DOI:
10.1039/D1SC01133B
(Edge Article)
Chem. Sci., 2021,
12, 9124-9139
Antimicrobial α-defensins as multi-target inhibitors against amyloid formation and microbial infection†
Received
25th February 2021
, Accepted 27th May 2021
First published on 28th May 2021
Abstract
Amyloid aggregation and microbial infection are considered as pathological risk factors for developing amyloid diseases, including Alzheimer's disease (AD), type II diabetes (T2D), Parkinson's disease (PD), and medullary thyroid carcinoma (MTC). Due to the multifactorial nature of amyloid diseases, single-target drugs and treatments have mostly failed to inhibit amyloid aggregation and microbial infection simultaneously, thus leading to marginal benefits for amyloid inhibition and medical treatments. Herein, we proposed and demonstrated a new “anti-amyloid and antimicrobial hypothesis” to discover two host-defense antimicrobial peptides of α-defensins containing β-rich structures (human neutrophil peptide of HNP-1 and rabbit neutrophil peptide of NP-3A), which have demonstrated multi-target, sequence-independent functions to (i) prevent the aggregation and misfolding of different amyloid proteins of amyloid-β (Aβ, associated with AD), human islet amyloid polypeptide (hIAPP, associated with T2D), and human calcitonin (hCT, associated with MTC) at sub-stoichiometric concentrations, (ii) reduce amyloid-induced cell toxicity, and (iii) retain their original antimicrobial activity upon the formation of complexes with amyloid peptides. Further structural analysis showed that the sequence-independent amyloid inhibition function of α-defensins mainly stems from their cross-interactions with amyloid proteins via β-structure interactions. The discovery of antimicrobial peptides containing β-structures to inhibit both microbial infection and amyloid aggregation greatly expands the new therapeutic potential of antimicrobial peptides as multi-target amyloid inhibitors for better understanding pathological causes and treatments of amyloid diseases.
1. Introduction
Protein-misfolding diseases (PMDs) including Alzheimer's disease (AD), type II diabetes (T2D), Parkinson's disease (PD) and medullary thyroid carcinoma (MTC) are complex, multifactorial, age-related disorders, which are generally associated with progressive damage in the localized regions of the central nervous system.1–3 Different evidence-driven hypotheses have been proposed to elucidate the pathological causes of PMDs, although they are still under hot debate. Among them, amyloid aggregation and microbial infection are often considered as the two major pathological causes for initiating and promoting the onset and progression of PMDs. Specifically, the current prevailing “amyloid cascade hypothesis” strongly believes that the misfolding and aggregation of intrinsically disordered proteins into highly ordered, β-structure-rich species (namely amyloids) is mainly responsible for a central pathogenic cause of PMDs,4–6e.g., the abnormal aggregation of Aβ, hIAPP, α-synuclein and hCT is associated with AD, T2D, PD, and MTC, respectively. Significant efforts and progress have been made to develop different types of amyloid inhibitors (i.e., small organic molecules,7,8 nanoparticles,9 antibodies,10,11 polymers,12 and peptides13,14) to prevent the production and aggregation of amyloid proteins. However, these inhibitors are mostly limited to single-target prevention strategies against specific amyloid proteins/aggregates, leading to no success for clinical cures of PMDs. Meanwhile, recent findings have shown the co-existence and mixtures of different amyloid proteins in blood and cerebrospinal fluids, which may correlate with the co-occurrence of different PMDs in the same individuals.15,16 This finding indicates that some different amyloid proteins/aggregates cross-interact with each other to mutually initiate or accelerate the pathogenic event of respective PMDs (this process is known as amyloid cross-seeding), which cannot be simply explained by the “amyloid cascade hypothesis”. The marginal benefits from the “amyloid cascade hypothesis” drive parallel efforts to examine an alternative “microbial infection hypothesis”.17–19 Accumulating genetic, epidemiological, and clinical data have shown that microbial infection caused by herpesvirus type 1–7,20 HIV,21 bacteria,22,23 and fungi24 promotes the overexpression, accumulation, and aggregation of amyloid proteins, thus triggering the neuroinflammation and neurodegeneration of PMDs.17–19,25–29 These findings have renewed our understanding of PMDs, but do not necessarily preclude a biological role of amyloid proteins/aggregates.
Both the “amyloid cascade hypothesis” and “microbial infection hypothesis” have been extensively studied regarding their own molecular mechanisms, thus providing different strategies to design (i) amyloid inhibitors for preventing amyloid aggregation and (ii) antibiotics for preventing microbial infection, most of which do not achieve clinical success.30–33 On the other hand, numerous studies have reported certain pathological links, despite still unknown, between amyloid aggregation and microbial infection,34–36i.e., microbial pathogens are reported to promote the production and overexpression of amyloid proteins (Aβ, prion, and SEVI),37–39 and a number of amyloid proteins (e.g., Aβ, hIAPP, and serum amyloid A) have been identified, which possess antimicrobial activity against several common microorganisms,40–43 while amyloid aggregates are found to induce prominent microbial/virus infection and inflammation.44,45 This indicates that both microbial pathogens and amyloid proteins could work together to form a bidirectional communication system that co-contributes to the pathogenesis of PMDs. Such potential links also suggest that single-target hypotheses and treatments provide marginal benefits for amyloid inhibition, medical treatments, and disease diagnosis. Therefore, it is fundamentally important, but a great challenge for developing a new multiple-target amyloid model that can re-examine or reconcile the two hypotheses for better understanding the pathological causes and links of PMDs.
Considering that (i) both amyloid aggregation and microbial infection are the key pathological causes of PMDs46 and (ii) some amyloid and antimicrobial peptides share certain structural and functional properties, i.e., amyloid proteins (Aβ,40,41 hIAPP,42 and SAA43) possess antibacterial and antifungal activity,47–49 while some antibacterial peptides (protegrin-1,50 plantaricin A,51 uperin 3.5,52 magainin,53,54 and dermaseptin S9 (ref. 55)) have amyloid-like aggregation behaviors, here we proposed and demonstrated a new “anti-amyloid and antimicrobial hypothesis” by discovering several host-defense antimicrobial peptides of α-defensins containing β-sheet structures (human neutrophil peptide of HNP-1 and rabbit neutrophil peptide of NP-3A), which possess multi-target inhibition functions against both amyloid aggregation and microbial infection. Specifically, both HNP-1 and NP-3A demonstrate multi-target functions to (i) prevent the aggregation and misfolding of different amyloid-β (Aβ, associated with AD), human islet amyloid polypeptide (hIAPP, associated with T2D), and human calcitonin (hCT, associated with MTC), (ii) reduce amyloid-induced cell toxicity, and (iii) kill four common microorganisms against microbial infection. Further structural analysis by molecular dynamics simulations showed that the new amyloid inhibition function of α-defensins mainly stems from their cross-interactions with amyloid proteins via β-structure interactions. This work provides a new “kill two birds with one stone” model to not only reconcile both the “amyloid cascade hypothesis” and “microbial infection hypothesis”, but also reveal the functional and structural correlations between antimicrobial peptides and amyloid proteins with their built-in bacterial killing and amyloid inhibition functions.
2. Results
Considering that β-rich structures are the common structural motifs in amyloid aggregates, irrespective of their sequences, we propose a testable “conformational selection binding” hypothesis by selecting α-defensins containing β-structures (or any other β-structure-forming peptide) to interact with the conformationally similar β-structures of amyloid aggregates via β-structure interactions, in which α-defensins–amyloid interactions will competitively reduce amyloid–amyloid interactions, thus preventing amyloid aggregation and amyloid-induced toxicity. Two α-defensins of the human neutrophil peptide of HNP-1 and the rabbit neutrophil peptide of NP-3A were selected as amyloid inhibitors, because they both consist of three β-strands, stabilized by three pairs of intramolecular disulfide bonds, oriented in an antiparallel way, and linked by short loops (Fig. S1a†). While both α-defensins have similar structures, they only exhibit 26% sequence similarity with different net charges (+3e in HNP-1 and +8e in NP-3A). The high structural similarity and diverse sequences of both α-defensins allow us to examine the conformational-specific, sequence-independent inhibition function against the aggregation of Aβ associated with AD, hIAPP associated with T2D, and hCT associated with MTC.
2.1 α-Defensins exhibit a general amyloid inhibition ability
To test the amyloid inhibition function of α-defensins, we first investigated the inhibition properties of both α-defensins (HNP-1 and NP-3A) against the aggregation of Aβ, hIAPP, and hCT in vitro using ThT, AFM, and TEM. Freshly prepared Aβ, hIAPP, and hCT (25 μM) were separately incubated with HNP-1 or NP-3A at different molar ratios of 0.004–2 and 37 °C for 24–32 h. ThT kinetic profiles in Fig. S1† showed that both HNP-1 and NP-3A enabled the inhibition of the aggregation of Aβ, hIAPP, and hCT at sub-stoichiometric concentrations (≤equimolar ratio) in a dose-dependent manner. Specifically, ThT data in Fig. 1b showed that at an equal molar ratio of χ = 1, HNP-1 can completely suppress the amyloid fibril formation of the three different amyloid peptides as evidenced by almost 0% relative ThT intensity. Even at χ = 0.004, HNP-1 was able to largely reduce Aβ fibrils by 30%, hIAPP fibrils by 33%, and hCT fibrils by 31%, respectively. TEM images (Fig. 1c) and AFM images (Fig. S2†) also showed that the co-incubation HNP-1–amyloid samples exhibited much less fibrillar aggregates than pure amyloid samples at each aggregation stage, confirming that HNP-1 greatly reduces the formation of amyloid fibrils, consistent with ThT results. Consistently, another α-defensin of NP-3A also exhibited a similar dose-dependent inhibition effect on the three amyloid aggregations. The increase of the NP-3A
:
amyloid molar ratio from χ = 0.004 to χ = 1 led to a large reduction in ThT signals by 69–100% (Fig. S3b†), the formation of less-fibrillar, amorphous-like aggregates (Fig. S2†), and the retention of the original disordered structures (Fig. S3c†). This finding provides preliminary evidence to support our hypothesis that α-defensins containing β-structures show a sequence-independent inhibition ability to prevent the misfolding and aggregation of Aβ, hIAPP, and hCT.
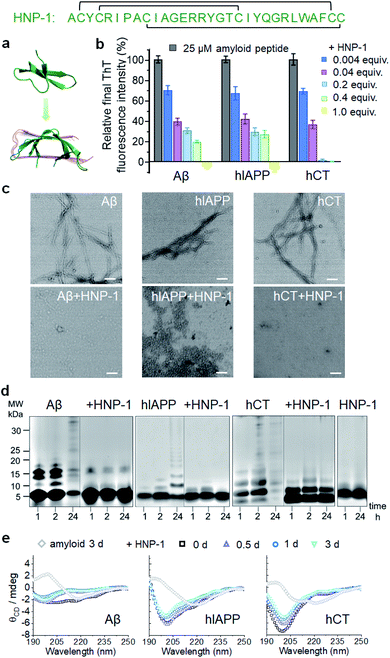 |
| Fig. 1 HNP-1 exhibits general inhibition properties against the fibrillization of different amyloid peptides. (a) Sequence and structure of α-defensin HNP-1 with a β-rich structure. (b) Dose-dependent inhibition effect of HNP-1 on Aβ, hIAPP, and hCT aggregation by ThT fluorescence assays. Inhibition efficiency of HNP-1 is determined by the relative final fluorescence intensity (%) normalized by that of pure amyloid aggregation. Equivalent value of HNP-1 is defined by the molar ratio of the HNP-1 : amyloid peptide. Error bar represents the standard deviation (s. d.) of triplicate measurements. (c) TEM image of 25 μM Aβ, hIAPP, and hCT in the absence or presence of an equimolar concentration of HNP-1. Samples were prepared after 3 days of incubation in a physiological environment (pH 7.4 and 37 °C). Scale bars = 200 nm. (d) SDS-PAGE characterization of 25 μM Aβ, hIAPP, and hCT homo-/hetero-assemblies in the absence or presence of 25 μM HNP-1. (e) Circular dichroism (CD) spectra of 25 μM Aβ, hIAPP, and hCT in the absence (gray diamond, control) or in the presence of HNP-1 after 0, 0.5, 1 and 3 days of incubation. HNP-1 was added to Aβ or hIAPP solution at 25 μM, but to hCT solution at 10 μM. | |
To better understand the possible inhibition pathways of both α-defensins imposed on amyloid aggregation, we further characterized the size distributions and structural transitions of α-defensins–amyloid co-assemblies using SDS-PAGE and CD. SDS-PAGE gels in Fig. 1d showed that upon co-incubation of α-defensins and amyloids at an equal molar ratio for 24 h, both HNP-1 and NP-3A displayed two major bands at 4–5 kDa of monomers and 7–8 kDa of dimers, indicating that they do not self-aggregate into large species at 25 μM. In contrast, all three amyloid peptides (Aβ, hIAPP, or hCT) displayed typical time-dependent, self-aggregation bands, which were initially dominated by small monomers and oligomers of 5–15 kDa at 1–2 h and then gradually shifted to large aggregates of >25 kDa at 24 h. The co-incubation of HNP-1 with amyloid peptides revealed the size distribution of HNP-1–amyloid co-assemblies to be located at ∼5 kDa and 16 kDa within 24 h, indicating that HNP-1 indeed suppresses the aggregation of amyloid peptides by stabilizing their monomeric or small oligomeric states and thus preventing amyloid peptides from growing into large species. Consistently, the co-incubation of NP-3A with amyloid peptides showed similar size distributions in SDS-PAGE gels, i.e., small homo-/hetero-assemblies of 5–20 kDa for NP-3A–Aβ, 5–10 kDa for NP-3A–hIAPP, and 5–15 kDa for NP-3A–hCT were retained almost unchanged (Fig. S3d†). Furthermore, time-dependent CD spectra in Fig. 1e and S3e† further showed that the presence of HNP-1 or NP-3A interfered with the structural transition of amyloid aggregates (Aβ, hIAPP and hCT) from disordered structures to β-sheet structures, as evidenced by almost unchanged CD curves and a minimal peak at ∼200 nm.
Taken together, side-by-side comparison between HNP-1–amyloid and NP-3A–amyloid co-assemblies revealed concentration- and sequence-dependent inhibition effects, i.e., (i) HNP-1 generally has better amyloid inhibition properties than NP-3A. NP-3A usually requires higher concentrations to achieve similar amyloid inhibition efficiency than HNP-1. (ii) HNP-1 and NP-3A inhibit the aggregation of different amyloid peptides in the same order hCT > Aβ > hIAPP. (iii) Both α-defensins (HNP-1 and NP-3A) displayed concentration-dependent inhibition pathways against amyloid formation. α-Defensins at low concentrations are more effective to slow down amyloid growth from small aggregates to larger ones, while those at higher concentrations favor the inhibition of the amyloid nucleus at a lag phase. Such differences in amyloid inhibition suggest the existence of cross-species energy barriers between different α-defensin and amyloid proteins.
2.2 α-Defensins bind to amyloid aggregates to induce their inhibition effects
In principle, the amyloid inhibition ability of any molecule (e.g., α-defensins) mainly stems from its strong interactions with amyloid peptides, because these inhibitor–amyloid interactions will competitively reduce amyloid–amyloid interactions and thus prevent amyloid aggregation. Here, to understand the binding-induced amyloid inhibition mechanism of α-defensins, we quantified the binding affinity and preference of α-defensins (HNP-1 and NP-3A) to different amyloid peptides (Aβ, hIAPP, and hCT) using surface plasmon resonance (SPR), ThT, and molecular simulations. Briefly, monomeric Aβ, hIAPP, and hCT were first covalently immobilized on a SPR chip surface via direct amine-coupling, followed by HNP-1 or NP-3A solutions of different concentrations (Fig. S5a, and b†). In general, SPR sensorgrams (Fig. 2a & S6a†) showed several common α-defensins–amyloid binding scenarios. At a first glance, both α-defensins showed a general, strong binding ability to all three different amyloid peptide-coated SPR surfaces in a concentration-dependent way, i.e., the number of α-defensins bound to the amyloid peptide-coated surfaces increased with their flowing concentrations. Second, HNP-1 exhibited a higher binding affinity to amyloid peptides than NP-3A, consistent with their amyloid inhibition properties. This again supports the α-defensin binding-induced amyloid inhibition mechanism. Third, the kinetic analysis of SPR sensorgrams yielded the binding affinity (KD, binding constant) of each sample (Fig. S5c, and d†). Specifically, the KD values of HNP-1 binding to Aβ, hIAPP, and hCT were 0.6 μM, 17.6 μM, and 1.6 μM, respectively, indicating that HNP-1 exhibits comparably high binding affinity to Aβ and hCT, but low binding to hIAPP. In parallel, NP-3A displayed an increasing order of binding affinity to amyloid peptides of hIAPP (KD = 27.6 μM) < Aβ (KD = 10.6 μM) < hCT (KD = 9.9 μM). The different binding affinities explain the amyloid inhibition efficiency of α-defensins to different amyloid peptides.56
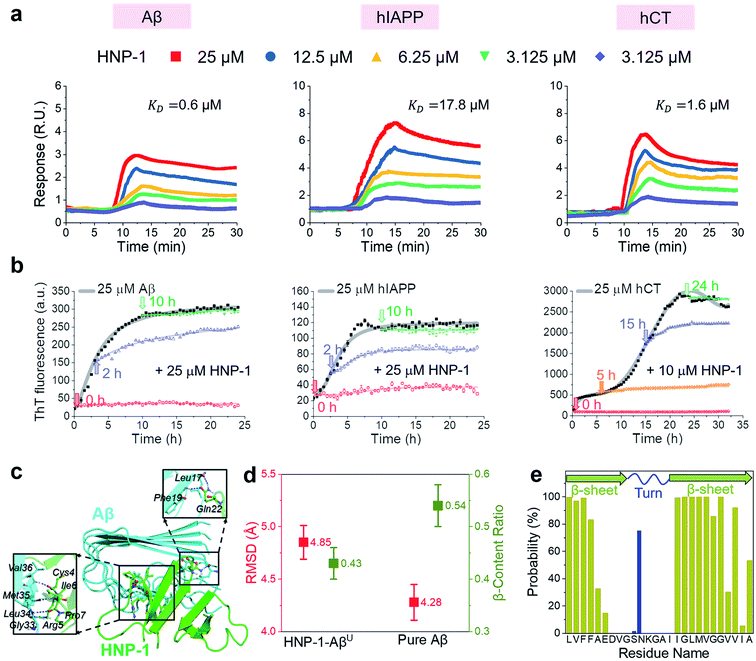 |
| Fig. 2 HNP-1 binds to amyloid aggregates to induce its amyloid inhibition effects. (a) SPR sensorgrams of the concentration-dependent binding of HNP-1 to Aβ-, hIAPP-, and hCT-coated surfaces. Binding affinity between HNP-1 and amyloid peptides is determined by the binding constant (KD) based on the Langmuir model (Fig. S5†). (b) Inhibition effect of HNP-1 on Aβ, hIAPP, and hCT seeds preformed at different aggregation stages by ThT fluorescence assay. Arrows indicate the time points of adding HNP-1 to specific amyloid seed solutions. (c) Binding of HNP-1 to the U-turn region of the Aβ pentamer determined from MD simulations via nonbonded interactions. At the Aβ/HNP-1 binding interface, Leu17, Phe19, Gly33, Leu34, Met35, and Val36 of Aβ showed a strong binding preference to Gln22, Cys4, Arg5, Ile6, and Pro7 of HNP-1 via hydrogen bonding and hydrophobic interactions. (d) Structural characterization and comparison of Aβ pentamers in the presence and absence of HNP-1 binding by using the RMSD (red) and β-content ratio (green). (e) Binding probability (%) of HNP-1 to Aβ residues. | |
Next, it is also important to examine the binding preferences (inhibition potency) of α-defensins to specific amyloid aggregates at different aggregation phases (e.g., monomers, oligomers, or fibrils). To this end, we added α-defensins of specific concentration to a specific amyloid seed solution (25 μM) prepared at the growth and equilibrium phases, followed by monitoring the change of ThT signals before and after adding α-defensins. The selection of α-defensin concentration was determined by the complete inhibition of amyloid aggregation from freshly prepared monomers (Fig. 1b and S3b†), i.e., 25 μM of HNP-1 and NP-3A to Aβ seed solutions, 25 μM of HNP-1 and 50 μM of NP-3A to hIAPP seed solutions, and 10 μM of HNP-1 and 25 μM of NP-3A to hCT seed solution. As shown in Fig. 2b and S6b,† ThT curves clearly showed that the addition of α-defensins to amyloid seeds at the growth phase immediately slowed down amyloid growth into higher order species, finally leading to a 34%/62%, 46%/54%, and 44%/52% decrease of Aβ, hIAPP, and hCT fibrils by HNP-1/NP-3A, respectively. However, both HNP-1 and NP-3A did not affect the growth of amyloid seeds preformed at the equilibrium phase into amyloid fibrils, as evidenced by the almost unchanged ThT curves. Thus, both HNP-1 and NP-3A enable the prevention of the aggregation of amyloid monomers and oligomers at early aggregation stages, but not amyloid protofibrils or fibrils at later stages, revealing different inhibition pathways of α-defensins by either stabilizing amyloid monomers or blocking the elongation of amyloid oligomers.
To better understand the amyloid binding mechanism of α-defensins on the atomic scale, we computationally study the binding structures, affinities, and residues between the HNP-1 dimer and amyloid pentamer (Aβ and hIAPP) using a combination of molecular docking and molecular dynamics (MD) simulations.57–59 A HNP-1 dimer was selected because it is a functional unit to form a dimeric pore to disrupt bacterial membranes with a crystal structure available,60,61 while Aβ and hIAPP pentamers were used because they were experimentally identified as highly populated aggregates.62–64 Briefly, we first applied PatchDock65 to generate a pool of binding models of HNP-1–Aβ and HNP-1–hIAPP based on the rigid shape complementarity docking principle, followed by structural optimization using FireDock with flexible residue refinement.66 As a result, the top ten binding complexes of HNP-1–Aβ and HNP-1–hIAPP were collected to obtain the most possible binding modes at lower energy states (Fig. S7†). At the first glance, while these HNP-1–amyloid assemblies exhibited different binding structures, they can be classified into two similar binding modes, i.e., HNP-1 displayed favorable binding to either the β-sheet region or the U-turn regions of Aβ and hIAPP pentamers. These two binding modes suggest the two possible amyloid inhibition pathways of HNP-1, i.e., binding of HNP-1 to the β-sheet and U-turn regions of amyloid aggregates allows blocking of the lateral association and elongation pathways of amyloid aggregation. Further energy analysis revealed that different HNP-1–amyloid complexes were stabilized by favorable interfacial interactions, but vdW and electrostatic interactions contributed differently to different HNP-1–amyloid assemblies, strongly depending on interfacial residues and their packing details (Fig. S7†).
We then selected four HNP-1–amyloid assemblies, each presenting a distinct interfacial binding mode of HNP-1–AβC, HNP-1–AβU, HNP-1–hIAPPC, and HNP-1–hIAPPU (square ones in Fig. S7†), for subsequent all-atom, explicit-water MD simulations to determine their dynamic binding behaviors. The visual inspection of MD trajectories showed that in sharp contrast to unstable HNP-1–AβC and HNP-1–hIAPPC, HNP-1–AβU (Fig. 2c) and HNP-1–hIAPPU (Fig. S8a†) were found to be more structurally stable, in which no interfacial separation and no peptide disassociation between and within HNP-1 and amyloid pentamer were observed. On the other hand, the presence of HNP-1 induced a slight increase of the RMSD of Aβ from 4.28 to 4.85 Å (Fig. 2d) and hIAPP from 3.13 to 3.79 Å (Fig. S8b†) and a decrease of the β-content of Aβ from 0.54 to 0.43 (Fig. 2d) and hIAPP from 0.62 to 0.58 (Fig. S8b†). This indicates that HNP-1 not only has a higher probability to prevent the elongation of Aβ or hIAPP from growing in their U-turn regions, but also disturbs the overall and secondary structures of Aβ or hIAPP, in which both effects improve the amyloid inhibition capacity of HNP-1. We further computationally identified whether HNP-1 has binding preferences to certain Aβ or hIAPP residues using the averaged contact probabilities between each amyloid residue and HNP-1 based on their atomic contacts of HNP-1 within 4 Å of each amyloid residue (Fig. 2e & S8c†). HNP-1 exhibited inhomogeneous residue binding probability to both Aβ and hIAPP pentamers, clearly indicating that HNP-1 favored to interact with certain residues over others. Specifically, HNP-1 showed high binding probabilities to Leu17, Val18, Phe19, and Phe20 from the N-terminal β-sheet and Ile32, Gly33, Leu34, Met35, Val36, Gly37, Gly38, and Val40 from the C-terminal β-sheet of the Aβ pentamer (Fig. 2c). Differently, HNP-1 preferred to bind to Leu27, Ser29, and Asn31 from the C-terminal β-sheet of hIAPP. Close-up investigation revealed the continuous formation of hydrogen bonds along interfacial structures, which serve as glue to associate HNP-1 and amyloid pentamers (Fig. S8a†).
2.3 α-Defensins reduce amyloid-induced cell toxicity
Due to the complex pathological nature of amyloids, the inhibition of amyloid aggregation does not necessarily reduce amyloid-induced cell toxicity. Here, we applied MTT, LDH, and live/dead assays to study (i) Aβ- and hCT-induced cytotoxicity using the human neuroblastoma SH-SY5Y cell line and (ii) hIAPP-induced β-cell dysfunction using the rat pancreatic insulinoma RIN-5fm cell line. As controls, both HNP-1 and NP-3A were almost non-toxic to SH-SY5Y and RIN-5fm cells (86–100% cell viability at 1–25 μM concentration), while Aβ, hCT, and hIAPP (25 μM) alone significantly decreased cell viability to 67%, 69%, and 58%, respectively. MTT assays further showed that the co-incubation of HNP-1 with the three different amyloid proteins (25 μM) exhibited dose-dependent inhibition properties for rescuing cells from amyloid-induced toxicity to different extents. Under optimal conditions, HNP-1 increased the cell viability from 67% to 90% with Aβ, from 69% to 97% with hCT, and from 58% to 82% with hIAPP, respectively (Fig. 3a). Furthermore, visual inspection by live/dead cell assays also showed that the introduction of HNP-1 (5 μM) allowed the significant reduction of the number of dead cells (red stains) for each cell line, again confirming the protective role of HNP-1 from amyloid-induced cell toxicity (Fig. 3c).
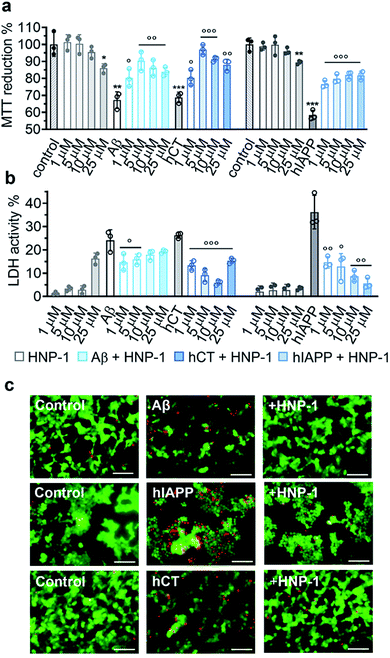 |
| Fig. 3 HNP-1 rescues mammalian cells from amyloid-induced cell toxicity. Dose-dependent protection effect of HNP-1 against amyloid Aβ-, hCT-, and hIAPP-induced (a) cell toxicity determined by MTT assay and (b) cell membrane disruption determined by LDH assay. Cells were incubated with amyloid peptides (25 μM) for 24 h in the absence or presence of 1–25 μM HNP-1. Untreated cells were set as controls for 100% MTT reduction and 0% LDH activity, cells after lysis were set for 100% LDH activity, and cells only incubated with HNP-1 (grey bars) were also analyzed for comparison. All data represent mean ± s. d. of three independent experiments. Statistical analysis (n = 3) was conducted for cells treated with HNP-1 or amyloid peptides alone relative to the control (○p < 0.05; ○○p < 0.01; ○○○p < 0.001), as well as cells treated with both HNP-1 and amyloid peptides relative to cells treated with amyloid peptides alone (*p < 0.05; **p < 0.01; ***p < 0.001). (c) Representative fluorescence microscopy images of cells treated with freshly prepared amyloid peptides (25 μM) in the absence or presence of 5 μM HNP-1. Untreated cells were set as a control. Red and green fluorescence indicate dead and live cells, respectively. Scale bars = 100 μm. | |
Numerous studies have shown that amyloid-induced cell toxicity is likely attributed to the disruption and leakage of cell membranes.67,68 To explore the protection mechanism of HNP-1 against amyloid-induced cell apoptosis, we examined the effect of HNP-1 (1–25 μM) on modulating the amyloid-induced membrane leakage using LDH assays. As controls, while both HNP-1 and NP-3A are known to permeabilize the bacterial cell membrane and kill broad-spectrum of bacteria,69,70 neither HNP-1 (Fig. 3b) nor NP-3A (Fig. S9b†) induced obvious membrane leakage of SH-SY5Y and RIN-5fm cells (≤5% LDH activity at 1–10 μM and ≤10% for NP-3A at 25–50 μM). More interestingly, HNP-1 exhibited a concentration-dependent membrane-disruption ability to SH-SY5Y cells where LDH activity increased from ∼1% at 1 μM to ∼16% at 25 μM but was inert to RIN-5fm cells. In contrast, Aβ-, hCT-, and hIAPP-treated cells experienced a significant membrane leakage (LDH activity) of 24%, 26%, and 36%, respectively (Fig. 3b). For comparison, under optimal conditions, HNP-1 reduced Aβ-induced membrane leakage by 39%, hIAPP-induced membrane leakage by 84%, and hCT-induced membrane leakage by 77%, relative to HNP-1-untreated controls (Fig. 3b).
In parallel, NP-3A alone was also found to be non-toxic to both SH-SY5Y and RIN-5fm cells (≥95% cell viability at 1–50 μM) and non-susceptible to their membrane disruption (≤5% LDH activity at 1–25 μM) (Fig. S9a and b†). The co-incubation of NP-3A with the three different amyloid peptides indeed improved cell viability to 81–92% and decrease cell apoptosis to 5–16%, as compared to the amyloid-induced cell viability of 57–69% and cell apoptosis of 26–36% (Fig. S9a and b†). Collective cell data suggest several mechanistic possibilities for α-defensins to reduce amyloid-induced toxicity. Strong interactions between α-defensins and amyloid peptides could reduce the formation of toxic amyloid aggregates that are highly active to disrupt cell membranes, form less or nontoxic α-defensin–amyloid complexes that are largely inert to cell membranes, and competitively decrease the binding propensity of amyloids to cell membranes, all of which endow α-defensins with an improved cell protection function.
2.4 α-Defensins, amyloid peptides, and cross-species of α-defensins–amyloid peptides retain their antimicrobial activity
Microbe-induced neuroinflammation is considered as a potential risk factor that triggers the pathologies of amyloid diseases.71–74 Given the facts that (i) α-defensins have an intrinsic antimicrobial function; (ii) Aβ75 and hIAPP76 have been identified with new antimicrobial activities against bacteria, fungi, and viruses, it still remains unknown whether cross-species of α-defensins–amyloids can retain antimicrobial activities; and (iii) antimicrobial and amyloid peptides share some commonality to kill their targets by disrupting cell membranes in some scenarios,77–79 we examined the antimicrobial activity of α-defensins, amyloid proteins, and their complexes using Gram-negative bacterial strains of E. coli and P. aeruginosa and Gram-positive bacterial strains of S. aureus and S. epidermidis. The bacteria growth profiles in Fig. S10† demonstrated the broad-spectrum antimicrobial properties of α-defensins (HNP-1 and NP-3A) and amyloid peptides (Aβ and hIAPP), but with different antimicrobial efficiencies. Specifically, α-defensins (10–50 μM) exhibit an overall higher antimicrobial activity against Gram-positive bacteria than against Gram-negative bacteria80 (Fig. S10†). Also, HNP-1 showed higher antimicrobial activity against all four different bacterial strains than NP-3A. Both Aβ and hIAPP against different bacteria was in a descending order of S. aureus ≈ P. aeruginosa > E. coli, with an exception of S. epidermidis that was not susceptible to Aβ and hIAPP. Differently, among the four different bacterial strains, hCT only inhibited the growth of P. aeruginosa.
Upon demonstrating the antimicrobial activities of α-defensins (HNP-1 and NP-3A) and amyloid peptides (Aβ and hIAPP), we further tested the antimicrobial activity of co-assemblies of α-defensins and amyloid peptides as compared to that of native α-defensins or amyloid peptides. Considering that amyloid peptides were expected to be largely sequestered in reaction with α-defensins, here we used the antimicrobial activity of α-defensins as a basis for evaluating the antimicrobial activities of α-defensins–amyloid assemblies. As a result, α-defensins–amyloid assemblies generally presented a comparable or even higher antimicrobial efficiency than the corresponding α-defensins. Of note, there were several exceptions due to the complex cross-species interactions between the two different families of antimicrobial and amyloid peptides. Aβ–HNP-1 assemblies displayed minor antimicrobial activity against E. coli (95.2%) and P. aeruginosa (70.5%), as compared to the HNP-1-induced antimicrobial activity of E. coli (73.8%) and P. aeruginosa (45.7%) and the Aβ-induced antimicrobial activity of E. coli (78.6%) and P. aeruginosa (41.7%) (Fig. 4a). In another case of NP-3A–hIAPP assemblies, NP-3A–hIAPP assemblies (37.9%) exhibited less antimicrobial activity against S. epidermidis than NP-3A (19.4%) (Fig. S11b†). Additionally, we further monitored the bacterial membrane integrity by using fluorescent probes. Fluorescence microscopy showed that all α-defensins–amyloid assemblies caused massive red fluorescent signals, an indicator of a damaged membrane, in representative Gram-negative E. coli (Fig. 4b & S11c†) and Gram-positive S. aureus (Fig. 4c & S11d†), further confirming that α-defensins–amyloid assemblies still retain bacterial killing properties.
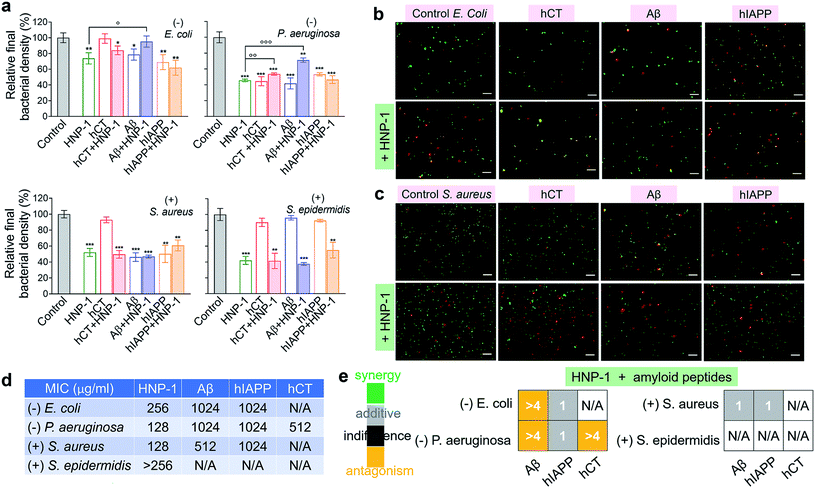 |
| Fig. 4 Cross-species of HNP-1–amyloids retain a broad-spectrum antimicrobial activity against both Gram-negative and Gram-positive bacteria. (a) Antimicrobial activity of HNP-1 (10 μM), amyloid peptides (25 μM), and cross-species of HNP-1–amyloids against Gram-negative bacteria of E. coli and P. aeruginosa and Gram-positive bacteria of S. aureus and S. epidermidis. Bacterial density is determined by OD600 and relative bacterial density (%) was determined by the ratio of OD600 values from untreated (100%) and peptide-treated bacteria assays. Statistical analysis (n = 3) of all samples was conducted relative to control (*p < 0.05; **p < 0.01; ***p < 0.001) and HNP-1-treated bacteria (○p < 0.05; ○p < 0.01). Representative fluorescence microscopy images of (b) Gram-negative E. coli bacteria and (c) Gram-positive S. aureus bacteria treated with or without peptides (25 μM amyloid peptides and 10 μM HNP-1). Red fluorescence of propidium iodide and green fluorescence of SYTO 9 were used to identify dead bacteria with damaged membranes and live bacteria with intact membranes, respectively. Scale bars = 20 μm. (d) Minimal inhibitory concentration (MIC) of HNP-1 and amyloid peptides against bacteria. (e) Fractional inhibitory concentration (FIC) index of the combination of HNP-1 and amyloid peptides as calculated from the checkerboard assay as shown in Fig. S12.† Synergy (FIC ≤ 0.5), additive (0.5 < FIC ≤ 1), indifferent (1 < FIC ≤ 4), and antagonism (FIC > 4). | |
In addition, the antibacterial potency of α-defensins and amyloids in combination was quantitively evaluated by checkerboard assay (Fig. S12†). Considering that S. epidermidis was not susceptible to Aβ, hIAPP, and hCT, while hCT can actively inhibit P. aeruginosa (Fig. 4a), herein we purposely designed an antibacterial checkerboard assay to evaluate the combination antimicrobial activity of (1) amyloid (Aβ/hIAPP)–α-defensins (HNP-1/NP-3A) against E. coli, P. aeruginosa, and S. aureus and (2) amyloid hCT–α-defensins (HNP-1/NP-3A) against P. aeruginosa. We firstly analyzed the MICs of pure amyloid peptides and pure α-defensins in the checkerboard assay, whose MIC values are set as controls and defined as the lowest concentrations to quantify the combined effect of amyloid peptides and α-defensins. As shown in Fig. 4d & S11e,† HNP-1 showed higher antibacterial activity (i.e., lower MIC values) against all bacterial strains than NP-3A. HNP-1 displayed a comparable inhibition effect to P. aeruginosa and S. aureus (MICs = 128 μg ml−1), but lower inhibition efficiency to E. coli (MIC = 256 μg ml−1) and S. epidermidis (MIC > 256 μg ml−1). NP-3A exhibited low antimicrobial activity to all of the four bacteria with MICs ≥ 256 μg ml−1. Both Aβ and hIAPP peptides exhibited similar antibacterial efficiency against E. coli and P. aeruginosa (MICs = 1024 μg ml−1), but Aβ (MIC = 512 μg ml−1) was more effective to inhibit Gram-positive S. aureus than hIAPP (MIC = 1024 μg ml−1). hCT had a MIC value of 512 μg ml−1 against P. aeruginosa growth. As shown in Fig. 4e & S11f,† in most cases, the combination of α-defensins and amyloids led to a FIC index of 0.75–1.5, suggesting no or a slight increase in inhibitory activity as compared to the sum of individual FIC values of α-defensins and amyloids alone. Meanwhile, the combination of HNP-1 and Aβ resulted in a FIC index of >4 against both E. coli and P. aeruginosa, likely indicating an antagonism effect of their assemblies with lower antibacterial activity. In another combination case of HNP-1 and hCT, a FIC value of >4 suggests reduced antibacterial activity against P. aeruginosa, in contrast to the increase inhibitory activity of NP-3A and Aβ against P. aeruginosa (FIC index = 0.5). Of note, similar to many challenges in antimicrobial studies (e.g., multi-drug-resistance, bacterial membrane disruption, and metal/oxide-induced bacterial killing effects), while this study has revealed different antimicrobial activities of combined antimicrobial and amyloid peptides (beyond α-defensins, Aβ, hIAPP, and hCT), the underlying antimicrobial mechanisms induced by amyloid peptides still remain fundamentally unclear.
The different antimicrobial efficiencies of α-defensins–amyloid assemblies indicate different interaction patterns to (i) prevent or promote amyloid aggregation, (ii) remodel mutual conformational changes of α-defensins and amyloids, (iii) change their native functions of antimicrobial activity, self-aggregation, or both, and (iv) modulate their membrane disruption properties, all of which depend on the complex interplays of the sequence-structure-interaction between α-defensins and amyloid in the context of cell membranes, thus leading to different antimicrobial scenarios. For instance, numerous studies have shown that Gram-negative bacteria normally possess a higher negative surface charge than Gram-positive ones, thus undergoing a larger surface perturbation in response to electrostatic interactions with antimicrobial agents.81,82 Of note, HNP-1, NP-3A, and hIAPP are cationic peptides with a net charge of +3e, +8e, and +2e, while Aβ is anionic with a net charge of −3e. Electrostatic attractions make the formation of α-defensins–Aβ assemblies relatively easy at the expense of the decrease of cationic charges, which in turn decrease their membrane disruption properties,83,84 thus explaining the lower antimicrobial performance of α-defensins–Aβ assemblies as compared to α-defensins alone.
3. Discussion
Abnormal protein aggregation and microbial infection are considered as major pathological risk factors for initiating and promoting the onset and progression of PMDs.27–29 While the exact pathological link between the two risk factors is still largely unknown, different prevention and treatment strategies as driven by the “amyloid cascade hypothesis” and “microbial infection hypothesis” have not achieved any clinical success. Due to the multifactorial nature of PMDs, the single-target prevention hypotheses and strategies, in which amyloid inhibitors prevent the abnormal aggregation of amyloid proteins, while antimicrobial peptides inhibit microbial infection, provide marginal benefits for amyloid inhibition and medical treatments. On the other hand, a number of amyloid peptides (Aβ, hIAPP, SAA, and prion proteins) have been identified with antimicrobial activity,41,43,49,76,85 while several antimicrobial peptides (protegrins, dermaseptin S9, and uperin 3.5) enable the formation of β-structure-rich, amyloid-like fibrils.50,52,55,86–88 While antimicrobial and amyloid peptides belong to different families of biomolecules with distinct native functions, such mutual common functionalities provide a fundamental basis to developing multi-target amyloid inhibitors to block different pathological pathways of PMDs.
To this end, we propose a new “anti-amyloid and antimicrobial hypothesis” to explore and identify antimicrobial α-defensins with a new repurposing function of amyloid inhibition, which serve as multi-target inhibitors to prevent microbial infection and amyloid aggregation both pathologically linked to PMDs. Our working hypothesis is that apart from the intrinsic antimicrobial activity of α-defensins, β-rich structures in α-defensins are expected to interact with conformationally similar β-structure-rich amyloid aggregates via conformational selective binding mode, which will in turn reduce amyloid–amyloid interactions and thus inhibit amyloid aggregation. To test these conformational-specific, sequence-independent amyloid inhibition properties of α-defensins, we selected two different β-structure-rich α-defensins (HNP-1 and NP-3A) to inhibit the misfolding and aggregation of three amyloid peptides (Aβ, hIAPP, and hCT) at different aggregation stages from monomers to oligomers to protofibrils.
First, upon co-incubation of α-defensins with freshly prepared amyloid monomers, both HNP-1 and NP-3A at sub-stoichiometric concentrations (0.1–25 μM) exhibited a general and strong amyloid inhibition ability to prevent the amyloid aggregation of the three different amyloid peptides to different extents (Fig. 1 & S1–S4†), independent of amyloid sequences. It should be noted that current dual-target89,90 or multi-target inhibitors91,92 require 2–20 molar ratios to achieve at least 50% amyloid inhibition. For comparison, both α-defensins at equimolar concentration can completely suppress the three amyloid fibrillizations, while α-defensins at even 0.004 molar ratios can still achieve 20–33% amyloid inhibition. Further analysis by CD and SDS-PAGE revealed the α-defensins can stabilize disordered amyloid monomers, block the growth of small amyloid aggregates into larger ones, or both (Fig. 1d, e, S3d and e†), indicating the two different amyloid inhibition pathways of α-defensins.
Moreover, cross-seeding experiments in Fig. 2b and S6b† showed that both α-defensins can also interact with different amyloid seeds preformed at the growth phase to prevent their aggregation into final mature fibrils. However, once higher-ordered amyloid protofibrils are formed, α-defensins do not have the ability to either disassemble or inhibit these amyloid aggregates. These findings confirm that α-defensins are more effective to inhibit the aggregation and conversion of amyloid species formed at the early lag and growth phases, but unable to prevent the amyloid fibrillation of higher-order protofibrils preformed at the equilibrium phase.
Comparative molecular simulations confirmed that on one hand, the greater common inhibition of α-defensins is to establish strong interaction with the target amyloid aggregates to prevent amyloid–amyloid interactions for their self-aggregation. α-Defensins showed a strong binding preference to the turn and β-sheet regions, both of which involve highly populated β-structures in both Aβ and hIAPP aggregates (Fig. S7†). On the other hand, different α-defensin–amyloid systems involved different β-structure binding sites with different binding populations and binding affinities, thus suggesting different routes to inhibit amyloid formation by α-defensins. The binding of α-defensins to the β-sheet groove regions of amyloids is to prevent the lateral association between different amyloid aggregates, while the binding of α-defensins to the turn region is to prevent the addition of amyloid peptides to the edges of amyloid aggregates. Additionally, the binding of α-defensins also disturbed the local structure of amyloid aggregates, which provide an additional steric barrier for amyloid aggregation.
As multi-target inhibitors, apart from showing intrinsic antimicrobial activity and low/non-toxicity to mammal cells,78,93 α-defensins are also required to (i) rescue mammalian cells from amyloid-induced cell toxicity and (ii) retain their antimicrobial activity in the presence of amyloid peptides. MTT cell assays confirmed that α-defensin-treated cell samples are enabled to rescue cells from both Aβ-, hIAPP-, and hCT-induced toxicity by 70–71%, 42–57%, and 74–90% (Fig. 3a & S9a†). LDH assays explained the protective role of α-defensins against cell toxicity due to the suppression of toxic amyloid aggregates, the formation of less toxic α-defensins–amyloid assemblies, and the prevention of membrane leakage. More importantly, α-defensins–amyloid assemblies mostly possessed a similar or even better antimicrobial activity against the growth of both Gram-negative and Gram-positive bacteria, in comparison with pure α-defensins (Fig. 4 & S10–S12†).
Conventional single-target, sequence-dependent amyloid inhibitors or antimicrobial agents usually preserve their broad-spectrum inhibition activity at the expense of inhibition efficiency (Fig. 5).94–96 Differently, we demonstrated a new strategy by repurposing antimicrobial peptides to simultaneously achieve both general and strong amyloid inhibition and to prevent both amyloid aggregation and antimicrobial infection, both of which are pathologically linked to the causes of PMDs. α-Defensins as multi-target, sequence-independent inhibitors present general and strong inhibition activity against the aggregation of other amyloid peptides (not limited to Aβ, hIAPP, and hCT) at different aggregation stages. This work not only demonstrates new antimicrobial-based amyloid inhibitors and prevention strategies beyond the few available today, but also provides a new strategy to design/discover β-structure-rich antimicrobial peptides as amyloid inhibitors, which will greatly expand potential therapeutic drugs for amyloid diseases. More fundamentally, cross-species interactions between the two different families of antimicrobial and amyloid peptides also allow revealing potential biological links and functions of each family of peptides and their complexes.
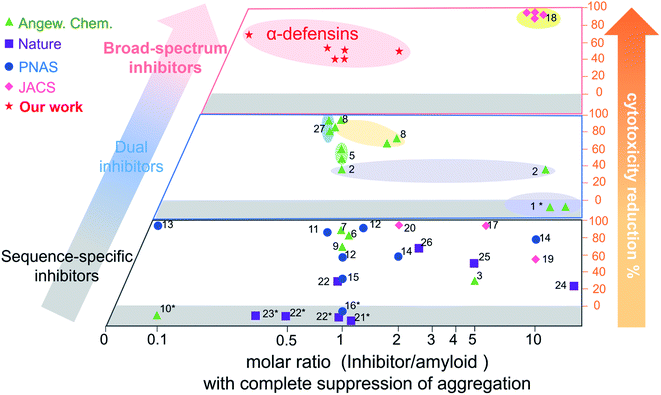 |
| Fig. 5 Comparison of the overall inhibition performances of amyloid inhibitors using the minimal molar ratio of an inhibitor and cell cytotoxicity reduction. Minimal molar ratio of inhibitor : amyloid on the x-axis indicates the minimal concentration of the inhibitor to completely suppress amyloid aggregation, while cell cytotoxicity reduction on the y-axis is quantified by a maximal ratio of amyloid-induced cell toxicity in the presence and absence (control) of the inhibitor. *No cytotoxicity data are available in the references (for references refer to Table S1†). | |
4. Methods
4.1 Preparation of amyloid and antimicrobial peptides
Full-length amyloid peptides including amyloid–β 1–42 (Aβ, purity ≥ 95.0%), human islet amyloid polypeptide 1–37 (hIAPP, purity ≥ 95.0%), and human calcitonin 1–32 (hCT, purity ≥ 95.0%) peptides were obtained from AnaSpec (CA, USA). α-Defensins, human defensin-1 (HNP-1, purity 97.0%) and rabbit corticostatin (NP-3A, purity 96.2%) were obtained from CPC Scientific (CA, USA). All the lyophilized peptide powder was reconstituted in 1,1,1,3,3,3-hexafluoro-2-propanol (HFIP, ≥99.9%) at 1 mg ml−1 concentration, sonicated in an ice bath and subsequently centrifugated at 14
000 rpm and 4 °C for 30 min to obtain a homogeneous, aggregate-free peptide solution. The peptide solution was aliquoted and lyophilized to remove HFIP, and stored under −20 °C. Unless otherwise states, both Aβ and hIAPP were first solubilized in 10 mM NaOH to further dissolve in different buffers. Aβ, hIAPP, and hCT were dissolved in 10 mM phosphate-buffered saline buffer (PBS, pH 7.4) to reach 25 μM concentration. α-Defensins, HNP-1 and NP-3A were solubilized in 10 mM PBS (pH 7.4) directly to prepare peptide solutions at desired concentrations.
4. 2Thioflavin T (ThT) fluorescence assay
The ThT spectra of amyloid aggregation were recorded by using a SpectraMax M3 microplate reader (Molecular Devices, CA, USA) at an excitation wavelength of 450 nm and emission wavelength in the range of 470 nm to 500 nm under kinetic bottom-read mode. Samples were prepared on ice by dissolving amyloid peptides in 10 μM ThT–10 mM Tris buffer solution (pH = 7.4) in the absence or presence of α-defensins. ThT fluorescence kinetic measurements were carried out after transferring the samples to a 96-well plate and incubating at 37 °C. The pitch of the data points: 30 min.
4.3 Negative staining electron microscopy
Negative-stained amyloid samples were imaged by using a JSM-1230 transmission electron microscope (JEOL) operating at 120 kV. For negative staining, the samples were prepared after dropping amyloid solutions onto formvar-coated 400-mesh copper grids, blotted, and immediately negatively stained with UAR-EMS uranyl acetate replacement stain (Electron Microscopy Sciences, PA, USA) for 30 min. The images were recorded with a bottom mount CCD camera with 2048 × 2048 pixels using digital micrograph software.
4.4 Atomic force microscopy (AFM)
The morphological changes of amyloid peptides during aggregation were imaged by using a Nanoscope III multimode AFM (Veeco, NY, USA). At different time intervals, 20 μl amyloid solution was deposited on a mica surface for 2 min and rinsed with water to remove salts. The as-prepared samples were blotted and subsequently imaged by using a silicon AFM probe with a nominal radius of <10 nm and 300 kHz resonant frequency (Aspire, USA), in tapping mode with a scan rate of 1.0 Hz.
4.5 Sodium dodecyl sulfate-polyacrylamide gel electrophoresis (SDS-PAGE)
The amyloid aggregates in the absence or presence of α-defensins were separated and assessed by SDS-PAGE. Freshly isolated amyloid solutions after different aggregation times were immediately subjected to photo-induced crosslinking of unmodified proteins (PICUP) and subsequently analyzed by SDS-PAGE. An equal volume mixture of the sample and 2× Laemmli sample buffer (2.1% SDS, 26.3% w/v glycerol, 0.01% bromophenol blue, and 65.8 mM Tris–HCl buffer pH 6.8) was electrophoresed in 8% Bis-Tris SDS-PAGE gels (GenScript). Electrophoresis was performed under 80 V in MES–SDS buffer (50 mM Tris-base, 50 mM MES, 0.1% SDS, and 1 mM EDTA) and subsequently visualized by silver staining.
4.6 Circular dichroism (CD) spectroscopy
The secondary structural change of amyloid peptides along the aggregation process was measured by far-UV CD spectroscopy using a J-1500 spectropolarimeter (Jasco Inc., Japan). The samples were prepared by dissolving amyloid peptides in 10 mM sodium phosphate buffer (pH = 7.4) and incubated at 37 °C in the presence or absence of α-defensins. After 0, 0.5 d, 1 d, and 3 d incubation times, 150 μl of samples were transferred into a cuvette (1 mm optical path length) and scanned between 190 and 250 nm with a step size of 0.5 nm and 50 nm min−1 scan rate. Far-UV CD spectroscopy was performed and the results were analyzed by using the Beta Structure Selection (BeStSel) algorithm (http://bestsel.elte.hu/) to determine the secondary structure contents of each sample.
4.7 Surface plasmon resonance (SPR) spectroscopy
A custom-built four-channel SPR instrument was used to measure the interaction between amyloid peptides and α-defensins. The interaction was studied by injecting α-defensins, HNP-1/NP-3A, to flow over the surface of amyloid peptides, Aβ/hIAPP/hCT, immobilized SPR sensor chips, and the association and disassociation processes were revealed by the change of resonance signals due to the incident angle shift. For amyloid peptide immobilization, a dextran modified SPR sensor chip was first prepared following a well-established method. In brief, a self-assembled monolayer of thiols was first prepared on a SPR gold chip by immersing in 5 mM 11-mercapto-1-undecanol (ethanol/water 8
:
2 solution) for 24 h, followed by a 3 h reaction in epichlorohydrin (2% v/v in 0.1 M NaOH), and a 24 h reaction in 300 g l−1 dextran (500 kDa) 0.1 M NaOH solution. The resultant dextran modified SPR sensor chip surface was further carboxymethylated in 1.0 M bromoacetic acid in 2 M NaOH for 24 h to achieve the final carboxymethyl dextran SPR sensor chip.
The amyloid peptides (Aβ, hIAPP, or hCT) were dissolved in immobilization buffer (10 mM sodium acetate) and immobilized on sensor chip surface through amine coupling right after activating the sensor chip surface with an equimolar mixture of NHS (N-hydroxysuccinimide) and EDC (N-ethyl-N-(diethylaminopropyl) carbodiimide). The remaining unreacted free amines were further blocked by ethanolamine–HCl solution (pH = 8.5) and the final coupling level of amyloid peptides was kept constant at 6–8 response units (1 RU = 150 pg protein per mm2). Serial two-folded diluted α-defensins in running buffer (10 mM PBS and 0.005 wt% Tween-20, pH 7.4) were injected at a flow rate of 10 μl min−1. Dissociation constant (KD) values were evaluated using Anabel software (http://anabel.skscience.org/) by fitting the data using a 1
:
1 Langmuir binding model and observed binding constant (kobs) linearization method.
4.8 Cell viability assay
To evaluate the amyloid-induced cytotoxicity in vitro, different cell lines were chosen for each amyloid peptide. Human SH-SY5Y neuroblastoma cells (ATCC® CRL-2266™, VA, USA) were used for both Aβ and hCT, while rat insulinoma cells RIN-m5F (ATCC® CRL-11605™, VA, USA) were chosen for hIAPP-induced cytotoxicity. In general, SH-SY5Y cells were cultured in 1
:
1 Eagle's minimum essential medium (EMEM)/Ham's F-12 medium (F12), and RIN-m5F cells were cultured in RPMI-1640 medium. In addition, all culture media were supplemented with 10% fetal bovine serum (FBS) and 1% penicillin/streptomycin. After being incubated in a 5% CO2 humidified incubator at 37 °C and reaching over 80% confluence, the cells were separately seeded onto a 96-well plate (104 cells in 100 μl) and further incubated for 24 h at 37 °C under 5% CO2.
For evaluating cell viability upon treatment with different amyloid peptides, the media were replaced with fresh media containing amyloid peptides (25 μM), α-defensins (5–50 μM), and amyloid peptides in the presence of α-defensins. The cells were further incubated for 24 h or 48 h, followed by replacing the media with 0.5 mg ml−1 3-(4,5-dimethylthiazole-2-yl)-2,5-diphenyltetrazolium bromide (MTT) fresh media. After 4 h of incubation at 37 °C under 5% CO2, the media were replaced with dimethyl sulfoxide to dissolve the formazan crystals formed through MTT reduction in cells. The absorbance value was read at 540 nm, and the cell viability was determined as the percentage of MTT reduction as compared to untreated cells. Data were exhibited as mean ± s. d. of three independent tests.
4.9 Lactate dehydrogenase (LDH) activity assay
The amyloid-induced cytotoxicity was further evaluated by LDH assay. The amount of cytosolic enzyme LDH released to media due to plasma membrane damage was measured as a biomarker to quantify the cytotoxicity. Spontaneous LDH release was measured as a negative control in untreated cells, and a maximal LDH release was measured as a positive control by lysing the cells with Triton X-100. The leaked LDH activity in media was measured using a Pierce™ LDH cytotoxicity assay kit (Thermo, USA), and the absorbance was read at a wavelength of 490 nm by using a microplate reader. All LDH activity values were normalized to spontaneous LDH release (negative control), and cytotoxicity values were calculated as percentages of maximal LDH release (positive control). Data were exhibited as mean ± s. d. of three independent tests.
4.10 Bacterial growth assays
Escherichia coli (ATCC 8739), Pseudomonas aeruginosa (ATCC 27853), Staphylococcus aureus (ATCC 6538P) and Staphylococcus epidermidis (ATCC 14990) were grown to a late-lag phase and diluted to an OD600 value of 0.05. α-Defensins or amyloid peptides at desired concentration were added into experimental groups and an equal volume of PBS was added correspondingly into control groups. The growth curves of bacteria were recorded by measuring OD600 in the following 12 h at 37 °C by using a microplate reader (SpectraMax M3). The final bacterial density after the addition of α-defensins, amyloid peptides and their complexes was compared in relative to that of an un-treated control (100% bacterial density).
The representative images of the live and dead bacteria were acquired to evaluate the antibacterial effects of each α-defensin, amyloid peptide and their complexes. The Gram-negative E. coli bacteria and Gram-positive S. aureus bacteria incubated with or without peptides were stained using a LIVE/DEAD® BacLight™ bacterial viability kit (L7012, Invitrogen) and imaged by using a fluorescence microscope (Olympus IX81) to visualize the live and dead bacteria.
4.11 Minimum inhibitory concentration (MIC) test
The MIC of each individual peptide is determined by the broth microdilution method. All tested strains, including Escherichia coli (E. coli, ATCC 8739), Pseudomonas aeruginosa (P. aeruginosa, ATCC 27853), Staphylococcus aureus (S. aureus, ATCC 6538P) and Staphylococcus epidermidis (S. epidermidis, ATCC 14990), were used at a final suspension concentration of 5 × 105 CFU ml−1. The amount of growth in each well is quantified by OD600 after 20 h of incubation at 37 °C and compared with that in the pure bacteria growth control. The MIC is recorded as the lowest concentration of the peptide that inhibits over 90% of growth.
4.12 Checkerboard testing for synergy analysis
A checkerboard analysis is conducted to determine the antibacterial potency of the combination of amyloid peptides and α-defensins in comparison to their individual activities.97 To quantify the interactions between amyloid peptides and α-defensins, the Fractional Inhibitory Concentration (FIC) index is introduced by the equation:
where A and B are the MIC of each peptide in combination, and MICA and MICB are the MIC of each peptide alone. The combining effect is interpreted as: synergy, FIC index ≤ 0.5; additive or indifference, 0.5 < FIC index < 4.0; antagonism, FIC index ≥ 4.0.98
In detail, in a 96-well plate, the 2-fold serial dilutions of amyloid peptides (at concentrations from 1024 to 4 μg ml−1) were added from columns 2 to 10, while the 2-fold serial dilutions of α-defensins (at concentrations from 256 to 16 μg ml−1) were added from row B to G. Column 12 contains a serial dilution of α-defensins alone, while row H contains a serial dilution of amyloid peptides alone, which are set as controls to determine the MIC value for individual peptides alone (Fig. S12d†). Assay plates are inoculated with 100 μL bacterial suspensions (5 × 105 CFU mL−1), incubated at 37 °C for 20 h. The amount of growth in each well is quantified by OD600 and compared with that in the pure bacteria growth control. The MIC is recorded as the lowest concentration of the peptide that inhibits over 90% of growth.
4.13 Amyloid–HNP-1 models
The initial coordinate of HNP-1 dimer (PDB code: 3GNY) was taken from the X-ray crystal structure,61 while the initial structures of Aβ17–42 (PDB code: 2BEG) and hIAPP pentamers were obtained from NMR crystal structures by the Riek lab99 and the Tycko labs,100 respectively. Aβ17–42 was used in the simulations, because (1) the Aβ fragment of 1–16 has a disordered structure and has not been resolved by solid NMR or X-ray; (2) the N-terminal of Aβ is responsible for the cell membrane anchoring, binding, and penetration, while the C-terminal or hydrophobic regions are responsible for the aggregation. To determine the initial inhibition models of HNP-1 on Aβ and hIAPP (HNP-1–Aβ and HNP-1–hIAPP), a rigid docking method of PatchDock was firstly carried out to roughly determine the binding sites of Aβ and hIAPP pentamers by HNP-1. Then, a refinement docking method of FireDock was conducted for flexible optimization of docking results. Top ten docking models of HNP-1–Aβ or HNP-1–hIAPP with the highest scores evaluated by global energy in FireDock were chosen to screen out the optimal inhibition model. For the ten obtained docking models of HNP-1–Aβ or HNP-1–hIAPP, different binding modes of HNP-1–Aβ or HNP-1–hIAPP were classified based on the three different external surfaces of Aβ (AβC, AβN, and AβU) or hIAPP (hIAPPC, hIAPPN, and hIAPPU) pentamers by HNP-1. The docking models with the same external binding surface of Aβ or hIAPP pentamers by HNP-1 were regarded as the same binding mode for HNP-1–Aβ (HNP-1–AβC, HNP-1–AβN, and HNP-1–AβU) or hIAPP–HNP-1 (HNP-1–hIAPPC, HNP-1–hIAPPN, and HNP-1–hIAPPU). After that, these resulting inhibition models of HNP-1–Aβ or HNP-1–hIAPP were energy minimized in an implicit solvent model. The nonbonded interaction energy between HNP-1 and amyloid peptides was calculated for these minimized models to determine the optimal inhibition models of HNP-1–Aβ or HNP-1–hIAPP. For any given binding mode of HNP-1–Aβ or HNP-1–hIAPP, an optimal model with the lowest interaction energy of HNP-1 with Aβ or hIAPP pentamer was regarded as the optimal inhibition model. The optimal models of HNP-1–Aβ or HNP-1–hIAPP with different binding surfaces of Aβ or hIAPP by HNP-1 were solvated using an explicit TIP3P water model. Counterions of Cl− and Na+ were added into the resulting systems to neutralize the systems and mimic 150 mM ionic strength.
4.14 MD simulation protocol
Prior to equilibrium and production of MD simulations, all resulting systems were initially energy minimized using 5000-step conjugate gradient minimizations to remove bad contacts between atoms and relax the systems. After energy minimizations, 80 ns all-atom MD simulations were subjected to the NAMD 2.13 package101 with CHARMM27 force field under the 3D periodic boundary conditions and the NPT ensemble (constant number of atoms, constant pressure, and constant temperature). The Langevin thermostat method with a damping coefficient of 1 ps−1 was applied to maintain the temperature of the systems at 310 K. The pressure of the systems was continuously kept at 1 atm by the Langevin Piston method with a decay period of 100 fs and a damping time of 50 fs based on the Nose–Hoover algorithm. Long-range electrostatic potentials were estimated by the particle mesh Ewald (PME) method with a griding space of 1 Å, while short-range vdW potentials were calculated using switch function with the twin-range cutoff at 12 Å and 14 Å, respectively. All covalent bonds of molecules in the systems including hydrogen bonds were constrained using the RATTLE method, and hence the velocity Verlet method was conducted to integrate Newton's equations of motion with a larger timestep of 2 fs. MD trajectories of all systems were saved every 2 ps for further analysis. All analyses were conducted in the VMD package102 using in-house codes.
Data availability
All data have been provided in main text and supplemental materials.
Author contributions
Y. Zhang designed this research, performed the characterization, assays and analysis. Y. Liu performed molecular dynamics simulations and analysis. Y. Zhang and Y. Liu contributed equally to this work. Y. Tang and D. Zhang assisted to perform part of the SPR and antibacterial experiments. H. He and J. Wu helped to develop characterization methods. Y. Zhang and J. Zheng wrote the manuscript. J. Zheng concepted the project and supervised this research. All authors contributed to the analysis and discussion of the results and have given approval to the final version of the manuscript.
Conflicts of interest
There are no conflicts to declare.
Acknowledgements
J. Z. acknowledges financial support from a NSF grant (2107619).
References
- P. Watkins and P. Thomas, Diabetes mellitus and the nervous system, J. Neurol., Neurosurg. Psychiatry, 1998, 65, 620–632 Search PubMed.
- B. Halliwell, Oxidants and the central nervous system: some fundamental questions. Is oxidant damage relevant to Parkinson's disease, Alzheimer's disease, traumatic injury or stroke?, Acta Neurol. Scand., 1989, 80, 23–33 Search PubMed.
- C. Singaram, E. Gaumnitz, C. Torbey, W. Ashraf, E. Quigley, A. Sengupta and R. Pfeiffer, Dopaminergic defect of enteric nervous system in Parkinson's disease patients with chronic constipation, Lancet, 1995, 346, 861–864 Search PubMed.
- S. M. Butterfield and H. A. Lashuel, Amyloidogenic protein-membrane interactions: mechanistic insight from model systems, Angew. Chem., Int. Ed., 2010, 49, 5628–5654 Search PubMed.
- M. G. Iadanza, M. P. Jackson, E. W. Hewitt, N. A. Ranson and S. E. Radford, A new era for understanding amyloid structures and disease, Nat. Rev. Mol. Cell Biol., 2018, 19, 755–773 Search PubMed.
- E. Karran and B. De Strooper, The amyloid cascade hypothesis: are we poised for success or failure?, J. Neurochem., 2016, 139, 237–252 Search PubMed.
- G. Henríquez, A. Gomez, E. Guerrero and M. Narayan, Potential Role of Natural Polyphenols against Protein Aggregation Toxicity: In Vitro, In Vivo, and Clinical Studies, ACS Chem. Neurosci., 2020, 11, 2915–2934 Search PubMed.
- A. Aliyan, N. P. Cook and A. A. Martí, Interrogating Amyloid Aggregates using Fluorescent Probes, Chem. Rev., 2019, 119, 11819–11856 Search PubMed.
- M. Zhang, X. Mao, Y. Yu, C.-X. Wang, Y.-L. Yang and C. Wang, Nanomaterials for reducing amyloid cytotoxicity, Adv. Mater., 2013, 25, 3780–3801 Search PubMed.
- C. H. van Dyck, Anti-Amyloid-β Monoclonal Antibodies for Alzheimer's Disease: Pitfalls and Promise, Biol. Psychiatry, 2018, 83, 311–319 Search PubMed.
- J. Liu, B. Yang, J. Ke, W. Li and W.-C. Suen, Antibody-Based Drugs and Approaches Against Amyloid-β Species for Alzheimer's Disease Immunotherapy, Drugs Aging, 2016, 33, 685–697 Search PubMed.
- P. Ghosh and P. De, Modulation of Amyloid Protein Fibrillation by Synthetic Polymers: Recent Advances in the Context of Neurodegenerative Diseases, ACS Appl. Bio Mater., 2020, 3, 6598–6625 Search PubMed.
- A. Mitra and N. Sarkar, Sequence and structure-based peptides as potent amyloid inhibitors: A review, Arch. Biochem. Biophys., 2020, 695, 108614 Search PubMed.
- R. K. Saini, D. Goyal and B. Goyal, Targeting Human Islet Amyloid Polypeptide Aggregation and Toxicity in Type 2 Diabetes: An Overview of Peptide-Based Inhibitors, Chem. Res. Toxicol., 2020, 33, 2719–2738 Search PubMed.
- B. Ren, Y. Zhang, M. Zhang, Y. Liu, D. Zhang, X. Gong, Z. Feng, J. Tang, Y. Chang and J. Zheng, Fundamentals of cross-seeding of amyloid proteins: an introduction, J. Mater. Chem. B, 2019, 7, 7267–7282 Search PubMed.
- M. I. Ivanova, Y. Lin, Y.-H. Lee, J. Zheng and A. Ramamoorthy, Biophysical processes underlying cross-seeding in amyloid aggregation and implications in amyloid pathology, Biophys. Chem., 2021, 269, 106507 Search PubMed.
- T. Fulop, J. M. Witkowski, K. Bourgade, A. Khalil, E. Zerif, A. Larbi, K. Hirokawa, G. Pawelec, C. Bocti and G. Lacombe,
et al., Can an Infection Hypothesis Explain the Beta Amyloid Hypothesis of Alzheimer's Disease?, Front. Aging Neurosci., 2018, 10, 224 Search PubMed.
- F. Panza, M. Lozupone, V. Solfrizzi, M. Watling and B. P. Imbimbo, Time to test antibacterial therapy in Alzheimer's disease, Brain, 2019, 142, 2905–2929 Search PubMed.
- N. Shanmugam, M. O. D. G. Baker, S. R. Ball, M. Steain, C. L. L. Pham and M. Sunde, Microbial functional amyloids serve diverse purposes for structure, adhesion and defence, Biophys. Rev., 2019, 11, 287–302 Search PubMed.
- K. Bourgade, H. Garneau, G. Giroux, A. Y. Le Page, C. Bocti, G. Dupuis, E. H. Frost and T. Fülöp, β-Amyloid peptides display protective activity against the human Alzheimer's disease-associated herpes simplex virus-1, Biogerontology, 2015, 16, 85–98 Search PubMed.
- D. A. Green, E. Masliah, H. V. Vinters, P. Beizai, D. J. Moore and C. L. Achim, Brain deposition of beta-amyloid is a common pathologic feature in HIV positive patients, Aids, 2005, 19, 407–411 Search PubMed.
- C. S. Little, C. J. Hammond, A. MacIntyre, B. J. Balin and D. M. Appelt, Chlamydia pneumoniae induces Alzheimer-like amyloid plaques in brains of BALB/c mice, Neurobiol. Aging, 2004, 25, 419–429 Search PubMed.
- S. S. Dominy, C. Lynch, F. Ermini, M. Benedyk, A. Marczyk, A. Konradi, M. Nguyen, U. Haditsch, D. Raha and C. Griffin, Porphyromonas gingivalis in Alzheimer's disease brains: Evidence for disease causation and treatment with small-molecule inhibitors, Sci. Adv., 2019, 5, eaau3333 Search PubMed.
- R. Alonso, D. Pisa, A. I. Marina, E. Morato, A. Rabano and L. Carrasco, Fungal infection in patients with Alzheimer's disease, J. Alzheim. Dis., 2014, 41, 301–311 Search PubMed.
- M. Tolar, S. Abushakra and M. Sabbagh, The path forward in Alzheimer's disease therapeutics: Reevaluating the amyloid cascade hypothesis, Alzheimer's Dementia, 2020, 16, 1553–1560 Search PubMed.
- K. Bourgade, A. Le Page, C. Bocti, J. M. Witkowski, G. Dupuis, E. H. Frost and T. Fülöp Jr, Protective Effect of Amyloid-β Peptides Against Herpes Simplex Virus-1 Infection in a Neuronal Cell Culture Model, J. Alzheim. Dis., 2016, 50, 1227–1241 Search PubMed.
- W. Chehadeh, N. Abdella, A. Ben-Nakhi, M. Al-Arouj and W. Al-Nakib, Risk factors for the development of diabetes mellitus in chronic hepatitis C virus genotype 4 infection, J. Gastroenterol. Hepatol., 2009, 24, 42–48 Search PubMed.
- H.-K. Huang, J.-H. Wang, W.-Y. Lei, C.-L. Chen, C.-Y. Chang and L.-S. Liou, Helicobacter pylori infection is associated with an increased risk of Parkinson's disease: a population-based retrospective cohort study, Park. Relat. Disord., 2018, 47, 26–31 Search PubMed.
- K. Honjo, R. van Reekum and N. P. Verhoeff, Alzheimer's disease and infection: do infectious agents contribute to progression of Alzheimer's disease?, Alzheimer's Dementia, 2009, 5, 348–360 Search PubMed.
- J. L. Cummings, T. Morstorf and K. Zhong, Alzheimer's disease drug-development pipeline: few candidates, frequent failures, Alzheimer's Res. Ther., 2014, 6, 1–7 Search PubMed.
- D. Mehta, R. Jackson, G. Paul, J. Shi and M. Sabbagh, Why do trials for Alzheimer's disease drugs keep failing? A discontinued drug perspective for 2010-2015, Expert Opin. Invest. Drugs, 2017, 26, 735–739 Search PubMed.
- Group, A. s. D. A.-i. P. T. R., Results of a follow-up study to the randomized Alzheimer's Disease Anti-inflammatory Prevention Trial (ADAPT), Alzheimer's Dementia, 2013, 9, 714–723 Search PubMed.
- Group, A.-F. R., Follow-up evaluation of cognitive function in the randomized Alzheimer's Disease Anti-inflammatory Prevention Trial and its Follow-up Study, Alzheimer's Dementia, 2015, 11, 216–225 Search PubMed.
- T. E. Golde, Host immune defence, amyloid-β peptide and Alzheimer disease, Nat. Rev. Neurol., 2016, 12, 433–434 Search PubMed.
- W. H. DePas and M. R. Chapman, Microbial manipulation of the amyloid fold, Res. Microbiol., 2012, 163, 592–606 Search PubMed.
- R. P. Friedland and M. R. Chapman, The role of microbial amyloid
in neurodegeneration, PLoS Pathog., 2017, 13, e1006654 Search PubMed.
- B. J. Balin and A. P. Hudson, Herpes viruses and Alzheimer's disease: new evidence in the debate, Lancet Neurol., 2018, 17, 839–841 Search PubMed.
- K. Hartman, J. R. Brender, K. Monde, A. Ono, M. L. Evans, N. Popovych, M. R. Chapman and A. Ramamoorthy, Bacterial curli protein promotes the conversion of PAP248-286 into the amyloid SEVI: cross-seeding of dissimilar amyloid sequences, PeerJ, 2013, 1, e5 Search PubMed.
- P. Caruso, R. Burla, S. Piersanti, G. Cherubini, C. Remoli, Y. Martina and I. Saggio, Prion expression is activated by Adenovirus 5 infection and affects the adenoviral cycle in human cells, Virology, 2009, 385, 343–350 Search PubMed.
- S. J. Soscia, J. E. Kirby, K. J. Washicosky, S. M. Tucker, M. Ingelsson, B. Hyman, M. A. Burton, L. E. Goldstein, S. Duong and R. E. Tanzi, The Alzheimer's disease-associated amyloid β-protein is an antimicrobial peptide, PLoS One, 2010, 5, e9505 Search PubMed.
- M. L. Gosztyla, H. M. Brothers and S. R. Robinson, Alzheimer's amyloid-β is an antimicrobial peptide: a review of the evidence, J. Alzheim. Dis., 2018, 62, 1495–1506 Search PubMed.
- N. B. Last and A. D. Miranker, Common mechanism unites membrane poration by amyloid and antimicrobial peptides, Proc. Natl. Acad. Sci. U.S.A., 2013, 110, 6382–6387 Search PubMed.
- Y. Hirakura, I. Carreras, J. D. Sipe and B. L. Kagan, Channel formation by serum amyloid A: a potential mechanism for amyloid pathogenesis and host defense, Amyloid, 2002, 9, 13–23 Search PubMed.
- W. M. Wojtowicz, M. Farzan, J. L. Joyal, K. Carter, G. J. Babcock, D. I. Israel, J. Sodroski and T. Mirzabekov, Stimulation of enveloped virus infection by β-amyloid fibrils, J. Biol. Chem., 2002, 277, 35019–35024 Search PubMed.
- J. Münch, E. Rücker, L. Ständker, K. Adermann, C. Goffinet, M. Schindler, S. Wildum, R. Chinnadurai, D. Rajan and A. Specht, Semen-derived amyloid fibrils drastically enhance HIV infection, Cell, 2007, 131, 1059–1071 Search PubMed.
- S. Makin, The amyloid hypothesis on trial, Nature, 2018, 559, S4 Search PubMed.
- M. R. White, R. Kandel, S. Tripathi, D. Condon, L. Qi, J. Taubenberger and K. L. Hartshorn, Alzheimer's Associated β-Amyloid Protein Inhibits Influenza A Virus and Modulates Viral Interactions with Phagocytes, PLoS One, 2014, 9, e101364 Search PubMed.
- W. A. Eimer, D. K. Vijaya Kumar, N. K. Navalpur Shanmugam, A. S. Rodriguez, T. Mitchell, K. J. Washicosky, B. György, X. O. Breakefield, R. E. Tanzi and R. D. Moir, Alzheimer's Disease-Associated β-Amyloid Is Rapidly Seeded by Herpesviridae to Protect against Brain Infection, Neuron, 2018, 99, 56–63 Search PubMed.
- B. L. Kagan, H. Jang, R. Capone, F. Teran Arce, S. Ramachandran, R. Lal and R. Nussinov, Antimicrobial properties of amyloid peptides, Mol. Pharm., 2012, 9, 708–717 Search PubMed.
- H. Jang, F. T. Arce, M. Mustata, S. Ramachandran, R. Capone, R. Nussinov and R. Lal, Antimicrobial protegrin-1 forms amyloid-like fibrils with rapid kinetics suggesting a functional link, Biophys. J., 2011, 100, 1775–1783 Search PubMed.
- H. Zhao, R. Sood, A. Jutila, S. Bose, G. Fimland, J. Nissen-Meyer and P. K. J. Kinnunen, Interaction of the antimicrobial peptide pheromone
Plantaricin A with model membranes: Implications for a novel mechanism of action, Biochim. Biophys. Acta, Biomembr., 2006, 1758, 1461–1474 Search PubMed.
- A. N. Calabrese, Y. Liu, T. Wang, I. F. Musgrave, T. L. Pukala, R. F. Tabor, L. L. Martin, J. A. Carver and J. H. Bowie, The amyloid fibril-forming properties of the amphibian antimicrobial peptide uperin 3.5, ChemBioChem, 2016, 17, 239–246 Search PubMed.
- R. Urrutia, R. A. Cruciani, J. L. Barker and B. Kachar, Spontaneous polymerization of the antibiotic peptide magainin 2, FEBS Lett., 1989, 247, 17–21 Search PubMed.
- D. W. Juhl, E. Glattard, M. Lointier, P. Bampilis and B. Bechinger, The Reversible Non-covalent Aggregation Into Fibers of PGLa and Magainin 2 Preserves Their Antimicrobial Activity and Synergism, Front. Cell. Infect. Microbiol., 2020, 10, 526459 Search PubMed.
- L. Caillon, J. A. Killian, O. Lequin and L. Khemtémourian, Biophysical investigation of the membrane-disrupting mechanism of the antimicrobial and amyloid-like peptide dermaseptin S9, PLoS One, 2013, 8, e75528 Search PubMed.
- C. W. Cairo, A. Strzelec, R. M. Murphy and L. L. Kiessling, Affinity-based inhibition of β-amyloid toxicity, Biochemistry, 2002, 41, 8620–8629 Search PubMed.
- M. Baram, S. Gilead, E. Gazit and Y. Miller, Mechanistic perspective and functional activity of insulin in amylin aggregation, Chem. Sci., 2018, 9, 4244–4252 Search PubMed.
- Y. Atsmon-Raz and Y. Miller, Non-amyloid-β component of human α-synuclein oligomers induces formation of new Aβ oligomers: insight into the mechanisms that link Parkinson's and Alzheimer's diseases, ACS Chem. Neurosci., 2016, 7, 46–55 Search PubMed.
- Y. Atsmon-Raz, V. Wineman-Fisher, M. Baram and Y. Miller, Unique Inversion Events of Residues around the Backbone in the Turn Domain of β-Arches in Amylin Fibrils, ACS Chem. Neurosci., 2018, 10, 1209–1213 Search PubMed.
- A. Szyk, Z. Wu, K. Tucker, D. Yang, W. Lu and J. Lubkowski, Crystal structures of human α-defensins HNP4, HD5, and HD6, Protein Sci., 2006, 15, 2749–2760 Search PubMed.
- G. Wei, E. de Leeuw, M. Pazgier, W. Yuan, G. Zou, J. Wang, B. Ericksen, W.-Y. Lu, R. I. Lehrer and W. Lu, Through the looking glass, mechanistic insights from enantiomeric human defensins, J. Biol. Chem., 2009, 284, 29180–29192 Search PubMed.
- M. Wolff, B. Zhang-Haagen, C. Decker, B. Barz, M. Schneider, R. Biehl, A. Radulescu, B. Strodel, D. Willbold and L. Nagel-Steger, Aβ42 pentamers/hexamers are the smallest detectable oligomers in solution, Sci. Rep., 2017, 7, 2493 Search PubMed.
- Y. P. Y. Lam, C. A. Wootton, I. Hands-Portman, J. Wei, C. K. C. Chiu, I. Romero-Canelon, F. Lermyte, M. P. Barrow and P. B. O'Connor, Determination of the Aggregate Binding Site of Amyloid Protofibrils Using Electron Capture Dissociation Tandem Mass Spectrometry, J. Am. Soc. Mass Spectrom., 2020, 31, 267–276 Search PubMed.
- Y. Liu, D. Zhang, Y. Zhang, Y. Tang, L. Xu, H. He, J. Wu and J. Zheng, Molecular Dynamics Simulations of Cholesterol Effects on the Interaction of hIAPP with Lipid Bilayer, J. Phys. Chem. B, 2020, 124, 7830–7841 Search PubMed.
- D. Schneidman-Duhovny, Y. Inbar, R. Nussinov and H. J. Wolfson, PatchDock and SymmDock: servers for rigid and symmetric docking, Nucleic Acids Res., 2005, 33, W363–W367 Search PubMed.
- E. Mashiach, D. Schneidman-Duhovny, N. Andrusier, R. Nussinov and H. J. F. D. Wolfson, a web server for fast interaction refinement in molecular docking, Nucleic
Acids Res., 2008, 36, W229–W232 Search PubMed.
- J. R. Brender, S. Salamekh and A. Ramamoorthy, Membrane disruption and early events in the aggregation of the diabetes related peptide IAPP from a molecular perspective, Acc. Chem. Res., 2011, 45, 454–462 Search PubMed.
- S. Han, M. Kollmer, D. Markx, S. Claus, P. Walther and M. Fändrich, Amyloid plaque structure and cell surface interactions of β-amyloid fibrils revealed by electron tomography, Sci. Rep., 2017, 7, 43577 Search PubMed.
- T. Ganz, M. E. Selsted, D. Szklarek, S. Harwig, K. Daher, D. F. Bainton and R. I. Lehrer, Defensins. Natural peptide antibiotics of human neutrophils, J. Clin. Invest., 1985, 76, 1427–1435 Search PubMed.
- K. Hristova, M. E. Selsted and S. H. White, Critical role of lipid composition in membrane permeabilization by rabbit neutrophil defensins, J. Biol. Chem., 1997, 272, 24224–24233 Search PubMed.
- T. Wyss-Coray, Inflammation in Alzheimer disease: driving force, bystander or beneficial response?, Nat. Med., 2006, 12, 1005–1015 Search PubMed.
- M. T. Heneka, M. J. Carson, J. El Khoury, G. E. Landreth, F. Brosseron, D. L. Feinstein, A. H. Jacobs, T. Wyss-Coray, J. Vitorica and R. M. Ransohoff, Neuroinflammation in Alzheimer's disease, Lancet Neurol., 2015, 14, 388–405 Search PubMed.
- H. Tilg and A. R. Moschen, Microbiota and diabetes: an evolving relationship, Gut, 2014, 63, 1513–1521 Search PubMed.
- C. Ohlsson and K. Sjögren, Effects of the gut microbiota on bone mass, Trends Endocrinol. Metab., 2015, 26, 69–74 Search PubMed.
- R. D. Moir, R. Lathe and R. E. Tanzi, The antimicrobial protection hypothesis of Alzheimer's disease, Alzheimer's Dementia, 2018, 14, 1602–1614 Search PubMed.
- L. Wang, Q. Liu, J.-C. Chen, Y.-X. Cui, B. Zhou, Y.-X. Chen, Y.-F. Zhao and Y.-M. Li, Antimicrobial activity of human islet amyloid polypeptides: An insight into amyloid peptides' connection with antimicrobial peptides, Biol. Chem., 2012, 393, 641–646 Search PubMed.
- K. A. Brogden, Antimicrobial peptides: pore formers or metabolic inhibitors in bacteria?, Nat. Rev. Microbiol., 2005, 3, 238–250 Search PubMed.
- S. Liu, L. Zhou, J. Li, A. Suresh, C. Verma, Y. H. Foo, E. P. Yap, D. T. Tan and R. W. Beuerman, Linear analogues of human β-defensin 3: concepts for design of antimicrobial peptides with reduced cytotoxicity to mammalian cells, ChemBioChem, 2008, 9, 964–973 Search PubMed.
- P. Spitzer, M. Condic, M. Herrmann, T. J. Oberstein, M. Scharin-Mehlmann, D. F. Gilbert, O. Friedrich, T. Grömer, J. Kornhuber and R. Lang, Amyloidogenic amyloid-β-peptide variants induce microbial agglutination and exert antimicrobial activity, Sci. Rep., 2016, 6, 32228 Search PubMed.
- E. de Leeuw, C. Li, P. Zeng, C. Li, M. Diepeveen-de Buin, W.-Y. Lu, E. Breukink and W. Lu, Functional interaction of human neutrophil peptide-1 with the cell wall precursor lipid II, FEBS Lett., 2010, 584, 1543–1548 Search PubMed.
- A. Borges, C. Ferreira, M. J. Saavedra and M. Simoes, Antibacterial activity and mode of action of ferulic and gallic acids against pathogenic bacteria, Microb. Drug Resist., 2013, 19, 256–265 Search PubMed.
- P. P. Pillai, B. Kowalczyk, K. Kandere-Grzybowska, M. Borkowska and B. A. Grzybowski, Engineering gram selectivity of mixed-charge gold nanoparticles by tuning the balance of surface charges, Angew. Chem., Int. Ed., 2016, 55, 8610–8614 Search PubMed.
- K. Matsuzaki, A. Nakamura, O. Murase, K.-i. Sugishita, N. Fujii and K. Miyajima, Modulation of magainin 2− lipid bilayer interactions by peptide charge, Biochemistry, 1997, 36, 2104–2111 Search PubMed.
- L. Chen, X. Li, L. Gao and W. Fang, Theoretical insight into the relationship between the structures of antimicrobial peptides and their actions on bacterial membranes, J. Phys. Chem. B, 2015, 119, 850–860 Search PubMed.
- M. Pasupuleti, M. Roupe, V. Rydengård, K. Surewicz, W. K. Surewicz, A. Chalupka, M. Malmsten, O. E. Sörensen and A. Schmidtchen, Antimicrobial activity of human prion protein is mediated by its N-terminal region, PLoS One, 2009, 4, e7358 Search PubMed.
- S. M. Häffner and M. Malmsten, Influence of self-assembly on the performance of antimicrobial peptides, Curr. Opin. Colloid Interface Sci., 2018, 38, 56–79 Search PubMed.
- C. K. Wang, G. J. King, A. C. Conibear, M. C. Ramos, S. Chaousis, S. T. Henriques and D. J. Craik, Mirror images of antimicrobial peptides provide reflections on their functions and amyloidogenic properties, J. Am. Chem. Soc., 2016, 138, 5706–5713 Search PubMed.
- Y. Jin, J. Hammer, M. Pate, Y. Zhang, F. Zhu, E. Zmuda and J. Blazyk, Antimicrobial activities and structures of two linear cationic peptide families with various amphipathic β-sheet and α-helical potentials, Antimicrob. Agents Chemother., 2005, 49, 4957–4964 Search PubMed.
- G. Hopping, J. Kellock, B. Caughey and V. Daggett, Designed Trpzip-3 β-hairpin inhibits amyloid formation in two different amyloid systems, ACS Med. Chem. Lett., 2013, 4, 824–828 Search PubMed.
- K. N. L. Huggins, M. Bisaglia, L. Bubacco, M. Tatarek-Nossol, A. Kapurniotu and N. H. Andersen, Designed Hairpin Peptides Interfere with Amyloidogenesis Pathways: Fibril Formation and Cytotoxicity Inhibition, Interception of the Preamyloid State, Biochemistry, 2011, 50, 8202–8212 Search PubMed.
- J. Kellock, G. Hopping, B. Caughey and V. Daggett, Peptides composed of alternating L-and D-amino acids inhibit amyloidogenesis in three distinct amyloid systems independent of sequence, J. Mol. Biol., 2016, 428, 2317–2328 Search PubMed.
- H. Shaykhalishahi, E. A. Mirecka, A. Gauhar, C. S. Grüning, D. Willbold, T. Härd, M. Stoldt and W. Hoyer, A β-Hairpin-Binding Protein for Three Different Disease-Related Amyloidogenic Proteins, ChemBioChem, 2015, 16, 411–414 Search PubMed.
- R. I. Lehrer, A. K. Lichtenstein and T. Ganz, Defensins: antimicrobial and cytotoxic peptides of mammalian cells, Annu. Rev. Immunol., 1993, 11, 105–128 Search PubMed.
- L. O. Tjernberg, J. Näslund, F. Lindqvist, J. Johansson, A. R. Karlström, J. Thyberg, L. Terenius and C. Nordstedt, Arrest of-amyloid fibril formation by a pentapeptide ligand, J. Biol. Chem., 1996, 271, 8545–8548 Search PubMed.
- L.-M. Yan, M. Tatarek-Nossol, A. Velkova, A. Kazantzis and A. Kapurniotu, Design of a mimic of nonamyloidogenic and bioactive human islet amyloid polypeptide (IAPP) as nanomolar affinity inhibitor of IAPP cytotoxic fibrillogenesis, Proc. Natl. Acad. Sci. U.S.A., 2006, 103, 2046–2051 Search PubMed.
- J. Zheng, C. Liu, M. R. Sawaya, B. Vadla, S. Khan, R. J. Woods, D. Eisenberg, W. J. Goux and J. S. Nowick, Macrocyclic β-sheet peptides that inhibit the aggregation of a tau-protein-derived hexapeptide, J. Am. Chem. Soc., 2011, 133, 3144–3157 Search PubMed.
- M. M. Sopirala, J. E. Mangino, W. A. Gebreyes, B. Biller, T. Bannerman, J.-M. Balada-Llasat and P. Pancholi, Synergy testing by Etest, microdilution checkerboard, and time-kill methods for pan-drug-resistant Acinetobacter baumannii, Antimicrob. Agents Chemother., 2010, 54, 4678–4683 Search PubMed.
- F. C. Odds, Synergy, antagonism, and what the chequerboard puts between them, J. Antimicrob. Chemother., 2003, 52, 1 Search PubMed.
- R. Riek, 3D structure of Alzheimer's amyloid-beta (1-42) fibrils, Proc. Natl. Acad. Sci. U.S.A., 2005, 102, 17342–17347 Search PubMed.
- S. Luca, W.-M. Yau, R. Leapman and R. Tycko, Peptide conformation and supramolecular organization in amylin fibrils: constraints from solid-state NMR, Biochemistry, 2007, 46, 13505–13522 Search PubMed.
- J. C. Phillips, R. Braun, W. Wang, J. Gumbart, E. Tajkhorshid, E. Villa, C. Chipot, R. D. Skeel, L. Kale and K. Schulten, Scalable molecular dynamics with NAMD, J. Comput. Chem., 2005, 26, 1781–1802 Search PubMed.
- W. Humphrey, A. Dalke and K. Schulten, VMD: visual molecular dynamics, J. Mol. Graph., 1996, 14, 33–38 Search PubMed.
Footnotes |
† Electronic supplementary information (ESI) available. See DOI: 10.1039/d1sc01133b |
‡ The authors contributed equally to this work. |
|
This journal is © The Royal Society of Chemistry 2021 |
Click here to see how this site uses Cookies. View our privacy policy here.