binding affinity of anastrozole with GSK3 was measured at −7.6 kcal mol−1, forming one conventional hydrogen bond with Gln 280 at 2.42 Å, Trp 301 at 2.00 Å, and Glu 322 at 3.71 Å. Additionally, it established a pi–pi T-shaped interaction with Trp 301 at 4.50 Å and a pi-alkyl hydrophobic interaction with Ala 298 at bond distances of 5.29 Å and 4.81 Å. Letrozole exhibited a binding affinity of −8.1 kcal mol−1 with GSK3, forming one conventional hydrogen bond with Lys 85 at 2.58 Å and a pi-sulfur hydrophobic interaction with Cys 199 at 5.90 Å. Furthermore, it created three pi-alkyl hydrophobic interactions with Leu 188, Val 70, and Ala 83, with bond distances of 5.46 Å, 4.53 Å, and 4.66 Å, respectively.
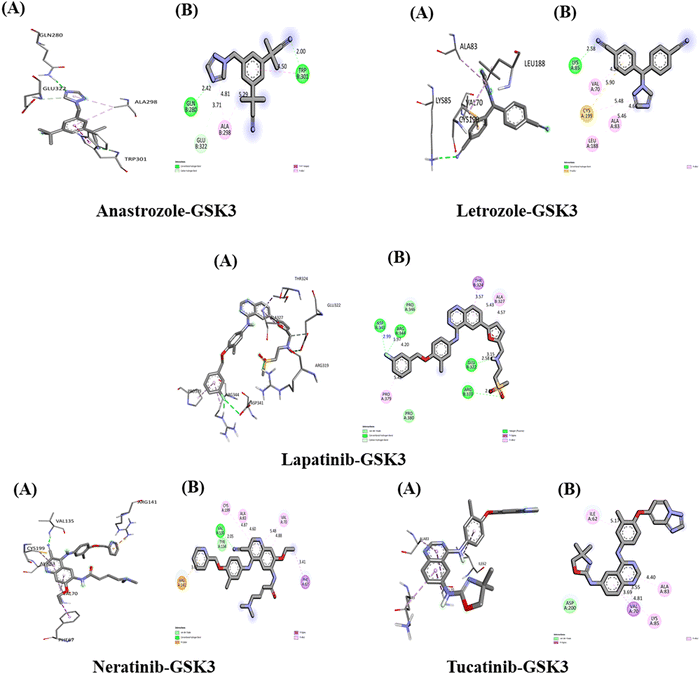 |
| Fig. 12 Interaction of five anti-cancerous drug with GSK3. (A) 3D interaction of anti-cancer drug at the active site if the target protein. (B) 2D interaction of anticancer drugs with GSK3, including type of bond. | |
The binding affinity of lapatinib with GSK3 was measured at −11.4 kcal mol−1, establishing three conventional hydrogen bonds with amino acids Glu 322, Arg 319, and Arg 344 at distances of 2.58 Å, 2.63 Å, and 1.97 Å, respectively. It also formed a carbon hydrogen bond with Glu 322 at 3.15 Å, a pi-Sigma hydrophobic interaction with Thr 324 at 3.57 Å, and three pi-alkyl hydrophobic interactions with Pro 379 (5.42 Å) and Ala 327 (5.43 Å and 4.57 Å). Additionally, the fluorine atom in lapatinib formed a bond with Asp 341 at a distance of 2.99 Å. Neratinib exhibited a binding affinity of −10.3 kcal mol−1 with GSK3, forming one conventional hydrogen bond with Val 135 at 2.05 Å, a pi-Sigma hydrophobic interaction with Phe 67 at 3.41 Å, and a pi-cation hydrophobic interaction with Arg 141 at 3.76 Å. It also established three pi-alkyl hydrophobic interactions with Val 70 (4.88 Å and 5.48 Å) and Ala 83 (4.87 Å and 4.60 Å), along with one van der Waals interaction with Tyr 134. Tucatinib's binding affinity with GSK3 was found to be −10 kcal mol−1, creating one pi-Sigma hydrophobic interaction with Val 70 (3.69 Å and 3.55 Å) and three pi-alkyl hydrophobic interactions with Ala 83, Lys 85, and Ile 62 at bond distances of 4.81 Å, 4.40 Å, and 5.17 Å, respectively. Additionally, it formed one van der Waals interaction with Asp 200. Among the five drugs studied, lapatinib demonstrated the strongest interaction with the target protein GSK3, exhibiting the highest binding affinity.
The interaction of anastrozole, letrozole, lapatinib, neratinib and tucatinib with PDK1 (PDB ID: 1UU7)
The interactions between the five studied drugs and the target protein are illustrated in Fig. 13, and the binding affinities in terms of docking scores are presented in Table 5. Anastrozole binds to the PDK1 target protein with a binding affinity of −7.8 kcal mol−1, forming two conventional hydrogen bonds with Phe 93 and Ser 94 at distances of 2.06 Å and 2.60 Å, respectively. It also creates one pi-Sigma interaction and one pi–pi stacked hydrophobic interaction with Tyr 126 and Phe 93 at bond distances of 3.83 Å and 4.82 Å, along with one van der Waals interaction with Gly 225. Letrozole exhibits a binding affinity of −7.5 kcal mol−1 with the PDK1 target protein, forming one pi-cation hydrophobic interaction with Lys 111 at 4.26 Å and two pi-alkyl hydrophobic interactions with Lys 123 and Val 127 at distances of 5.16 Å and 4.82 Å, respectively. Lapatinib shows a strong binding affinity of −12.2 kcal mol−1 with PDK1, forming a carbon–hydrogen bond with Glu 166 at 2.98 Å. It also establishes a pi-Sigma hydrophobic interaction with Leu 212 at distances of 3.39 Å and 3.75 Å, along with five pi-alkyl hydrophobic interactions with Leu 88 (4.99 Å and 4.17 Å), Ala 162 (5.21 Å), Ala 109 (4.84 Å and 4.65 Å), Val 143 (5.23 Å), and Val 96 (5.47 Å). Additionally, there are several van der Waals interactions with Asp 223, Lys 169, Gly 89, and Glu 209. Neratinib demonstrates a binding affinity of −9.8 kcal mol−1 with the PDK1 target protein, forming two carbon–hydrogen bonds with Gly 165 and Gly 89 at distances of 3.66 Å and 3.33 Å, respectively. It also establishes two pi-anion hydrophobic interactions with Asp 223 and Glu 166 at 3.89 Å and 3.50 Å, respectively, as well as four pi-alkyl hydrophobic interactions with Val 96 (4.67 Å), Leu 212 (5.05 Å), Leu 88 (4.74 Å and 5.48 Å), and Lys 169 (4.01 Å). Lastly, there is one van der Waals interaction with Glu 209. Tucatinib demonstrates a binding affinity of −10.9 kcal mol−1 with PDK1, forming one conventional hydrogen bond with Ala 162 at a bond distance of 2.02 Å and one carbon–hydrogen bond with Gly 165 at 3.23 Å. It also establishes three pi-alkyl hydrophobic interactions with Ala 109 (4.31 Å and 4.87 Å), Leu 88 (5.18 Å), Val 96 (5.40 Å and 4.23 Å), and Leu 212 (5.31 Å). Additionally, there is one van der Waals interaction with the amino acid Glu 166. Our results indicate that among all five drugs, lapatinib exhibits the strongest interaction with the PDK1 target protein, demonstrating the highest binding affinity.
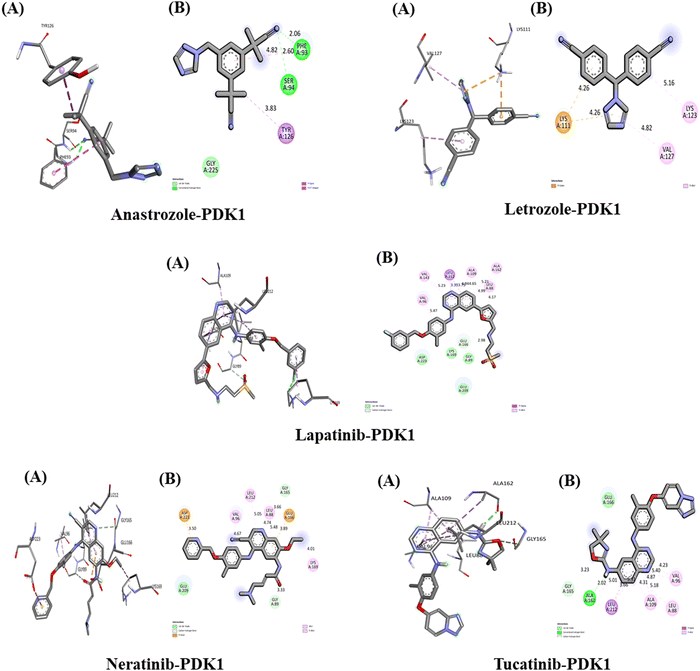 |
| Fig. 13 Interaction of five anti-cancerous drug with PDK1. (A) 3D interaction of anti-cancer drug at the active site if the target protein. (B) 2D interaction of anticancer drugs with PDK1, including type of bond. | |
In summary, each drug's structural features and target specificity dictate its use in cancer treatment. Lapatinib demonstrates robust binding affinities, whereas neratinib and tucatinib show the strongest interaction toward the target site due to their complex structures featuring multiple functional groups. Lapatinib's fluorinated quinazoline core, combined with its bulky structure and various substituents, enables it to form numerous favorable interactions within the binding pocket,105 evidenced by a low binding energy of −554 kJ mol−1 of lapatinib, indicating a high binding affinity.106 Tucatinib, with its pyrrolopyrimidine core, and neratinib, possessing a cyano-substituted acrylamide moiety, also influence their structural diversity to engage effectively with the targets.107 In contrast, anastrozole and letrozole are simpler molecules with fewer functional groups, resulting in limited interaction points. Their binding primarily relies on the coordination of the triazole ring with heme iron, which leads to a less favorable binding profile compared to the more complex inhibitors.108 Overall, the multi-functional groups and various combinations of hydrophobic interactions, hydrogen bonding, and polar interactions of lapatinib, neratinib, and tucatinib contribute significantly to their higher binding affinities and show potential therapeutic efficacy against breast cancer treatment.
Conclusions
Density functional theory (DFT) and molecular docking studies were conducted to gain insights into the anti-cancer efficacy and specificity of lapatinib, tucatinib, neratinib, anastrozole, and letrozole. DFT analysis provides detailed insights into the structural, electronic, optical, and vibrational properties of these drugs, enhancing our understanding of their molecular stability and reactivity through global reactivity descriptors. Furthermore, molecular docking studies elucidate the binding conformations and interaction profiles of these drugs with key breast cancer target proteins, highlighting their therapeutic potential.
Molecular docking results indicate that lapatinib, tucatinib, and neratinib have high binding affinities for HER2, with lapatinib demonstrating the strongest overall binding, especially with PDK1 (PDB ID: 1UU7), PAK4(PDB ID: 2X4Z), GSK3 (PDBID:1GNG) with a docking score of −12.2 kcal mol−1, 11.5 kcal mol−1 and 11.4 kcal mol−1 respectively. The highest binding affinity was observed against HER-2 (PDB ID: 2IOK) with a docking score of −9.8 kcal mol−1 by lapatinib drug. The strong hydrogen bonding and other interactions within the binding sites observed with lapatinib suggest a stable and effective interaction that supports its application in HER2-positive breast cancer treatment. Tucatinib's selective HER2 binding minimizes off-target effects, while neratinib's irreversible binding contributes to sustained inhibition, effectively countering resistance in HER2-positive cases.
In contrast, anastrozole and letrozole show lower binding affinities for HER2 and EGFR due to their simpler structures, but they remain potent inhibitors of estrogen biosynthesis by targeting aromatase in estrogen receptor-positive (ER-positive) cancers. The triazole rings in these molecules effectively coordinate with the heme iron in aromatase, highlighting their efficacy as primary treatments for ER-positive breast cancers.
In summary, DFT and molecular docking studies highlight the suitability of lapatinib, tucatinib, and neratinib for HER2-positive breast cancers, while anastrozole and letrozole are well-suited for ER-positive breast cancers. This analysis underscores the critical role of molecular structure and binding affinity in selecting appropriate treatments based on receptor specificity, optimizing therapeutic outcomes in breast cancer treatment.
Data availability
Authors confirm that all relevant data are included in the article.
Conflicts of interest
The authors have no conflicts of interest to declare that are relevant to the content of this article.
Acknowledgements
Md. Ashraf Ayub acknowledges the University for providing financial assistance in the form of UGC-non-NET Research fellowship.
References
- A. M. Metwaly, M. M. Ghoneim, I. H. Eissa, I. A. Elsehemy, A. E. Mostafa, M. M. Hegazy, W. M. Afifi and D. Dou, Saudi J. Biol. Sci., 2021, 28, 5823–5832 CrossRef.
- M. Akram and S. A. Siddiqui, Indian J. Cancer, 2012, 49, 277–282 CrossRef CAS PubMed.
- D. Nagarajan and S. McArdle, Biomedicines, 2018, 6, 20 CrossRef.
- I. Makhoul, M. Atiq, A. Alwbari and T. Kieber-Emmons, Breast Cancer: Basic Clin. Res., 2018, 12, 1178223418774802 CrossRef.
- M. Akram, M. Iqbal, M. Daniyal and A. U. Khan, Biol. Res., 2017, 50, 33 CrossRef PubMed.
- C. Liedtke, C. Mazouni, K. R. Hess, F. André, A. Tordai, J. A. Mejia, W. F. Symmans, A. M. Gonzalez-Angulo, B. Hennessy, M. Green, M. Cristofanilli, G. N. Hortobagyi and L. Pusztai, J. Clin. Oncol., 2008, 26, 1275–1281 CrossRef.
- B. D. Lehmann, J. A. Bauer, X. Chen, M. E. Sanders, A. B. Chakravarthy, Y. Shyr and J. A. Pietenpol, J. Clin. Invest., 2011, 121, 2750–2767 CrossRef CAS PubMed.
- G. Landskron, M. De La Fuente, P. Thuwajit, C. Thuwajit and M. A. Hermoso, J. Immunol. Res., 2014, 2014, 1–19 CrossRef PubMed.
- X. Chen, D. Xu, X. Li, J. Zhang, W. Xu, J. Hou, W. Zhang and J. Tang, J. Cancer, 2019, 10, 6608–6617 CrossRef CAS PubMed.
- H. Kuhl and H. P. G. Schneider, Climacteric, 2013, 16, 54–68 CrossRef CAS PubMed.
- R. Blamey, J. Collins, P. G. Crosignani, E. Diczfalusy, L. A. J. Heinemann, C. La Vecchia, G. Reeves, I. E. Smith, D. Trichopoulos, E. Arisi, D. T. Baird, G. Benagiano, G. C. Frigerio, A. Glasier, K. Schmidt-Gollwitzer, S. O. Skouby and A. Volpe, Hum. Reprod. Update, 2004, 10, 281–293 CrossRef PubMed.
- Z. Y. Wang and L. Yin, Mol. Cell. Endocrinol., 2015, 418, 193–206 CrossRef CAS PubMed.
- J. Kiani, A. Khan, H. Khawar, F. Shuaib and S. Pervez, Pathol. Oncol. Res., 2006, 12, 223–227 CrossRef.
- N. Jiang, J.-J. Lin, J. Wang, B.-N. Zhang, A. Li, Z.-Y. Chen, S. Guo, B.-B. Li, Y.-Z. Duan, R.-Y. Yan, H.-F. Yan, X.-Y. Fu, J.-L. Zhou, H.-M. Yang and Y. Cui, Exp. Ther. Med., 2018, 16, 2183–2192 Search PubMed.
- O. Martínez-Sáez and A. Prat, JCO Oncol. Pract., 2021, 17, 594–604 CrossRef.
- L. Guo, W. Shao, C. Zhou, H. Yang, L. Yang, Q. Cai, J. Wang, Y. Shi, L. Huang and J. Zhang, Mol. Med., 2023, 29, 134 CAS.
- J. Xu, L. Huang and G. P. Sun, Sci. Rep., 2017, 7, 5353 CrossRef PubMed.
- G. Chilà, V. Guarini, D. Galizia, E. Geuna and F. Montemurro, Drug Des., Dev. Ther., 2021, 15, 2711–2720 CrossRef.
- L. Huang and A. M. Xu, Expert Rev. Anticancer Ther., 2017, 17, 327–334 CrossRef CAS PubMed.
- J. P. Leone and N. U. Lin, Curr. Oncol. Rep., 2019, 21, 49 CrossRef PubMed.
- J. Xu, L. Huang and J. Li, Oncotarget, 2016, 7, 60218–60229 CrossRef PubMed.
- C. Corti, G. Antonarelli, C. Criscitiello, N. U. Lin, L. A. Carey, J. Cortés, P. Poortmans and G. Curigliano, Cancer Treat. Rev., 2022, 103, 102324 CrossRef CAS.
- N. Shah, A. S. Mohammad, P. Saralkar, S. A. Sprowls, S. D. Vickers, D. John, R. M. Tallman, B. P. Lucke-Wold, K. E. Jarrell, M. Pinti, R. L. Nolan and P. R. Lockman, Pharmacol. Res., 2018, 132, 47–68 CrossRef CAS.
- A. Pellerino, V. Internò, F. Mo, F. Franchino, R. Soffietti and R. Rudà, Int. J. Mol. Sci., 2020, 21, 1–26 Search PubMed.
- M. Ban, B. Petrić Miše and E. Vrdoljak, Breast Care, 2020, 15, 560–569 CrossRef PubMed.
- J. Baselga, R. E. Coleman, J. Cortés and W. Janni, Crit. Rev. Oncol. Hematol., 2017, 119, 113–122 CrossRef.
- H. R. Kourie, E. El Rassy, F. Clatot, E. de Azambuja and M. Lambertini, OncoTargets Ther., 2017, 10, 3363–3372 CrossRef.
- S. Loibl, P. Poortmans, M. Morrow, C. Denkert and G. Curigliano, Lancet, 2021, 397, 1750–1769 CrossRef CAS.
- M. Martin and S. López-Tarruella, Am. Soc. Clin. Oncol. Educ. Book, 2016, 36, e64–e70 CrossRef PubMed.
- M. A. Binmujlli, Processes, 2024, 12, 1659 CrossRef.
- D. Bilancia, G. Rosati, A. Dinota, D. Germano, R. Romano and L. Manzione, Ann. Oncol., 2007, 18, vi26–vi30 CrossRef.
- S. R. D. Johnston, R. Hegg, S. A. Im, I. H. Park, O. Burdaeva, G. Kurteva, M. F. Press, S. Tjulandin, H. Iwata, S. D. Simon, S. Kenny, S. Sarp, M. A. Izquierdo, L. S. Williams and W. J. Gradishar, J. Clin. Oncol., 2021, 39, 79–89 CrossRef CAS.
- L. S. Schwartzberg, S. X. Franco, A. Florance, L. O’Rourke, J. Maltzman and S. Johnston, Oncologist, 2010, 15, 122–129 CrossRef PubMed.
- S. Dhillon, Clin. Drug Invest., 2019, 39, 221–229 CrossRef CAS.
- G. Iancu, D. Serban, C. D. Badiu, C. Tanasescu, M. S. Tudosie, C. Tudor, D. O. Costea, A. Zgura, R. Iancu and D. Vasile, Exp. Ther. Med., 2022, 23, 114 CrossRef CAS PubMed.
- S. López-Tarruella, Y. Jerez, I. Márquez-Rodas and M. Martín, Future Oncol., 2012, 8, 671–681 CrossRef PubMed.
- A. Nasrazadani and A. Brufsky, Future Oncol., 2020, 16, 247–254 CrossRef CAS.
- M. S. Dai, Y. H. Feng, S. W. Chen, N. Masuda, T. Yau, S. T. Chen, Y. S. Lu, Y. S. Yap, P. C. S. Ang, S. C. Chu, A. Kwong, K. S. Lee, S. Ow, S. B. Kim, J. Lin, H. C. Chung, R. Ngan, V. C. Kok, K. M. Rau, T. Sangai, T. Y. Ng, L. M. Tseng, R. Bryce, J. Bebchuk, M. C. Chen and M. F. Hou, Breast Cancer Res. Treat., 2021, 189, 665–676 CrossRef CAS PubMed.
- R. Paranjpe, D. Basatneh, G. Tao, C. De Angelis, S. Noormohammed, E. Ekinci, S. Abughosh, R. Ghose and M. V. Trivedi, Ann. Pharmacother., 2019, 53, 612–620 CrossRef CAS PubMed.
- J. W. Park, M. C. Liu, D. Yee, C. Yau, L. J. van’t Veer, W. F. Symmans, M. Paoloni, J. Perlmutter, N. M. Hylton, M. Hogarth, A. DeMichele, M. B. Buxton, A. J. Chien, A. M. Wallace, J. C. Boughey, T. C. Haddad, S. Y. Chui, K. A. Kemmer, H. G. Kaplan, C. Isaacs, R. Nanda, D. Tripathy, K. S. Albain, K. K. Edmiston, A. D. Elias, D. W. Northfelt, L. Pusztai, S. L. Moulder, J. E. Lang, R. K. Viscusi, D. M. Euhus, B. B. Haley, Q. J. Khan, W. C. Wood, M. Melisko, R. Schwab, T. Helsten, J. Lyandres, S. E. Davis, G. L. Hirst, A. Sanil, L. J. Esserman and D. A. Berry, N. Engl. J. Med., 2016, 375, 11–22 CrossRef CAS.
- E. Stoen, J. Kagihara, E. Shagisultanova, C. M. Fisher, A. Nicklawsky, P. Kabos, V. F. Borges and J. R. Diamond, Breast Cancer Res. Treat., 2021, 187, 883–891 CrossRef CAS PubMed.
- S. L. Moulder, V. F. Borges, T. Baetz, T. Mcspadden, G. Fernetich, R. K. Murthy, R. Chavira, K. Guthrie, E. Barrett and S. K. Chia, Clin. Cancer Res., 2017, 23, 3529–3536 CrossRef CAS.
- G. R. R. Ricciardi, A. Russo, T. Franchina, S. Schifano, G. Mastroeni, A. Santacaterina and V. Adamo, BMC Cancer, 2018, 18, 97 CrossRef PubMed.
- V. Dinkel, D. Anderson, S. Winski, J. Winkler, K. Koch and P. A. Lee, Cancer Res., 2012, 72, 852 CrossRef.
- A. Kulukian, P. Lee, J. Taylor, R. Rosler, P. De Vries, D. Watson, A. Forero-Torres and S. Peterson, Mol. Cancer Ther., 2020, 19, 976–987 CrossRef CAS.
- S. Kunte, J. Abraham and A. J. Montero, Cancer, 2020, 126, 4278–4288 CrossRef CAS PubMed.
- C. C. O’Sullivan, N. N. Davarpanah, J. Abraham and S. E. Bates, Semin. Oncol., 2017, 44, 85–100 CrossRef.
- R. Mariam Raju, J. Joy A, R. Nulgumnalli Manjunathaiah, A. Justin and B. R. Prashantha Kumar, Results Chem., 2024, 7, 101490 CrossRef CAS.
- R. K. Rajan, S. S. Kumar M. and B. Balaji, Pharm.
Biol., 2017, 55, 242–251 CrossRef CAS.
- S. Huang, R. Wang, R. Li, H. Wang, J. Qiao and B. W. J. Mol, Fertil. Steril., 2018, 109, 872–878 CrossRef CAS PubMed.
- K. D. Miller, L. Nogueira, A. B. Mariotto, J. H. Rowland, K. R. Yabroff, C. M. Alfano, A. Jemal, J. L. Kramer and R. L. Siegel, CA. Cancer J. Clin., 2019, 69, 363–385 CrossRef.
- A. Italiano, J. Clin. Oncol., 2011, 29, 4718 CrossRef PubMed.
- W. R. Miller, Semin. Oncol., 2003, 30, 3–11 CrossRef CAS.
- P. E. Goss and K. Strasser, J. Clin. Oncol., 2001, 19, 881–894 CrossRef CAS PubMed.
- L. A. Anthony, D. Rajaraman, G. Sundararajan, M. Suresh, P. Nethaji, R. Jaganathan and K. Poomani, J. Mol. Struct., 2022, 1266, 133483 CrossRef CAS PubMed.
- M. Merajoddin, S. Piri, Z. Mokarian and F. Piri, Asian J. Green Chem, 2021, 5, 335–342 CAS.
- K. T. Schütt, F. Arbabzadah, S. Chmiela, K. R. Müller and A. Tkatchenko, Nat. Commun., 2017, 8, 13890 CrossRef.
- R. Nagai, R. Akashi and O. Sugino, npj Comput. Mater., 2020, 6, 43 CrossRef CAS.
-
M. J. Frisch, G. W. Trucks, H. B. Schlegel, G. E. Scuseria, M. A. Robb, J. R. Cheeseman, G. Scalmani, V. Barone, G. A. Petersson, H. Nakatsuji, M. Caricato, A. V. Marenich, J. Bloino, B. G. Janesko, R. Gomperts, B. Mennucci, H. P. Hratchian, J. V. Ortiz, A. F. Izmaylov, J. L. Sonnenberg, D. Williams-Young, F. Ding, F. Lipparini, F. Egidi, J. Goings, B. Peng, A. Petrone, T. Henderson, D. Ranasinghe, V. G. Zakrzewski, J. Gao, N. Rega, G. Zheng, W. Liang, M. Hada, M. Ehara, K. Toyota, R. Fukuda, J. Hasegawa, M. Ishida, T. Nakajima, Y. Honda, O. Kitao, H. Nakai, T. Vreven, K. Throssell, J. A. Montgomery, Jr., J. E. Peralta, F. Ogliaro, M. J. Bearpark, J. J. Heyd, E. N. Brothers, K. N. Kudin, V. N. Staroverov, T. A. Keith, R. Kobayashi, J. Normand, K. Raghavachari, A. P. Rendell, J. C. Burant, S. S. Iyengar, J. Tomasi, M. Cossi, J. M. Millam, M. Klene, C. Adamo, R. Cammi, J. W. Ochterski, R. L. Martin, K. Morokuma, O. Farkas, J. B. Foresman and D. J. Fox, Gaussian 16 Revision C.01, Gaussian, Inc., Wallingford, CT, 2016.
- A. D. Becke, J. Chem. Phys., 1993, 98, 5648–5652 CrossRef CAS.
-
R. Dennington, T. A. Keith and J. M. Millam, GaussView, Version 6. Semichem Inc. Shawnee Mission KS.
- J. Prashanth, G. Ramesh, J. Laxman Naik, J. Kishan Ojha and B. Venkatram Reddy, Mater. Today: Proc., 2016, 3, 3761–3769 Search PubMed.
- R. G. Parr and R. G. Pearson, J. Am. Chem. Soc., 1983, 105, 7512–7516 CrossRef CAS.
- J. P. Perdew and M. Levy, Phys. Rev. Lett., 1983, 51, 1884–1887 CrossRef CAS.
- V. Enisoglu Atalay and S. Asar, Eur. Chem. Biotechnol. J., 2024, 1, 27–38 CrossRef.
- F. N. Ajeel, M. H. Mohammed and A. M. Khudhair, Russ. J. Phys. Chem. B, 2017, 11, 850–858 CrossRef CAS.
- S. Bagheri Novir and M. R. Aram, Chem. Phys. Lett., 2020, 757, 137869 CrossRef CAS PubMed.
- S. Celik, S. Akyuz and A. E. Ozel, Vib. Spectrosc., 2017, 92, 287–297 CrossRef CAS.
- S. Celik, A. T. Albayrak, S. Akyuz, A. E. Ozel and B. D. Sigirci, J. Biomol. Struct. Dyn., 2021, 39, 2376–2386 CrossRef CAS.
- Z. Akbari, C. Stagno, N. Iraci, T. Efferth, E. A. Omer, A. Piperno, M. Montazerozohori, M. Feizi-Dehnayebi and N. Micale, J. Mol. Struct., 2024, 1301, 137400 CrossRef CAS.
- V. Rani, B. Pal Singh and A. Kumar, Nano LIFE, 2023, 13, 2350011 CrossRef CAS.
- M. Priya, A. Zochedh, K. Chandran, K. Arumugam, S. Banu, C. Chakaravarthy and A. B. Sultan, Lett. Appl. NanoBioScience, 2023, 12, 165 Search PubMed.
- L. R. Domingo and P. Pérez, Org. Biomol. Chem., 2011, 9, 7168–7175 RSC.
- N. Sepay, N. Sepay, A. Al Hoque, R. Mondal, U. C. Halder and M. Muddassir, Struct. Chem., 2020, 31, 1831–1840 CrossRef CAS PubMed.
- K. B. Benzon, H. T. Varghese, C. Y. Panicker, K. Pradhan, B. K. Tiwary, A. K. Nanda and C. Van Alsenoy, Spectrochim. Acta, Part A, 2015, 151, 965–979 CrossRef CAS PubMed.
- V. Darugar, M. Vakili, S. F. Tayyari and F. S. Kamounah, J. Mol. Graphics Modell., 2021, 107, 107976 CrossRef CAS PubMed.
- B. H. Stuart, Infrared Spectrosc. Fundam. Appl., 2005, 1–224 Search PubMed.
- V. Arjunan and S. Mohan, Spectrochim. Acta, Part A, 2009, 72, 436–444 CrossRef CAS PubMed.
-
J. Coates, Encyclopedia of Analytical Chemistry, Wiley, 2000 Search PubMed.
-
A. Segneanu, I. Gozescu, A. Dabici and P. Sfirloaga, Organic compounds FT-IR spectroscopy, 2012 Search PubMed.
-
A. Segneanu, I. Gozescu, A. Dabici and P. Sfirloaga, Organic compounds FT-IR spectroscopy, 2012 Search PubMed.
- P. Banerjee, A. O. Eckert, A. K. Schrey and R. Preissner, Nucleic Acids Res., 2018, 46, W257–W263 CrossRef CAS PubMed.
- E. A. Perez, M. Koehler, J. Byrne, A. J. Preston, E. Rappold and M. S. Ewer, Mayo Clin. Proc., 2008, 83, 679–686 CrossRef.
- T. E. Lacourt and C. J. Heijnen, Curr. Breast Cancer Rep., 2017, 9, 70–81 CrossRef PubMed.
- L. Chap, R. Shpiner, M. Levine, L. Norton, M. Lill and J. Glaspy, Bone Marrow Transplant., 1997, 20, 1063–1067 CrossRef CAS.
- N. B. Fernandes, V. Velagacherla, K. J. Spandana, N. Bhagya, C. H. Mehta, S. Gadag, J. N. Sabhahit and U. Y. Nayak, Int. J. Pharm., 2024, 650, 123686 CrossRef CAS.
- T. Kacan, A. Altun, G. G. Altun, S. B. Kacan, B. Sarac, M. M. Seker, A. Bahceci and N. Babacan, Asian Pacific J. Cancer Prev., 2014, 15, 3185–3189 CrossRef.
- B. Liao, S. Shroff, C. Kamiya-Matsuoka and S. Tummala, Neuro-Oncol., 2014, 16, 589 CrossRef CAS.
- J. B. Stone and L. M. DeAngelis, Nat. Rev. Clin. Oncol., 2016, 13, 92–105 CrossRef CAS PubMed.
- S. R. Plotkin and P. Y. Wen, Neurol. Clin., 2003, 21, 279–318 CrossRef PubMed.
- M. Yang, J. S. Kim, J. Kim, S. H. Kim, J. C. Kim, J. Kim, H. Wang, T. Shin and C. Moon, Biochem. Pharmacol., 2011, 82, 72–80 CrossRef CAS PubMed.
- A. S. Jaggi and N. Singh, Toxicology, 2012, 291, 1–9 CrossRef CAS PubMed.
- L. Crews, E. Rockenstein and E. Masliah, Brain Struct. Funct., 2010, 214, 111 CrossRef CAS PubMed.
- J. Galica, D. Rajacich, D. Kane and G. R. Pond, Clin. J. Oncol. Nurs., 2012, 16, 163–169 CrossRef PubMed.
- Q. Chen, J. Xiong, Y. Ma, J. Wei, C. Liu and Y. Zhao, Front. Oncol., 2023, 12, 1086821 CrossRef.
- M. Amin, A. Pourshohod, A. Kheirollah, M. Afrakhteh, F. Gholami-Borujeni, M. Zeinali and M. Jamalan, J. Drug Delivery Sci. Technol., 2018, 47, 209–214 CrossRef CAS.
- G. M. Morris, H. Ruth, W. Lindstrom, M. F. Sanner, R. K. Belew, D. S. Goodsell and A. J. Olson, J. Comput. Chem., 2009, 30, 2785–2791 CrossRef CAS PubMed.
- B. Ni, H. Wang, H. K. S. Khalaf, V. Blay and D. R. Houston, J. Chem. Inf. Model., 2024, 64, 3779–3789 CrossRef CAS PubMed.
- R. R. Love, J. Clin. Oncol., 1989, 7, 803–815 CrossRef CAS PubMed.
- C. Saura, M. Oliveira, Y. H. Feng, M. S. Dai, S. W. Chen, S. A. Hurvitz, S. B. Kim, B. M. Moy, S. Delaloge, W. Gradishar, N. Masuda, M. Palacova, M. E. Trudeau, J. Mattson, Y. S. Yap, M. F. Hou, M. De Laurentiis, Y. M. Yeh, H. T. Chang, T. Yau, H. Wildiers, B. Haley, D. Fagnani, Y. S. Lu, J. Crown, J. Lin, M. Takahashi, T. Takano, M. Yamaguchi, T. Fujii, B. Yao, J. Bebchuk, K. Keyvanjah, R. Bryce and A. Brufsky, J. Clin. Oncol., 2020, 38, 3138–3149 CrossRef CAS PubMed.
- R. K. Murthy, S. Loi, A. Okines, E. Paplomata, E. Hamilton, S. A. Hurvitz, N. U. Lin, V. Borges, V. Abramson, C. Anders, P. L. Bedard, M. Oliveira, E. Jakobsen, T. Bachelot, S. S. Shachar, V. Müller, S. Braga, F. P. Duhoux, R. Greil, D. Cameron, L. A. Carey, G. Curigliano, K. Gelmon, G. Hortobagyi, I. Krop, S. Loibl, M. Pegram, D. Slamon, M. C. Palanca-Wessels, L. Walker, W. Feng and E. P. Winer, N. Engl. J. Med., 2020, 382, 597–609 CrossRef CAS.
- K. Feldinger and A. Kong, Breast Cancer: Targets Ther., 2015, 7, 147–162 Search PubMed.
- M. Z. Kabir, A. K. Mukarram, S. B. Mohamad, Z. Alias and S. Tayyab, J. Photochem. Photobiol., B, 2016, 160, 229–239 CrossRef CAS.
- M. Priya, A. Zochedh, K. Chandran, K. Arumugam, S. Banu, C. Chakaravarthy and A. B. Sultan, Lett. Appl. NanoBioScience, 2023, 12, 165 Search PubMed.
- A. Şandor, I. Ionuţ, G. Marc, I. Oniga, D. Eniu and O. Oniga, Pharmaceuticals, 2023, 16, 534 CrossRef.
- S. Verma, S. Goyal, A. Kumari, A. Singh, S. Jamal and A. Grover, PLoS One, 2018, 13, e0190942 CrossRef PubMed.
- I. Schlam and S. M. Swain, npj Breast Cancer, 2021, 7, 1–12 CrossRef.
-
A. Peters and P. Tadi, Growth Disord, 2nd edn, 2023, pp. 626–637 Search PubMed.
|
This journal is © The Royal Society of Chemistry 2025 |
Click here to see how this site uses Cookies. View our privacy policy here.