DOI:
10.1039/C9BM00349E
(Review Article)
Biomater. Sci., 2019,
7, 4472-4481
Analyzing the scaffold immune microenvironment using flow cytometry: practices, methods and considerations for immune analysis of biomaterials
Received
4th March 2019
, Accepted 24th June 2019
First published on 19th August 2019
Abstract
The immune system has evolved as a powerful tool for our body to combat infections, and is being engineered for new treatments in cancer and autoimmune disease. More recently, the complex role of the immune system is being recognized in tissue repair, regenerative medicine and biomaterial responses. From these combined interests, the field of immunoengineering is rapidly growing. However, bridging immunology with engineering poses numerous challenges including the biological complexity, language of immunology and accurately leveraging the powerful techniques of immunology to new applications. Elucidating the identity and function of immune cell populations responding to engineering systems will be required for continued advancement. Multi-color flow cytometry is a central technique used by immunologists for this purpose that requires careful control of variables, data acquisition, and interpretation. Here, we present methods for multi-color flow cytometry experimental design and analysis focused on characterizing the scaffold immune microenvironment in regenerative medicine research.
1. Introduction
Any material that is implanted within the body will elicit an immune response through a process described as the foreign body response. First described in the 1980s by James Anderson, this entails protein adsorption to the surface of the implanted device, followed by neutrophil and macrophage recruitment, an attempt to degrade the material, then fusion of macrophages into foreign body giant cells, recruitment of fibroblasts and deposition of a dense fibrotic capsule around the device.1–3 This occurs at a different extent on different materials, owing to their surface chemistry, stiffness, degradability, and other yet to be elucidated factors.4 With certain applications, such as pacemakers, drug delivery devices, or the needles of insulin pumps, the desired reaction to an implant is either ignorance or tolerance. However, with other materials, such as those used in regenerative medicine, an immune response is desired to help integrate the scaffold with the surrounding tissue and grow new tissue.5 In development of materials for either purpose, it is necessary to analyze the immune environment created by the implanted material. To this extent a variety of techniques have been employed ranging from microscopy to gene expression. Over the past several years, engineers have been adopting specific techniques used and expanded by translational immunologists, including flow cytometry.
Flow cytometry is a critical tool in the study of the immune system.6 This tool can create a description of the scaffold immune microenvironment of multiple tissues on a quantitative level at a single-cell resolution. Beyond analysis, this technique can be used to isolate specific cell types for sequencing or gene expression (RT-PCR/probe-based) on a population or single-cell level. Flow cytometry has been used widely in the immunology community since the late 1980s and early 1990s and has been advancing to expand the complexity and reliability of analysis.7 For many years, stem cells have been the focus of biomaterial analysis in regenerative medicine. In depth lineage tracing of stem cells is not general practice in the biomaterials fields but is required for accurate identification of specific immune cells.
With the rising appreciation of the immune system in tissue engineering and regenerative medicine, biomaterials researchers are beginning to implement flow cytometry in the analysis of biomaterials response. There is a significant need for implementation of consistent methodologies to avoid inaccuracies in experimental techniques and analysis. Application to biomaterial scaffolds also introduces specific concerns for analyzing tissue engineered constructs and materials that are not addressed with standard immunologic practices that have been primarily focused on infectious disease and cancer. An approach involving spatial characterization with immunohistochemistry or immunofluorescence, and flow cytometry providing a quantitative description of the immune microenvironment provides a more complete description of the interactions of the scaffold with the surrounding tissue and immune cells. Here, we present an in-depth approach to analyzing biomaterial scaffolds with flow cytometry, focusing on murine models but applicable to clinical samples.
2. Methods & discussion
2.1. Cell isolation methods
A general rule is to use the gentlest cell isolation method that will still result in a clean single-cell suspension for subsequent staining and analysis. These isolation methods generally fall into both enzyme-based and chelation-based cell isolation. In most cases, a matrix-degrading enzyme will be required to free the cells from the extracellular matrix (ECM).8 If isolating cells from the blood or a lavage (such as a peritoneal lavage), utilization of gentle chelation with filtration will suffice – but if the tissue is dense, with strong fibrosis or collagen deposition, or an ECM-based or collagen-based scaffold, then the sample requires a collagenase to degrade the surrounding tissue. If looking for abundant low-adherence cells (ex. lymphocytes in central lymphoid organs like the spleen or lymph nodes) then mechanical disruption through a sieve/strainer and re-straining after centrifugation will decrease preparation time and provide enough cells for analysis. It is critical to realize that different digestion protocols may enrich for certain cell population or specific cell populations may be lost, biasing the resulting data.
2.1.1. Enzyme-based.
Enzyme-based methods for cell isolation are used very frequently for flow cytometry preparation.8–10 Most protease methods utilize combinations of different collagenases and DNase. There are several products that are commercially available including dispase,11 liberase,12–16 elastase,17,18 and purified collagenases.8,19–21 The targets of these enzymes and reaction conditions are described in Table 1. DNase is utilized to prevent the aggregation of DNA from dead cells in the sample which can cause clumping and decrease cell yields. Most of these enzymes are resuspended in serum-free media (such as RPMI or DMEM) or HBSS, added to diced tissues, then incubated on a shaker at 37 °C for 1 hour for soft tissues such as spleen, skin and muscle, as well as capsules around subcutaneous implants made of synthetic materials or extracellular matrix proteins. Resulting non-digested pieces can be manually pressed through a strainer or added to fresh enzyme for further digestion. Higher concentrations of enzymes, stronger proteases, and longer incubation times can lead to decreased signals of surface proteins and skew cell populations. Some proteins are more susceptible to this degradation than others and multiple digestion methods should be tested to balance cell yield and efficiency of digestion with the side-effects of surface degradation of proteins.22 Care must be taken with fatty or lipid-dense tissues, as lipids may interfere with antibody staining, however a detailed peer-reviewed analysis of this effect has yet to be completed.
Table 1 Enzymes and concentrations used for digestion of tissues to isolate single cell suspensions for flow cytometry studies
Enzymea |
Working concentration |
Tissues |
Trypsin should not be used for FACS digestion as it will degrade many surface proteins.
|
Dispase |
1–2 U ml−1 |
Various (mainly soft) |
Collagenase type I |
100–200 U ml−1 |
Various |
Liberase TL |
0.25–0.5 mg ml−1 |
Soft tissues, low density collagen (ex. muscle, spleen) |
Liberase TM |
0.1–0.5 mg ml−1 |
High density collagen (ex. skin) |
DNase I |
0.1 mg ml−1 |
All |
In combination with these methods, there are other mechanical separation approaches that can assist in isolation of cells, including commercially available processors such as the gentleMACS Dissociator, which can be programmed to dice and, in some models, heat samples for simultaneous mechanical and enzymatic separation.23,24
2.1.2. Chelators.
Certain samples do not require enzyme digestion, such as those with minimal fibrosis and matrix deposition, and can be processed using a gentler chelation-based disassociation. Chelators can also be used for dissolution of ion-crosslinked (i.e. alginate) scaffolds.25 EDTA dissolved in PBS at a neutral pH can be used by manually dicing samples followed by incubation in the chelator at 4 °C. This will dissociate many cation-based cell adhesions as well as scaffold crosslinks. As with enzyme digestion, particles or pieces of scaffolds that are left after a first round of dissociation can be mechanically separated, or sieved out from the single-cell suspension and then re-treated with fresh chelating agent to further dissociate.
2.2. Fluorophore selection & panel design
When designing a new antibody panel, especially for flow cytometry beginners, it is important to minimize the number of fluorophores per panel. This decreases error due to compensation issues, and provides and a robust signal. The general rule of thumb is that if it is possible to split the sample into two panels and not lose any information (if you have enough cells and are not relying on co-expression of many markers), then split the sample into two panels. One example is detecting different general cell populations. It would be easiest to create a myeloid and lymphoid panel for general screening as opposed to trying to fit all of the markers into one larger (10+ color) panel. Scaffolds can elicit a broad range of immune responses, and design of larger more intricate panels will rely on proper pilot testing with lower color panels.
2.2.1. Selection of proper fluorophore for antigen.
When first designing a panel, one important factor to consider is the brightness of the fluorophore compared to the abundance of protein/antigen on the cell of interest. For example, certain proteins such as MHCII, CD45, CD3, Ly6G, and CD19, are very highly abundant on the surface of cells. Therefore, when stained, the cells will have a denser number of antibodies bound to the cell. Due to this increased density of proteins on the cell, these proteins can be paired with a lower-brightness fluorophore such as AlexaFluor700, PerCP, or Pacific Blue. However, for those proteins that are of lower abundance on or inside the cell of interest (ex. FoxP3), they will need to be paired with brighter fluorophores (ex. PE or APC). Unfortunately, higher color panels cannot solely be designed based on the brightness of fluorophores and the abundance of antigen. As fluorophores do not emit at one wavelength and thus are subject to fluorescence overlap and bleed-over into other channels, it is important to consider compensation when designing multi-color experiments. Two fluorophores with a large amount of theorized overlap (ex. Pacific Blue and Brilliant Violet 510, or APC and AlexaFluor700) should be labeled on cells that will not co-express both of these markers. If staining murine lymphoid cells, CD19 (B cells) could be labeled with BV510 and CD8 (cytotoxic T lymphocytes) with Pacific Blue. All panels should also include a viability dye as dead and dying cells which cannot always be gated out using their scatter profile can have variability in autofluorescence and marker expression due to their status as dead/apoptotic and thus provide unreliable data. This is critical for analysis of implanted materials as foreign body responses to the material and cell isolation methods (ex. enzyme digestion) can decrease viability.
2.2.2. Accepting unknowns: co-expression of markers.
As many principles of immunology have been derived from infectious disease and cancer immunology studies, there are still many unknowns in the context of biomaterials and tissue engineering. To this extent, we are actively discovering new subsets of cells that co-express markers that are canonically thought of as proteins on different cell types, or those that were originally thought of as a dichotomy, but have overlap (expressed on the same cell) when viewed carefully.26 A strong example of this is the expression of CD86 and CD206 in mice. These markers were used to identify “M1” and “M2” macrophages.27 However, we now appreciate a large spectrum of macrophage phenotype, wherein cells do not fit into binary categories, and within samples such as scaffold-treated muscle wounds, we observe large numbers of CD86+CD206+ macrophages.28–31 If following historical definitions when designing a panel, these two markers could be placed on fluorophores with fluorescence overlap; now we know that these proteins are co-expressed and thus should be placed in channels that are less subject to compensation issues. This same phenomenon occurs with CD11c (dendritic cell marker) and CD11b (macrophage/myeloid marker) that in some cases are mutually exclusive, but in the context of an implanted material, scaffold associated macrophages express both CD11c and CD11b.29,30,32,33 When these proteins are expressed on the same cell, if the panel is designed thinking they will not be, then it may be difficult to determine real signal from a compensation issue if the fluorophores chosen to label these cells have close emission spectra (see FMO controls for more information on controlling for fluorescence overlap/bleed over).
Selection of the proper number of markers to describe a cell type will depend on the experience with cytometry, as well as the amount of specificity and detail that is desired. For example, using only CD11b to define macrophages will result in quantification of in general myeloid cells, which can be mostly macrophages, however will also include neutrophils, eosinophils, and other cell types. Using CD11b and F4/80 will further key down to macrophages, but, eosinophils can express low levels of F4/80, so macrophages must be “F4/80hi”. Frequently, staining blood and tissue samples, researchers will use Gr1 which includes both Ly6G and Ly6C. In that case, evaluation of monocytes (Ly6ChiLy6G−) and neutrophils (Ly6C+Ly6G+) is determined by Gr1hiversus Gr1+.34 The more markers that can be included in a panel, the more confident you can be that the cells of interest are the cells you are detecting.
2.2.3. Online resources.
Several tools have been developed in order to properly design a panel with fluorophore brightness and overlap in consideration. The most useful are online fluorescence spectra viewers. These are available through multiple companies that manufacture flow cytometry supplies including BD Biosciences, BioLegend, and ThermoFisher Scientific.35–37 These tools allow a user to select the excitation lasers in their flow cytometer, along with the filters for each channel, to properly design a panel. It is important to note that not all proteins have antibodies that are tagged with every fluorophore, so technical considerations of antibody availability need to be considered during panel design.
We have included examples of flow cytometry panels that have been in use and validated in skin, muscle, and intraperitoneal implant models which can be found in Table 2. These panels should all be validated with antibody titers, fluorescence minus one (FMO) controls, and isotype controls on the specific samples and flow cytometers to ensure proper data acquisition.
Table 2 Examples of potential fluorophore panels that can be used to analyze myeloid or lymphoid responses to scaffolds
7 Color panel |
Lymphoid phenotyping |
Myeloid phenotyping |
Antibody |
Marker for: |
Antibody |
Marker for: |
CD45 BV421 |
Immune cells |
CD45 BV421 |
Immune cells |
CD19 BV510 |
B cell |
Ly6G BV510 |
Neutrophils |
CD8a AF488 |
Cytotoxic T (CTL) |
Ly6C AF488 |
Monocytes |
NKp46 PE |
NK cell |
CD11c PE |
Dendritic cells |
CD4 PE/Cy7 |
T helper (Th) |
F4/80 PE/Cy7 |
Macrophage |
CD3 APC |
T cell |
SiglecF APC |
Eosinophils |
Viability eFluor780 |
Dead cells |
Viability eFluor780 |
Dead cells |
14 Color panel |
Lymphoid phenotyping |
Myeloid phenotyping |
Antibody |
Marker for: |
Antibody |
Marker for: |
Note: optimization and adjustments, as discussed in the text, should be completed for each study to ensure validity of results. Myeloid cells are very autofluorescent, this allows to negative gate and remove them from lymphoid analyses. NK1.1 is not present in BALB/c mice, panel used for C57BL/6 mice. Intracellular antigen. |
IgD BUV395 |
Mature B cells |
CD86 BUV395 |
“M1” macrophage |
CD45 BUV737 |
Immune cells |
CD45 BUV737 |
Immune cells |
TcRb BV421 |
ab T cell |
CD8a BV421 |
Cross-presenting DCs |
CD38 BV510 |
Plasmablasts |
Ly6G BV510 |
Neutrophils |
NK1.1 BV605b |
NK cell |
Siglec F BV605 |
Eosinophils |
IgM BV786 |
Immature/early B cells |
MHCII BV786 |
Antigen presentation to Th |
CD8a AF488 |
Cytotoxic T (CTL) |
Ly6C AF488 |
Monocytes |
CD4 PerCP/Cy5.5 |
T helper (Th) |
CD11c PerCP/Cy5.5 |
Dendritic cells (DCs) |
NKp46 PE |
NK cell |
CD206 PE |
“M2” macrophage |
CD19 PE/594 |
B cell |
CD197 PE/594 |
Lymph node-homing |
CD3 PE/Cy7 |
T cell |
F4/80 PE/Cy7 |
Macrophage |
FoxP3 APCc |
Treg |
CD200R3 APC |
Basophils & mast cells |
CD11b AF700a |
Myeloid |
CD11b AF700 |
Myeloid |
Viability eFluor780 |
Dead cells |
Viability eFluor780 |
Dead cells |
2.2.4. Technical note: antibody titrations.
When testing new antibodies in your application, just as in immunofluorescence microscopy it is important to titer antibodies to determine the optimal working concentration of your antibody. Each manufacturer publishes a standard working concentration for the antibody, usually in a microgram per milliliter or microgram per cell count; however, this is often an over-estimate and will result in using far more antibody than required for optimal separation and visualization of a population. Most antibodies are used in a range of 1
:
100–1
:
500 (antibody
:
buffer) when staining. To titer the antibodies, you will need to acquire samples that act as both a positive control, and well as your sample of interest. If the sample of interest is a precious or difficult to obtain sample, titers can be done on tissues such as spleen or lymph node, but will need to follow-up with pilot staining an actual sample alongside positive control tissue to ensure proper staining. Titers are frequently calculated against a cell count of one million (1 × 106) cells per sample. Each antibody should be tested in a dilution series, for example 1
:
100, 1
:
200, 1
:
300, 1
:
400 and 1
:
500. Further optimization of staining can be tested after an initial dilution series. The dilution selected should represent a concentration that displays an obvious separation in positive versus negative populations, but not beyond the saturation point of the antibody. For markers that experience a shift in expression as opposed to a binary positive/negative,38 the same protocol is applied but the dilution that is selected is based on the peak staining. To this extent, positive controls with known expression of the marker of interest are critical.
2.3. Intracellular cytokine staining & stimulation
2.3.2. Selection of fixative and permeabilization reagents.
Multiple reagents are available for fixation and permeabilization and range from commercially available compounds to in-house made reagents. The most common fixatives are paraformaldehyde based, ranging from 2.0–4.0% paraformaldehyde diluted in PBS. Methanol can also be used and serves as a permeabilization agent as well, and is used in combination with aldehyde-based fixatives.40 Aldehyde-based fixatives can yield increases in autofluorescence, but still remain the most popular fixatives in flow cytometry.41 Permeabilization reagents, often saponin (0.1%) or Triton-X100 (0.01–0.05%), can be selected through testing to evaluate which compound yields best results for your antibodies and proteins of interest.42
2.3.3. Technical notes for cell viability.
Due to the strong stimulation with calcium ionophores, stimulation for cytokine staining can reduce viability of cells, especially murine lymphocytes. This is especially true if there are a large number of neutrophils in the sample that can secrete oxide radicals and harmful proteins. To combat oxidative stress of the stimulation, beta-mercaptoethanol (2-mercaptoethanol, b-ME) is added to the media.43 b-ME is a reducing agent that will prevent damage to cells by oxide radicals that can build up in the stimulation media. Additionally, cells should be kept at a manageable concentration in the stimulation media and avoid over-crowding during stimulation which can also decrease viability. Certain cell types such as neutrophils can be activated44 and greatly decrease the viability of T cells during PMA-ionomycin stimulation. When analyzing samples with large numbers of neutrophils, such as implanted materials that elicit a severe FBR, care should be taken to remove as many neutrophils as possible before stimulation. Furthermore, as stimulation can selectively decrease viability of different cell types, any measurements of overall cellular abundance in a sample (ex. percent of CD45+ immune cells that are T cells) should be completed in a panel that does not involve ICS.
2.4. Specific considerations for cell types
2.4.1. Myeloid.
Myeloid cells can be difficult to work with for multiple reasons. These cells are very adherent and can require stronger dissociation protocols compared to lymphoid cells, and are frequently the dominant population in the response to an implanted material. For example, mechanical disruption is sufficient for cell isolation to analyze T or B cells in a spleen, but a collagenase-mediated tissue digestion is the preferred method for isolation of macrophages and dendritic cells. Furthermore, they are a very heterogeneous population without very strong binary markers, meaning that classification of these cells via flow cytometry can be difficult without the proper controls. They also tend to have higher levels of autofluorescence due to high phagocytic and exocytic activities that can create false signals in multiple channels, especially green channels.45 To combat these difficulties, there must be ample preparation time and testing of your antibodies with proper controls for the sample type that you will be ultimately analyzing. Continuous use of controls throughout each experiment is required to ensure the signal that you are detecting on the flow cytometer is not due to compensation issues or autofluorescence.
As myeloid cells tend to reside on more of a spectrum, it is very difficult to find markers that accurately stain all cell types that you are hoping to classify. One example is the case of peripheral blood-derived (blood monocyte-derived) versus tissue-resident macrophages.46 In mice, a standard peripheral blood-derived macrophage marker is F4/80; however, F4/80 will not stain many tissue-resident macrophages. Other markers used to identify tissue-resident macrophages include CD169, CD68, CD209, and others. Some tissue-resident macrophages express markers associated with other cells.47 Alveolar macrophages express Siglec-F (commonly associated with eosinophils) and many others express CD11c (associated with dendritic cells). Monocytes that have extravasated and matured to macrophages in the tissue can also be characterized via the expression of Ly6C, CD43, CCR2, CD115, and CX3CR1.48
Furthermore, functional assessments of myeloid cells can also be performed via flow cytometry. For example, evaluation of phagocytosis potential can be evaluated through incubation with fluorescent microparticles that can be measured simultaneously with surface marker phenotyping on a flow cytometer.49 Cells can be exposed to fluorescent microparticles both in vivo and in vitro depending upon the experimental setup.
2.4.2. Lymphoid.
Lymphoid cells may be smaller in number in response to biomaterials, often making their analysis more challenging. As with myeloid cells, proper identification of lymphoid cells requires multiple markers, implementing both positive and negative gates. Discrimination from myeloid cells using CD11b can help remove many myeloid cells from the analysis creating a cleaner gating strategy for lymphoid cells, as some myeloid cells can express lymphoid markers as well. Certain lymphoid or lymphoid-like cells can express proteins that are usually thought of as a binary marker of a certain cell type. For example, NK cells (specifically NKT cells) can express CD3 and TCRb, which are normally used to identify T cells. Therefore, to properly identify a pure T cell population, one can include NK markers, and gate on cells that are not NK cells, then further analyze the T cells. Furthermore, some markers are often used alone (i.e. CD25) to identify cell populations (CD25 for Tregs) but for confirmation of cell population a second marker (FoxP3) is included in thorough analyses.50
Examples of several publications with their digestion enzymes and selected markers for myeloid and lymphoid cell identification from in vivo samples can be found in Table 4.
Table 4 Examples of digestion and staining used in different scaffold environments.21,25,27,29,50 PCL = polycaprolactone. PLGA = polylactic co-glycolic acid. PEI = polyetherimide. ECM = decellularized extracellular matrix. PEG-DA = polyethylene glycol diacrylate. PE = polyethylene. A comprehensive list of various digestion conditions used in applications other than scaffolds has been compiled by Worthington Biochemical60
Publication |
Tissue |
Material |
Digestion |
Cells of interest/markers |
Ballestas et al., Acta Biomater., 201927 |
Oral mucosa (oronasal fistula) |
PCL & PLGA nanofibers |
Collagenase I (1 mg ml−1) |
Myeloid: CD11b, Ly6C, CD64, MerTK, CD206 |
Li et al., Nat. Mater., 201821 |
Subcutaneous implant |
PEI adsorbed to mesoporous silica rods |
Collagenase IV (250 U ml−1) |
Dendritic cells: CD11c, CD86, CCR7 and SIINFEKL/H-2Kb |
Sadtler et al., Biomaterials, 201829 |
Subcutaneous implant and muscle (injury) |
ECM particles, PEG-DA hydrogel, PE particles |
Liberase TL (0.5 mg ml−1) + DNase I (0.2 mg ml−1) |
Myeloid: CD45, CD11b, CD11c, Ly6C, Ly6G, F4/80, CD86, CD206, MHCII. Lymphoid: CD3, CD19, NK1.1 |
Headen et al., Nat. Mater., 201850 |
Kidney capsule (+spleen, lymph node) |
Maleimide-terminated four-arm poly(ethylene) glycol microgels |
Liberase TL |
T Cell: CD3, CD4, CD8, CD62L, CD44, CD25, FoxP3 |
Vegas et al., Nat. Med., 201625 |
Subcutaneous and intraperitoneal implants |
Alginate hydrogels |
gentleMACS Dissociator and 2 mM EDTA |
Myeloid: CD68, Ly6G, CD11b. Lymphoid: CD19, IgM, CD8, NK1.1 |
2.5. Selecting proper controls
2.5.1. Positive controls.
When running new samples, especially with biomaterial implants when there is very little known regarding the types of cells that make up the immune microenvironment, it is important to include controls that will have the cells and proteins of interest to validate the antibody staining. Frequently, central lymphoid organs such as spleens and lymph nodes are used for controls which will allow for the testing of the antibody and serve as a confirmation that staining was technically accurate in the case that there are cells that are not present in the sample of interest. In order to get strong positive controls for some activation markers, cells can be stimulated in vitro with cytokines to induce a stronger expression of proteins of interest. For example, macrophages can be stimulated with bacterial lipopolysaccharide and interferon gamma to induce expression of co-stimulatory and M1/inflammatory markers.
2.5.2. Negative controls, isotypes & FMO's.
In addition to positive controls, negative controls are critically important for proper analysis of flow cytometry, especially for myeloid cells that as previously mentioned can have high levels of autofluorescence.51 Prior to beginning with experiments, it is important to set up and evaluate fluorescence minus one (FMO) and isotype controls (Fig. 1). These tests can be combined into an FMO-isotype control for each marker. FMO controls, as the name suggests, are samples stained with the full panel minus one marker. The marker that is excluded is the fluorophore that the sample is controlling for. FMO's allow for the detection of any positive signal due to compensation and fluorescence overlap. To extend the use of these controls, in addition to the FMO panel, an isotype control antibody in place of the marker of interest. This control will then serve two purposes, one to ensure that the isotype does not cause any background staining in the channel, and two, monitor as compensation/fluorescence overlap.
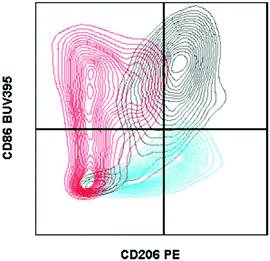 |
| Fig. 1 Fluorescence minus one controls on macrophages from biomaterial implant. Example of two fluorescence minus one controls gated against the full-stained sample. A fluorescence minus one control is a sample stained with every antibody of the tested panel except for the marker that the control is evaluating. Cells isolated from a C57BL/6 (Jackson Labs) murine muscle injury (using Liberase TL digestion) were stained with the antibody panel described in Table 2. CD206 FMO = red. CD86 FMO = blue. Full stained sample = black. | |
In the context of material implantation, such controls are critical as there is often phagocytosis of particles (from the scaffold or surrounding tissue damage) that will increase cellular autofluorescence. Furthermore, as there is still relatively little known about the identity and behavior of these cells from different locations, each model or material may have different background signals and signal intensity which can change what is considered negative versus positive.
2.6. Analysis
2.6.1. Hand-gating.
Gates should be set through use of FMO controls and positive controls. In higher color experiments, incorporation of and utilization of negative gates to both positively and negatively identify cell types allows for a cleaner analysis. When staining more than one panel for each sample, if common markers are included in both panels (ex. Viability dye and CD45) then an overall population (percent of viable CD45+ cells) can be described and used to integrate both panels into one data set. After gates are set, it is important to check back-gating to ensure that you have not accidentally gated out any cells of interest. As previously mentioned, myeloid cells, especially macrophages, tend to be more autofluorescent than lymphoid cells. If gating for viable cells, it is imperative to ensure that higher autofluorescent cells have not been gated out due to this property. An example of hand-gating is seen in Fig. 2.
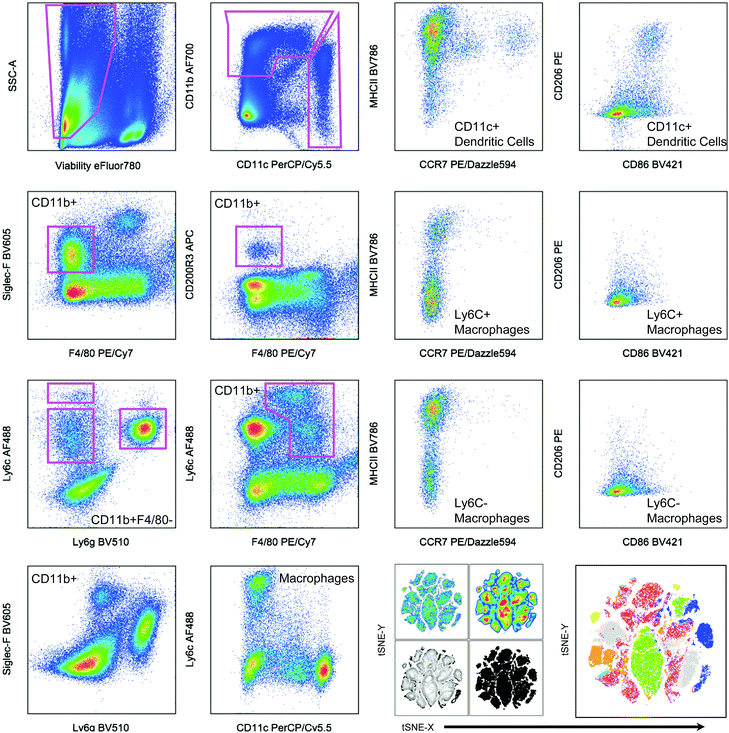 |
| Fig. 2 Hand gating and dimensionality-reduction algorithms used on 14-color myeloid panel. Cells isolated from the spleen of a tumor bearing mouse as a control for panel development. C57BL/6 (Jackson Labs) murine spleen cells, isolated with Liberase TL digestion, were stained with the antibody panel described in Table 2. Samples were then gated based on controls (example of FMO shown in Fig. 1). tSNE clustering was performed with the following parameters: iterations – 1000, perplexity – 20, eta/learning rate – 200, theta – 0.05. | |
2.6.2. Dimensionality-reduction algorithms.
As flow cytometry technology has advanced, so have the number of fluorophores and channels on cytometers, allowing for higher-plex analysis of immune cells, which can provide vital information on cellular environments that are less understood such as those created by biomaterials (Fig. 2). Different computational methods have been adapted for use in flow cytometry experiments.52,53 These include dimensionality-reduction methods such as tSNE (t-stochastic neighbor embedding), UMAP (Uniform Manifold Approximation and Projection), and SPADE (Spanning-tree Progression Analysis of Density-normalized Events) as well as more common approaches such as principle component analysis (PCA).54,55 Each uses a different algorithm to evaluate the similarity or difference of single cells given their matrix of expression values (fluorescence intensity and scatter). Plugins on user-friendly software have made these algorithms accessible to scientists with minimal experience in big data and statistical methods, which is both positive and negative in the terms of analyzing flow cytometry data. Such algorithms are great for all levels to be able to display a high-dimensionality data set in one plot, thereby conveying the complexity of immune cell types and subtypes within a given sample. Utilizing multiple dimensionality-reduction algorithms to confirm the same observations can also help interpret data in a more reliable manner. However, it is possible to over-cluster and over-interpret these data. If these algorithms are forced to undergo more iterations or form more clusters than the data that is being analyzed calls for, then it is possible to falsely identify novel populations that appear due to over-clustering. It is important to consult with a statistician or data scientist prior to making any definitive conclusions based on these algorithms. When running these algorithms for a basic visualization of population, programs such as FlowJo allow the user to concatenate files (from biologic replicates or different samples) run the algorithm with default settings, then in the case of different samples, re-separate the different samples via gating on the “Sample ID” parameter to display differences between treatments, timepoints, or other variables. However, as mentioned, any in-depth computational analysis should be evaluated with a statistician or systems biologist.
2.7. Long-term experiments
In some cases, researchers will be conducting studies over multiple years that relate to each other. In this scenario, it is important to carry over enough controls throughout the study to ensure validity of data and ability to compare across different timepoints. To control for any lot-to-lot variability of antibodies or drifting of PMT (photomultiplier tube) voltages on the flow cytometer due to servicing or unforeseen issues with laser power, one technique is to utilize control samples such as PMT beads to ensure that the voltage aligns with the proper fluorescence intensity read on the detector.56 Using these beads, which are used to calibrate the machine or for compensation purposes, will give a signal that is independent of any biologic variability, and yield a bookmark for confirming the setup of the machine over a longitudinal study.
When working in animal models, it is important to include biological controls that can be used to compare across timepoints and serve for monitoring shifts in fluorescence intensity via fold change of MFI. These would include sham surgical controls for implantation or injury studies, as well as control implants if a material is being modified. Percent positive measurements are more reliable across longitudinal studies as compared to MFI as they are not as sensitive to shifts in the mean of the population. However, if sham and control implants are included for each study, fold changes of MFI over the control sample provide an alternative when MFI is the proper measurement (i.e. shifts in expression as opposed to binary on/off signal). Through the use of these controls, data can be acquired and analyzed reliably over long-term studies without concern for technical deviations in instrumentation or reagents.
2.8. Data reporting
As with many techniques, proper data reporting is critical for peer review and complete transparency and communication of conclusions. When including flow cytometry data in a manuscript, best practices are to include examples of your gating strategies used to identify cell populations, controls such as FMOs especially for low-intensity markers or highly autofluorescent cells, and example dot plots of the data from which you have derived any dot- or bar graphs.
3. Conclusions
Flow cytometry is a powerful technique that is growing in importance in the fields of tissue engineering and regenerative medicine. Modifications of standard protocols are necessary when considering the immune environment of scaffolds, which can differ greatly from responses to infections or malignancies. As such, attentiveness at all stages of panel design, cell isolation, data acquisition, and analysis are necessary to properly gather and interpret data. As we any method, validation of results using other experimental modalities is critical.
Benefits of flow cytometry, including protein- and cell-level analysis for the immune cell (and other) populations in the scaffold microenvironment, does come with limitations. At this time, higher-end cytometers advertise simultaneous detection of 50 parameters on cells; however, this is confounded by compensation and spectral overlap variables, and limited based on reagent availability. Advances in technologies have led to the innovation of so-called “spectral” cytometers that remove spectral overlap through evaluation of the emission spectra of a given fluorophore as opposed to a percent-based overlap of signals of fluorophores into other detectors. Nevertheless, there is a limitation on parameters of analysis that can be overcome by combining flow cytometry, specifically sorting capabilities, with bulk and single-cell sequencing. Cytometers can sort single cells into wells for sequencing in a well-based single cell format, or sort populations that can be further processed by microfluidic-based sequencing platforms. After sequencing, any results on the gene expression level can be further validated through a second flow cytometry assay or other protein-level assay such as ELISA. Such sorting techniques can also be combined with ELISA, proteomics, or western blots directly to measure protein secretion, proteome, or specific phosphorylation events that are best suited for molecular biology analyses.
As previously mentioned, there is a loss of location-specific data that cannot be learned from flow cytometry alone. Therefore, quantitative characterization of the immune cell environment, couple with immunofluorescence or histologic evaluations can synergize to yield both a quantitative and structural view of the environment. Imaging has also been combined simultaneously with flow cytometers, through imaging flow cytometers (IFC) that provide single-cell images of stained cells. Such cytometers can gather information regarding cell morphology and localization of protein expression that would be lost with a standard cytometer. These methods have been used in comparison of cancerous and non-cancerous cells,57 discriminating different stages of apoptosis and necrosis,58 and even classifying phytoplankton.59
Further expansion of utilizing these techniques in combination with imaging modalities and molecular biology platforms will promote a thorough understanding of the immune interactions with materials in both pre-clinical and clinical testing (Fig. 3). These examinations will both characterize developed materials as well as uncover fundamental biologic processes involved in device implantation and tissue engineering that may be exploited for future therapeutics.
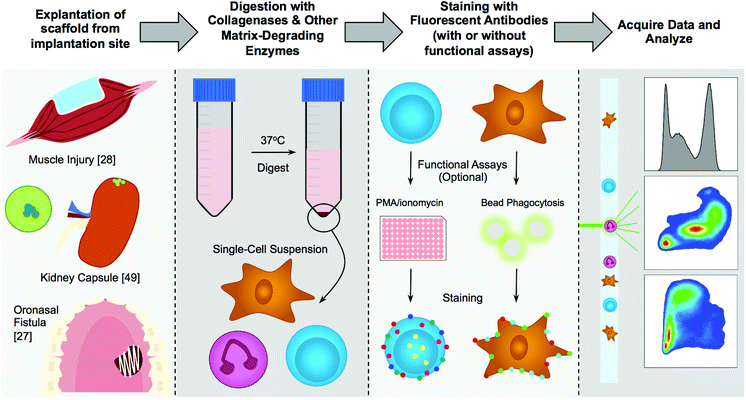 |
| Fig. 3 An overview of flow cytometry on biomaterial scaffolds. The tissue is explanted, finely diced, then digested with a matrix-degrading enzyme (commonly collagenases), followed by optional stimulation of T cells for intracellular cytokine staining or other functional assays such as phagocytosis of fluorescent beads by macrophages, then samples are run on a cytometer and data is analyzed via hand gating or analytic algorithms. | |
Ethics statement
All procedures described in this manuscript were reviewed and approved by the Committee for Animal Care at Johns Hopkins University School of Medicine or the Massachusetts Institute of Technology in accordance with the guidelines set forth by IACUC.
Conflicts of interest
There are no conflicts of interest to declare.
Acknowledgements
The authors acknowledge the following financial support: J. E. was supported by the Morton Goldberg Professorship, the Bloomberg∼Kimmel Institute for Cancer Immunotherapy, and grants from the Department of Defense. K. S. was supported by the Ruth L. Kirschstein NRSA Postdoctoral Fellowship #1F32EB025688-01A1 from the National Institute of Biomedical Imaging and Bioengineering of the National Institutes of Health.
References
- N. P. Ziats, K. M. Miller and J. M. Anderson, Biomaterials, 1988, 9, 5–13 CrossRef CAS PubMed
.
-
J. M. Anderson, A. Rodriguez and D. T. Chang, in Seminars in immunology, Elsevier, 2008, vol. 20, pp. 86–100 Search PubMed
.
- J. M. Anderson and K. M. Miller, Biomaterials, 1984, 5, 5–10 CrossRef CAS PubMed
.
- W. G. Brodbeck, M. S. Shive, E. Colton, Y. Nakayama, T. Matsuda and J. M. Anderson, J. Biomed. Mater. Res., 2001, 55, 661–668 CrossRef CAS PubMed
.
- S. F. Badylak, J. E. Valentin, A. K. Ravindra, G. P. McCabe and A. M. Stewart-Akers, Tissue Eng., Part A, 2008, 14, 1835–1842 CrossRef CAS PubMed
.
-
G. Radcliff and M. J. Jaroszeski, in Flow Cytometry Protocols, Springer, 1998, pp. 1–24 Search PubMed
.
- L. A. Herzenberg, D. Parks, B. Sahaf, O. Perez, M. Roederer and L. A. Herzenberg, Clin. Chem., 2002, 48, 1819–1827 CAS
.
- K. Vermaelen and R. Pauwels, Cytometry, Part A, 2004, 61, 170–177 CrossRef PubMed
.
- A. E. Cardona, D. Huang, M. E. Sasse and R. M. Ransohoff, Nat. Protoc., 2006, 1, 1947 CrossRef CAS PubMed
.
- B. Weigmann, I. Tubbe, D. Seidel, A. Nicolaev, C. Becker and M. F. Neurath, Nat. Protoc., 2007, 2, 2307 CrossRef CAS PubMed
.
- L. Wilson, C. Fathke and F. Isik, BioTechniques, 2002, 32, 548–551 CrossRef CAS PubMed
.
- N. Seach, K. Wong, M. Hammett, R. L. Boyd and A. P. Chidgey, J. Immunol. Methods, 2012, 385, 23–34 CrossRef CAS PubMed
.
- W. Thiele, M. Rothley, A. Schmaus, D. Plaumann and J. Sleeman, Lymphology, 2014, 47, 177–186 CAS
.
- M. Iannacone, E. A. Moseman, E. Tonti, L. Bosurgi, T. Junt, S. E. Henrickson, S. P. Whelan, L. G. Guidotti and U. H. Von Andrian, Nature, 2010, 465, 1079 CrossRef CAS PubMed
.
- J. C. Nussbaum, S. J. Van Dyken, J. Von Moltke, L. E. Cheng, A. Mohapatra, A. B. Molofsky, E. E. Thornton, M. F. Krummel, A. Chawla and H.-E. Liang, Nature, 2013, 502, 245 CrossRef CAS PubMed
.
- A. R. Harris, J. X. Yuan and J. M. Munson, Methods, 2018, 134, 20–31 CrossRef PubMed
.
- A. Mohapatra, S. J. Van Dyken, C. Schneider, J. C. Nussbaum, H.-E. Liang and R. M. Locksley, Mucosal Immunol., 2016, 9, 275–286 CrossRef CAS PubMed
.
- J. R. Rock, C. E. Barkauskas, M. J. Cronce, Y. Xue, J. R. Harris, J. Liang, P. W. Noble and B. L. M. Hogan, Proc. Natl. Acad. Sci. U. S. A., 2011, 108, E1475–E1483 CrossRef CAS PubMed
.
- D. H. D. Gray, A. P. Chidgey and R. L. Boyd, J. Immunol. Methods, 2002, 260, 15–28 CrossRef CAS PubMed
.
- T. Später, F. S. Frueh, R. M. Nickels, M. D. Menger and M. W. Laschke, J. Biol. Eng., 2018, 12, 24 CrossRef PubMed
.
- A. W. Li, M. C. Sobral, S. Badrinath, Y. Choi, A. Graveline, A. G. Stafford, J. C. Weaver, M. O. Dellacherie, T.-Y. Shih and O. A. Ali, Nat. Mater., 2018, 17, 528 CrossRef CAS PubMed
.
- M. Abuzakouk, C. Feighery and C. O'Farrelly, J. Immunol. Methods, 1996, 194, 211–216 CrossRef CAS PubMed
.
- M. Jungblut, K. Oeltze, I. Zehnter, D. Hasselmann and A. Bosio, J. Visualized Exp., 2008, e1029 Search PubMed
.
- M. Jungblut, K. Oeltze, I. Zehnter, D. Hasselmann and A. Bosio, J. Visualized Exp., 2008, 22, e1029 Search PubMed
.
- A. J. Vegas, O. Veiseh, M. Gürtler, J. R. Millman, F. W. Pagliuca, A. R. Bader, J. C. Doloff, J. Li, M. Chen and K. Olejnik, Nat. Med., 2016, 22, 306 CrossRef CAS PubMed
.
- D. M. Mosser and J. P. Edwards, Nat. Rev. Immunol., 2008, 8, 958 CrossRef CAS PubMed
.
- S. A. Ballestas, T. C. Turner, A. Kamalakar, Y. C. Stephenson, N. J. Willett, S. L. Goudy and E. A. Botchwey, Acta Biomater., 2019, 91, 209–219 CrossRef CAS PubMed
.
- K. Sadtler, K. Estrellas, B. W. Allen, M. T. Wolf, H. Fan, A. J. Tam, C. H. Patel, B. S. Luber, H. Wang and K. R. Wagner, Science, 2016, 352, 366–370 CrossRef CAS PubMed
.
- K. Sadtler, M. T. Wolf, S. Ganguly, C. A. Moad, L. Chung, S. Majumdar, F. Housseau, D. M. Pardoll and J. H. Elisseeff, Biomaterials, 2019, 192, 405–415 CrossRef CAS PubMed
.
- K. Sadtler, B. W. Allen, K. Estrellas, F. Housseau, D. M. Pardoll and J. H. Elisseeff, Tissue Eng., Part A, 2017, 23, 1044–1053 CrossRef CAS PubMed
.
-
K. Sadtler, S. D. Sommerfeld, M. T. Wolf, X. Wang, S. Majumdar, L. Chung, D. S. Kelkar, A. Pandey and J. H. Elisseeff, in Seminars in immunology, Elsevier, 2017, vol. 29, pp. 14–23 Search PubMed
.
- J. M. Wentworth, G. Naselli, W. A. Brown, L. Doyle, B. Phipson, G. K. Smyth, M. Wabitsch, P. E. O'Brien and L. C. Harrison, Diabetes, 2010, 59, 1648–1656 CrossRef CAS PubMed
.
- J. Helft, J. Böttcher, P. Chakravarty, S. Zelenay, J. Huotari, B. U. Schraml, D. Goubau and C. R. e Sousa, Immunity, 2015, 42, 1197–1211 CrossRef CAS PubMed
.
- S. Rose, A. Misharin and H. Perlman, Cytometry, Part A, 2012, 81, 343–350 CrossRef PubMed
.
- ThermoFisher Scientific, Fluorescence SpectraViewer, https://www.thermofisher.com/order/spectra-viewer.
- BD Biosciences, BD Spectrum Viewer, http://www.bdbiosciences.com/en-us/applications/research-applications/multicolor-flow-cytometry/product-selection-tools/spectrum-viewer.
- BioLegend, Fluorescence Spectra Analyzer, https://www.biolegend.com/en-us/spectra-analyzer.
-
O. D. Perez, P. O. Krutzik and G. P. Nolan, in Flow Cytometry Protocols, Springer, 2004, pp. 67–94 Search PubMed
.
- M. T. Wolf, S. Ganguly, T. L. Wang, C. W. Anderson, K. Sadtler, R. Narain, C. Cherry, A. J. Parrillo, B. V. Park and G. Wang, Sci. Transl. Med., 2019, 11, eaat7973 CrossRef CAS PubMed
.
- A. A. Pollice, J. Philip Mccoy Jr., S. E. Shackney, C. A. Smith, J. Agarwal, D. R. Burnolt, L. E. Janocko, F. J. Hornicek, S. G. Singh and R. J. Hartsock, Cytometry, 1992, 13, 432–444 CrossRef CAS PubMed
.
- J. S. Collins and T. H. Goldsmith, J. Histochem. Cytochem., 1981, 29, 411–414 CrossRef CAS PubMed
.
- H. Y. Lan, P. Hutchinson, G. H. Tesch, W. Mu and R. C. Atkins, J. Immunol. Methods, 1996, 190, 1–10 CrossRef CAS PubMed
.
- M. J. Bevan, R. Epstein and M. Cohn, J. Exp. Med., 1974, 139, 1025–1030 CrossRef CAS PubMed
.
- H. Sengeløv, L. Kjeldsen and N. Borregaard, J. Immunol., 1993, 150, 1535–1543 Search PubMed
.
- F. Li, M. Yang, L. Wang, I. Williamson, F. Tian, M. Qin, P. K. Shah and B. G. Sharifi, J. Immunol. Methods, 2012, 386, 101–107 CrossRef CAS PubMed
.
- S. Gordon, A. Plüddemann and F. Martinez Estrada, Immunol. Rev., 2014, 262, 36–55 CrossRef CAS PubMed
.
- L. C. Davies, S. J. Jenkins, J. E. Allen and P. R. Taylor, Nat. Immunol., 2013, 14, 986 CrossRef CAS PubMed
.
- L. Ziegler-Heitbrock, P. Ancuta, S. Crowe, M. Dalod, V. Grau, D. N. Hart, P. J. M. Leenen, Y.-J. Liu, G. MacPherson and G. J. Randolph, Blood, 2010, 116, e74–e80 CrossRef CAS PubMed
.
- J. A. Steinkamp, J. S. Wilson, G. C. Saunders and C. C. Stewart, Science, 1982, 215, 64–66 CrossRef CAS PubMed
.
- D. M. Headen, K. B. Woodward, M. M. Coronel, P. Shrestha, J. D. Weaver, H. Zhao, M. Tan, M. D. Hunckler, W. S. Bowen and C. T. Johnson, Nat. Mater., 2018, 17, 732 CrossRef CAS PubMed
.
- J. W. Tung, D. R. Parks, W. A. Moore, L. A. Herzenberg and L. A. Herzenberg, Clin. Immunol., 2004, 110, 277–283 CrossRef CAS PubMed
.
- Y. Saeys, S. Van Gassen and B. N. Lambrecht, Nat. Rev. Immunol., 2016, 16, 449 CrossRef CAS PubMed
.
- H. Zare, P. Shooshtari, A. Gupta and R. R. Brinkman, BMC Bioinformatics, 2010, 11, 403 CrossRef PubMed
.
- E. Lugli, M. Roederer and A. Cossarizza, Cytom. Part A, 2010, 77, 705–713 CrossRef PubMed
.
- C. E. Pedreira, E. S. Costa, Q. Lecrevisse, J. J. M. van Dongen, A. Orfao and E. Consortium, Trends Biotechnol., 2013, 31, 415–425 CrossRef CAS PubMed
.
- S. P. Perfetto, D. Ambrozak, R. Nguyen, P. Chattopadhyay and M. Roederer, Nat. Protoc., 2006, 1, 1522–1530 CrossRef CAS PubMed
.
- D. A. Basiji, W. E. Ortyn, L. Liang, V. Venkatachalam and P. Morrissey, Clin. Lab. Med., 2007, 27, 653–670 CrossRef PubMed
.
- T. C. George, D. A. Basiji, B. E. Hall, D. H. Lynch, W. E. Ortyn, D. J. Perry, M. J. Seo, C. A. Zimmerman and P. J. Morrissey, Cytom. Part A J. Int. Soc. Anal. Cytol., 2004, 59, 237–245 CrossRef PubMed
.
- H. M. Sosik and R. J. Olson, Limnol. Oceanogr. Methods, 2007, 5, 204–216 CrossRef
.
-
W. B. Corporation, Worthington Biochemical Online Tissue Dissociation Guide, http://www.worthington-biochem.com/tissuedissociation/basic.html Search PubMed.
|
This journal is © The Royal Society of Chemistry 2019 |
Click here to see how this site uses Cookies. View our privacy policy here.