DOI:
10.1039/D0SC06528E
(Edge Article)
Chem. Sci., 2021,
12, 4722-4739
Molecular recognition of SARS-CoV-2 spike glycoprotein: quantum chemical hot spot and epitope analyses†
Received
27th November 2020
, Accepted 21st February 2021
First published on 2nd March 2021
Abstract
Due to the COVID-19 pandemic, researchers have attempted to identify complex structures of the severe acute respiratory syndrome coronavirus 2 (SARS-CoV-2) spike glycoprotein (S-protein) with angiotensin-converting enzyme 2 (ACE2) or a blocking antibody. However, the molecular recognition mechanism—critical information for drug and antibody design—has not been fully clarified at the amino acid residue level. Elucidating such a microscopic mechanism in detail requires a more accurate molecular interpretation that includes quantum mechanics to quantitatively evaluate hydrogen bonds, XH/π interactions (X = N, O, and C), and salt bridges. In this study, we applied the fragment molecular orbital (FMO) method to characterize the SARS-CoV-2 S-protein binding interactions with not only ACE2 but also the B38 Fab antibody involved in ACE2-inhibitory binding. By analyzing FMO-based interaction energies along a wide range of binding interfaces carefully, we identified amino acid residues critical for molecular recognition between S-protein and ACE2 or B38 Fab antibody. Importantly, hydrophobic residues that are involved in weak interactions such as CH–O hydrogen bond and XH/π interactions, as well as polar residues that construct conspicuous hydrogen bonds, play important roles in molecular recognition and binding ability. Moreover, through these FMO-based analyses, we also clarified novel hot spots and epitopes that had been overlooked in previous studies by structural and molecular mechanical approaches. Altogether, these hot spots/epitopes identified between S-protein and ACE2/B38 Fab antibody may provide useful information for future antibody design, evaluation of the binding property of the SARS-CoV-2 variants including its N501Y, and small or medium drug design against the SARS-CoV-2.
1. Introduction
Coronavirus disease 2019 (COVID-19), caused by the severe acute respiratory syndrome coronavirus 2 (SARS-CoV-2), has been declared a pandemic by the World Health Organization and has caused worldwide social and economic problems.1 Despite its significant infectious strength and the worldwide research efforts, to date, no specific treatment has been established against this new virus. The SARS-CoV-2 is composed of a variety of proteins, including the spike glycoprotein (S-protein),2 which is believed to promote the invasion of host cells and the proliferation of the virus by binding to human angiotensin-converting enzyme 2 (ACE2) (Fig. 1A). The SARS-CoV-2 S-protein monomer comprises nine domains/regions: N-terminal domain (NTD), receptor-binding domain (RBD), subdomain 1 (SD1), subdomain 2 (SD2), fusion peptide (FP), heptad repeat 1 (HR1), heptad repeat 2 (HR2), transmembrane region (TM), and intracellular domain (IC) (Fig. 1B). The RBD domain plays a key role in cell infection via ACE2 recognition. Therefore, the SARS-CoV-2 S-protein is one of the target proteins used for antibody-drug design to prevent virus invasion, including S-protein neutralizing antibodies.2–5 To tackle the SARS-CoV-2 pandemic, researchers are extracting and designing neutralizing antibodies to block the binding of S-protein to ACE2.2–5 Understanding the binding mode of S-protein with ACE2 and existing neutralizing antibodies will help design potent neutralizing antibodies. Moreover, a better understanding of the molecular recognition differences of ACE2 among the SARS-CoV-2, its variant and other coronaviruses including SARS-CoV can improve the antibody-drug design. Several SARS-CoV-2 variants have already emerged and are rampant throughout the world.6–8 Thus, certain X-ray crystallographic and electron microscopic analyzes have already been performed, and geometry-based interactions have been discussed in previous studies.3,9–13
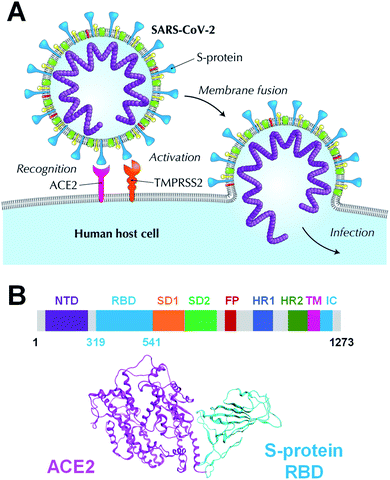 |
| Fig. 1 Human host cell infection via ACE2 recognition by SARS-CoV-2 S-protein. (A) ACE2 is the host cell receptor responsible for mediating the SARS-CoV-2 infection, the new coronavirus responsible for coronavirus disease 2019 (COVID-19). (B) Overall topology of the SARS-CoV-2 S-protein monomer that comprises an N-terminal domain (NTD), receptor-binding domain (RBD), subdomain 1 (SD1), subdomain 2 (SD2), fusion peptide (FP), heptad repeat 1 (HR1), heptad repeat 2 (HR2), transmembrane region (TM), and an intracellular domain (IC). A complex between the SARS-CoV-2 S-protein and ACE2 is shown in cyan and magenta ribbon models. | |
Analyses of molecular recognition hots spots between the SARS-CoV-2 S-protein and ACE2 were conducted by several computational simulations, including electrostatic potential,14,15 molecular mechanics (MM)-based interaction energy analysis with classical force field,16 and quantum mechanics (QM)-based interaction energy analysis with or without evaluation of semiempirical dispersion energy.17–19 Molecular dynamics (MD) simulations for the complex formed by the S-protein and ACE2 were conducted to better understand their association.20–26 Approaches for drug/neutralizing antibody design targeting S-protein have been reported, such as a de novo design peptide inhibitors for SARS-CoV-2,27,28 a virtual screening of antiviral compounds for the SARS-CoV-2 (ref. 29) and sequence-based epitope analysis for the SARS-CoV-2, among others.30 These previous studies have been based on the molecular recognition of S-protein and ACE2/antibody and were highly beneficial. These studies had focused on more on hydrogen bonds, which certainly play key roles in determining binding poses of a protein–protein interaction (PPI) system, than on hydrophobic interactions, which influence the binding affinity.31 Even when hydrophobic interactions were considered, they were limited by the non-quantitative or inaccurate evaluation using a structure-based, MM-based, or semi-empirical based analysis. Moreover, in the prediction of binding ability, the enthalpic interactions, such as electrostatic and dispersion interactions, as well as the effects of solvation and sugar chain have not been examined sufficiently. Therefore, we used ab initio electron-correlated QM theory32–35 that yields rigorous dispersion energy in order to quantitatively and accurately evaluate hydrophobic interactions such as CH/π interactions.
The QM calculation of whole protein system consisting of hundreds to thousands of residues can be conducted using the fragment molecular orbital (FMO) approach.36–39 The hydrogen bonds, electrostatic interactions, salt bridges, and hydrophobic interactions were quantitatively analyzed using the inter-fragment interaction energy (IFIE) and its energy decomposition analysis (PIEDA) based on FMO calculations.40–42 In recent years, the FMO method has been widely used as a drug discovery tool.35,43–52 Recent studies have also provided detailed information on the underlying antigen–antibody or ligand-binding characteristics of several drug-targeted proteins, main protease,53–55 ribonucleic acid (RNA)-dependent RNA polymerase,56 S-protein,17,19 in COVID-19. To date, the FMO method has been successfully used for identifying key amino acid residues and sugar chains for PPI, such as molecular recognition for an influenza hemagglutinin of a fragment antigen-binding (Fab) antibody57–67 and a measles hemagglutinin of a signaling lymphocytic activation molecule.68,69 In addition, it is essential to combine desolvation energy and enthalpic binding energy to predict binding ability.43,51 Therefore, the present study aimed to address additional calculations that could shed light on the molecular process underlying the SARS-CoV-2, the SARS-CoV-2 variant and SARS-CoV S-protein recognition by ACE2 or B38 Fab antibody, which remains to be understood in more detail, in particularly concerning hydrophobic interactions such as CH/π interactions. In particular, the study explored the amino acid residues involved in, as well as types of interactions that make, hot spots for S-protein binding to ACE2. This FMO-based hot spot and epitope analysis can be applied to other PPI systems in drug-antigen design as well.
2. Computational methods
To clarify the important interactions between the SARS-CoV-2 S-protein and ACE2, interaction energy analysis was performed according to the procedures described below.
2.1 Preparation of the SARS-CoV-2 S-protein and ACE2 complex
We retrieved the crystal structures of the complexes formed by the SARS-CoV-2 S-protein with ACE2 and B38 Fab antibody from the protein data bank (PDB) repository (with its codes of 6LZG and 7BZ5, respectively) for the FMO calculation. To compare the epitope candidate of S-proteins related to the SARS-CoV-2, FMO calculations were also performed for complexes between SARS-CoV-2 chimeric and SARS-CoV S-proteins with ACE2 (PDB IDs: 6VW1 and 2AJF, respectively). We would consider that the binding interface with the ACE2/B38 Fab antibody was robust because the amino acid residues on the binding surface in X-ray crystal structures almost overlap well (Fig. S1†). Thus, we performed the calculations and analysis employing prepared structures based on the X-ray crystal structures. Here, it will consider that the monomer structure of a complex between RBD and ACE2/antibody is suitable as a model to analyze molecular recognition. Although the S-protein existing on the virus surface basically forms a trimer structure,13 each RBD of S-protein trimer in the case of an open structure does not retain intramolecular interactions between another monomer of S-protein.67 Since the trimer is almost observed as the open structure when it binds to ACE2 or an antibody, the monomer model will be appropriate to analyze molecular recognition between the S-protein and the ACE2/antibody.
The structures of the different viral protein/ACE2 or B38 Fab antibody complexes are shown in Fig. 2. All sugar chains and crystal water molecules were retained. Since Cl− and Zn2+ ions existed far away from the binding surface between the SARS-CoV-2 S-protein and ACE2, they were deleted to simplify the interaction analysis. The missing atoms and missing residues were complemented by structure preparation with the Molecular Operating Environment (MOE) graphical software package (Chemical Computing Group, Montreal, QC, Canada).70 Hydrogen atoms were added to each complex using Protonate 3D with MOE, and sequences termini were capped with amine (–NH2) and carboxylic acid (–COOH) groups. These molecular changes were optimized concurrently using the Amber10:EHT force field with MOE.
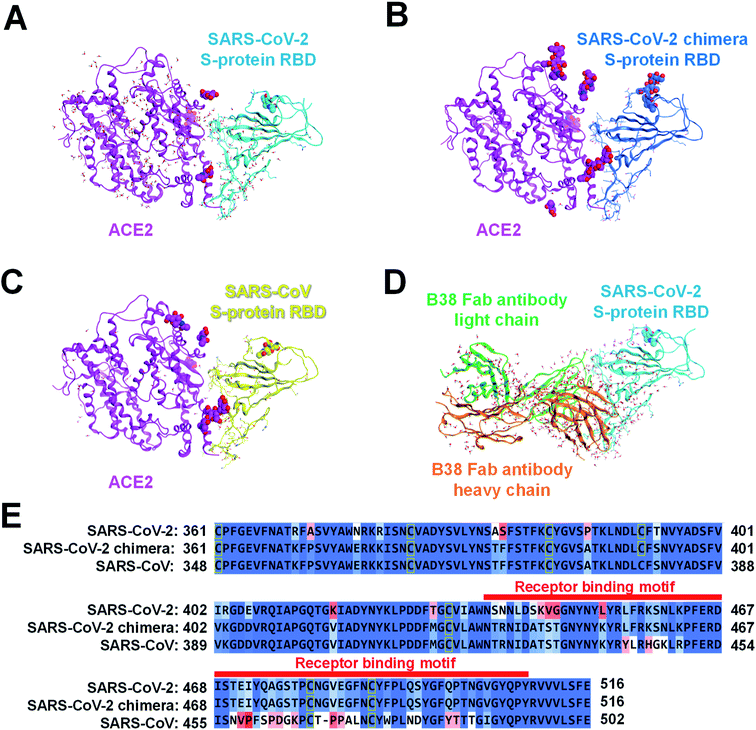 |
| Fig. 2 Complexes of several S-proteins with ACE2 or B38 Fab antibody. The complexed structure of ACE2 (magenta ribbon; Chain A: seqs#19–615, 19–614, and 19–615 in (A), (B), and (C), respectively) with each S-protein is depicted using a ribbon model, and residues whose sequences differ between the three S-proteins are shown in a stick model. The crystal water molecules are shown by the ball and stick model, and the sugar chains are shown by the CPK space-filling model. The S-protein of (A) SARS-CoV-2 (Chain B: seq#333–527), (B) SARS-CoV-2 chimera (Chain E: seq#334–527), and (C) SARS-CoV (Chain E: seq#323–502) are illustrated in the cyan, blue, yellow ribbon model (PDB IDs: 6LZG, 6VW1, and 2AJF), respectively. (D) A complex of B38 Fab antibody (orange ribbon, Chain H, seq#0–217; green ribbon, Chain L, seq#0–215) with SARS-CoV-2 S-protein (cyan ribbon, Chain A, seq#334–528) is shown. (E) In sequence alignment of the SARS-CoV-2, SARS-CoV-2 chimeric, and SARS-CoV S-proteins, the well-aligned and poorly aligned residues are shown in color gradation as blue and red, respectively, evaluated using the BLOSUM62 scoring matrix.70 A receptor binding motif on the binding surface with ACE2 is shown by a red belt. | |
Considering the common sequence of the SARS-CoV-2 (C361–E516) and the SARS-CoV (C348–E502), 50 and 36 amino acid mutations were identified in the common sequence and the receptor binding motif, respectively (Fig. 2E). Sequence homology between the SARS-CoV-2 and the SARS-CoV S-proteins was 84.4% for the whole Pro337–Phe515 and 47.2% for Tyr453–Tyr505. The sugar chain located on the SARS-CoV-2 chimeric/SARS-CoV S-protein and ACE2 binding surface was the only β-D-mannopyranose (BMA) extending from the side chain of N90 on ACE2 (Fig. 2B and C). The BMA sugar chain consists of the terminal chain in the N-acetyl-β-D-glucosamine (NAG) and the BMA sugar chains (NAG–NAG–BMA) on N90ACE2. These X-ray structures of ACE2 have a common sequence (S19–A614).
2.2 FMO method and intermolecular interaction energy analysis
The ab initio FMO method used was as follows. Briefly, a large molecule or molecular cluster was divided into small fragments and the molecular orbital (MO) calculations for each fragmented monomer and dimer were performed to obtain the properties of the entire system. The many-body effects were considered through the environmental electrostatic potentials. The total energies of the FMO calculations were given by: | 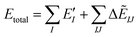 | (1) |
where
represents the monomer energy without the environmental electrostatic potential (ΔẼIJ) of the IFIE; and I and J are the fragment indices. In addition, PIEDA was used to decompose IFIE into its energy components ΔẼIJ including the electrostatic (ES), exchange–repulsion (EX), dispersion interaction (DI), and charge transfer with higher-order mixed terms (CT+mix), according to: | ΔẼIJ = ΔẼESIJ + ΔẼEXIJ + ΔẼCT+mixIJ + ΔẼDIIJ | (2) |
Summation of IFIEs (IFIE-sum) over a set of multiple fragments (A) with a fragment J is expressed as following:
|  | (3) |
By analyzing IFIE-sum over a target protein, one can extract amino acid residues that specifically interact with the target protein, on its binding protein. Moreover, summing over a set of fragments (B) as
| 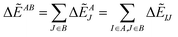 | (4) |
one can obtain the interaction energy between
A and
B sets which is used as the binding energy between two proteins in this study.
All FMO calculations were performed using the second-order Møller–Plesset perturbation theory (MP2) with 6-31G* basis set using the ABINIT-MP program.38,71,72 The MP2 electron-correlation energy corresponds to the DI term and can accurately and quantitatively evaluate CH/π and π–π interactions that cannot be sufficiently evaluated using the MM and semi-empirical methods. A Cholesky decomposition integral approximation73 was applied to speed up the MP2 calculation while keeping the accuracy. The fragment unit for proteins was each amino acid residue and each sugar chain. During the standard fragmentation process it is desirable to cleave at a sp3 hybridized single bond; therefore, single amino acid residues were cleaved between Cα and C
O in the main chain rather than at the sp2 hybridized amide bond. Interaction energy between amino acid residues was evaluated using IFIE analysis with BioStation Viewer.74 The FMO calculation results of the PDB ID entries 6LZG, 6VW1, 2AJF, and 7BZ5 are registered in the FMO database (FMODB; https://drugdesign.riken.jp/FMODB/)75–77 with its codes (FMODB IDs) as 4NZVN, KR5L3, 596VZ, and Q86GY, respectively.
2.3 Geometric interaction fingerprint analysis
The hydrogen-bond, ion pair, and π-orbital interactions, such as XH/π, cation/π, and π–π interactions (Fig. 3), were detected by geometric interaction fingerprint (IFP) analysis based on the detected interatomic distance and angle using the “prolig_Calculate” function of the MOE software.78 MM-based energy restrictions defined by the “prolig_Calculate” function were employed to extract all possible atom pairs.
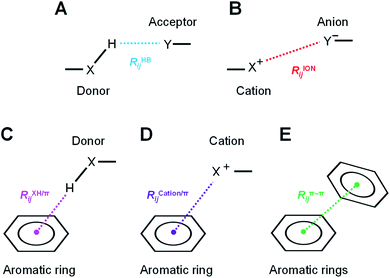 |
| Fig. 3 Types of intermolecular and intramolecular interactions by geometric interaction fingerprint (IFP). Schematic diagrams of the (A) XH–Y hydrogen bond, (B) ion pair interaction, (C) XH/π interaction, (D) cation/π interaction, and (E) π–π interaction, respectively. Characteristic distances of each interaction were measured between detected heavy atoms or centroids of aromatic rings. | |
3. Results and discussion
3.1. How does the SARS-CoV-2 S-protein recognize ACE2 in human host cells?
In a first approach, a detailed structural analysis was performed to elucidate which key amino acid residues are important for the molecular recognition of ACE2 by the SARS-CoV-2 S-protein, as well as which types of interactions were involved. Such information could help identify epitope candidates for novel antibodies to prevent the binding between the S-protein and ACE2. FMO-based interaction energy and geometric IFP analyses of the S-protein and ACE2 were performed.
To determine key residues for molecular recognition, it is necessary to identify amino acid residue pairs with attractive interactions between proteins. IFIE and IFIE-sum analyses and their energy decomposition analyses with PIEDA are useful for quantitatively evaluating interaction energies such as hydrogen bonds and CH/π interactions. In 3.1, 3.2, and 3.3, IFIE-sums over S-protein and ACE2 were calculated using a common amino acid sequence aligned to compare among complexes because the X-ray crystal structures were composed of different lengths of amino acid sequences. Besides, the IFIE-sums did not include IFIEs of sugar chains and water molecules. Fig. 4 shows IFIE-sums of the SARS-CoV-2 S-protein (C361–E516) and ACE2 (S19–A614) using PIEDA. The data revealed that the ES components were dominant in their binding. Six acidic residues (D30, E35, E37, D38, E329, and D355) of ACE2 and five basic residues of the S-protein (R403, R408, K417, R457, and K458) showed a remarkable attractive interaction. These results were considered to be caused by total charges of molecular systems on ACE2 and S-protein of −26e and +2e, respectively. In the CT+mix component, attractive interactions were shown by 10 fragment residues of ACE2 (Q24, D30, K31, H34, D38, Y41, Q42, Y83, K353, and G354) and nine residues of the S-protein (K417, Y449, F456, F486, N487, Q493, T500, N501, and G502). In the case of the DI components, characteristic interactions were identified on 15 residue fragments of ACE2 (Q24, T27, F28, D39, K31, H34, E35, D38, Y41, Q42, M82, Y83, K353, G354, and D355) and 14 residue fragments of the S-protein (K417, Y449, Y453, K455, F456, G476, F487, Y489, Q493, Q498, T500, N501, G502, and Y505). Since these amino acid residues are considered to play key roles in the molecular recognition between ACE2 and the S-protein, the origin of the interaction energy of each fragment pair was further explored (Fig. 5, Section S2†). Nonetheless, it is important to note that in FMO analysis, in general, the ES component is effective over a long distance and tends to be overestimated as compared with the CT and DI ones. Thus, in IFIE-sums with a charged fragment, the weak CT and DI components that show hydrogen bonding and CH/π interaction tend to be hidden by the ES one. Moreover, a direct comparison of the interaction strengths of the charged fragment pair and the neutral one can be challenging using IFIEs that include the ES components. Therefore, herein, the study focused on the amino acid residues contributing for attractive interactions in the CT and DI components caused by short-range interactions, such as hydrogen bonds and XH/π interactions, to reveal the molecular recognition mechanism on the interface between the SARS-CoV-2 S-protein and ACE2.
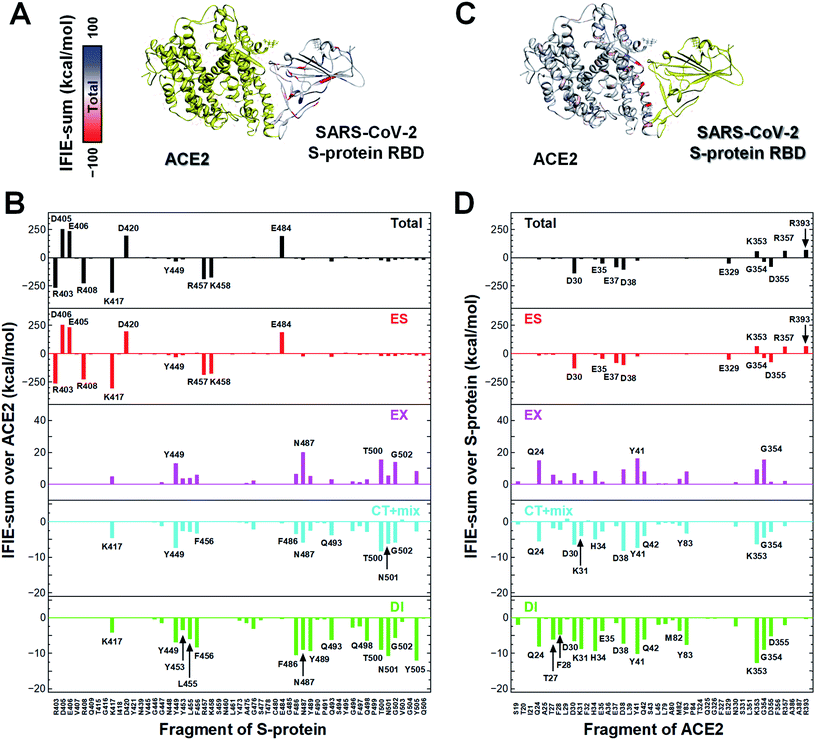 |
| Fig. 4 Intermolecular interaction energies between the SARS-CoV-2 S-protein and ACE2 in host cells. The IFIE-sums over (A and B) ACE2 (S19–A614) and (C and D) S-protein (C361–E516) with each amino acid residue near the binding surface of the S-protein and ACE2 are shown. Visualization of IFIE-sums over the fragments of ACE2 (A) and SARS-CoV-2 S-protein (C) with attractive and repulsive interactions are represented by red and blue, respectively. Sugar chains are depicted by the ball and stick model. Figures (B) and (D) illustrate the IFIE-sums over ACE2 and SARS-CoV-2 S-protein, respectively, total, electrostatic (ES), exchange–repulsion (EX), charge transfer with higher-order mixed terms (CT+mix), and dispersion interaction (DI) components at the amino acid residue. The residue names of the corresponding fragments with interaction energies of ES, CT+mix, and DI components lower than −30, −3, and −3 kcal mol−1, respectively, are shown. | |
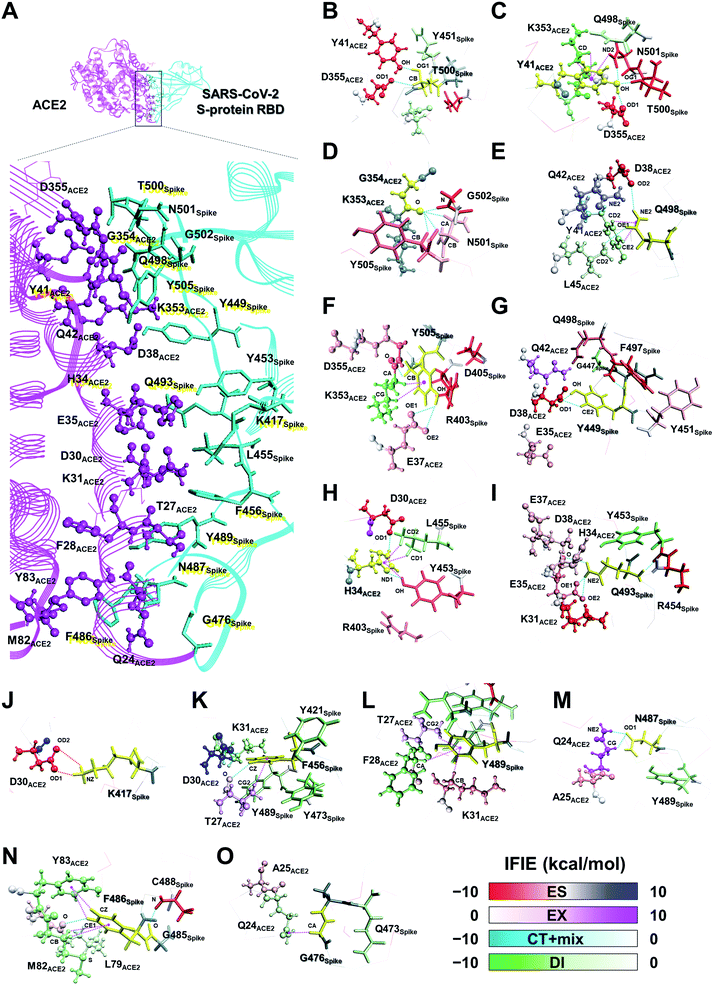 |
| Fig. 5 3D visualization of the interaction energies between the SARS-CoV-2 S-protein amino acid residues near the contact surface with ACE2 (A). The fragments of T500Spike (B), Y41ACE2 (C), G354ACE2 (D), Q498Spike (E), Y505Spike (F), Y449Spike (G), H34ACE2 (H), Q493Spike (I), K417Spike (J), F456Spike (K), Y489Spike (L), N487Spike (M), F486Spike (N), and G476Spike (O) are shown in yellow. The main components of the attractive and repulsive interaction energies are represented by the following color scheme: ES component, red and blue; DI component, green and white. The SARS-CoV-2 S-protein and ACE2 are shown using stick and ball model and stick model, respectively. Hydrogen bonds, ion pair interactions, and XH/π interactions are shown by the cyan, red, and magenta dotted lines. | |
Next, the relationship between the energy intensity and the observed interaction was described based on the above the interaction analysis between the SARS-CoV-2 spike and ACE2. Note, a more detailed description of the interactions between the SARS-CoV-2 S-protein and ACE2 based on Fig. 5 was provided in Section S2.†
3.1.1 XH–Y hydrogen bonds.
The heavy atom pairs detected as hydrogen bonds in the geometric IFP and their FMO-based interaction energies are summarized in Table S1.† It was confirmed that the fragments showing attractive interaction energies of ES and CT+mix terms less than −30 and −3 kcal mol−1 (Fig. 4B and D), respectively, were mainly associated with NH–O and OH–O hydrogen bonds with a distance between heavy atoms within 3 Å. The hydrogen bonds between the O oxygen atom of K353ACE2, which was contained within the G354ACE2 fragment, and the hydrogen atom bonded to N nitrogen atom of G502Spike (Fig. 5D), the OD1 oxygen atom of D38ACE2 and the hydrogen atom bonded to OH oxygen atom of Y449Spike (Fig. 5G), the ND1 nitrogen atom of H34ACE2 and the hydrogen atom bonded to OH oxygen atom of Y453Spike (Fig. 5H), and the OD1 oxygen atom of N487Spike and the hydrogen atom bonded to NE2 nitrogen atom of Q24ACE2 (Fig. 5M) were typical examples such attractive interactions. The interaction energy between D30ACE2 and K417Spike was the largest at −116.4 kcal mol−1, where the distance between the heavy atoms of OD1 and OD2 oxygen atoms of D30ACE2 and NZ nitrogen atom of K417Spike (Fig. 5J) were 2.9 and 3.8 Å, respectively. The atom pairs were also the only salt bridge pair between the S-protein and ACE2 that was detected from the geometric IFP. It was confirmed that these amino acid residues forming hydrogen bonds had attractive interaction energy lower than −5 kcal mol−1, even in the ES component.
On the other hand, although weaker than the interaction energies of the NH–O and OH–O hydrogen bonds, CH–O hydrogen bonds also showed an attractive interaction energy that contributed to the molecular recognition of the SARS-CoV-2 S-protein and ACE2, such as the oxygen and carbon atom pairs for the O oxygen atom of T27ACE2, which was contained within the F28ACE2 fragment, and the hydrogen atom bonded to CZ carbon atom of F456Spike (Fig. 5K), as well as the OD1 oxygen atom of N487Spike with the hydrogen atom bonded to CG carbon atom of Q24ACE2 (Fig. 5M).
3.1.2 XH/π interactions.
Besides the π-orbital of the aromatic ring, the heavy atom pairs detected as XH/π in the geometric IFP and their FMO-based interaction energies are summarized in Table S2.† It was confirmed that the fragments showing attractive interaction energy of DI component lower than −3 kcal mol−1 (Fig. 4B and D) were mainly associated with XH/π interactions with a distance between the centroid of the aromatic ring and heavy atom of X within 4 Å. For example, there were CH/π interactions via π and σCH orbitals on the imidazole ring of H34ACE2 and the hydrogen atoms bonded to CD1 and CD2 carbon atoms of L455Spike (Fig. 5H), the phenol ring of Y83ACE2 and the hydrogen atoms bonded to CE1 and CZ carbon atoms of F486Spike (Fig. 5N), and the benzene ring of F486Spike and the hydrogen atoms bonded to CB carbon atom of M82ACE2 (Fig. 5N). Moreover, it was clarified that not only the CH of the hydrophobic amino acid residues but also CH/π interaction between the phenol ring of Y505Spike and the hydrogen atom bonded to CA carbon atom of K353ACE2 (Fig. 5F) contributed to the SARS-CoV-2 and ACE2 binding.
In addition to the CH/π interactions described, OH/π interaction between the imidazole ring of H34ACE2 and the hydrogen atom bonded to OH oxygen atom of Y453Spike (Fig. 5H), and the NH/π interaction between the phenol ring of Y41ACE2 and the hydrogen atom bonded to ND2 nitrogen atom of N501Spike (Fig. 5C), were also found. It was confirmed that interaction energies of DI component for H34ACE2 with Y453Spike, and Y41ACE2 with N501Spike had stable interaction energies of −3.4 and −4.6 kcal mol−1, respectively.
3.1.3 Ion pair, cation/π, and π–π interactions.
Based on PIEDA and geometric IFP analyses, ion pair interaction was detected only on the NZ nitrogen atom of the side chain on K417Spike and between OD1 and OD2 oxygen atoms of the side chain on E30ACE2. In addition, there were no cation/π and π–π interactions between the S-protein and ACE2.
Taken together, the FMO data revealed that interaction networks were formed between the SARS-CoV-2 S-protein and ACE2 via hydrogen bond, XH/π, and salt bridge interactions spanning multiple residues. The 15 residues of ACE2 selected by CT and DI energy analyses play key roles in the recognition of the SARS-CoV-2 S-protein, representing hot spot residues for inhibiting the binding to ACE2 in the context of drug and antibody design. Although the XH–Y hydrogen bond and XH/π interactions were detected based on the geometric IFP analysis, this approach may fail to detect some molecular interactions. In particular, PIEDA can detect various types of XH/π interactions and also evaluate them quantitatively, whereas structure-based analysis detects only typical interactions. In fact, the CH/π interaction between the phenol ring of Y489Spike and the hydrogen atom bonded to CA carbon atom of F28ACE2 (Fig. 5L), which was not detected by geometric IFP analysis, was found by interaction energy of DI component with PIEDA. By the geometric IFP, the XH/π interactions with the π-orbital of the amide were not detected because this analysis method targeted the π-orbital of the aromatic ring. By PIEDA; however, it was confirmed that the XH/π interactions with the π-orbital of the amide in the side chain on Q498Spike and Q24ACE2 were also crucial for molecular recognition between the SARS-CoV-2 S-protein and ACE2 (Fig. 5E and O).
The amino acid residue pairs that formed the NH–O and OH–O hydrogen bonds were in good agreement with similar interactions reported in previous studies.10–12,79 Herein, it was the first report describing that the formation of CH–O hydrogen bonds with weak interaction energy are important for molecular recognition of ACE2 by the SARS-CoV-2. In addition, although it was described in reports of X-ray crystallography and electron microscopic analyses that hydrophobic residues are involved in molecular recognition as an effect of van der Waals interaction with ambiguous contributions, the findings here reported provide further clarification that such hydrophobic residues form XH/π interactions.
3.2. What are the differences and similarities in the ACE2 recognition mechanism among three SARS S-proteins?
To clarify the differences in the molecular recognition of ACE2 between the SARS-CoV-2 (Fig. 4), the SARS-CoV-2 chimeric (Fig. S2†), and the SARS-CoV S-proteins (Fig. S3†), the FMO-based interaction energies of all three structures were analyzed and compared. Furthermore, the difference in the binding ability of ACE2 (S19–A614) between the SARS-CoV-2 (C348–E502), the SARS-CoV-2 chimeric (C348–E502), and the SARS-CoV S-proteins was also investigated by QM-based interaction energy analysis (Fig. 6 and 7). To easy understanding, Fig. S5† shows the hot spots on molecular surface of the ACE2 and the SARS-CoV-2 S-protein complex (PDB ID: 6LZG) based on our research. This approach revealed hot spot residues of ACE2 for designing low, medium, and peptide inhibitors, as well as epitope candidates of S-protein, to prevent S-protein and ACE2 binding.
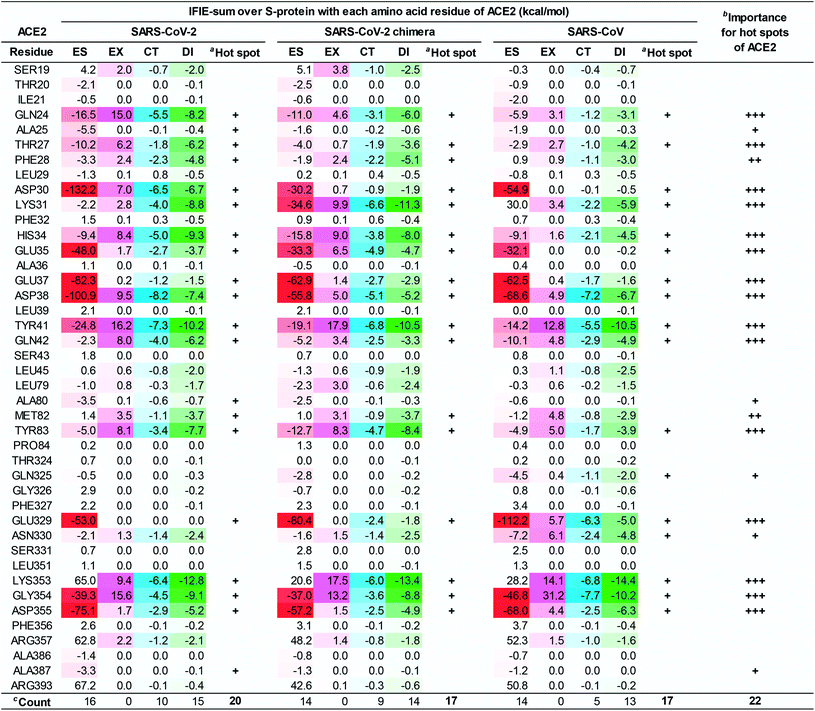 |
| Fig. 6 The IFIE-sums over the S-protein of the SARS-CoV-2 (C348–E502), the SARS-CoV-2 chimera (C348–E502), and the SARS-CoV (C348–E502) with each amino acid residue of ACE2 by PIEDA. Attractive interaction energies of the electrostatic (ES), charge transfer with higher-order mixed terms (CT), and dispersion interaction (DI) are indicated using red, light blue, and green gradations, respectively, and repulsive interaction energy of the exchange–repulsion (EX) is indicated using magenta gradation. aHot spots with an interaction energy of −3 kcal mol−1 or less in any of the ES, EX, CT, and DI components, were labeled as “+”. bThe number of “+” shows importance of hot spots between three complexes. cCount is number of hot spot residues. | |
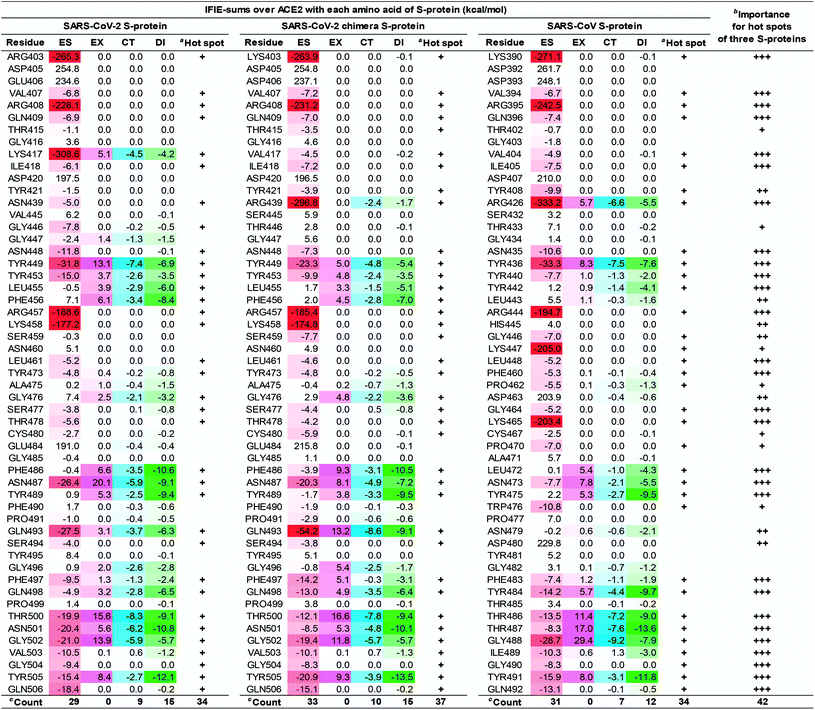 |
| Fig. 7 IFIE-sums over ACE2 (S19–A614) with each amino acid residue of the SARS-CoV-2, the SARS-CoV-2 chimera, and the SARS-CoV S-proteins by PIEDA. Attractive interaction energies of the electrostatic (ES), charge transfer with higher-order mixed terms (CT), and dispersion interaction (DI) are indicated using red, light blue, and green gradations, respectively, and repulsive interaction energy of the exchange–repulsion (EX) is indicated using magenta gradation. aHot spots with an interaction energy of −3 kcal mol−1 or less in any of the ES, EX, CT, and DI components, were labeled as “+”. bThe number of “+” shows importance of hot spots between three complexes. cCount is number of hot spot residues. | |
3.2.1 Hot spot analysis of ACE2.
The three types of S-proteins were compared with each other for their ability to interact with ACE2, and key amino acid residues that were candidates for hot spot for inhibiting S-protein binding were examined. Fig. 6 shows a heat map of ES, EX, CT, and DI interaction energy components for each amino acid residue in ACE2 on the binding surface between ACE2 and the S-proteins. As a result, 22 amino acid residues of ACE2 were found to be important for binding to the three S-proteins, among which 15 were common amino acid residues (Q24, T27, D30, K31, H34, E35, E37, D38, Y41, Q42, Y83, E329, K353, G354, and D35) on ACE2 that could be considered essential amino acid residues for recognition of the S-protein. It was also confirmed that the SARS-CoV-2 and the SARS-CoV-2 chimeric S-proteins formed several short-range interactions with the amino acid residues of ACE2 compared with the SARS-CoV S-protein. The amino acid residues in which the CT and DI interactions were more strongly bound than −3 kcal mol−1 could form short-range interactions, such as hydrogen bonds and XH/π interactions. It is expected that the design of small/medium molecules targeting these 22 amino acid residues may lead to effective inhibition of S-protein binding.
3.2.2 Hot spot analysis of S-protein.
Similar analyses of potential hot spots for ACE2 on the S-protein (Fig. 7) revealed 34, 37, and 34 amino acid residues on the SARS-CoV-2, the SARS-CoV-2 chimeric, and the SARS-CoV S-proteins, respectively, that attractively interacted with ACE2. The SARS-CoV-2 and the SARS-CoV-2 chimeric S-proteins had similar interaction results as the amino acid sequences of the receptor binding motif were almost the same (Fig. 2). In addition, 28 amino acid residues showed a common attractive interaction among the three S-proteins, being considered to be essential hot spots for the S-protein and ACE2 recognition regardless of the protein type. It was also found that, similar to the hot spot analysis of ACE2, the complex of the SARS-CoV-2 and the SARS-CoV-2 chimera had more amino acid residues that formed short-range interactions than the complex with the SARS-CoV. The 28 common hot spots including 17 conserved residues were highly homologous among the three SARS S-proteins and can be designated as essential hot spots for recognizing ACE2 in S-proteins (SARS-CoV-2, SARS-CoV, and MARS virus).
As shown in Fig. 6 and 7, the SARS-CoV-2 S-protein had more hot spots that interacted with ACE2 by CT and DI interaction than the SARS-CoV S-protein; in addition, the SARS-CoV-2 S-protein is considered to bind more firmly to ACE2 than the SARS-CoV S-protein.
These results are consistent with previous findings2,17,28,80 in Section S5.† We also discovered 17 and 4 new hot spots of the SARS-CoV-2 S-protein and the ACE2, respectively (Fig. S5†), that were overlooked in other QM researches for the following reasons: Lim et al. reported the interaction analysis based on the FMO calculations using the self-consistent charge density-functional tight-binding method with the third-order expansion using semi-empirical dispersion (DFTB3/D) method.17 The DFTB3/D method with a partially semi-empirical approach tended to underestimate CT and DI components compared to the MP2 method with ab initio electron-correlated QM theory. In addition, there were several amino acid residues for which the tendency of attractive and repulsive interactions of CT and DI components was different between DFTB3/D and MP2 methods.
Focusing on amino-acid-residue pairs forming NH–O and OH–O hydrogen bonds, Gómez et al. performed higher precision QM calculation with computed accurate interaction energies using highly correlated domain based local pair-natural orbital-coupled cluster (DLPNO-CCSD(T)/aug-cc-pVDZ) level to investigate accurate interaction energies for its excision model not considering the entire complex.18 On the other hand, we analyzed interactions for all the amino-acid-residue pairs of the entire system taking into account their polarization caused by the surrounding environment. We focused not only on typical hydrogen bonds above but also on the CH–O hydrogen bonds and the XH/π interactions to address a wide range of possibilities of the key residues of molecular recognition. Therefore, we succeeded in finding known and new hot spots that were structurally and quantum chemically valid.
3.3. Is FMO-based epitope analysis of the SARS-CoV-2 S-protein useful?
Based on the complex between the SARS-CoV-2 S-protein and ACE2, the expected epitope candidates analyzed by IFIE-sum over B38 Fab antibody using PIEDA were further explored as potential neutralizing epitopes of S-protein. Using a complex of the SARS-CoV-2 S-protein (C361–E516) and B38 Fab antibody (D0–S217 on heavy chain and D0–C215 on light chain), PIEDA and geometric IFP analyses were performed similarly as described in the Sections 3.1 and 3.2. The actual epitope residues of the B38 Fab antibody on the SARS-CoV-2 S-protein were revealed and compared to the candidate epitope residues upon ACE2 binding.
First, FMO-based interaction energy and geometric IFP analyses of the S-protein and B38 Fab antibody were performed to clarify the amino acid residues and their interaction type that were critical for molecular recognition. FMO-based interaction energy was analyzed by IFIE-sum (Fig. S4†). Next, the hydrogen bonds, XH/π interactions detected by geometric IFP, and IFIEs of the corresponding fragment pair are assessed (Tables S3 and S4†). Based on the IFIE and the geometric IFP analyses, the interaction of 20 amino acid residues in the SARS-CoV-2 S-protein played a key role in B38 Fab antibody recognition.
3.3.1 XH–Y hydrogen bonds.
IFIEs between fragments that include heavy atom pairs detected as hydrogen bonds in the geometric IFP are summarized in Table S3.† It was confirmed that the fragments showing attractive interaction energies of ES and CT+mix components lower than −30 and −3 kcal mol−1 (Fig. S4B and D†) were mainly associated with NH–O, OH–O hydrogen bonds with a distance between heavy atoms within 3 Å. The hydrogen bonds between the OG oxygen atom of S30B38-H and NZ nitrogen atom of K458Spike, the OD2 oxygen atom of D429Spike and OG oxygen atom of S56B38-H, and the O oxygen atom of the main chain on L455Spike (F456Spike fragment) and OH oxygen atom of Y33B38-H, were typical examples of such interaction. There was no interaction between the SARS-CoV-2 S-protein and B38 Fab antibody indicating a salt bridge by IFIE and geometric IFP analyses. Although weaker than the interaction energies of NH–O and OH–O hydrogen bonds, CH–O hydrogen bonds also showed an attractive interaction energy that contributed to the molecular recognition of the SARS-CoV-2 S-protein and B38 Fab antibody, such as oxygen and carbon atom pairs for the O oxygen atom of the main chain on L455Spike (F456Spike fragment) and CE2 carbon atom of Y33B38-H, and the OG oxygen atom of S30B38-L and CG carbon atom of Q498Spike.
3.3.2 XH/π interactions.
IFIEs between fragments that include heavy atom pairs detected as XH/π in the geometric IFP are summarized in Table S4.† It was confirmed that the fragments showing attractive interaction energy by DI components lower than −3 kcal mol−1 (Fig. S4B and D†) were mainly associated with XH/π interactions with a distance between the centroid of the aromatic ring and heavy atom of X within 5 Å. For example, there were CH/π interactions via π and σCH orbitals on the benzene ring of F456Spike and the CE2 carbon atom of Y33B38-H, the phenol ring of Y32B38-L and the CD2 and CE2 carbon atoms of Y505Spike, and the phenol ring of Y505Spike and the CA and CB carbon atoms of I29B38-L. It was also clarified that not only the CH of the hydrophobic amino acid residues but also the CH/π interaction between the phenol ring of Y94B38-L and the CB carbon atom of D405Spike contributed to the SARS-CoV-2 S-protein and B38 Fab antibody binding. Moreover, CH/π and NH/π interactions between the phenol ring of Y505Spike and NE2 nitrogen atom of Q90B38-L were also identified.
3.3.3 FMO-based epitope analysis.
The actual epitope residues of the B38 Fab antibody on the SARS-CoV-2 S-protein were revealed by IFIE-sums. We compared the actual epitope residues of the SARS-CoV-2 S-protein recognized by B38 Fab antibody and the epitope candidate residues bounded to ACE2 (Fig. 8). There were 21 common amino acid residues of the SARS-CoV-2 S-protein among the 31 epitope residues bound by B38 Fab antibody and the 34 hot spots bound by ACE2. On the other hand, the amino acid residues of S-protein in which attractive interaction was observed with ACE2 but not with the B38 Fab antibody were V407, R408, I418, N439, G446, N448, Y449, Y453, R457, T478, Q493, F497, and T500. It was also revealed new amino acid residues through which the S-protein interacted with the B38 Fab antibody (D405, E406, T415, G416, D420, Y421, N460, Y473, A475, E484, and G496). Several amino acid residues of S-protein that had an attractive interaction with ACE2 did not interact with the B38 Fab antibody. Nevertheless, the B38 Fab antibody showed more CT and DI interactions with the S-protein amino acid residues that did not interact with ACE2.
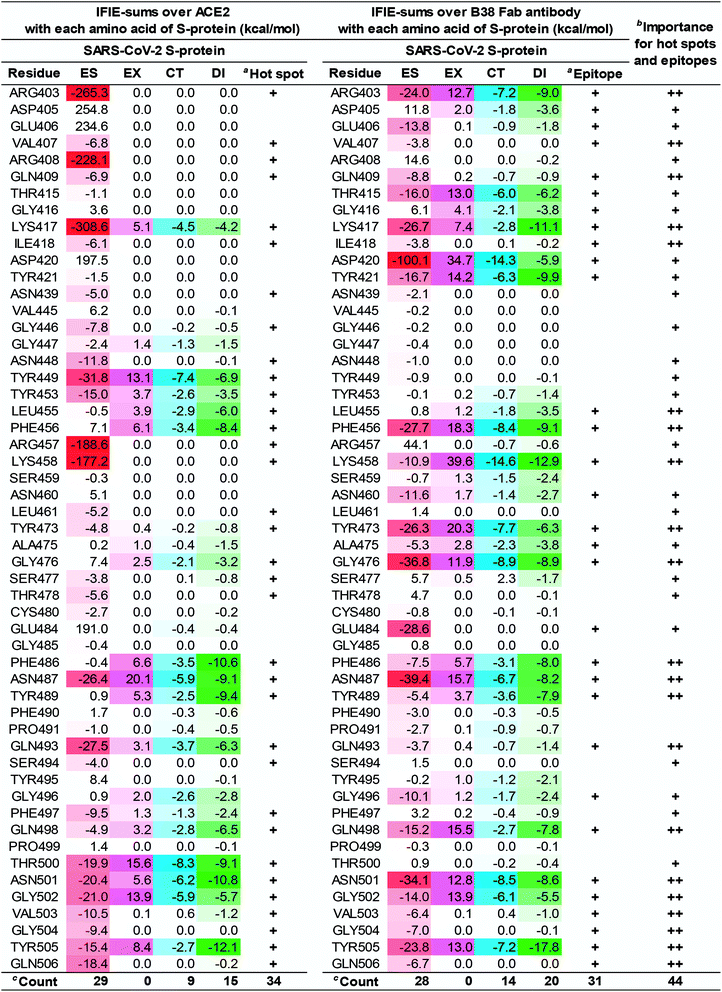 |
| Fig. 8 Difference of the IFIE-sums over the SARS-CoV-2 S-protein (C361–E516) between ACE2 and B38 Fab antibody. Interaction of each amino acid residue on S-protein with ACE2 and B38 Fab antibody are listed. Attractive interaction energies of the electrostatic (ES), charge transfer with higher-order mixed terms (CT), and dispersion interaction (DI) are identified using red, light blue, and green gradations, respectively, and repulsive interaction energy of the exchange–repulsion (EX) is identified using magenta gradation. aHot spots and epitopes with an interaction energy of −3 kcal mol−1 or less in any of the ES, EX, CT, and DI components, were labeled as “+”. bThe number of “+” shows importance for hot spots and epitopes. cCount is number of hot spot and epitope residues. | |
The numbers of amino acid residues in the SARS-CoV-2 S-protein showing CT and DI interaction were 9 and 15, respectively, when complexed with ACE2; whereas it was 14 and 20, respectively, when the S-protein was complexed with the B38 Fab antibody. This indicated that B38 Fab acquired more hydrogen bonds and XH/π interactions than ACE2. From these results, the 21 amino acid residues of the SARS-CoV-2 S-protein shared by the ACE2 and B38 Fab antibody interactions would be essential epitopes that directly inhibit ACE2 recognition and binding. This data (Fig. 8) is expected to provide useful information for the development of potentially therapeutic antibodies. For example, for residues without attractive interaction with the antibody, the substitution of amino acid residues that mimic the interaction with ACE2 will lead to the design of antibodies with higher binding capacity. These amino acid residues were also consistent with the description of NH–O and OH–O hydrogen bonds reported by a previous study.3 New NH–O and OH–O hydrogen bonds, not shown by the previous study,3 were identified and their interaction was clarified by FMO calculations. This study demonstrated that CH–O hydrogen bonds and XH/π interactions are crucial for the molecular recognition between the SARS-CoV-2 S-protein and B38 Fab antibody, with 21 epitope residues of the SARS-CoV-2 S-protein being critical for antibody design.
The difference in the interaction energy of each amino acid residue of the S-protein between ACE2 and B38 Fab antibody will provide worthwhile information for improving B38 Fab and other antibodies. Lim et al. has shown two large hot spot regions from FMO-based interaction analysis of ACE2 and S-proteins of the SARS-CoV-2.17 Since the hot spots of S-protein with the several neutralizing antibodies of the SARS-CoV coincided with the 2nd hot spot region, the authors speculated that the 2nd hot spot region was crucial for drug design against the SARS-CoV-2. However, our results suggest that all key hot spots for molecular recognition between the SARS-CoV-2 S-protein and ACE2 may play a significant role in the design of neutralizing antibodies. This is because the first and the second sizable hot spot regions of S-protein, defined by Lim et al., were both found as epitopes of the complex between S-protein and B38 Fab antibody.
As Fig. 8 shows, in ACE2 binding, we confirmed that the primary contribution of local hot spots was long-distance electrostatic interaction, such as that of R403 and K417; however, in B38 Fab antibody binding, the local hot spots (e.g., R403 and K417) and their surrounding amino acid residues acquired a more complex and robust interaction network by short-distance interaction such as hydrogen bond and XH/π interactions. We also confirmed that short-range interactions, such as hydrogen bond and XH/π interactions, form a complex interaction network. That is, the critical amino acid residues in ACE2 binding determined, using FMO-based interaction analysis as hot spots, have the potential to become epitopes of the antibody; hence, Fig. 6–8 may be useful as drug-antibody design guidelines.
3.4. Is the binding potential predictable by FMO-based binding energy?
The ability of ACE2 to bind to the three S-proteins has been previously reported as Kd values (SARS-CoV-2: 44.2 nM, SARS-CoV chimera: 23.2 nM, SARS-CoV: 185 nM).12 It can also be assumed that B38 Fab antibody binds to the SARS-CoV-2 S-protein more strongly than ACE2 because the B38 Fab antibody has been reported to strongly inhibit the SARS-CoV-2 S-protein.3 Therefore, these binding abilities were further examined in light of the FMO calculation results. We evaluated the binding energy between the SARS-CoV-2 S-protein and ACE2/B38 Fab antibody with and without sugar moieties. In preparation for the emergence of various variants in the future, we also tried to estimate the mutant effect based on FMO calculation. The complex structure of the mutant N501Y of the S-protein, similar to the variants that were first recognized in the United Kingdom and South Africa,6–8 had not yet been published as of February 1, 2021. Thus, the IFIE and binding energy between the N501Y S-protein and the ACE2 were investigated with the aid of computer modeling, as seen in Section S7.†
3.4.1 FMO-based binding energies.
The predicted binding energies using summation of the IFIEs (total, ES, EX, CT, and DI) between S-proteins and ACE2/B38 Fab antibody with sugar moieties are listed in Table 1. The total binding energy using IFIE approach was evaluated by: |  | (5) |
where I is the fragment of the S-protein and J is the fragment of the ACE2/B38 Fab antibody.
Table 1 Predicted binding energies (kcal mol−1) between the S-protein and ACE2/B38 Fab antibodya
S-protein |
SARS-CoV-2 |
SARS-CoV-2 chimera |
SARS-CoV |
SARS-CoV-2 |
Abbreviations: CT+mix, charge transfer with higher-order mixed terms; Desolv, desolvation; DI, dispersion interaction; ES, electrostatic; EX, exchange–repulsion; Kd, binding dissociation constant; SC, statistically correction; SC-ES, statistically corrected electrostatic.
|
Binding protein |
ACE2 |
ACE2 |
ACE2 |
B38 Fab antibody |
K
d (nM) |
44.2 |
23.2 |
185 |
— |
pKd |
7.35 |
7.63 |
6.73 |
— |
ES |
−887.15 |
−645.09 |
−818.50 |
−432.38 |
EX |
122.71 |
121.73 |
110.62 |
269.35 |
CT+mix |
−70.93 |
−67.92 |
−56.88 |
−130.86 |
DI |
−125.36 |
−123.48 |
−105.42 |
−179.50 |
Total |
−960.73 |
−714.75 |
−870.19 |
−473.38 |
SC-ES |
−612.88 |
−491.07 |
−549.43 |
−498.64 |
Total (SC) |
−686.46 |
−560.74 |
−601.11 |
−539.65 |
Desolv |
592.53 |
395.91 |
531.82 |
98.78 |
Total (Desolv) |
−368.20 |
−318.84 |
−338.36 |
−374.61 |
Total (SC+Desolv) |
−93.92 |
−164.83 |
−69.29 |
−440.87 |
In the case of the three S-proteins with ACE2, the main component of the binding energies was electrostatic interaction energy. This is probably due to the positive charge (SARS-CoV-2: +2e, SARS-CoV-2 chimera: +1e, SARS-CoV: +2e) of the viral S-proteins and the highly negative charge of ACE2 (−26e). The IFIEs of total and ES components did not correlate with pKd values. On the other hand, quantum chemical short-range interactions, such as the CT and DI energies, were aligned in the same magnitudes as pKd, for which the order of pKd for the SARS-CoV-2 and the SARS-CoV S-proteins were regarded as almost the same (7.35 and 7.63, respectively).
In the case of the SARS-CoV-2 S-protein with B38 Fab antibody, the main component of the binding energies was electrostatic interaction energy. The binding energies of the SARS-CoV-2 S-protein with B38 Fab antibody at the total and ES components were weaker than those for the three S-proteins with ACE2, because the B38 Fab antibody has a positive charge (+5e). In contrast, the binding energies for the DI and CT components were the strongest of the four complexes. As described in Sections 3.1–3.3, it is considered that contributions of the CT+mix and the DI components came from the XH–Y hydrogen bonding involving charge transfer, the XH/π interaction at the contact surface, and orbital interaction of the aromatic rings. The overall CT and DI energies for the four complexes seem to comply with the strength of the binding affinity; however, the total interaction energy including ES does not work well. Therefore, the solvation effect was next considered for evaluating the binding energy.
3.4.2 Solvation effect.
To account for the solvation effect, the statistically corrected IFIE (SCIFIE)81 and FMO method combined with molecular mechanics Poisson–Boltzmann surface area (FMO+MM-PBSA)43 approaches were used for predicting the binding energy (Table 1). The SCIFIE approach is a method to consider electrostatic and solvation shielding effects. In this research, a statistically corrected ES interaction energy (ΔẼSC-ESIJ), which felt the shielding effect by both the solvent and the inside of the molecule, was used to analyze the ES component. Then the modified IFIE with the SC-ES component is given by | ΔẼSCIJ = ΔẼSC-ESIJ + ΔẼEXIJ + ΔẼCT+mixIJ + ΔẼDIIJ | (6) |
and the total binding energy using SCIFIE approach was evaluated by: | 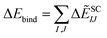 | (7) |
where I is the fragment of the S-protein and J is the fragment of the ACE2/B38 Fab antibody. On the other hand, the FMO+MM-PBSA approach43 is a method used to evaluate binding energy incorporating the solvation effect by combining the desolvation energy with the sum of IFIEs as follows: | ΔGDesolv = GSolvAB − GSolvA − GSolvB | (8) |
where ΔGDesolv is desolvation energy, and GSolv is solvation energy with A representing the S-protein and B the ACE2/B38 Fab antibody. The total binding energy was predicted using FMO+MM-PBSA approach as follows: | 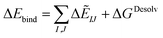 | (9) |
Lastly, the total binding energy was predicted using SCIFIE combined with the desolvation evaluated using MM-PBSA method as follows:
| 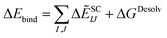 | (10) |
These total binding energies are shown in Table 1. While ΔẼSC-ESIJ gives a weak contribution by shielding effect, the binding energy alone using the SCIFIE approach for the four complexes was not in agreement with the experimental binding affinity. By adding the desolvation energies from the MM-PBSA approach, the excessive electrostatic interaction energies were suppressed, and the predicted binding energies approximate to reproduce the experimental binding ability under the physiological condition. Moreover, the binding energy including both statistical correction and desolvation (SC+Desolv) for the three complexes, excluding the SARS-CoV-2 chimeric S-protein and ACE2 complex, seemed to be in agreement with the order of the binding affinity.2,80 The large difference seen in the interaction energy due to the electric charge of the molecules was eliminated by adding shielding and the desolvation effects, and the binding energies became comparable to that of the experimental value. Thus, when the PPI binding ability is estimated by FMO calculation among differently charged molecular systems, it may be useful to incorporate both the shielding and desolvation effects.
Taken together, it remains challenging to predict the strength of the binding ability of the differently charged proteins, such as ACE2, having highly negative charges, and of the B38 Fab antibody, having highly positive charges with a receptor such as S-protein using bare IFIE. However, incorporating desolvation energy and SCIFIE demonstrates that the predicted binding energies between differently charged proteins could be improved for suppressing the overestimated ES interactions. Meanwhile, ACE2 comprises large amount of aspartic acids, glutamic acids, and histidines that can have multiple protonated states. Therefore, while the calculation was performed using only one protonation state in this study, it will be necessary to examine multiple plausible protonation states that may exist in vivo and investigate the predicted binding energy of PPI.
3.4.3 Sugar chain effect.
The role of sugar chains in the molecular recognition of S-protein and ACE2/B38 Fab antibody was investigated. The sugar chain located on the SARS-CoV-2/SARS-CoV S-protein and ACE2 binding surface was the only BMA sugar chain, and was the terminal sugar chain of the NAG–NAG–BMA sugar chain extending from the side chain of N90 on ACE2 (Fig. 2B and C). In all complexes between the SARS-CoV-2 S-protein and ACE2 (PDB IDs: 6LZG, 6M0J, and 6M17) a sugar chain was not observed, in which a sugar chain consisting of three sugars from N90 of ACE2 was likely to reach and interact with the S-protein. There was one sugar chain (NAG) from N90 of ACE2, which did not directly interact with the SARS-CoV-2 S-protein (PDB ID: 6LZG). Moreover, no sugar chains were identified on the binding surface between the SARS-CoV-2 S-protein and B38 Fab antibody.
Table S5† lists the predicted binding energies using the IFIEs between the S-protein and ACE2/B38 Fab antibody without interaction energy of the sugar chain fragment. By comparing Tables 1 and S5,† the predicted binding energies of IFIEs between the SARS-CoV-2 chimeric S-protein and ACE2 was stabilized at −15.5 kcal mol−1 by the sugar chains, whereas that of the SARS-CoV S-protein and ACE2 was stabilized at −2.8 kcal mol−1. In the SARS-CoV-2 chimeric S-protein/ACE2 complex, the sugar chain on ACE2 attractively interacted with R408 via hydrogen bond and was critical for the molecular recognition of the S-protein. Moreover, the IFIEs between the BMA703 sugar chain on ACE2 and R408 of the SARS-CoV-2 S-protein showed an attractive interaction of −16.2 kcal mol−1 (ES: −14.4 kcal mol−1, EX: 1.5 kcal mol−1, CT+mix: −1.3 kcal mol−1, DI: −2.0 kcal mol−1), which accounts for most of the summation of IFIEs in sugar chain effects. In the complex between the SARS-CoV S-protein and ACE2, weak attractive interaction between the BMA1092 sugar chain on ACE2 with the S-protein was confirmed, representing a repulsive interaction energy of +3.0 kcal mol−1; however, several residues (e.g., D392, D393, and T402) of the S-protein interacted with the BMA1092 sugar chain with IFIEs of −1.0 kcal mol−1. In this study, only three sugar chains from N90 on ACE2 played key roles in the molecular recognition between the SARS-CoV-2 chimeric S-protein and ACE2. Since sugar chains are generally present on the molecular surface, the electron density tends to be difficult to see in the experimental setting. Thus, it will be necessary to discuss not only one X-ray crystal structure but also other X-ray crystal structures and structural fluctuations by MD simulations. The flexibility of sugar chains on the SARS-CoV-2 S-protein and the ACE2 was already investigated by several MD simulations.22–26 One of the results that the sugar chain on N90ACE2 works favorably for molecular recognition of the S-protein was reported as follows: the sugar chain on N90ACE2 was close enough to the S-protein to repeatedly form interactions.24 Although the sugar chains (NAG–NAG–BMA) of N90ACE2 were present near the interface with the S-protein, the sugar chain (BMA) of N90ACE2 and the S-protein did not always form hydrogen bonds judging from our FMO calculation results. In other words, it was suggested that the sugar chains of N90ACE2 would be located near the S-protein; however, they may not form a robust interaction with the S-protein. In fact, only one sugar chain (NAG) of N90ACE2, which could not reach the interface with the S-protein, was observed in the complex of the SARS-CoV-2 S-protein and the ACE2, and it will be considered that the remaining sugar chains (NAG-BMA) of N90ACE2 would be fluctuating and may not be observed by X-ray crystal structures.
3.4.4 Mutation effect of the SARS-CoV-2 S-protein (N501Y).
Currently, the highly transmissible variants of the United Kingdom (N501Y) and South Africa (N501Y, E484K, and K417N) are prevalent in various regions.6,7 Here, we investigated a mutation N501Y of RBD in common between S-protein variants of the United Kingdom and South Africa to understand the mutation effect by using modeling structure (FMODB ID: 7J11K), as seen in Section S7.† From interaction energy analysis (Fig. S7†), the N501Y mutation of the S-protein enhanced the attractive interaction because of the hydrogen bond and the XH/π interactions with Y41ACE2 and K353ACE2 more than a wild type of the S-protein (Fig. S6†). It was confirmed that the N501Y mutation of the S-protein attractively enhances the DI energy of Y41ACE2 on the peptide motif (E37–Q42), which was essential for recognizing the S-protein, as proposed by Larue et al.28 Along with these attractive interactions, it was confirmed that the binding energy including both statistical correction and desolvation (SC+Desolv) of the ACE2 with the mutant S-protein (N501Y) was further strengthened by ca. −10 kcal mol−1 compared to that with the wild type S-protein as seen in Tables 1 and S6.† These FMO results may explain one of the reasons for the high infectivity of the mutant (N501Y). Recently, Zhu et al. analyzed the complex between the SARS-CoV-2 S-protein of the N501Y variant and a neutralizing antibody by cryo-electron microscopy (not yet published at February 7, 2021).8 They reported that the overall conformation and the interactions between the S-protein and the antibody of the variant almost were unchanged compared with those of the wild type; however, there was replacement of the asparagine residue by the bulkier tyrosine side chain results in subtle local rearrangements. Also, the binding ability of ACE2 with the variant was enhanced considerably compared with the wild type. Their results are in good agreement with our FMO results using the modeling structure of the complex between the S-protein of the N501Y variant and the ACE2. We hope that the experimental structure of the complex between the S-protein of the novel variant and ACE2/antibody is published for more detailed analysis.
4. Conclusion
The amino acid residues that are the key to the molecular recognition of three SARS S-proteins and ACE2/B38 Fab antibody were revealed by FMO-based interaction energy analysis with ab initio electron-correlated theory. The collected data provided new insights in the importance of forming a complex interaction network for the molecular recognition between the S-protein and ACE2/B38 Fab antibody, not only via NH–O and OH–O hydrogen bonds and salt bridges, but also via the CH–O hydrogen bonds and XH/π interactions. Since the XH/π interaction is specifically found by the interaction energy of DI components, it is difficult to accurately understand XH/π interaction solely through MM-based electrostatic interaction analysis and structure-based geometry analysis. Moreover, QM-based hot spot and epitope analyses by FMO calculations were useful in clarifying the type and strength of molecular interactions, such as hydrogen bond and XH/π interactions. Prediction of the binding ability between the three types of SARS S-proteins and ACE2/antibody was performed by FMO-based interaction energy where incorporation of the shielding and desolvation effects was an essential factor. Since sugar chains are generally present on the molecular surface and have a disordered structure, the role of sugar chains in molecular recognition between the S-protein and ACE2 should be examined using structural fluctuation sampling, such as MD simulation, in future investigations. In addition, we investigated IFIE and binding energy between the ACE2 and the mutant N501Y on the SARS-CoV-2 S-protein regarding the mutation in common of the variants between the United Kingdom and South Africa, and our results can explain the high infectivity of the mutant.
We plan to release all the FMO data in this study on the public database, FMODB,75–77 so that all researchers can access it and utilize it for designing effective antibody-drugs. Also, our group has recently performed over 336 FMO calculations for the COVID-19-related proteins such as S-protein, main protease, RNA-dependent RNA polymerase,56 based on the representative PDB structures selected in the PDB Japan database in line with this global fight effort against the coronavirus epidemic. These data have already been published on FMODB75–77 and can easily be analyzed IFIEs for inter- and intramolecular interactions on the Web interface. Finally, we expect that these findings of novel hot spots/epitopes between the SARS-CoV-2 S-protein and ACE2/B38 Fab antibody will provide useful information for future antibody design, evaluation of the binding property of variant, and small or medium drug design that overcome COVID-19.
Author contributions
The manuscript was written by CW and YO. The FMO calculations were performed and analyzed by CW. All authors discussed the results and have given approval to the final version of the manuscript.
Abbreviations
ACE2 | Angiotensin-converting enzyme 2 |
BMA | β-D-mannopyranose |
COVID-19 | Coronavirus disease 2019 |
CT+mix | Charge transfer with higher-order mixed terms |
Desolv | Desolvation |
DFTB3/D | Self-consistent charge density-functional tight-binding method with the third-order expansion using semi-empirical dispersion |
DI | Dispersion interaction |
DLPNO-CCSD(T) | domain based local pair-natural orbital-coupled cluster |
ES | Electrostatic |
EX | Exchange–repulsion |
Fab | Fragment antigen-binding |
FMO | Fragment molecular orbital |
FMODB | FMO database |
FP | Fusion peptide |
HR1 | Heptad repeat 1 |
HR2 | Heptad repeat 2 |
IC | Intracellular domain |
IFIE | Inter-fragment interaction energy |
IFIE-sum | Summation of IFIEs |
IFP | Interaction fingerprint |
Kd | Binding dissociation constant |
MD | Molecular dynamics |
MM | Molecular mechanics |
MM-PBSA | Molecular mechanics Poisson–Boltzmann surface area |
MO | Molecular orbital |
MOE | Molecular Operating Environment |
MP2 | Second-order Møller–Plesset perturbation theory |
NAG |
N-acetyl-β-D-glucosamine |
NTD | N-terminal domain |
PDB | Protein data bank |
PIEDA | Pair interaction energy decomposition analysis |
PPI | Protein–protein interaction |
QM | Quantum mechanics |
RBD | Receptor-binding domain |
RNA | Ribonucleic acid |
SARS-CoV-2 | Severe acute respiratory syndrome coronavirus 2 |
SC | Statistically correction |
SC-ES | Statistically corrected electrostatic |
SCIFIE | Statistically corrected IFIE |
SD1 | Subdomain 1 |
SD2 | Subdomain 2 |
Solv | Solvation |
S-protein | Spike glycoprotein |
TM | Transmembrane region |
Conflicts of interest
There are no conflicts to declare.
Acknowledgements
The authors thank Dr Daisuke Takaya and Dr Kikuko Kamisaka at RIKEN for data registration in the FMO database (FMODB). CW acknowledges JST, PRESTO grant (JPMJPR18GD). This study was partially supported by the Platform Project for Supporting Drug Discovery and Life Science Research (Basis for Supporting Innovative Drug Discovery and Life Science Research) (BINDS) from the Japan Agency for Medical Research and Development (AMED) (grant number JP20am0101113). This research was supported by the RIKEN programs for Drug Discovery and Medical Technology Platforms (DMP) and COVID-19 project. This research was performed in the activities of the FMO drug design consortium (FMODD). The FMO calculations were partially performed using the Oakforest-PACS supercomputer (project ID: hp200101), the HOKUSAI supercomputer (RIKEN Advanced Center for Computing and Communications, Saitama, Japan), and the TSUBAME3.0 supercomputer (Tokyo Institute of Technology, Tokyo, Japan). PIEDA calculations were performed with the MIZUHO/BioStation software package (version 4.0). We also thank Editage (www.editage.jp) for English language editing.
References
-
Coronavirus disease (COVID-19) – World Health Organization, https://www.who.int/emergencies/diseases/novel-coronavirus-2019, accessed October 2, 2020 Search PubMed.
- A. C. Walls, Y.-J. Park, M. A. Tortorici, A. Wall, A. T. McGuire and D. Veesler, Cell, 2020, 181, 281–292 CrossRef CAS PubMed.
- Y. Wu, F. Wang, C. Shen, W. Peng, D. Li, C. Zhao, Z. Li, S. Li, Y. Bi, Y. Yang, Y. Gong, H. Xiao, Z. Fan, S. Tan, G. Wu, W. Tan, X. Lu, C. Fan, Q. Wang, Y. Liu, C. Zhang, J. Qi, G. F. Gao, F. Gao and L. Liu, Science, 2020, 368, 1274–1278 CrossRef CAS PubMed.
- J. Huo, A. Le Bas, R. R. Ruza, H. M. E. Duyvesteyn, H. Mikolajek, T. Malinauskas, T. K. Tan, P. Rijal, M. Dumoux, P. N. Ward, J. Ren, D. Zhou, P. J. Harrison, M. Weckener, D. K. Clare, V. K. Vogirala, J. Radecke, L. Moynié, Y. Zhao, J. Gilbert-Jaramillo, M. L. Knight, J. A. Tree, K. R. Buttigieg, N. Coombes, M. J. Elmore, M. W. Carroll, L. Carrique, P. N. M. Shah, W. James, A. R. Townsend, D. I. Stuart, R. J. Owens and J. H. Naismith, Nat. Struct. Mol. Biol., 2020, 27, 846–854 CrossRef CAS PubMed.
- D. Pinto, Y.-J. Park, M. Beltramello, A. C. Walls, M. A. Tortorici, S. Bianchi, S. Jaconi, K. Culap, F. Zatta, A. De Marco, A. Peter, B. Guarino, R. Spreafico, E. Cameroni, J. B. Case, R. E. Chen, C. Havenar-Daughton, G. Snell, A. Telenti, H. W. Virgin, A. Lanzavecchia, M. S. Diamond, K. Fink, D. Veesler and D. Corti, Nature, 2020, 583, 290–295 CrossRef CAS PubMed.
- S. A. Kemp, W. T. Harvey, R. P. Datir, D. A. Collier, I. Ferreira, A. M. Carabelli, D. L. Robertson and R. K. Gupta, bioRxiv, 2020 DOI:10.1101/2020.12.14.422555.
- H. Tegally, E. Wilkinson, M. Giovanetti, A. Iranzadeh, V. Fonseca, J. Giandhari, D. Doolabh, S. Pillay, E. J. San, N. Msomi, K. Mlisana, A. von Gottberg, S. Walaza, M. Allam, A. Ismail, T. Mohale, A. J. Glass, S. Engelbrecht, G. Van Zyl, W. Preiser, F. Petruccione, A. Sigal, D. Hardie, G. Marais, M. Hsiao, S. Korsman, M.-A. Davies, L. Tyers, I. Mudau, D. York, C. Maslo, D. Goedhals, S. Abrahams, O. Laguda-Akingba, A. Alisoltani-Dehkordi, A. Godzik, C. K. Wibmer, B. T. Sewell, J. Lourenço, L. C. J. Alcantara, S. L. K. Pond, S. Weaver, D. Martin, R. J. Lessells, J. N. Bhiman, C. Williamson and T. de Oliveira, medRxiv, 2020 DOI:10.1101/2020.12.21.20248640.
- X. Zhu, D. Mannar, S. S. Srivastava, A. M. Berezuk, J.-P. Demers, J. W. Saville, K. Leopold, W. Li, D. S. Dimitrov, K. S. Tuttle, S. Zhou, S. Chittori and S. Subramaniam, bioRxiv, 2021 DOI:10.1101/2021.01.11.426269.
- Y. Wang, M. Liu and J. Gao, Proc. Natl. Acad. Sci. U. S. A., 2020, 117, 13967–13974 CrossRef CAS PubMed.
- J. Lan, J. Ge, J. Yu, S. Shan, H. Zhou, S. Fan, Q. Zhang, X. Shi, Q. Wang, L. Zhang and X. Wang, Nature, 2020, 581, 215–220 CrossRef CAS.
- R. Yan, Y. Zhang, Y. Li, L. Xia, Y. Guo and Q. Zhou, Science, 2020, 367, 1444–1448 CrossRef CAS.
- J. Shang, G. Ye, K. Shi, Y. Wan, C. Luo, H. Aihara, Q. Geng, A. Auerbach and F. Li, Nature, 2020, 581, 221–224 CrossRef CAS PubMed.
- D. J. Benton, A. G. Wrobel, P. Xu, C. Roustan, S. R. Martin, P. B. Rosenthal, J. J. Skehel and S. J. Gamblin, Nature, 2020, 588, 327–330 CrossRef CAS PubMed.
- M. Amin, M. K. Sorour and A. Kasry, J. Phys. Chem. Lett., 2020, 11, 4897–4900 CrossRef CAS PubMed.
- K. Hassanzadeh, H. Perez Pena, J. Dragotto, L. Buccarello, F. Iorio, S. Pieraccini, G. Sancini and M. Feligioni, ACS Chem. Neurosci., 2020, 11, 2361–2369 CrossRef CAS PubMed.
- A. Spinello, A. Saltalamacchia and A. Magistrato, J. Phys. Chem. Lett., 2020, 11, 4785–4790 CrossRef CAS PubMed.
- H. Lim, A. Baek, J. Kim, M. S. Kim, J. Liu, K.-Y. Nam, J. Yoon and K. T. No, Sci. Rep., 2020, 10, 16862 CrossRef CAS PubMed.
- S. A. Gómez, N. Rojas-Valencia, S. Gómez, F. Egidi, C. Cappelli and A. Restrepo, ChemBioChem, 2020, 22, 724–732 CrossRef PubMed.
- K. Akisawa, R. Hatada, K. Okuwaki, Y. Mochizuki, K. Fukuzawa, Y. Komeiji and S. Tanaka, RSC Adv., 2021, 11, 3272–3279 RSC.
-
D. E. Shaw, Molecular Dynamics Simulations Related to SARS-CoV-2, D. E. Shaw Research Technical Data, 2020, http://www.deshawresearch.com/resources_sarscov2.html, accesed September 15, 2020 Search PubMed.
- S. Pach, T. N. Nguyen, J. Trimpert, D. Kunec, N. Osterrieder and G. Wolber, bioRxiv, 2020 DOI:10.1101/2020.05.14.092767.
- L. Casalino, Z. Gaieb, J. A. Goldsmith, C. K. Hjorth, A. C. Dommer, A. M. Harbison, C. A. Fogarty, E. P. Barros, B. C. Taylor, J. S. McLellan, E. Fadda and R. E. Amaro, ACS Cent. Sci., 2020, 6, 1722–1734 CrossRef CAS PubMed.
- A. R. Mehdipour and G. Hummer, bioRxiv, 2020 DOI:10.1101/2020.07.09.193680.
- P. Zhao, J. L. Praissman, O. C. Grant, Y. Cai, T. Xiao, K. E. Rosenbalm, K. Aoki, B. P. Kellman, R. Bridger, D. H. Barouch, M. A. Brindley, N. E. Lewis, M. Tiemeyer, B. Chen, R. J. Woods and L. Wells, Cell Host Microbe, 2020, 28, 586–601 CrossRef CAS.
- E. P. Barros, L. Casalino, Z. Gaieb, A. C. Dommer, Y. Wang, L. Fallon, L. Raguette, K. Belfon, C. Simmerling and R. E. Amaro, Biophys. J., 2021, 120, 1072–1084 CrossRef CAS PubMed.
- T. Mori, J. Jung, C. Kobayashi, H. M. Dokainish, S. Re and Y. Sugita, Biophys. J., 2021, 120, 1060–1071 CrossRef CAS PubMed.
- L. Cao, I. Goreshnik, B. Coventry, J. B. Case, L. Miller, L. Kozodoy, R. E. Chen, L. Carter, A. C. Walls, Y.-J. Park, E.-M. Strauch, L. Stewart, M. S. Diamond, D. Veesler and D. Baker, Science, 2020, eabd9909 Search PubMed.
- R. C. Larue, E. Xing, A. D. Kenney, Y. Zhang, J. A. Tuazon, J. Li, J. S. Yount, P.-K. Li and A. Sharma, Bioconjugate Chem., 2021, 32, 215–223 CrossRef CAS PubMed.
- P. K. Panda, M. N. Arul, P. Patel, S. K. Verma, W. Luo, H.-G. Rubahn, Y. K. Mishra, M. Suar and R. Ahuja, Sci. Adv., 2020, 6, eabb8097 CrossRef CAS PubMed.
- M. Zheng and L. Song, Cell. Mol. Immunol., 2020, 17, 536–538 CrossRef CAS.
- C. Bissantz, B. Kuhn and M. Stahl, J. Med. Chem., 2010, 53, 5061–5084 CrossRef CAS PubMed.
- S. Tsuzuki, K. Honda, T. Uchimaru, M. Mikami and K. Tanabe, J. Am. Chem. Soc., 2000, 122, 3746–3753 CrossRef CAS.
- S. Tsuzuki, K. Honda, T. Uchimaru, M. Mikami and K. Tanabe, J. Am. Chem. Soc., 2000, 122, 11450–11458 CrossRef CAS.
- S. Tsuzuki, K. Honda, T. Uchimaru, M. Mikami and K. Tanabe, J. Am. Chem. Soc., 2002, 124, 104–112 CrossRef CAS PubMed.
- C. Watanabe, K. Fukuzawa, Y. Okiyama, T. Tsukamoto, A. Kato, S. Tanaka, Y. Mochizuki and T. Nakano, J. Mol. Graphics Modell., 2013, 41, 31–42 CrossRef CAS.
- K. Kitaura, E. Ikeo, T. Asada, T. Nakano and M. Uebayasi, Chem. Phys. Lett., 1999, 313, 701–706 CrossRef CAS.
-
The Fragment Molecular Orbital Method: Practical Applications to Large Molecular Systems, ed. D. Fedorov, K. Kitaura, CRC Press, Boca Raton, 2009, p. 288, DOI:10.1201/9781420078497.
- S. Tanaka, Y. Mochizuki, Y. Komeiji, Y. Okiyama and K. Fukuzawa, Phys. Chem. Chem. Phys., 2014, 16, 10310–10344 RSC.
-
Recent Advances of the Fragment Molecular Orbital Method: Enhanced Performance and Applicability, ed. Y. Mochizuki, S. Tanaka and K. Fukuzawa, Springer, Singapore, 2021, p. 616, DOI:10.1007/978-981-15-9235-5.
- D. G. Fedorov and K. Kitaura, J. Comput. Chem., 2007, 28, 222–237 CrossRef CAS PubMed.
- D. G. Fedorov and K. Kitaura, J. Phys. Chem. A, 2012, 116, 704–719 CrossRef CAS PubMed.
- T. Tsukamoto, K. Kato, A. Kato, T. Nakano, Y. Mochizuki and K. Fukuzawa, J. Comput. Chem., Jpn., 2015, 14, 1–9 CrossRef CAS.
- C. Watanabe, H. Watanabe, K. Fukuzawa, L. J. Parker, Y. Okiyama, H. Yuki, S. Yokoyama, H. Nakano, S. Tanaka and T. Honma, J. Chem. Inf. Model., 2017, 57, 2996–3010 CrossRef CAS PubMed.
- K. Fukuzawa, Y. Mochizuki, S. Tanaka, K. Kitaura and T. Nakano, J. Phys. Chem. B, 2006, 110, 24276 CrossRef CAS.
- S. Amari, M. Aizawa, J. Zhang, K. Fukuzawa, Y. Mochizuki, Y. Iwasawa, K. Nakata, H. Chuman and T. Nakano, J. Chem. Inf. Model., 2006, 46, 221–230 CrossRef CAS PubMed.
- T. Ozawa, E. Tsuji, M. Ozawa, C. Handa, H. Mukaiyama, T. Nishimura, S. Kobayashi and K. Okazaki, Bioorg. Med. Chem., 2008, 16, 10311–10318 CrossRef CAS PubMed.
- S. Hitaoka, M. Harada, T. Yoshida and H. Chuman, J. Chem. Inf. Model., 2010, 50, 1796–1805 CrossRef CAS PubMed.
- T. Yoshida, Y. Munei, S. Hitaoka and H. Chuman, J. Chem. Inf. Model., 2010, 50, 850–860 CrossRef CAS PubMed.
- S. Hitaoka, H. Matoba, M. Harada, T. Yoshida, D. Tsuji, T. Hirokawa, K. Itoh and H. Chuman, J. Chem. Inf. Model., 2011, 51, 2706–2716 CrossRef CAS PubMed.
- Y. Munei, K. Shimamoto, M. Harada, T. Yoshida and H. Chuman, Bioorg. Med. Chem. Lett., 2011, 21, 141–144 CrossRef CAS PubMed.
- Y. Okiyama, C. Watanabe, K. Fukuzawa, Y. Mochizuki, T. Nakano and S. Tanaka, J. Phys. Chem. B, 2019, 123, 957–973 CrossRef CAS PubMed.
-
A. Heifetz, T. James, M. Southey, M. J. Bodkin and S. Bromidge, in Quantum Mechanics in Drug Discovery, ed. A. Heifetz, Springer US, New York, NY, 2020, pp. 37–48 Search PubMed.
- R. Hatada, K. Okuwaki, Y. Mochizuki, Y. Handa, K. Fukuzawa, Y. Komeiji, Y. Okiyama and S. Tanaka, J. Chem. Inf. Model., 2020, 60, 3593–3602 CrossRef CAS PubMed.
- B. Nutho, P. Mahalapbutr, K. Hengphasatporn, N. C. Pattaranggoon, N. Simanon, Y. Shigeta, S. Hannongbua and T. Rungrotmongkol, Biochemistry, 2020, 59, 1769–1779 CrossRef CAS PubMed.
- R. Hatada, K. Okuwaki, K. Akisawa, Y. Mochizuki, Y. Handa, K. Fukuzawa, Y. Komeiji, Y. Okiyama and S. Tanaka, Appl. Phys. Express, 2021, 14, 027003 CrossRef CAS.
- K. Kato, T. Honma and K. Fukuzawa, J. Mol. Graphics Modell., 2020, 100, 107695 CrossRef CAS PubMed.
- T. Sawada, T. Hashimoto, H. Nakano, T. Suzuki, H. Ishida and M. Kiso, Biochem. Biophys. Res. Commun., 2006, 351, 40–43 CrossRef CAS PubMed.
- T. Sawada, T. Hashimoto, H. Nakano, T. Suzuki, Y. Suzuki, Y. Kawaoka, H. Ishida and M. Kiso, Biochem. Biophys. Res. Commun., 2007, 355, 6–9 CrossRef CAS PubMed.
- T. Sawada, T. Hashimoto, H. Tokiwa, T. Suzuki, H. Nakano, H. Ishida, M. Kiso and Y. Suzuki, Glycoconjugate J., 2008, 25, 805–815 CrossRef CAS PubMed.
- T. Iwata, K. Fukuzawa, K. Nakajima, S. Aida-Hyugaji, Y. Mochizuki, H. Watanabe and S. Tanaka, Comput. Biol. Chem., 2008, 32, 198–211 CrossRef CAS PubMed.
- K. Takematsu, K. Fukuzawa, K. Omagari, S. Nakajima, K. Nakajima, Y. Mochizuki, T. Nakano, H. Watanabe and S. Tanaka, J. Phys. Chem. B, 2009, 113, 4991–4994 CrossRef CAS PubMed.
- T. Sawada, D. G. Fedorov and K. Kitaura, J. Am. Chem. Soc., 2010, 132, 16862–16872 CrossRef CAS PubMed.
- T. Sawada, D. G. Fedorov and K. Kitaura, J. Phys. Chem. B, 2010, 114, 15700–15705 CrossRef CAS PubMed.
- Y. Mochizuki, K. Yamashita, K. Fukuzawa, K. Takematsu, H. Watanabe, N. Taguchi, Y. Okiyama, M. Tsuboi, T. Nakano and S. Tanaka, Chem. Phys. Lett., 2010, 493, 346–352 CrossRef CAS.
- A. Yoshioka, K. Takematsu, I. Kurisaki, K. Fukuzawa, Y. Mochizuki, T. Nakano, E. Nobusawa, K. Nakajima and S. Tanaka, Theor. Chem. Acc., 2011, 130, 1197–1202 Search PubMed.
- A. Yoshioka, K. Fukuzawa, Y. Mochizuki, K. Yamashita, T. Nakano, Y. Okiyama, E. Nobusawa, K. Nakajima and S. Tanaka, J. Mol. Graphics Modell., 2011, 30, 110–119 CrossRef CAS PubMed.
- S. Anzaki, C. Watanabe, K. Fukuzawa, Y. Mochizuki and S. Tanaka, J. Mol. Graphics Modell., 2014, 53, 48–58 CrossRef CAS PubMed.
- F. Xu, S. Tanaka, H. Watanabe, Y. Shimane, M. Iwasawa, K. Ohishi and T. Maruyama, Viruses, 2018, 10, 236 CrossRef PubMed.
- S. Tanaka, C. Watanabe, T. Honma, K. Fukuzawa, K. Ohishi and T. Maruyama, J. Mol. Graphics Modell., 2020, 100, 107650 CrossRef CAS.
-
Molecular Operating Environment (MOE), Chemical Computing Group ULC, 1010 Sherbrooke St. West, Suite #910, Montreal, QC, Canada, H3A 2R7, 2019 Search PubMed.
-
T. Nakano, Y. Mochizuki, K. Fukuzawa, S. Amari and S. Tanaka, in Modern Methods for Theoretical Physical Chemistry of Biopolymers, ed. E. B. Starikov, J. P. Lewis and S. Tanaka, Elsevier Science, Amsterdam, 2006, pp. 39–52 Search PubMed.
-
Recent Advances of the Fragment Molecular Orbital Method: Enhanced Performance and Applicability, ed. Y. Mochizuki, S. Tanaka and K. Fukuzawa, Springer Singapore, 2021 Search PubMed.
- Y. Okiyama, T. Nakano, K. Yamashita, Y. Mochizuki, N. Taguchi and S. Tanaka, Chem. Phys. Lett., 2010, 490, 84–89 CrossRef CAS.
-
BioStation Viewer, https://fmodd.jp/biostationviewer-dl/, accessed December 7, 2020 Search PubMed.
-
FMO database (FMODB), https://drugdesign.riken.jp/FMODB/, accessed December 7, 2020 Search PubMed.
- C. Watanabe, H. Watanabe, Y. Okiyama, D. Takaya, K. Fukuzawa, S. Tanaka and T. Honma, Chem-Bio Inf. J., 2019, 19, 5–18 CAS.
- D. Takaya, C. Watanabe, S. Nagase, K. Kamisaka, Y. Okiyama, H. Moriwaki, H. Yuki, T. Sato, N. Kurita, Y. Yagi, T. Takagi, N. Kawashita, K. Takaba, T. Ozawa, M. Takimoto-Kamimura, S. Tanaka, K. Fukuzawa and T. Honma, J. Chem. Inf. Model., 2021, 61, 777–794 CrossRef CAS.
- A. M. Clark and P. Labute, J. Chem. Inf. Model., 2007, 47, 1933–1944 CrossRef CAS.
- Q. Wang, Y. Zhang, L. Wu, S. Niu, C. Song, Z. Zhang, G. Lu, C. Qiao, Y. Hu, K.-Y. Yuen, Q. Wang, H. Zhou, J. Yan and J. Qi, Cell, 2020, 181, 894–904 CrossRef CAS PubMed.
- J. Shang, G. Ye, K. Shi, Y. Wan, C. Luo, H. Aihara, Q. Geng, A. Auerbach and F. Li, Nature, 2020, 581, 221–224 CrossRef CAS.
- S. Tanaka, C. Watanabe and Y. Okiyama, Chem. Phys. Lett., 2013, 556, 272–277 CrossRef CAS.
Footnote |
† Electronic supplementary information (ESI) available: Table S1: IFIE and geometric IFP of XH–Y hydrogen bonds between SARS-CoV-2 S-protein and ACE2. Table S2: IFIE and geometric IFP of XH/π interactions between SARS-CoV-2 S-protein and ACE2. Table S3: IFIE and geometric IFP of XH–Y hydrogen bonds between SARS-CoV-2 S-protein and B38 Fab antibody. Table S4: IFIE and geometric IFP of XH/π interactions between SARS-CoV-2 S-protein and B38 Fab antibody. Table S5: Predicted binding energies between the S-protein and ACE2/B38 Fab antibody without the IFIEs of sugar chain fragments. Table S6: Predicted binding energies (kcal mol−1) between SARS-CoV-2 S-protein WT or N501Y variant and ACE2. Fig. S1: Structural differences on the binding interface of S-protein and ACE2. Fig. S2: Intermolecular interaction energies between SARS-CoV-2 chimeric S-protein and ACE2 in host cells. Fig. S3: Intermolecular interaction energies between SARS-CoV S-protein and ACE2 in host cells. Fig. S4: Intermolecular interaction energies between SARS-CoV-2 S-protein and B38 Fab antibody. Fig. S5: Hot spots of molecular recognition between ACE2 and SARS-CoV-2 S-protein. Fig. S6: Structure difference between the wild-type and N501Y mutant of SARS-CoV-2 S-protein. Fig. S7: Differences of IFIEs of ACE2 between the wild type (N501) and the variant (Y501) on SARS-CoV-2 S-protein. See DOI: 10.1039/d0sc06528e. |
|
This journal is © The Royal Society of Chemistry 2021 |
Click here to see how this site uses Cookies. View our privacy policy here.