DOI:
10.1039/D3LC00889D
(Critical Review)
Lab Chip, 2024,
24, 1542-1556
Understanding organotropism in cancer metastasis using microphysiological systems
Received
17th October 2023
, Accepted 28th December 2023
First published on 9th January 2024
Abstract
Cancer metastasis, the leading cause of cancer-related deaths, remains a complex challenge in medical science. Stephen Paget's “seed and soil theory” introduced the concept of organotropism, suggesting that metastatic success depends on specific organ microenvironments. Understanding organotropism not only offers potential for curbing metastasis but also novel treatment strategies. Microphysiological systems (MPS), especially organ-on-a-chip models, have emerged as transformative tools in this quest. These systems, blending microfluidics, biology, and engineering, grant precise control over cell interactions within organ-specific microenvironments. MPS enable real-time monitoring, morphological analysis, and protein quantification, enhancing our comprehension of cancer dynamics, including tumor migration, vascularization, and pre-metastatic niches. In this review, we explore innovative applications of MPS in investigating cancer metastasis, particularly focusing on organotropism. This interdisciplinary approach converges the field of science, engineering, and medicine, thereby illuminating a path toward groundbreaking discoveries in cancer research.
Introduction
Cancer, an affliction that has haunted humanity for centuries, continues to present a formidable challenge in the field of medicine. As the world's second-leading cause of death,1 it cast a long shadow in 2020, causing an estimated 19.3 million new cases and claiming 10.0 million lives worldwide.2 Among these cases, a staggering 90% of deaths from solid tumors can be attributed to metastasis, where cancer cells break away from the primary tumor and invade distant organs.3–5 The process from local invasion to colonization of new sites is a complex process that continues to baffle researchers.6
Despite significant advancements in our understanding of cancer biology and the development of innovative treatments, cancer remains enigmatic with numerous unanswered questions.7–9 To navigate this challenging landscape, scientists are crafting diverse research models to explore the intricate facets of cancer. One pivotal aspect of this exploration is the reconstruction of the tumor microenvironment – an intricate milieu where cancer cells interact with surrounding tissues, blood vessels, and the immune system.10,11 Further propelling our understanding is the intriguing concept of organotropism, deeply rooted in Stephen Paget's renowned “seed and soil theory”.12 This theory posits that metastasis thrives in environments uniquely conducive to specific cancer types. Deciphering the mechanisms underlying this phenomenon holds promise, not only in curbing the spread of certain cancers but also in crafting effective treatment strategies.
In recent years, microphysiological systems (MPS) have emerged as a transformative paradigm in cancer research, offering promising avenues to address these challenges.13 These systems, often encompassing the concept of “organ-on-a-chip”, are a testament to the synergy between microfluidics, biology, and engineering. They provide platforms where the geometry and surface characteristics are meticulously designed to enable the co-culturing of various cells, thereby facilitating intricate cell–cell interactions and enabling sophisticated disease modeling.14–17 Beyond their structural elegance, MPS equip researchers with an array of analytical tools, including real-time monitoring,18 assessment of morphological changes,19 and quantification of protein expression levels.20 These capabilities empower us to glean deeper insights into the complex dynamics of cancer within a controlled microenvironment.
In this article, we will explore the diverse applications and pioneering methodologies employed to simulate and analyze cancer metastasis within MPS. By reconstructing key mechanisms of the cancer metastasis process through advanced in vitro models, a wealth of previously untapped insights into cancer biology and treatment emerge. These unprecedented discoveries and innovative breakthroughs have the potential to revolutionize our perspectives on cancers that were historically deemed incurable, paving the way for transformative advancements in the field.
Overview of metastasis and organotropism
Metastasis in cancer unfolds through distinct stages marked by intricate molecular events. Beginning with local invasion, cancer cells breach neighboring tissues through a finely tuned interplay of proteolytic enzymes and matrix interactions.21–23 Intravasation signifies entry into the bloodstream, guided by molecular cues and the specific event, epithelial-mesenchymal transition.24–26 Circulation becomes a journey of survival, as cancer cells adeptly navigate the challenges posed by the bloodstream. Molecular adaptations enable cancer cells to evade immune surveillance and dynamically respond to the circulating milieu.27,28 The concept of organotropism becomes particularly relevant in this stage, as certain cancers show a preference for specific organs. The microenvironment of these target organs provides a favorable niche for circulating cancer cells to colonize.29–31 Extravasation marks the phase where cancer cells exit the bloodstream and confront the challenges of adapting to new microenvironments. This process involves interactions with endothelial cells and the establishment of a foothold in the secondary tissue.32–34 The intricacies of organotropism come into play, influencing the preferential colonization of certain organs over others. Colonization, the final stage, involves the establishment of secondary colonies. This phase requires a spectrum of survival mechanisms, including angiogenesis for vascular support.35,36 The concept of the premetastatic niche is critical here, representing a microenvironment preconditioned to support the seeding and survival of circulating cancer cells.31 Organ-specific factors, such as the unique composition of the extracellular matrix (ECM) and the presence of specific growth factors, contribute to the success of colonization. Organotropism emerges as a significant subplot, with microenvironmental intricacies, genetic influencers, and immune system dynamics playing pivotal roles.37,38 This exploration seeks to reconstruct the metastatic process within MPS and decipher the roles of the various protagonists through organotropisms that guide cells to specific destinations (Fig. 1).
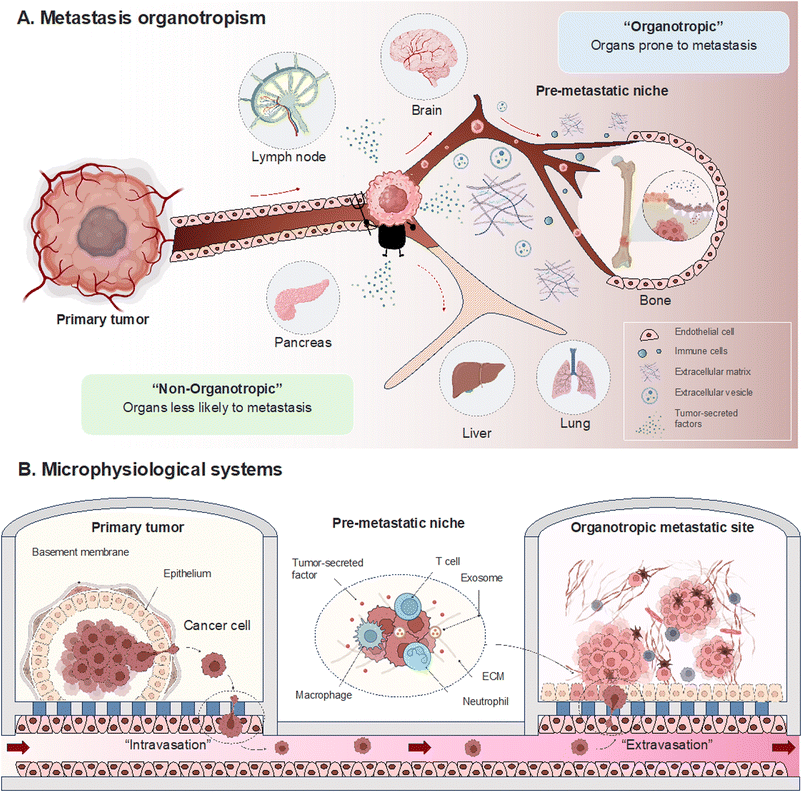 |
| Fig. 1 Metastasis organotropism within microphysiological systems. Establishing a microfluidic pre-metastatic niche within chamber, creating an organotropic environment for the primary tumor, offers insights into diverse factors governing the intricacies of the metastatic process. | |
Cancer progression and invasion
Cancer progression involves the stepwise development and advancement of cancer cells from a localized, primary tumor to more invasive and potentially distant sites. This process is intricate and regulated by a series of molecular events, genetic alterations, and microenvironmental factors. As cancer cells evolve, they acquire characteristics that enable them to break free from the constraints of the primary tumor and embark on a journey to establish secondary colonies.39
Gaining insights into chemotaxis, the orchestration of extracellular chemical gradients in the migration and invasion of cancer cells, is crucial.40 The migration of tumor cells, as well as inflammatory and stromal cells associated with tumors, is directed by chemokines, chemokine receptors, and growth factors. In quest to comprehend these processes, researchers engineered an in vitro cell migration assay to quantitatively evaluate cancer's invasive capacity. These assays allows for the analysis of cancer cell behavior in conditions mimicking the ECM or co-culture with immune cells. Departing from the traditional slide glass-based two-dimensional approach, microfluidic transwells have been developed that allow for three-dimensional (3D) analysis of cancer cell migration.41 A platform with microfluidic wells to accommodate a larger number of samples (>4000) for analysis under a wider range of conditions was also introduced. These innovations introduced a high-throughput 3D cell invasion assay capable of monitoring real-time cell invasion.42
As tumors grow, their accelerated growth frequently outpaces the oxygen supply provided by the existing vasculature, plunging them into regions of hypoxia. In response, a complex network of pro-angiogenic factors, including vascular endothelial growth factor (VEGF) and fibroblast growth factor, is activated. Microfluidic cell culture devices that facilitate the creation of hypoxic environments have been used to investigate the expression of the tumor microenvironment under a variety of conditions.43–45 The 3D assay, consisting of paper layers, proposed a model to separate subpopulations of cells based on their invasiveness in an oxygen concentration gradient.46 Cells in each layer migrate along the oxygen gradient, and this was the first study to show that oxygen acts as a chemoattractant for cancer cells. Cellular spheroids reconstituted from breast cancer cells and lung fibroblasts cultured in a hypoxic environment have been shown to further induce angiogenesis.47 Transcriptional testing of microfluidic pancreatic organoids cultured under hypoxic conditions revealed upregulation of genes associated with cancer-expressed proteins (KRAS, TP53, WNT5a, etc.) to assess metastatic potential.48 This hypoxia-driven angiogenic response not only supports tumor growth but also lays the groundwork for the metastatic cascade. The dynamics of the VEGF-induced tumor metastasis microenvironment were summarized in a multilayered blood vessel/tumor tissue chip.49 VEGF produced by tumor cells significantly reduced extravasation of T cells by inhibiting endothelial cell activation by inflammatory cytokines or inducing endothelial cell anergy, while chemokines produced by tumor cells triggered T cell chemotaxis against tumor cells. Another factor is that mesenchymal stem cells (MSC) have been shown to guide cancer cell invasion in a “cluster-sprout-infiltrating” migration mode.50 Under hypoxic conditions, H19 gene is shown to be responsible for MSC-mediated breast cancer cell migration by antagonizing let-7 and increasing MMP-1 expression. The consequential overexpression of VEGF, orchestrated by hypoxia-inducible factor (HIF) pathways, initiates the formation of new blood vessels, a critical feature that not only sustains tumor growth but also presents an opportunity for the intravasation of cancer cells into the bloodstream. The multiorgan microfluidic platform for hypoxia-induced lung cancer-liver metastasis studies was transcriptomically analyzed on lung cancer cells and showed that the hypoxia-inducible factor 1α (HIF-1α) pathway increases epithelial-mesenchymal transition (EMT) transcription factors (Snail 1, Snail 2), which may promote cancer intravasation.51
Intravasation
During intravasation, the tumor vasculature assumes a pivotal role, acting as the gateway for cancer cells to access the bloodstream.52 Characterized by structural anomalies and heightened permeability, tumor vessels afford cancer cells a swift entry point into the circulatory system.53,54 Once in the bloodstream, cancer cells confront challenges such as immune surveillance, shear forces, and interactions with platelets.55–58 Microfluidic chips have dissected the biomechanical factors influencing circulating tumor cell adhesion, unveiling scenarios where adhesion is more likely in vessels experiencing vascular glycocalyx shedding or hemodynamic disturbances.59 Surviving these challenges shapes subsequent metastatic steps, and microfluidic devices provide a tool to quantitatively measure endothelial barrier development and permeability.60,61 The microfluidic model for measuring endothelial permeability, specifically impaired by macrophage signaling via tumor necrosis factor-alpha secretion, exemplifies the utility of these devices.60
EMT plays a crucial role in facilitating intravasation.62 EMT is a biological process where epithelial cells undergo a series of changes that lead them to acquire a mesenchymal phenotype.63 Epithelial cells are typically found in tissues and have a more static, organized structure. On the other hand, mesenchymal cells are more migratory and invasive. During EMT, cancer cells lose their epithelial characteristics, such as cell–cell adhesion and polarity, and gain mesenchymal traits, which include increased motility and invasiveness.64,65 This phenotypic shift allows cancer cells to detach from the primary tumor site and invade the surrounding tissues, including entering blood or lymphatic vessels during intravasation. In this context, the microfluidic chip also vividly mimicked the EMT process in lung cancer tumors, where downregulation of E-cadherin expression and increased N-cadherin and Vimentin expression could be seen to be activated under flow conditions.66 Another model, encompassing the lymphatic system, tissue, and vasculature, simulated EMT conditions induced by the treatment with interleukin (IL)-6, an inflammatory cytokine, specifically in breast cancer (Fig. 2A).67 Moreover, the microfluidics' capability to replicate intricate microenvironments facilitated the co-culture of pancreatic stellate cells (PSC) and tumor spheroids within the pancreatic milieu. This not only assessed EMT phenotypes but also underscored heightened drug resistance.68 The mesenchymal phenotype acquired through EMT enables cancer cells to navigate through the ECM and breach the basement membrane, prerequisites for entering the bloodstream.69,70 Once in the bloodstream, these cells can be transported to distant sites in the body, initiating the formation of metastatic lesions. Utilizing a device that assesses endothelial barrier permeability, the platform demonstrates soluble biochemical factors such as TNF-α in conjunction with the presence of macrophages to enhance the intravascular penetration of cancer cells, while also influencing the interaction between tumor and endothelial cells.60,71 Other studies have demonstrated the analysis of epithelial or mesenchymal-specific antigens in tumor cells isolated through microfluidic devices.72 This approach effectively examines heterogeneous tumor cell populations, providing insights into tumor progression.
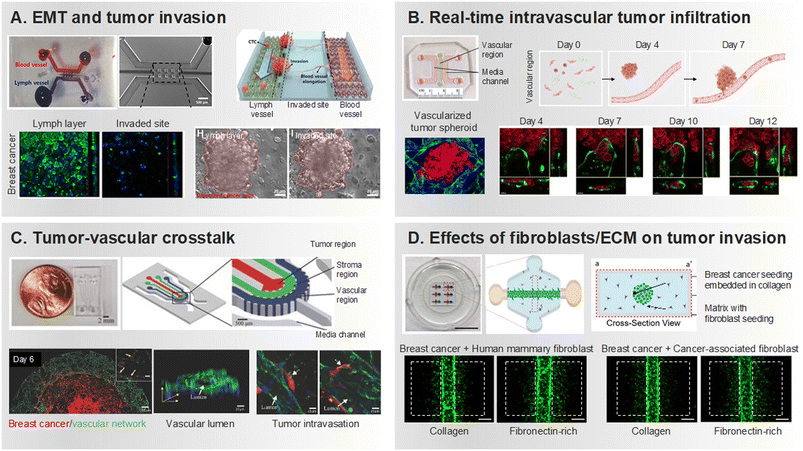 |
| Fig. 2 Development of tumor invasion and intravasation within MPS-based 3D cell co-culture platforms. (A) Reconstructing lymphatic metastasis with a microfluidic chip integrating lymphatic vessel-tissue–blood vessel. Evaluation of the extent of invasion of inflammatory cytokine-treated breast cancer cells into surrounding tissue. Reproduced with permission from Cho et al. (Frontiers in Bioengineering and Biotechnology 8, Copyright 2021 Frontiers Media S.A.). (B) Implemented infiltration of tumor cells through a network of perfusable vessels. Investigating tumor invasion by implementing a vascularized tumor spheroid in an ECM environment containing fibrin, collagen, and Matrigel. Reproduced with permission from Agrawal et al. (Organs-on-a-Chip 4, Copyright 2022 ELSEVIER). (C) A microfluidic chip, comprised of layers housing 3D cell cultures, facilitates the implementation of tumor-vascular crosstalk. Visualization of tumors when endothelial cells are co-cultured, illustrating the signaling cytokines crucial in tumor-vascular crosstalk. Reproduced with permission from Nagaraju et al. (Advanced healthcare materials 7.9, Copyright 2018 Wiley). (D) A platform to quantitatively assess tumor invasion based on the composition of fibroblasts and ECM. Boxes drawn with white dash lines are evidence of tumor invasion, especially tumor invasion in the presence of CAF is significant. Reproduced with permission from Lugo-Cintrón et al. (Cancers 12.5, Copyright 2020 MDPI). | |
Researchers have utilized 3D interfaces of tumor-vascular structures, employing hydrogels to establish endothelial interface, facilitating precise quantification of interactions between tumors and endothelium (Fig. 2B).73–75 Subsequently, their interactions play a crucial role in replicating physiological features and studying processes like intravasation and extravasation of tumor cells. Noteworthy studies employing microfluidic platforms reveal the invasion and intravasation of breast cancer cells, aligning with in vivo findings and shedding light on cytokine-driven mechanisms (Fig. 2C).76 These devices, by impeding HUVEC migration through an empty channel interface, enhance precision in quantifying cancer-induced angiogenesis and intravasation.77 The heterogeneity and hyperpermeability of tumor vessels significantly contribute to cancer cell dissemination, underscoring the importance of studying tumors and their associated vascular networks. These developments embody biochemical and biophysical factors in the body's microenvironment more effectively than classical modalities. These factors are essential for understanding the complex interactions between different cell types.78 The impact of fibroblast, especially cancer-associated fibroblast (CAF), on the transition from tumor migration to intravasation in a steady state environment is also well documented in microfluidic chips (Fig. 2D).79–81 The emphasis on the influence of immune cells in cancer metastasis is growing, with in vitro microfluidic models integrating tumor vasculature and immune cells exhibiting the regulation of endothelial barrier permeability and cancer cell intravasation by factors released from interactions with macrophages.82–84 We summarized how tumor cells and their surrounding components were reconstructed according to primary tumor site in MPS that recapitulated invasion and intravasation (Table 1).
Table 1 MPS-based invasion and intravasation models
Metastasis process |
Primary Tumor Site |
TME Components |
MPS-based Metastatic Characteristics |
Ref. |
TME, tumor microenvironment; TC, tumor cell; CAF, cancer-associated fibroblast; EMT, epithelial-mesenchymal transition; PSC, pancreatic stellate cell; EC, endothelial cell; PEG, Polyethylene glycol. |
Invasion |
Breast |
TC, vascular, collagen |
Cytokines involved in tumor–vascular crosstalk governs cancer cell invasion through collagen matrix |
76
|
Breast |
TC, vascular, fibrin matrix |
Based on an in vitro perfusable vasculature, MPS guides how luminal flow affects intravascular migration and transendothelial flow affects TC transmigrated across the endothelium |
73
|
Breast |
TC, vascular, CAF, fibrin matrix |
To quantitatively evaluate the impact of ECM heterogeneity on TC migration by CAFs, proteins, etc. and to identify the interrelationships between various components in the TME |
79
|
Pancreas |
TC, collagen |
Establishing the epithelial lining of pancreatic cancer cell, defining an invasion score to evaluate local invasion by different cell lines |
85
|
Pancreas |
TC, vascular, collagen |
Observation of pancreatic cancer cell invasion under the oxygen gradients (hypoxia conditions) |
45
|
Pancreas |
TC-spheroid, PSC, collagen |
Analysis of changes in TC growth, EMT phenotype assessment, and drug resistance by coculture with PSC based on TC-spheroid model |
68
|
Intravasation |
Breast |
TC, vascular, macrophage, collagen |
Developing a model of endothelial barrier damage by macrophages to elucidate tumor cell migration dynamics and TC–EC interactions |
60
|
Breast |
TC, vascular |
Developing microfluidic endothelium to assess TC adhesion regulated by CXCR4 on the surface of the endothelium, and showing CXCL12-CXCR4 signaling is relevant to TC intravasation |
74
|
Breast |
TC, vascular, fibroblast, PEG-fibrin matrix |
A microfluidic TME connected by a vascular network between the primary and secondary tumor chambers was established for 28 days under perfusive conditions, mimicking leaky blood vessels and implementing intravasation |
80
|
Colon |
TC-spheroid, vascular, fibrin–collagen–Matrigel matrix |
Observed that hydrogel heterogeneity and complexity increased TC intravasation in a TME composed of TC-spheroids and microvessels (clusters of about 3–17 cells per cluster at day 10) |
75
|
Sarcoma |
TC, vascular, fibroblast, collagen |
The molecular level impact of a shear stress sensor (TPRM7 expression) on intravasation is analyzed by evaluating TC migration through microfluidic channels |
81
|
Pancreas |
TC, vascular, collagen |
Quantified the proportion of TCs that migrated into collagen gel channels and vascular channels with and without oxygen gradient, assessing that approximately 10-fold more TCs migrated under oxygen gradient conditions |
45
|
Extravasation
As cancer cells circulate systemically, the vascular microenvironment of distant organs plays a crucial role in the subsequent chapter of the metastatic process – extravasation.86 Interactions between circulating cancer cells and the endothelial lining of distant organs dictate the success of this intricate process. Adherence to the endothelium and breaching the vascular barrier constitute pivotal steps that usher cancer cells into the target tissue. The organotropism of cancer cells, determined by the specific interactions between adhesion molecules on cancer cells and endothelial cells in target organs, influences the patterns of metastatic colonization.87
In the context of brain cancer metastasis, particularly in the intricate landscape of glioblastoma multiforme (GBM), the ability of cancer cells to breach the blood–brain barrier (BBB) is a decisive factor in establishing footholds within the cerebral terrain.88 The BBB, an intricate fortress comprising endothelial cells, pericytes, and astrocytes, acts as a highly selective barrier regulating substance passage between the bloodstream and the brain. In brain cancer metastasis, the extravasation process involves molecular signals, where cancer cells, armed with specific adhesion molecules, engage in a delicate interplay with endothelial cells (Fig. 3A).89,90 In a microfluidic device-based BBB model, several metastatic cancer cells were found to adhere to the endothelial lining of the barrier and allowed this process to unfold.91,92 An interesting result was that U87 glioma cells failed to cross the BBB, despite their inherent aggressiveness derived from highly invasive brain tumors. This observation was consistent with the clinical insight that gliomas rarely metastasize beyond the cerebrospinal fluid space despite their aggressive nature. Moreover, the microfluidic model simulating the brain-barrier niche, faithfully replicating the interplay between astrocytes and cancer cells constituting the BBB, unveiled the influence of gene expression associated with extravasation.93 The localized degradation of the ECM and basement membrane, facilitated by the proteolytic arsenal of cancer cells, is a key aspect of breaching the BBB. For instance, silencing AKR1B10 in brain metastatic tumor cells suppressed their extravasation through the BBB in the in vitro Transwell model, in the ex vivo microfluidic chip, as well as the in vivo model of brain metastasis in nude mice (Fig. 3B).94 This mechanical feat is coupled with dynamic molecular signaling, as cancer cells release factors influencing the BBB's permeability, creating a hospitable niche for colonization.
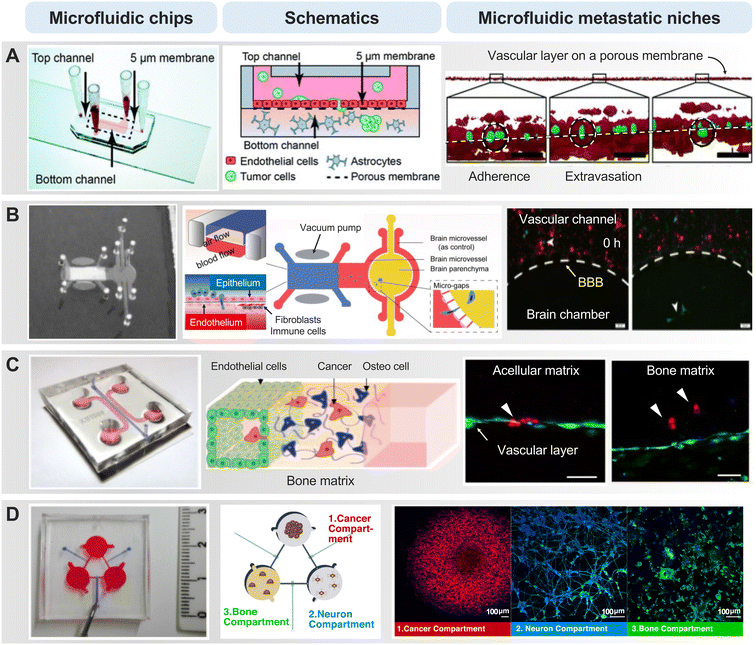 |
| Fig. 3 Representative MPS-based extravasation models. (A) Artificial intelligence analysis detects cells with the potential to metastasize to the brain by profiling the extravasation of cancer cells across a porous membrane coated with a layer of blood vessels. White dotted lines represent the porous layer, green represent tumor cells, and red represent endothelial cells. Reproduced with permission from Oliver et al. (Lab on a Chip 19.7, Copyright 2019 Royal Society of Chemistry). (B) The system, which incorporated chambers for the primary tumor site (lung) and metastasis site (brain), captured tumor cells acrossing through the BBB with real-time analysis. The effect was assessed by downregulating proteins (AKR1B10) that affect lung cancer brain metastasis. Reproduced with permission from Liu et al. (Acta Biomaterialia 91, Copyright 2019 Elsevier). (C) Investigating the impact of cell–cell interactions between cancer cells, ECs and osteo-differentiated (OD) human bone marrow-derived mesenchymal stem cells (hBM-MSCs) on the extravasation ability of cancer cells. Breast cancer cell (MDA-MB-231) extravasated into the extracellular matrix in acellular or bone matrix. Endothelial layer (green), cancer cells (red), cell nuclei (blue). Reproduced with permission from Bersini et al. (Oncotarget 9.90, Copyright 2018 Impact Journals, LLC). (D) Dynamic multicellular paracrine signaling cross-talk between regions by connecting three cell culture chambers including the sympathetic nervous system involved in breast cancer bone metastasis. Reproduced with permission from Conceição et al. (Materials Today Bio 13, Copyright 2022 Elsevier). | |
During breast cancer cell extravasation, they engage with the endothelial cells lining the blood vessels in the bone.95 They may adhere to these endothelial cells and, through various molecular signals, initiate the process of crossing the blood vessel wall. The microfluidic chip, replicating the bone microenvironment, vividly demonstrated the stages of the metastasis cascade from various primary tumor cells with a functional, luminalized vascular layer (Fig. 3C).96–99 The cancer cells release enzymes that facilitate the breakdown of the ECM, enabling them to traverse the vessel wall. Microfluidic Once beyond the confines of the blood vessels, breast cancer cells and colorectal cancer cells encounter the bone matrix, a sophisticated network of proteins and minerals.100,101 In this environment, they interact with bone cells, signaling their presence and priming the “soil” for potential colonization. This intricate interaction involves the release of factors that attract bone-resident cells, thereby creating a microenvironment conducive to the establishment of metastatic lesions. These mechanisms underscore the importance of selective multicellular crosstalk. In the context of microfluidic-based biochemical, microscopic, and proteomic profiling, they reveal a synergistic paracrine signaling dynamic between sympathetic neurons and osteoclasts, contributing to increased breast cancer aggressiveness, as indicated by elevated levels of inflammatory cytokines such as IL-6 and macrophage inflammatory protein 1α (Fig. 3D).102
Colonization
The narrative of metastasis further unfolds during the colonization phase, where the success of disseminated cancer cells hinges on the establishment of a nurturing microenvironment.103 Blood vessels in the target organ emerge as lifelines, furnishing essential nutrients and oxygen that fuel the growth and survival of metastatic niches. Additionally, these blood vessels play a role in establishing a pre-metastatic niche, a supportive microenvironment created by factors released from the primary tumor that prepares distant organs for the arrival of metastatic cells.31,104 The interactions between cancer cells and the vasculature of the target organ are dynamic, with reciprocal signaling influencing the fate of both the metastatic cells and the vascular network.105 For instance, a model proposed with a bone perivascular niche-on-a-chip demonstrated that breast cancer cells exposed to interstitial flow mediate cancer cell colonization with the finding that they remain in a slow proliferative state, which is associated with increased drug resistance.106 This diversity of ECM composition dictates the organotropism of cancer cells, guiding their preference for specific organs based on ECM characteristics.75 Cancer cells discern these ECM signals, deciphering whether the microenvironment is conducive for colonization. To understand this phenomenon, studies are also being conducted to understand the novel ECM protein profiles associated with colonization through the development of different scaffolds.107,108
On the other hand, certain disseminated cancer cells enter the metastatic dormancy for a certain period due to delayed adaptation to their secondary microenvironment.109 Cancer dormancy is a phenomenon in which cancer cells exit the cell cycle and enter a quiescent state,110 temporarily halting their progression.111 Understanding the mechanisms of dormancy is crucial because dormant tumor cells can evade conventional therapeutics, remain quiescent for a while, and then emerge later contributing to the recurrence of the disease.112,113 It is believed that the regulation of dormancy and reactivation involves reciprocal crosstalk between the microenvironment and the transcriptional process. In other words, the microenvironment plays a critical role in the establishment of a dormant state as well as in awakening the cells from a dormant state. Therefore, the use of MPS holds great potential for understanding key factors and processes correlated with tumor dormant-emergent metastatic progression.114,115 The versatility of MPS in replicating complex in vivo conditions facilitates more precise and physiologically relevant studies, offering researchers a sophisticated platform for investigating diverse biological processes related to the intricacies of tumor dormancy and reactivation. Furthermore, this versatility allows for meticulous control over various factors, enabling detailed investigations into the molecular and cellular mechanisms underlying the dormant state of tumor cells. For instance, the development of a 3D MPS with a hydrogel scaffold revealed that the softer matrix property increases the population of spontaneous dormant cells as well as their responsiveness to varying chemotherapeutic doses, indicating their capability to maintain native characteristics in the ex vivo system.108
There are reports that remodeling of the ECM involves the regulation of associated proteins by EVs, creating a fertile environment for colonization.116 The ECM's influence extends to the modulation of immune responses in the metastatic niche.117–119 It can act as a shield, protecting cancer cells from immune surveillance, or conversely, trigger inflammatory responses that foster tumor growth. This bidirectional interplay between tumor, ECM and immune system is evidenced by the immune landscape within the metastatic MPS.120,121
Beyond merely serving as conduits for metastatic cells, blood vessels exhibit a remarkable plasticity – undergoing remodeling in response to the presence of metastatic entities. Normal and stable microvasculature creates a dormant niche, whereas sprouting neovasculature initiates the outgrowth of micrometastases.122 This vascular adaptation contributes to the creation of a microenvironment hospitable to further cancer cell proliferation and survival. The intricate interplay between cancer cells and the vascular network involves processes such as angiocrine signaling, where endothelial cells release factors influencing the behavior of nearby cancer cells.123–126 Understanding the molecular and cellular mechanisms underlying pre-metastatic niches provides valuable insights into the plasticity of the patient-derived TME during different stages of metastasis.127–129 We summarized the MPS study that recapitulated the metastatic cascade by distinguishing between primary tumor sites and metastatic sites (Table 2).
Table 2 MPS-based organotropic extravasation and colonization models
Site on Metastasis |
Primary Tumor Site |
Metastatic cascade |
MPS-based Metastatic Characteristics |
Ref. |
Bone |
Breast |
Extravasation and colonization |
Compared micrometastatic behavior and extravasation in two cancer types using co-culture models: one with metastasis-suppressed breast cancer cells and another with malignant breast cancer cells alongside osteoblastic tissue |
96
|
Breast |
Extravasation and colonization |
Recapitulated neuro-breast cancer crosstalk in a bone metastatic context |
102
|
Breast |
Extravasation |
Conducted cancer cell extravasation through endothelial lumen and ECM |
97
|
Breast |
Colonization |
Cancer cells exposed to interstitial flow within this niche-on-a-chip exhibit slow-proliferative behavior, leading to increased drug resistance |
106
|
Hepatocellular carcinoma |
Extravasation |
Cancer cells (HepG2) were encapsulated in GelMA hydrogel. Hydroxyapatite encapsulated within the GelMA matrix to mimic the bone niche |
98
|
Lung |
Extravasation and colonization |
Osteoclastic RAW264.7 cells induced invadopodia formation via the cortactin pathway with paracrine influence, while A549 cells showed less invasiveness when cultured with MSCs |
99
|
Colorectal cancer |
Extravasation and colonization |
Mimicking mineralized bone tissue with HA/fibrin complexes to investigate the effects on extravasation, tumor-induced microenvironmental angiogenesis, and crosstalk between tumor and tumor microenvironment |
101
|
Brain |
Breast |
Extravasation and colonization |
Replicating the brain choroid plexus in a dynamic microenvironment, this study assessed cancer cell metastasis and immune responses by macrophages |
92
|
Breast |
Extravasation |
Developing a platform to quantitatively profile the dynamic phenotypes of cancer cells, including those from brain metastases, using advanced live cell imaging and artificial intelligence within a microfluidic blood–brain niche chip |
89
|
Breast |
Extravasation |
Astrocytic Dkk-1 is identified as a key factor, stimulating cancer cell migration and influencing gene expression in brain metastatic cancer cells |
93
|
Lung |
Colonization |
Metastatic tumor cells derived from patients were co-cultured with astrocytes and endothelial cells to investigate the impact of the brain tumor microenvironment on acquired drug resistance |
120
|
Lung |
Colonization |
Proteomic analysis revealed a substantially altered spectrum of protein expression in brain metastatic cells compared to primary lung cancer cells |
130
|
Lung |
Extravasation and colonization |
Investigated the role and mechanisms of AKR1B10 in brain metastasis that it promotes the extravasation of cancer cells through the BBB |
94
|
Liver |
Breast |
Colonization |
Elucidating the mechanisms by which breast cancer-derived EVs induce secondary metastasis to the liver |
116
|
Breast |
Colonization |
MPS with hydrogel scaffolds enhances the induction of spontaneous dormancy in breast cancer cells compared to traditional polystyrene scaffolds |
108
|
Lung |
Colonization |
The platform revealed that hypoxia-inducible factor 1α pathway activation led to increased expression of EMT transcription factors, promoting cancer metastasis |
51
|
Adipose tissue (AT) |
Breast |
Extravasation |
Investigating AT vascular dysfunction due to inflammation, recruitment of immune cells, and higher cancer cell metastasis observed in obese individuals |
125
|
Peritoneum |
Ovarian |
Colonization |
Developing vascularized model of the human peritoneum and ovarian cancer and elucidating tumor-stromal cell interactions during intraperitoneal metastasis of ovarian cancer |
126
|
Tumor Vascularization
In recent years, researchers have dedicated substantial efforts to constructing in vitro tumor vascularization through the application of advanced microfluidic technologies. These devices present a distinctive architecture that faithfully replicates the intricate microenvironment of tumors, offering precise control over fluid flow,131 cellular interactions,132 and gradients of chemical cues.133,134 The replication of the vascular network and cellular components of the tumor microenvironment provides an unprecedented opportunity to unravel the dynamic processes of tumor neovascularization and its responses to therapeutic interventions. Recognizing the significance of understanding tumors and their surrounding vasculature is paramount, as these vessels not only serve as conduits for heterogeneous metastasis but also significantly influence the efficacy of anti-cancer treatments.135,136 To mimic tumors and their surrounding vasculature, the widely used transwell system has certain limitations in achieving precise control over chemokines, growth factors, and cell culture conditions.137,138 In contrast, microfluidic devices offer more physiologically relevant and reproducible results, facilitating the investigation of multiple aspects of tumor vascularization. These models enable researchers to delve into the effects of specific angiogenic factors on the formation of new blood vessels,139 the migration and sprouting behavior of endothelial cells,140 and the intricate cross-talk between tumor cells and the vascular network.49 The 3D co-culture capability of microfluidic devices allows the development of in vitro tumor vasculature models that closely mimic physiological characteristics.
These models are categorized into three approaches based on the construction of the tumor microenvironment: endothelial barrier, template-based vasculature, and self-assembled vascular networks. The 3D tumor-vascular interface, developed using hydrogel to provide clear boundaries, facilitates accurate quantification of interactions between tumor cells and endothelium monolayers, particularly concerning tumor invasion and intravasation (Fig. 4A).60 While the endothelial barrier model offers a clear interface for quantitative analysis, its pseudo-3D nature limits it to replicating only the endothelial cell lining within the vasculature. Template-based tumor vasculature models, where a cylindrical channel is created using a microneedle or rod as a template, faithfully replicate the neovascularization process, offering precise control over angiogenic directions and complete embedding of vasculature within a 3D matrix (Fig. 4B).141 Additionally, microfluidic devices designed to facilitate the formation of self-assembled 3D tubular structures through controlled co-culture exhibit promise in understanding aberrant vasculature characteristics, such as poorly developed luminal structures and immaturities, when co-cultured with highly malignant cells (Fig. 4C).142,143 Accordingly, an in-depth investigation into the metabolic heterogeneity presented by tumor and stromal cells in response to therapeutic drugs becomes feasible, providing valuable outcomes.144 These models, at times, produce results that deviate from conventional expectations, thereby serving as critical catalysts for substantial advancements in our understanding and research. For instance, alterations in tumor spheroid volume under anti-cancer treatment do not adhere to the conventional dose-dependent pattern observed in static conditions.47 This atypical result outcome underscores the necessity of accounting for sustained nutrient and oxygen supply via circulatory pathways in the assessment of drug efficacy. These advancements, coupled with the development of vascularized microtumor-on-a-chip and spheroid-based models, underscore the importance of considering continuous nutrient and oxygen supply through blood flow when evaluating drug efficacy under perfusion conditions.47,144 The versatility and precision of microfluidic technology provide an exceptional platform for advancing our understanding of tumor vascularization and its implications for cancer research and therapeutic development.
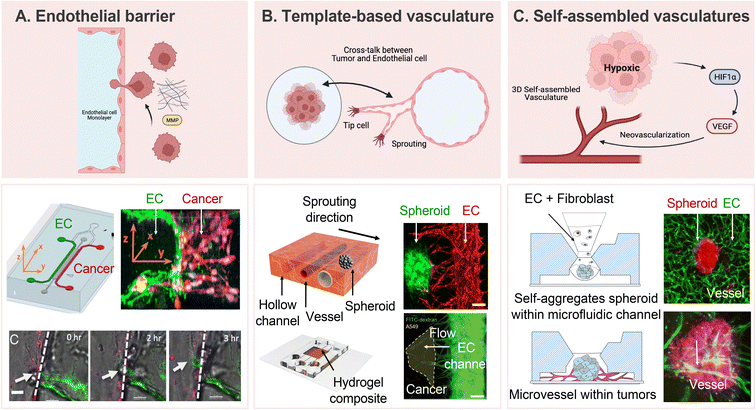 |
| Fig. 4 Representative approaches to develop vascularized tumor models. (A) Formation of endothelial monolayers on a 3D ECM for studying cell–cell interaction during tumor cell invasion and intravasation. Reproduced with permission from Zervantonakisa et al. (Proceedings of the National Academy of Sciences, 109, 34, Copyright 2022 National Academy of Science). (B) Template-based tumor vasculature models provide precise control over angiogenic directions and a comprehensive understanding of neovascularization based on crosstalk between tumor cells and endothelial cells. HUVEC showed angiogenic sprouting toward the cancer spheroid. Reproduced with permission from Kim et al. (Advanced Healthcare Materials, 11, 12, Copyright 2022 John Wiley & Sons, Inc.). (C) Self-assembled 3D tubular structures serve as a model for replicating the natural cellular programs observed during angiogenesis. Fully vascularized tumor spheroid can be utilized for evaluating the performance of anti-cancer treatment. Reproduced with permission from Kim et al. (Biotechnology and Bioengineering, 119, 12, Copyright 2022 John Wiley & Sons, Inc.). | |
In the exploration of the intricate interplay between tumor vascularization and metastasis, researchers venture into uncharted realms in search of therapeutic insights. Promisingly, anti-angiogenic strategies, designed to disrupt the formation of new blood vessels, emerge as contenders to halt metastatic progression. Simultaneously, interventions directed at the crosstalk between cancer cells and the vascular microenvironment present a nuanced approach to impede the relentless spread of cancer. Unraveling these complexities not only deepens our comprehension of metastasis but also reveals potential vulnerabilities that could be strategic targets for the next generation of anti-metastatic therapies. Targeting tumor vascularization has been a focal point in cancer research, with anti-angiogenic therapies aiming to disrupt tumor vascularization indirectly by inhibiting pro-angiogenic factors or directly targeting endothelial cells involved in new blood vessel formation.145 The complex interplay of multiple signaling pathways, involving growth factors, receptors, and intracellular cascades, adds intricacy to tumor vascularization.146,147 By inhibiting neovascularization, the supply of essential nutrients to tumors is restricted, thereby hindering their growth through starvation.148 Notably, anti-VEGF treatment, such as bevacizumab (Avastin®), received FDA approval for colorectal cancer treatment in 2004, marking a milestone in anti-angiogenic therapies.149,150 Various drug candidates, including monoclonal antibodies, receptor tyrosine kinase inhibitor small molecules (RTKIs), and fusion proteins, have since been developed and applied in in vitro model.128,151,152 While substantial progress has been made in identifying key players in tumor angiogenesis, cancer's complexity prompts ongoing research to uncover additional factors and interactions. Controversially, there are concerns that cancer treatments targeting neovascularization for vascular normalization might increase tumor metastasis by attenuating endothelial barrier function.153,154 For instance, sunitinib's anti-VEGF treatment has been associated with increased vascular permeability, promoting tumor cell extravasation.155 A better understanding of tumor and tumor vasculature is imperative for innovative treatment strategies, including advanced targeted therapies and personalized medicine, as we navigate the intricate landscape of cancer metastasis.
Meanwhile, immunotherapy stands out as a breakthrough in cancer treatment. It exploits the patient's immune system to eradicate cancerous cells, holding the potential to enhance outcomes and reduce side effects. Specifically, adoptive cell therapy with tumor-infiltrating lymphocytes (TIL) or genetically modified chimeric antigen receptor (CAR) T or NK cells displays promise in treating hematological malignancies but poses challenges in solid tumors.156 The primary obstacle stems from aberrant vascular networks near solid tumors, which not only act as physical barriers but also induce an immune-hostile microenvironment, thereby increasing tumor resistance to immunotherapy.157 Therefore, understanding the intricacies of tumor-immune cell interactions within the tumor-immune microenvironment (TIME) is crucial for improving the therapeutic efficacy of solid tumor treatment. To achieve this, there is an ongoing effort to develop MPS models that ensure the precise replication of tumor cell-immune cell interactions.158,159 These models encompass various elements, including immune cells, endothelial cells, fibroblasts, and their associated cytokines and matrix.83,160,161 The cutting-edge MPS models hold promise in bridging existing gaps between in vivo and in vitro settings, facilitating the study of tumor–immune cell interactions in a context that closely mimics physiological conditions.162 This approach not only identifies biological barriers to immunotherapy but also offers essential insights into the fundamental mechanisms of cancer biology.
Perspective
In exploring the nuanced concept of the “soil” in cancer metastasis, deeply rooted in the “seed and soil” theory and driven by organotropism, constructing meticulous in vitro microenvironments becomes imperative. These environments aim to faithfully replicate the unique characteristics of target organs favored by cancer cells for metastasis. This ambitious endeavor considers diverse factors and integrates relevant elements to accurately recreate the dynamic interplay between cancer cells and specific organ microenvironments. Critical components include the establishment of a network of perfusable blood vessels as vital conduits, faithful representation of the ECM, and incorporation of functional mediators like EVs. The ECM emerges as a central player, influencing cancer cell behavior, migration, and colonization. Achieving this replication involves utilizing biomimetic materials such as hydrogels, scaffolds, or decellularized matrices. Integrating organ-specific ECM components enhances the in vitro model's ability to recapitulate signaling cues guiding cancer cell interactions. EVs, encompassing exosomes and microvesicles, play a pivotal role in mediating communication, shuttling bioactive molecules capable of influencing cancer cell behavior and promoting metastatic colonization. in vitro models replicating EV signaling can incorporate target organ-derived EVs or engineer synthetic EVs tailored for specific cargo. A comprehensive understanding of EV composition within the target organ microenvironment is essential for accurately reproducing their effects. Researchers should also consider factors like oxygen levels, pH, and the presence of stromal and immune cells, integrating them into the in vitro model for a more physiologically relevant system. This holistic approach facilitates the study of intricate interactions between cancer cells and the organ microenvironment.
A notable strength of 3D cell culture devices based on microfluidic chips is their perfusion capability, allowing real-time monitoring of cells migrating through blood vessels into organotropic environments. Researchers employ dynamic conditions, such as continuous flow or interstitial flow, to investigate physiologically relevant effects on cancer cell behavior. Replicating the dynamic properties of the ECM under flow conditions is crucial, achieved using hydrogels, fibers, or decellularized substrates to simulate ECM remodeling observed during tumor progression. Ideal organotropism within MPS is realized when both the seed and soil areas are modeled, and connected by a perfusable passage in a single system. Integrating patient-derived cells within microfluidic devices ensures a personalized and clinically relevant representation of tumor microenvironmental dynamics. Through the thoughtful integration of these microfluidic strategies, we aim to provide a comprehensive understanding of tumor progression, metastasis, and potential therapeutic interventions guided by organotropism.
Considering these factors, there has been a recent proposal for MPS built on high-throughput, scalable, automated systems. These systems present opportunities to explore organotropism from diverse perspectives, catering to various end-users, including the clinical and pharmaceutical domains. In addition to laying the groundwork for therapeutic strategies to target metastatic cancer, extensive testing can provide insights into driving metastatic cancer cells into less favorable microenvironments. The creation of models reconstructed from patient-derived tumors and tissues seamlessly aligns with precision medicine principles, unlocking substantial potential for clinical applications.
Conclusion
In summary, MPS-based organotrophic modeling strategies rooted in the seed and soil theory have made significant advances in our understanding of disease-related physiological phenomena, such as cancer metastasis. By incorporating cues and interactions specific to each organ, researchers can gain valuable insights into the mechanisms driving organ-specific metastasis and develop innovative approaches to prevent or treat metastatic spread. Understanding the organ specificity of different cancer types enables clinicians to tailor treatment strategies, leading to improved patient outcomes. Moreover, this knowledge of organ-specific metastasis patterns informs surveillance strategies, facilitating early detection of metastases in high-risk organs and allowing for timely intervention. As MPS technology continues to advance, its integration into personalized cancer treatment approaches holds tremendous potential for enhancing treatment outcomes and elevating patient care.
Author contributions
J. Ko and J. Song contributed equally to this study. H. N. Kim conceived this work. All the authors contributed to the writing, discussion, and editing of the manuscript.
Conflicts of interest
None.
Acknowledgements
This study was supported by National Research Foundation of Korea (NRF) grants funded by the Korean government (MSIT) (No. 2021R1A2B5B02086828; 2022R1A6A3A01086914; 2020M3E5D9079744; RS-2023-00253722; RS-2023-00222838). Figures were created using BioRender (https://biorender.com/).
References
- H. Sung, J. Ferlay, R. L. Siegel, M. Laversanne, I. Soerjomataram, A. Jemal and F. Bray, Ca-Cancer J. Clin., 2021, 71, 209–249 CrossRef PubMed
.
- J. Ferlay, M. Colombet, I. Soerjomataram, D. M. Parkin, M. Piñeros, A. Znaor and F. Bray, Int. J. Cancer, 2021, 149, 778–789 CrossRef CAS PubMed
.
- X. Guan, Acta Pharm. Sin. B, 2015, 5, 402–418 CrossRef PubMed
.
- G. P. Gupta and J. Massagué, Cell, 2006, 127, 679–695 CrossRef CAS PubMed
.
- P. S. Steeg, Nat. Med., 2006, 12, 895–904 CrossRef CAS PubMed
.
- C. A. Klein, Nat. Rev. Cancer, 2020, 20, 681–694 CrossRef CAS PubMed
.
- A. I. Riggio, K. E. Varley and A. L. Welm, Br. J. Cancer, 2021, 124, 13–26 CrossRef PubMed
.
- Y. Yang and D. J. Klionsky, Cell Death Differ., 2020, 27, 858–871 CrossRef PubMed
.
- T. N. Schumacher and D. S. Thommen, Science, 2022, 375, eabf9419 CrossRef CAS PubMed
.
- G. Landskron, M. De la Fuente, P. Thuwajit, C. Thuwajit and M. A. Hermoso, J. Immunol. Res., 2014, 2014, 149185 Search PubMed
.
- M. Wang, J. Zhao, L. Zhang, F. Wei, Y. Lian, Y. Wu, Z. Gong, S. Zhang, J. Zhou and K. Cao, J. Cancer, 2017, 8, 761 CrossRef CAS PubMed
.
- I. J. Fidler, Nat. Rev. Cancer, 2003, 3, 453–458 CrossRef CAS PubMed
.
- F. Chen, X. Zhuang, L. Lin, P. Yu, Y. Wang, Y. Shi, G. Hu and Y. Sun, BMC Med., 2015, 13, 1–14 CrossRef CAS PubMed
.
- M. Verhulsel, A. Simon, M. Bernheim-Dennery, V. R. Gannavarapu, L. Gérémie, D. Ferraro, D. Krndija, L. Talini, J.-L. Viovy and D. M. Vignjevic, Lab Chip, 2021, 21, 365–377 RSC
.
- A. Selahi, T. Fernando, S. Chakraborty, M. Muthuchamy, D. C. Zawieja and A. Jain, Lab Chip, 2022, 22, 121–135 RSC
.
- D. Baptista, L. Moreira Teixeira, D. Barata, Z. Tahmasebi Birgani, J. King, S. Van Riet, T. Pasman, A. A. Poot, D. Stamatialis and R. J. Rottier, ACS Biomater. Sci. Eng., 2022, 8, 2684–2699 CrossRef CAS PubMed
.
- D. Huh, D. C. Leslie, B. D. Matthews, J. P. Fraser, S. Jurek, G. A. Hamilton, K. S. Thorneloe, M. A. McAlexander and D. E. Ingber, Sci. Transl. Med., 2012, 4, 159ra147 Search PubMed
.
- J. U. Lind, T. A. Busbee, A. D. Valentine, F. S. Pasqualini, H. Yuan, M. Yadid, S.-J. Park, A. Kotikian, A. P. Nesmith and P. H. Campbell, Nat. Mater., 2017, 16, 303–308 CrossRef CAS PubMed
.
- A. Adamo, A. Sharei, L. Adamo, B. Lee, S. Mao and K. F. Jensen, Anal. Chem., 2012, 84, 6438–6443 CrossRef CAS PubMed
.
- C. Chen, J. Skog, C.-H. Hsu, R. T. Lessard, L. Balaj, T. Wurdinger, B. S. Carter, X. O. Breakefield, M. Toner and D. Irimia, Lab Chip, 2010, 10, 505–511 RSC
.
- T. R. Cox, Nat. Rev. Cancer, 2021, 21, 217–238 CrossRef CAS PubMed
.
- J. Winkler, A. Abisoye-Ogunniyan, K. J. Metcalf and Z. Werb, Nat. Commun., 2020, 11, 5120 CrossRef CAS PubMed
.
- S. U. Nazir, R. Kumar, A. Singh, A. Khan, P. Tanwar, R. Tripathi, R. Mehrotra and S. Hussain, Gene, 2019, 711, 143952 CrossRef CAS PubMed
.
- S. V. Vasaikar, A. P. Deshmukh, P. den Hollander, S. Addanki, N. A. Kuburich, S. Kudaravalli, R. Joseph, J. T. Chang, R. Soundararajan and S. A. Mani, Br. J. Cancer, 2021, 124, 259–269 CrossRef CAS PubMed
.
- M. K. Sznurkowska and N. Aceto, FEBS J., 2022, 289, 4336–4354 CrossRef CAS PubMed
.
- V. Das, S. Bhattacharya, C. Chikkaputtaiah, S. Hazra and M. Pal, J. Cell. Physiol., 2019, 234, 14535–14555 CrossRef CAS PubMed
.
- F. Zanconato, M. Cordenonsi and S. Piccolo, Nat. Rev. Cancer, 2019, 19, 454–464 CrossRef CAS PubMed
.
- P. L. Crispen and S. Kusmartsev, Cancer Immunol., Immunother., 2020, 69, 3–14 CrossRef PubMed
.
- X.-Q. Li, R. Zhang, H. Lu, X.-M. Yue and Y.-F. Huang, Cancer Res., 2022, 82, 1560–1574 CrossRef CAS PubMed
.
- Y. del Pozo Martin, D. Park, A. Ramachandran, L. Ombrato, F. Calvo, P. Chakravarty, B. Spencer-Dene, S. Derzsi, C. S. Hill and E. Sahai, Cell Rep., 2015, 13, 2456–2469 CrossRef CAS PubMed
.
- Y. Liu and X. Cao, Cancer Cell, 2016, 30, 668–681 CrossRef CAS PubMed
.
- H. Kobayashi, K. C. Boelte and P. C. Lin, Curr. Med. Chem., 2007, 14, 377–386 CrossRef CAS PubMed
.
- F. L. Miles, F. L. Pruitt, K. L. Van Golen and C. R. Cooper, Clin. Exp. Metastasis, 2008, 25, 305–324 CrossRef CAS PubMed
.
- F. Peng, M. I. Setyawati, J. K. Tee, X. Ding, J. Wang, M. E. Nga, H. K. Ho and D. T. Leong, Nat. Nanotechnol., 2019, 14, 279–286 CrossRef CAS PubMed
.
- D. Weinstat-Saslow and P. S. Steeg, FASEB J., 1994, 8, 401–407 CrossRef CAS PubMed
.
- P. S. Steeg and D. Theodorescu, Nat. Clin. Pract. Oncol., 2008, 5, 206–219 CrossRef CAS PubMed
.
- X. Nan, J. Wang, H. N. Liu, S. T. Wong and H. Zhao, J. Clin. Med., 2019, 8, 747 CrossRef CAS PubMed
.
- Y. Gao, I. Bado, H. Wang, W. Zhang, J. M. Rosen and X. H.-F. Zhang, Dev. Cell, 2019, 49, 375–391 CrossRef CAS PubMed
.
- N. Sethi and Y. Kang, Nat. Rev. Cancer, 2011, 11, 735–748 CrossRef CAS PubMed
.
- E. T. Roussos, J. S. Condeelis and A. Patsialou, Nat. Rev. Cancer, 2011, 11, 573–587 CrossRef CAS PubMed
.
- C. Zhang, M. P. Barrios, R. M. Alani, M. Cabodi and J. Y. Wong, Exp. Cell Res., 2016, 342, 159–165 CrossRef CAS PubMed
.
- Y. Zhang, L. Zhou and L. Qin, J. Am. Chem. Soc., 2014, 136, 15257–15262 CrossRef CAS PubMed
.
- K. Funamoto, I. K. Zervantonakis, Y. Liu, C. J. Ochs, C. Kim and R. D. Kamm, Lab Chip, 2012, 12, 4855–4863 RSC
.
- J. Lim, H. Choi, J. Ahn and N. L. Jeon, Adv. NanoBiomed Res., 2021, 1, 2100078 CrossRef CAS
.
- M. A. Acosta, X. Jiang, P.-K. Huang, K. B. Cutler, C. S. Grant, G. M. Walker and M. P. Gamcsik, Biomicrofluidics, 2014, 8, 054117 CrossRef PubMed
.
- B. Mosadegh, M. R. Lockett, K. T. Minn, K. A. Simon, K. Gilbert, S. Hillier, D. Newsome, H. Li, A. B. Hall and D. M. Boucher, Biomaterials, 2015, 52, 262–271 CrossRef CAS PubMed
.
- Y. Nashimoto, R. Okada, S. Hanada, Y. Arima, K. Nishiyama, T. Miura and R. Yokokawa, Biomaterials, 2020, 229, 119547 CrossRef CAS PubMed
.
- M. Geyer, D. Schreyer, L.-M. Gaul, S. Pfeffer, C. Pilarsky and K. Queiroz, Cell Death Discovery, 2023, 9, 20 CrossRef CAS PubMed
.
- J. Lee, S.-E. Kim, D. Moon and J. Doh, Lab Chip, 2021, 21, 2142–2152 RSC
.
- F. An, Z. Hou, X. Wang, Z. Wang, C. Jing, R. Sui, Y. Wu, Y. Ma, C. Chang and S. Liu, Biomaterials, 2022, 290, 121848 CrossRef CAS PubMed
.
- L. Zheng, B. Wang, Y. Sun, B. Dai, Y. Fu, Y. Zhang, Y. Wang, Z. Yang, Z. Sun and S. Zhuang, ACS Sens., 2021, 6, 823–832 CrossRef CAS PubMed
.
- S. P. Chiang, R. M. Cabrera and J. E. Segall, Am. J. Physiol., 2016, 311, C1–C14 CrossRef PubMed
.
- H. Hashizume, P. Baluk, S. Morikawa, J. W. McLean, G. Thurston, S. Roberge, R. K. Jain and D. M. McDonald, Am. J. Pathol., 2000, 156, 1363–1380 CrossRef CAS PubMed
.
- D. Kedrin, B. Gligorijevic, J. Wyckoff, V. V. Verkhusha, J. Condeelis, J. E. Segall and J. Van Rheenen, Nat. Methods, 2008, 5, 1019–1021 CrossRef CAS PubMed
.
- G. Bendas and M. Schlesinger, Exp. Hematol. Oncol., 2022, 11, 19 CrossRef CAS PubMed
.
- C. Robert and T. S. Kupper, N. Engl. J. Med., 1999, 341, 1817–1828 CrossRef CAS PubMed
.
- Q. Huang, X. Hu, W. He, Y. Zhao, S. Hao, Q. Wu, S. Li, S. Zhang and M. Shi, Am. J. Cancer Res., 2018, 8, 763 CAS
.
- X.-L. Lou, J. Sun, S.-Q. Gong, X.-F. Yu, R. Gong and H. Deng, Chin. J. Cancer Res., 2015, 27, 450 CAS
.
- Y. Wu, Y. Zhou, R. Paul, X. Qin, K. Islam and Y. Liu, Anal. Chem., 2022, 94, 12159–12166 CrossRef CAS PubMed
.
- I. K. Zervantonakis, S. K. Hughes-Alford, J. L. Charest, J. S. Condeelis, F. B. Gertler and R. D. Kamm, Proc. Natl. Acad. Sci., 2012, 109, 13515–13520 CrossRef PubMed
.
- C. F. Buchanan, S. S. Verbridge, P. P. Vlachos and M. N. Rylander, Cell Adhes. Migr., 2014, 8, 517–524 CrossRef PubMed
.
- J. H. Tsai and J. Yang, Genes Dev., 2013, 27, 2192–2206 CrossRef CAS PubMed
.
- J. Yang, P. Antin, G. Berx, C. Blanpain, T. Brabletz, M. Bronner, K. Campbell, A. Cano, J. Casanova and G. Christofori, Nat. Rev. Mol. Cell Biol., 2020, 21, 341–352 CrossRef CAS PubMed
.
- A. Gandalovičová, T. Vomastek, D. Rosel and J. Brábek, Oncotarget, 2016, 7, 25022 CrossRef PubMed
.
- G. F. Le Bras, K. J. Taubenslag and C. D. Andl, Cell Adhes. Migr., 2012, 6, 365–373 CrossRef PubMed
.
- V. Mani, Z. Lyu, V. Kumar, B. Ercal, H. Chen, S. V. Malhotra and U. Demirci, Adv. Biosyst., 2019, 3, 1800223 CrossRef PubMed
.
- H.-Y. Cho, J.-H. Choi, K.-J. Kim, M. Shin and J.-W. Choi, Front. Bioeng. Biotechnol., 2021, 8, 611802 CrossRef PubMed
.
- J. Plou, Y. Juste-Lanas, V. Olivares, C. Del Amo, C. Borau and J. García-Aznar, Sci. Rep., 2018, 8, 12723 CrossRef CAS PubMed
.
- M. Hakim, L. Kermanshah, H. Abouali, H. M. Hashemi, A. Yari, F. Khorasheh, I. Alemzadeh and M. Vossoughi, Biophys. Rev., 2022, 14, 517–543 CrossRef PubMed
.
- S. Azadi, E. Torkashvand, E. Mohammadi and M. Tafazzoli-Shadpour, Life Sci., 2023, 320, 121545 CrossRef CAS PubMed
.
- A. S. Harney, E. N. Arwert, D. Entenberg, Y. Wang, P. Guo, B.-Z. Qian, M. H. Oktay, J. W. Pollard, J. G. Jones and J. S. Condeelis, Cancer Discovery, 2015, 5, 932–943 CrossRef CAS PubMed
.
- X. Wang, L. Deng and B. T. Gjertsen, Anal. Chim. Acta, 2020, 1129, 1–11 CrossRef CAS PubMed
.
- C. Hajal, L. Ibrahim, J. C. Serrano, G. S. Offeddu and R. D. Kamm, Biomaterials, 2021, 265, 120470 CrossRef CAS PubMed
.
- J. W. Song, S. P. Cavnar, A. C. Walker, K. E. Luker, M. Gupta, Y.-C. Tung, G. D. Luker and S. Takayama, PLoS One, 2009, 4, e5756 CrossRef PubMed
.
- A. Agrawal, S. Shahreza, Y. Javanmardi, N. Szita and E. Moeendarbary, Organs-on-a-Chip, 2022, 4, 100024 CrossRef CAS
.
- S. Nagaraju, D. Truong, G. Mouneimne and M. Nikkhah, Adv. Healthcare Mater., 2018, 7, 1701257 CrossRef PubMed
.
- H. Lee, W. Park, H. Ryu and N. L. Jeon, Biomicrofluidics, 2014, 8, 054102 CrossRef PubMed
.
- T. Pukrop, F. Dehghani, H. N. Chuang, R. Lohaus, K. Bayanga, S. Heermann, T. Regen, D. V. Rossum, F. Klemm and M. Schulz, Glia, 2010, 58, 1477–1489 CrossRef PubMed
.
- K. M. Lugo-Cintrón, M. M. Gong, J. M. Ayuso, L. A. Tomko, D. J. Beebe, M. Virumbrales-Munoz and S. M. Ponik, Cancers, 2020, 12, 1173 CrossRef PubMed
.
- S. Pradhan, A. M. Smith, C. J. Garson, I. Hassani, W. J. Seeto, K. Pant, R. D. Arnold, B. Prabhakarpandian and E. A. Lipke, Sci. Rep., 2018, 8, 3171 CrossRef PubMed
.
- C. L. Yankaskas, K. Bera, K. Stoletov, S. A. Serra, J. Carrillo-Garcia, S. Tuntithavornwat, P. Mistriotis, J. D. Lewis, M. A. Valverde and K. Konstantopoulos, Sci. Adv., 2021, 7, eabh3457 CrossRef CAS PubMed
.
- S. Mi, Z. Liu, Z. Du, X. Yi and W. Sun, Biotechnol. Bioeng., 2019, 116, 1731–1741 CrossRef CAS PubMed
.
- H. Kim, H. Chung, J. Kim, D. H. Choi, Y. Shin, Y. G. Kang, B. M. Kim, S. U. Seo, S. Chung and S. H. Seok, Adv. Sci., 2019, 6, 1900195 CrossRef PubMed
.
- R. G. Mannino, A. N. Santiago-Miranda, P. Pradhan, Y. Qiu, J. C. Mejias, S. S. Neelapu, K. Roy and W. A. Lam, Lab Chip, 2017, 17, 407–414 RSC
.
- M. J. Bradney, S. M. Venis, Y. Yang, S. F. Konieczny and B. Han, Small, 2020, 16, 1905500 CrossRef CAS PubMed
.
- S. Weis, J. Cui, L. Barnes and D. Cheresh, J. Cell Biol., 2004, 167, 223–229 CrossRef CAS PubMed
.
-
U. F. Azubuike and K. Tanner, Trends in Cancer, 2022 Search PubMed
.
- J. F. Deeken and W. Löscher, Clin. Cancer Res., 2007, 13, 1663–1674 CrossRef CAS PubMed
.
- C. R. Oliver, M. A. Altemus, T. M. Westerhof, H. Cheriyan, X. Cheng, M. Dziubinski, Z. Wu, J. Yates, A. Morikawa and J. Heth, Lab Chip, 2019, 19, 1162–1173 RSC
.
- C. D. Arvanitis, G. B. Ferraro and R. K. Jain, Nat. Rev. Cancer, 2020, 20, 26–41 CrossRef CAS PubMed
.
- H. Xu, Z. Li, Y. Yu, S. Sizdahkhani, W. S. Ho, F. Yin, L. Wang, G. Zhu, M. Zhang and L. Jiang, Sci. Rep., 2016, 6, 36670 CrossRef CAS PubMed
.
- J. Lim, S. Rhee, H. Choi, J. Lee, S. Kuttappan, T. T. Y. Nguyen, S. Choi, Y. Kim and N. L. Jeon, Mater. Today Bio, 2023, 22, 100773 CrossRef CAS PubMed
.
- T. M. Westerhof, B. A. Yang, N. M. Merrill, J. A. Yates, M. Altemus, L. Russell, A. J. Miller, L. Bao, Z. Wu and P. J. Ulintz, Adv. NanoBiomed Res., 2023, 3, 2200036 CrossRef CAS PubMed
.
- W. Liu, J. Song, X. Du, Y. Zhou, Y. Li, R. Li, L. Lyu, Y. He, J. Hao and J. Ben, Acta Biomater., 2019, 91, 195–208 CrossRef CAS PubMed
.
- T. A. Guise, W. M. Kozlow, A. Heras-Herzig, S. S. Padalecki, J. J. Yin and J. M. Chirgwin, Clin. Breast Cancer, 2005, 5, S46–S53 CrossRef CAS PubMed
.
- S. Hao, L. Ha, G. Cheng, Y. Wan, Y. Xia, D. M. Sosnoski, A. M. Mastro and S. Y. Zheng, Small, 2018, 14, 1702787 CrossRef PubMed
.
- S. Bersini, A. Miermont, A. Pavesi, R. D. Kamm, J. P. Thiery, M. Moretti and G. Adriani, Oncotarget, 2018, 9, 36110 CrossRef PubMed
.
- F. Sharifi, O. Yesil-Celiktas, A. Kazan, S. Maharjan, S. Saghazadeh, K. Firoozbakhsh, B. Firoozabadi and Y. S. Zhang, Bio-Des. Manuf., 2020, 3, 189–202 CrossRef CAS
.
- X. Ji, H. P. Bei, G. Zhong, H. Shao, X. He, X. Qian, Y. Zhang and X. Zhao, Small, 2023, 2207606 CrossRef CAS PubMed
.
- S. Choi, M. A. Whitman, A. A. Shimpi, N. D. Sempertegui, A. E. Chiou, J. E. Druso, A. Verma, S. C. Lux, Z. Cheng and M. Paszek, Nat. Biomed. Eng., 2023, 1–18 Search PubMed
.
- J. Ahn, J. Lim, N. Jusoh, J. Lee, T.-E. Park, Y. Kim, J. Kim and N. L. Jeon, Front. Bioeng. Biotechnol., 2019, 7, 168 CrossRef PubMed
.
- F. Conceição, D. M. Sousa, J. Loessberg-Zahl, A. R. Vollertsen, E. Neto, K. Søe, J. Paredes, A. Leferink and M. Lamghari, Mater. Today Bio, 2022, 13, 100219 CrossRef PubMed
.
- D. X. Nguyen, P. D. Bos and J. Massagué, Nat. Rev. Cancer, 2009, 9, 274–284 CrossRef CAS PubMed
.
- R. N. Kaplan, S. Rafii and D. Lyden, Cancer Res., 2006, 66, 11089–11093 CrossRef CAS PubMed
.
- M. J. Wolf, A. Hoos, J. Bauer, S. Boettcher, M. Knust, A. Weber, N. Simonavicius, C. Schneider, M. Lang and M. Stürzl, Cancer Cell, 2012, 22, 91–105 CrossRef CAS PubMed
.
- A. Marturano-Kruik, M. M. Nava, K. Yeager, A. Chramiec, L. Hao, S. Robinson, E. Guo, M. T. Raimondi and G. Vunjak-Novakovic, Proc. Natl. Acad. Sci., 2018, 115, 1256–1261 CrossRef CAS PubMed
.
- B. A. Aguado, J. R. Caffe, D. Nanavati, S. S. Rao, G. G. Bushnell, S. M. Azarin and L. D. Shea, Acta Biomater., 2016, 33, 13–24 CrossRef CAS PubMed
.
- A. Clark, S. Wheeler, C. Young, L. Stockdale, J. S. Neiman, W. Zhao, D. Stolz, R. Venkataramanan, D. Lauffenburger and L. Griffith, Lab Chip, 2017, 17, 156–168 RSC
.
- M. S. Sosa, P. Bragado and J. A. Aguirre-Ghiso, Nat. Rev. Cancer, 2014, 14, 611–622 CrossRef CAS PubMed
.
- G. Hadfield, Br. Med. J., 1954, 2, 607 CrossRef CAS PubMed
.
- C. A. Klein, Curr. Opin. Genet. Dev., 2011, 21, 42–49 CrossRef CAS PubMed
.
- G. G. Bushnell, A. P. Deshmukh, P. den Hollander, M. Luo, R. Soundararajan, D. Jia, H. Levine, S. A. Mani and M. S. Wicha, npj Breast Cancer, 2021, 7, 66 CrossRef PubMed
.
- S. Pradhan, J. L. Sperduto, C. J. Farino and J. H. Slater, J. Biol. Eng., 2018, 12, 1–19 CrossRef PubMed
.
- A. M. Clark, M. P. Kumar, S. E. Wheeler, C. L. Young, R. Venkataramanan, D. B. Stolz, L. G. Griffith, D. A. Lauffenburger and A. Wells, Mol. Cell. Proteomics, 2018, 17, 619–630 CrossRef CAS PubMed
.
- A. M. Clark, H. L. Heusey, L. G. Griffith, D. A. Lauffenburger and A. Wells, Front. Oncol., 2021, 11, 676135 CrossRef CAS PubMed
.
- J. Kim, C. Lee, I. Kim, J. Ro, J. Kim, Y. Min, J. Park, V. Sunkara, Y.-S. Park and I. Michael, ACS Nano, 2020, 14, 14971–14988 CrossRef CAS PubMed
.
- M. Kraman, P. J. Bambrough, J. N. Arnold, E. W. Roberts, L. Magiera, J. O. Jones, A. Gopinathan, D. A. Tuveson and D. T. Fearon, Science, 2010, 330, 827–830 CrossRef CAS PubMed
.
- D. Marvel and D. I. Gabrilovich, J. Clin. Invest., 2015, 125, 3356–3364 CrossRef PubMed
.
- M. Binnewies, E. W. Roberts, K. Kersten, V. Chan, D. F. Fearon, M. Merad, L. M. Coussens, D. I. Gabrilovich, S. Ostrand-Rosenberg and C. C. Hedrick, Nat. Med., 2018, 24, 541–550 CrossRef CAS PubMed
.
- H. Kim, J. K. Sa, J. Kim, H. J. Cho, H. J. Oh, D. H. Choi, S. H. Kang, D. E. Jeong, D. H. Nam and H. Lee, Adv. Sci., 2022, 9, 2201785 CrossRef CAS PubMed
.
- J. Lim, S. Rhee, H. Choi, J. Lee, S. Kuttappan, T. T. Y. Nguyen, S. Choi, Y. Kim and N. L. Jeon, Mater. Today Bio, 2023, 100773 CrossRef CAS PubMed
.
- C. M. Ghajar, H. Peinado, H. Mori, I. R. Matei, K. J. Evason, H. Brazier, D. Almeida, A. Koller, K. A. Hajjar and D. Y. Stainier, Nat. Cell Biol., 2013, 15, 807–817 CrossRef CAS PubMed
.
- D. M. Brantley-Sieders, C. M. Dunaway, M. Rao, S. Short, Y. Hwang, Y. Gao, D. Li, A. Jiang, Y. Shyr and J. Y. Wu, Cancer Res., 2011, 71, 976–987 CrossRef CAS PubMed
.
- J. M. Butler, H. Kobayashi and S. Rafii, Nat. Rev. Cancer, 2010, 10, 138–146 CrossRef CAS PubMed
.
- H. Yoon, J. K. Seo and T.-E. Park, Acta Biomater., 2023, 159, 188–200 CrossRef CAS PubMed
.
- L. I. Ibrahim, C. Hajal, G. S. Offeddu, M. R. Gillrie and R. D. Kamm, Biomaterials, 2022, 288, 121728 CrossRef CAS PubMed
.
- D. D. Truong, A. Kratz, J. G. Park, E. S. Barrientos, H. Saini, T. Nguyen, B. Pockaj, G. Mouneimne, J. LaBaer and M. Nikkhah, Cancer Res., 2019, 79, 3139–3151 CrossRef CAS PubMed
.
- S. Y. Lee, S. Byeon, J. Ko, S. Hyung, I. K. Lee, N. L. Jeon, J. Y. Hong, S. T. Kim, S. H. Park and J. Lee, Cancer Med., 2021, 10, 7253–7262 CrossRef CAS PubMed
.
- M. R. Haque, C. R. Wessel, D. D. Leary, C. Wang, A. Bhushan and F. Bishehsari, Microsyst. Nanoeng., 2022, 8, 36 CrossRef PubMed
.
- M. Xu, Y. Wang, W. Duan, S. Xia, S. Wei, W. Liu and Q. Wang, Front. Bioeng. Biotechnol., 2020, 8, 612091 CrossRef PubMed
.
- J. Lii, W.-J. Hsu, H. Parsa, A. Das, R. Rouse and S. K. Sia, Anal. Chem., 2008, 80, 3640–3647 CrossRef CAS PubMed
.
- V. Charwat, M. Rothbauer, S. F. Tedde, O. Hayden, J. J. Bosch, P. Muellner, R. Hainberger and P. Ertl, Anal. Chem., 2013, 85, 11471–11478 CrossRef CAS PubMed
.
- S. Garcia, R. Sunyer, A. Olivares, J. Noailly, J. Atencia and X. Trepat, Lab Chip, 2015, 15, 2606–2614 RSC
.
- K. Kwapiszewska, A. Michalczuk, M. Rybka, R. Kwapiszewski and Z. Brzózka, Lab Chip, 2014, 14, 2096–2104 RSC
.
- M. Eatock, A. Schätzlein and S. Kaye, Cancer Treat. Rev., 2000, 26, 191–204 CrossRef CAS PubMed
.
- E. Wagenblast, M. Soto, S. Gutiérrez-Ángel, C. A. Hartl, A. L. Gable, A. R. Maceli, N. Erard, A. M. Williams, S. Y. Kim and S. Dickopf, Nature, 2015, 520, 358–362 CrossRef CAS PubMed
.
- R. S. Taichman, C. Cooper, E. T. Keller, K. J. Pienta, N. S. Taichman and L. K. McCauley, Cancer Res., 2002, 62, 1832–1837 CAS
.
- T.-H. Lee, H. K. Avraham, S. Jiang and S. Avraham, J. Biol. Chem., 2003, 278, 5277–5284 CrossRef CAS PubMed
.
- J. Ko, Y. Lee, S. Lee, S. R. Lee and N. L. Jeon, Adv. Healthcare Mater., 2019, 8, 1900328 CrossRef PubMed
.
- S. Kim, H. Lee, M. Chung and N. L. Jeon, Lab Chip, 2013, 13, 1489–1500 RSC
.
- D. Kim, K. S. Hwang, E. U. Seo, S. Seo, B. C. Lee, N. Choi, J. Choi and H. N. Kim, Adv. Healthcare Mater., 2022, 11, 2102581 CrossRef CAS PubMed
.
- K. Haase, G. S. Offeddu, M. R. Gillrie and R. D. Kamm, Adv. Funct. Mater., 2020, 30, 2002444 CrossRef CAS PubMed
.
- Y. Kim, J. Ko, N. Shin, S. Park, S. R. Lee, S. Kim, J. Song, S. Lee, K. S. Kang and J. Lee, Biotechnol. Bioeng., 2022, 119, 3678–3693 CrossRef CAS PubMed
.
- A. Sobrino, D. T. Phan, R. Datta, X. Wang, S. J. Hachey, M. Romero-López, E. Gratton, A. P. Lee, S. C. George and C. C. Hughes, Sci. Rep., 2016, 6, 31589 CrossRef CAS PubMed
.
- G. Bergers and D. Hanahan, Nat. Rev. Cancer, 2008, 8, 592–603 CrossRef CAS PubMed
.
- S. M. Weis and D. A. Cheresh, Nat. Med., 2011, 17, 1359–1370 CrossRef CAS PubMed
.
- R. S. Kerbel, N. Engl. J. Med., 2008, 358, 2039–2049 CrossRef CAS PubMed
.
- N. Weidner, J. Folkman, F. Pozza, P. Bevilacqua, E. N. Allred, D. H. Moore, S. Meli and G. Gasparini, J. Natl. Cancer Inst., 1992, 84, 1875–1887 CrossRef CAS PubMed
.
- E. M. Conn, K. A. Botkjaer, T. A. Kupriyanova, P. A. Andreasen, E. I. Deryugina and J. P. Quigley, Am. J. Pathol., 2009, 175, 1638–1652 CrossRef CAS PubMed
.
- M. Muhsin, J. Graham and P. Kirkpatrick, Nat. Rev. Drug Discovery, 2004, 3, 995–997 CrossRef CAS PubMed
.
- O. Mocellin, A. Robinson, A. Olczyk, S. Treillard, T. Olivier, C. Ng, J. Heijmans, D. Goubert, A. Stok and G. van Tienderen, Cancer Res., 2023, 83, 5876 CrossRef
.
- S. Faivre, G. Demetri, W. Sargent and E. Raymond, Nat. Rev. Drug Discovery, 2007, 6, 734–745 CrossRef CAS PubMed
.
- X. Li, Y. Li, W. Lu, M. Chen, W. Ye and D. Zhang, Cell, 2019, 8, 1602 CrossRef CAS PubMed
.
- G. Mattheolabakis and C. M. Mikelis, Front. Oncol., 2019, 9, 1227 CrossRef PubMed
.
- A. S. Chung, M. Kowanetz, X. Wu, G. Zhuang, H. Ngu, D. Finkle, L. Komuves, F. Peale and N. Ferrara, J. Pathol., 2012, 227, 404–416 CrossRef CAS PubMed
.
- M. W. Rohaan, S. Wilgenhof and J. B. Haanen, Virchows Arch., 2019, 474, 449–461 CrossRef PubMed
.
- Z. Lamplugh and Y. Fan, Front. Immunol., 2021, 12, 811485 CrossRef CAS PubMed
.
- M. Campisi, S. E. Shelton, M. Chen, R. D. Kamm, D. A. Barbie and E. H. Knelson, Cancers, 2022, 14, 3561 CrossRef CAS PubMed
.
- N. Moore, D. Doty, M. Zielstorff, I. Kariv, L. Moy, A. Gimbel, J. Chevillet, N. Lowry, J. Santos and V. Mott, Lab Chip, 2018, 18, 1844–1858 RSC
.
- J. M. Ayuso, R. Truttschel, M. M. Gong, M. Humayun, M. Virumbrales-Munoz, R. Vitek, M. Felder, S. D. Gillies, P. Sondel and K. B. Wisinski, Oncoimmunology, 2019, 8, 1553477 CrossRef PubMed
.
- J. Song, H. Choi, S. K. Koh, D. Park, J. Yu, H. Kang, Y. Kim, D. Cho and N. L. Jeon, Front. Immunol., 2021, 12, 733317 CrossRef CAS PubMed
.
- O. T. Nguyen, P. M. Misun, C. Lohasz, J. Lee, W. Wang, T. Schroeder and A. Hierlemann, Front. Immunol., 2021, 12, 781337 CrossRef CAS PubMed
.
Footnote |
† These authors contributed equally to this study. |
|
This journal is © The Royal Society of Chemistry 2024 |
Click here to see how this site uses Cookies. View our privacy policy here.