DOI:
10.1039/D4LP00199K
(Paper)
RSC Appl. Polym., 2024,
2, 1043-1056
Polydiacetylene/copolymer sensors to detect lung cancer breath volatile organic compounds†
Received
17th June 2024
, Accepted 29th July 2024
First published on 30th July 2024
Abstract
Early lung cancer detection is imperative to increase the 5-year survival rate and reduce cancer mortality. Existing diagnosis techniques involve costly, time-consuming, and often invasive tests. The emergence of volatile organic compounds (VOCs) as a disease biomarker offers a non-invasive avenue for early detection of lung cancer through breath analysis. Recently, polydiacetylene (PDA)-based colorimetric sensors have shown the potential to detect VOCs. In this work, we developed PDA/copolymer paper sensors to detect 5 potential early lung cancer VOC biomarkers, including ethylbenzene, 2-butanone, hexanal, 2-ethylhexanol, and undecane. Polymethyl methacrylate (PMMA), polyvinylpyrrolidone (PVP), polystyrene (PST), and polyethylene glycol (PEG) were selected as copolymers based on their chemical affinity and solvating properties. Different copolymer molecular weights and PDA/copolymer mixing ratios were investigated and their responses to standard breath temperature and relative humidity (35 °C, 60% RH and 90% RH) were evaluated. We then developed an array containing 11 PDA/copolymers and exposed them to gaseous VOC biomarkers and common breath interferents (ethanol, acetone, and isoprene) in a custom-built reactor. The colorimetric data were simultaneously analyzed using principal component analysis and results showed highly discriminating properties. We demonstrated the detection of 2-butanone (LOD = 267 ppmv), ethylbenzene (LOD = 457 ppmv), and ethanol (LOD = 269 ppmv) within 15 min. This study aims to establish a cost-effective, user-friendly, and non-invasive methodology for early detection of lung cancer.
1. Introduction
Lung cancer (LC) is the second most common cancer worldwide and is the leading cause of cancer-related deaths in both men and women.1 In 2020, 18% of global cancer deaths (1.8 million people) were from LC and this number is projected to reach 3.2 million in 2050.2,3 The 5-year survival rate of LC is exceedingly poor (7–25%) compared to other major cancers.4 It is well known that early diagnosis saves lives. When diagnosed as early in stage I, LC patients’ 5-year survival rate goes up to 90%.5 Ironically, however, ∼75% of LC cases were discovered at an advanced stage (stage III/IV) when treatment options are limited.5 In addition, the majority of cases are often incidentally discovered from chest scans.6,7 Current LC detection methods involve a multi-stage process, typically beginning with a chest X-ray or computed tomography (CT) scan and progressing to tissue biopsy, blood tests, bronchoscopy, and staging scans (positron emission tomography (PET) or magnetic resonance imaging (MRI)).8 Undeniably, these techniques are valuable and powerful to screen and diagnose LC. However, their complexity, cost, and invasiveness hinder their widespread use and accessibility to low- and middle-income countries where cancer mortality rate is the highest.9,10 Thus, there is a pressing need for alternative technologies to equalize global healthcare access for early LC diagnosis.
Breath tests emerged as a predictive tool for pathological conditions following Linus Pauling's discovery in the 1970s, which established an association between human metabolism and breath composition.11–13 Building on this, approximately four decades ago, Michael Phillips pioneered the detection of volatile organic compounds (VOCs) from breath for cancer diagnosis, particularly focusing on lung and breast cancers.14–17 VOCs are ubiquitous small organic compounds with high vapor pressure at ambient conditions. At the onset of a disease, VOC profiles change due to the altered hormones and metabolic processes to cope with pathogens. VOC identification from the breath, urine, feces, and sweat paves the way to monitor health conditions or diagnose a disease in a minimally or non-invasive manner.18–20 In particular, breath sampling is attractive as it is easily accessible, non-invasive, and does not need extensive sample pre-treatment when compared to blood samples. Breath is faster to collect and has minimal environmental influence when compared to sweat and has the benefit of cleaner handling when compared to urine and feces specimens. The natural presence of VOCs in the breath is attributed to the metabolic processes occurring during the blood-gas exchange in the alveolar space, subsequently providing a snapshot of human health.21–23
The current gold standard for breath VOC detection is gas chromatography with mass spectrometry (GC-MS).24 However, it is time-consuming (>1 hour per sample), unsuitable for real-time analysis, and requires specialized skills to operate.25,26 Other analytical techniques, such as Selected Ion Flow Tube Mass Spectrometry (SIFT-MS), Proton Transfer Reaction Mass Spectrometry (PTR-MS), and Laser Absorption Spectroscopy (LAS) have been used to enable real-time testing.27–29 Despite the improvement in the analysis duration, all these technologies are bulky, expensive, and difficult to operate. Sensor technologies, including electrochemical13,30,31 and colorimetric32,33 have become increasingly vital in medical diagnostics as they are generally low-cost, easy-to-use, portable, rapid, and disposable. Generally, electrochemical sensors have the merits of greater sensitivity and longer lifetimes when compared to colorimetric sensors. However, color-changing sensors are cheaper, easier to interpret, simpler to produce, and more suited for disposable tests. They are commonly synthesized as an array of chemoresponsive dyes, such as porphyrins,34 pH indicators,35 natural pigments,36 and conjugated polymers.37 The color differences before and after analyte exposure were analyzed with statistical methods for results interpretation.34,38 Automated results generation is possible via a smartphone application.39
Polydiacetylene (PDA) is a class of color-responsive polymers that have been widely explored as colorimetric sensors owing to their distinct optical properties and facile yet high purity fabrication process. PDAs are prepared by photopolymerizing (254 nm UV) the self-assembling and highly aligned diacetylene (DA) monomers with specific packing geometry.40 The self-assembly of DA monomers is driven by three dominating interactions along the neighboring side chains, which are hydrogen bonding along the head group, π–π stacking along the conjugated backbone, and dispersion forces between the alkyl tails. The efficient overlap of the p-orbital along the backbone results in a non-fluorescent blue PDA with a characteristic absorption peak of ∼640 nm. Exposure to stimuli, such as VOC, shifts PDA to a fluorescent red phase and reduces the absorption peak to ∼540 nm. Most notably, PDA is attractive as on-site colorimetric sensors because the blue-to-red color change is visible via the naked eye. Whilst it is also possible to analyze its fluorescent intensity as it transitions from the non-fluorescent blue phase to the fluorescent red phase, this is less explored as it requires an additional read-out device. PDA photopolymerization process requires no initiator or catalyst which enables the production of PDAs with high purity and uniformity.37,41 PDA is versatile as it can be synthesized in various configurations (i.e.: mono- or multi-layer film, flat or tubular form, single or multilayer vesicles, or micelles) and phases (i.e.: solid, gel, or liquid).41
The first work on PDA for gaseous VOC detection was in the early 2000s, when PDA films were fabricated to detect and distinguish saturated chloroform, tetrahydrofuran, ethyl acetate, and hexane by the patterns generated in the 4 films.42 Since then, other studies have synthesized PDA as paper sensors,43,44 films,45,46 and in aerogels47,48 to detect VOCs.41 PDA sensitivity and selectivity can be tuned by various methods as detailed in our previous review.49 The three most common ways are to change its alkyl chain length,46,50 modify the head group,39,51 and embed support matrix.47,52 The last avenue is an effective yet least laborious method to tune PDA's response. It is done by incorporating PDA in matrix polymers that have different solubility in the target VOCs.44,53 Consequent to this solubility or “VOC capture” difference, PDA responses are varied. In this work, we synthesized initiator-free PDA/copolymer composites as color-changing sensors to detect breath biomarkers indicative of early lung cancer. We custom-built a reactor for the gaseous VOC sensor testing. The five VOC biomarker compounds, each from a different chemical class, are ethylbenzene,54,55 2-ethyl-1-hexanol,56–58 2-butanone,57,59–63 hexanal,55,57,60,64 and undecane.16,55,57 Sensor responses against three common breath interferents, including acetone, ethanol, and isoprene65 were also evaluated.
2. Experimental section
2.1 Materials
10,12-Pentacosadiynoic acid (PCDA; >97%), 10,12-Tricosadiynoic acid (TCDA; >98%), polymethyl methacrylate Mw 15
000 (PMMA15K), polyvinylpyrrolidone Mw 10
000 (PVP10K) and Mw 40
000 (PVP40K), polystyrene Mw 35
000 (PST35K), reagent grade absolute acetone, isoprene, ethylbenzene, 2-butanone, 2-ethyl-1-hexanol, undecane, and hexanal were obtained from Sigma Aldrich. Polyethylene glycol (PEG) with various molecular weights, including 4000 (PEG4K), 1500 (PEG1.5K), 1000 (PEG1K), 400 (PEG400), and 200 (PEG200) were also purchased from Sigma Aldrich. The chemical structure of each polymer is shown in Fig. S1 (ESI†). Whatman® Grade 1 filter paper (nominal thickness, 180 μm; typical particle retention, 11 μm; material, cellulose) was purchased from GE Healthcare Life Sciences. Ultrapure water (18.2 MΩ cm−1 resistance) was provided by arium® pro Ultrapure Water Systems (Sartorius). Absolute ethanol and chloroform were procured from ChemSupply. Chemicals were used as received without further purification.
2.2 Fabrication of PDA/copolymer paper sensors
Depending on the solubility of the copolymer, PCDA or TCDA monomers were dissolved in ethanol or chloroform at a concentration of 20 mg mL−1. For mixtures containing PMMA15K, PEG4K, PEG1.5K, and PST35K, chloroform was used. The remaining copolymers such as PVP10K, PVP40K, PEG1K, PEG400, and PEG 200 were dissolved in ethanol. DA solution was sonicated for 1 min and filtered with a 0.45 μm polytetrafluoroethylene (PTFE) filter to remove large aggregates. Stock DA solution was mixed with copolymer solution and topped up with the same solvent to make a 200 mg mL−1 (20 w/v%) total solids solution. 3 μL was dropcasted onto a filter paper and air-dried for 10 min. The paper sensor was photopolymerized with UV (254 nm, UV lamp 420 μW cm−2, 6 W, Analtech®Adta) to generate blue PDA. The distance between the paper and the UV source was 5 cm. For control PDA (PCDA and TCDA only), 20 mM DA monomers were used, and similar synthesis methods were followed.
2.3 Performance screening (resistance to humidity)
It is imperative for PDA to be resistant to humid conditions as human breath is humid (40–90% relative humidity (%RH)).66 As an initial screening, all sensors were evaluated at 60% RH and 90% RH at typical breath temperature of 35 °C, and the sensor is unsuitable if a red PDA is formed. Paper sensors were incubated in the dark for 30 min (Thermoline TCU-4-THR).
2.4 VOC testing
2.4.1 VOC dosing (saturated condition).
For saturated condition testing, sensor was incubated in a 250-mL Schott bottle. The bottles were pre-conditioned to a set temperature and relative humidity (Thermoline TCU-4-THR) for 15 min and sealed. Then, a pre-determined amount of solvent was injected. The PDA/copolymer paper sensors were exposed to gaseous VOCs for 30 min (Ratek OM11). After that, the paper sensor was scanned on a flatbed scanner (Epson Perfection V39). For dosing, the ideal gas equation (eqn (1)) and Antoine's semi-empirical equation (eqn (2)) were used. Antoine's equation can be used to correlate test temperature and absolute vapor pressure of pure substances and model the saturation pressures of liquids.where P is pressure (kPa), V is volume occupied by gas (L), n is the amount of gas in moles (mol), T is temperature (K), and R is the gas constant (8.314 kPa L K−1 mol−1). | 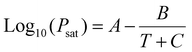 | (2) |
where Psat is saturation pressure (mmHg), T is temperature (°C) and A, B, C are constant values from Lange's Handbook of Chemistry67 or NIST Chemistry Webbook.68 Eight VOCs including ethylbenzene, 2-ethyl-1-hexanol, 2-butanone, hexanal, undecane, acetone, ethanol, and isoprene were evaluated.
2.4.2 Sensitivity testing.
Sensors that turned red under saturated conditions were further tested to determine their limit of detection (LOD). The experimental setup is shown in Fig. S2 (ESI†). A custom-built reactor was first pre-conditioned to 35 °C and 60% RH. Water dosing was done by injecting water from the bottom inlet and a heat plate was used to monitor the temperature. Once the desired temperature and RH were read from the portable probe (Testo 440 Humidity kit), gaseous VOCs were dosed from 25 to 2500 parts per million by volume (ppmv) using a bubbler filled with the VOC of interest. Depending on the VOC of interest, the bubbler may be heated to volatilize the solvent. Nitrogen (N2) was used as a carrier gas. The dosing was done by monitoring the reading on the portable photoionization (PID) detector (MiniRAE 3000), adjusting the VOC inlet and N2 gas inlet. All sensors were incubated for 15 min, and the temperature, RH and VOC concentration were kept consistent throughout. Paper sensors were suspended near the VOC detector and temperature and RH probe to ensure accurate conditions on the paper. Pressure monitoring was done using Digitron 2000P.
2.5 Colorimetric analysis
2.5.1 Colorimetric data collection and processing.
Digital colorimetric analysis using different color spaces, including RGB (red, green, blue), HSV (hue, saturation, value), or CIE LAB (lightness, red/green, blue/yellow) were used in different stages in this study to leverage the benefits of each space. RGB color space is widely used as it is simple, widely supported and easy to extract from software such as ImageJ and Adobe Illustrator/Photoshop.37,51,69 However, when comparing hue changes, RGB is not suitable because it is nonlinear and has a discontinuous space. In this case, the hue factor in the HSV color space is more suitable.70 PDA color transitions from blue to purple then red. As such, hue changes before and after analyte exposure were done using HSV in this study. H values of 180–240 is blue, 240–300 is purple, and 300–360 is red. The third color space, LAB, is beneficial in color analysis as its space is perceptually uniform.71 LAB was used to compute the Euclidian distance (ΔE),72 which measures how perceptually different PDA's color change is before and after analyte exposure. ΔE was calculated using eqn (3), where L2, a2, b2 are the LAB values after analyte exposure, and L1, a1, b1 are the LAB values before exposure. To determine PDA's blue intensity after photopolymerization, the initial LAB values are from a white filter paper. A greater ΔE value means the more visible the color difference is. Each person has a different color perception ability. However, the minimum ΔE value that can be perceived through detailed observation is 1–2, whereas ΔE ≥ 10 is perceptive to most observers. |  | (3) |
Paper sensor was scanned on a flatbed scanner and Trigit, a rapid colorimetric sensor reader web app that we developed,70 was used to extract the colorimetric data.
2.5.2 Principal component analysis (PCA).
Principal component analysis (PCA) was used to evaluate the PDA/copolymer array's ability to differentiate VOCs. PCA was selected due to its ability to reduce the dimensionality of a highly complex dataset without eliminating the crucial patterns and data variability.73,74 A single PDA/copolymer sensor has 3 colorimetric data (R, G, and B values). The distinguishing capability was done by simultaneously evaluating the 9 PDA/copolymer and 2 PDA sensors (3 color values × 11 PDAs × 3 repeats) across 8 different VOCs. PCA was run in BioVinci (by Bio Turing).
3. Results and discussion
3.1 PDA/copolymer synthesis and optimization
PMMA, PVP, PST, and PEG were selected as copolymers for PDA sensors based on how close their Hildebrand solubility parameters (δ) are to the target VOCs (Table S1 ESI†), where the smaller the difference, the more soluble the polymer is in the VOC. We first investigated the feasibility of fabricating PDA/copolymer sensors by varying the PDA-to-copolymer weight ratios (w/w) and evaluating the formation of blue PDA in the composites upon polymerization. The aim of this step is to determine the UV-polymerization time. The cut-off UV time was selected based on the ΔE value before and after UV exposure. A ΔE value of >10 indicates that the blue intensity is not pale and can be perceived via the naked eye. Three PDA-to-copolymer weight ratios were synthesized (1
:
25, 1
:
50, 1
:
100) and UV-polymerized up to 20 s (5 s interval). Two DA monomers, including PCDA (C25) and TCDA (C23) were used. Nine copolymers, including PMMA15K, PVP40K, PVP10K, PST35K, PEG4K, PEG1.5K, PEG1K, PEG400, and PEG200 were investigated. The total solids content is set at 200 mg mL−1 (20 w/v%) to mimic the composition of commercial inks for printing.75,76
Fig. S3 (ESI†) depicts the blue intensity of the PDA/copolymers and the corresponding ΔE values. More PDA/copolymer composites were formed using the longer chain PCDA (C25) (8 out of 9 copolymers tested) as opposed to TCDA (C23) (5 out of 9 copolymers tested) (Fig. S3a and b ESI†). For PCDA mixtures (Fig. S3a ESI†), blue PDA/PMMA15K was formed at PCDA
:
PMMA15K ratio of 1
:
25 w/w, however the color is pale (ΔE < 10) with noticeable coffee-ring effects. Blue PDA/PVP40K and PDA/PVP10K could be formed at ratios of 1
:
25 w/w and 1
:
50 w/w. At high copolymer content (1
:
100 w/w), no blue PDA was formed in both PVP40K and PVP10K, indicating that the intercalation of concentrated PVP chains inhibited the rearrangement of DA molecules for efficient photopolymerization. The hindered segmental movement of DA in the presence of PVP has previously been reported.77 PDA/PEG could be formed using both PCDA or TCDA monomers. In fact, when TCDA was used as a monomer (Fig. S3b ESI†), only PEG-containing PDA could be polymerized. Increasing PEG molecular weight in the mixtures produced higher ΔE values when polymerized for the same time (Fig. S3c and d ESI†).
PDA/PST films have been successfully synthesized in previous studies.78,79 However, blue PDA did not form in any of the evaluated PST composites across all tested concentrations. This is due to the combined effects of both the bulky PST benzene ring and the high PST concentration that interfered with the rearrangement of DA monomers for effective polymerization.80,81 In prior work, the DA concentration was 8 to 15 times higher compared to PST. In contrast, the DA-to-PST weight ratio in this study ranged from 1
:
25 to 1
:
100 w/w. PDA's polymerization ability is governed by its packing efficiency. Photopolymerization of DA is only possible when the distance between the two adjacent monomers is 4.7–5.2 Å and when the angle of the monomer and the stacking axis is about 45°.40,82 Pure PCDA or TCDA is polymerizable, however, upon the addition of copolymers, the shorter chain TCDA lacks amphiphilicity that is essential to promote self-assembly due to the denser amount of copolymers in the surrounding. Overall, 5-second photopolymerization is sufficient for most mixtures (ΔE > 10), and some require 10 seconds of UV exposure. The selected PDA/copolymer formulations and photopolymerization times to be used for the subsequent tests are marked with red borders in Fig. S3a and b (ESI†).
3.2 Screening tests
Following the optimization above, PDA/copolymers were subjected to standard breath temperature and RH (35 °C and 60% RH or 90% RH) in the absence of VOCs to determine their suitability as breath sensors. To be fit for breath sensors, PDA color after standard breath temperature and RH exposure must remain blue (H value between 180–240), and no significant change in its blue shade should be immediately perceivable (ΔE < 10) in both conditions. In its pure form (without copolymer), PDA made from both PCDA and TCDA are resistant to standard breath temperature and RH conditions (Fig. S4a ESI†). However, when PEG was introduced, exposure to humidity increased the ΔE value (Fig. S4b–e ESI†). Sensitivity to moisture was greater in longer chain PEG and higher copolymer contents. This is because PEG is a water soluble and hydrophilic molecule, and its hydrophilicity increases with higher MW.83 Moisture sensitivity due to PEG addition is particularly evident in shorter chain monomer (TCDA), and a higher copolymer weight ratio (>1
:
25 w/w) yielded a more perceivable color change. The molecular structural integrity contributing to the blue-PDA is dependent on the balance of three dominating forces, including hydrogen bonding on the -COOH head group, dispersion force along the alkyl tail, and π–π stacking along the conjugated backbone. PCDA and TCDA have the same carboxylic head chain length, but TCDA has a shorter alkyl tail and hence, weaker dispersion interactions. This caused PDA to shift from its trans (blue) to cis (purple/red) conformation. Overall, PDA/copolymer sensors that satisfied the criteria are marked with red borders (Fig. S4b and c ESI†) and selected for the VOC exposure testing.
3.3 Responses to VOC biomarkers
Based on the screening tests mentioned above, the selected 9 PDA/copolymer combinations with its photopolymerization time are PCDA/PVP40K (1
:
50, 5 s), PCDA/PVP10K (1
:
50, 10 s), PCDA/PEG1.5K (1
:
25, 5 s), PCDA/PEG1K (1
:
25, 5s), PCDA/PEG400 (1
:
25, 5 s), PCDA/PEG200 (1
:
50, 5 s), TCDA/PEG1K (1
:
25, 5s), TCDA/PEG400 (1
:
25, 5 s), and TCDA/PEG200 (1
:
25, 5 s). These 9 PDA/copolymers were incubated with the target VOCs (ethylbenzene, 2-ethylhexanol, hexanal, 2-butanone, and undecane) and breath interferents (ethanol, acetone, and isoprene) in the standard breath temperature and relative humidity conditions for 30 min. PDAs made from pure PCDA and TCDA were also tested as controls. Fig. 1a shows that the 11 PDAs generated distinctive patterns that could distinguish the VOCs.
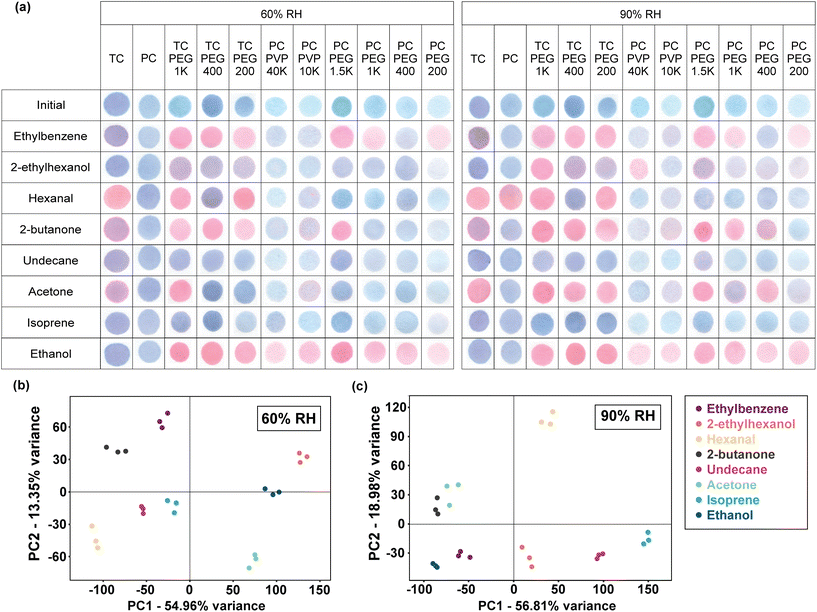 |
| Fig. 1 (a) Unique color responses of PDA and PDA/copolymer paper sensors when individually exposed to 8 different VOCs (saturated) under standard breath temperature and relative humidity (35 °C and 60% RH or 90% RH). PCA score plots of the RGB values of the PDA sensor array after exposure to gaseous VOCs at (b) 60% RH and (c) 90% RH. 792 color values (8 VOCs × 11 PDAs × 3 color values × 3 replicates) from each relative humidity were simultaneously analyzed to evaluate the VOC distinguishing ability of the PDA arrays. | |
PCA was applied to achieve a more visualized comprehensive result of the cross-responsive detection. 792 colorimetric data were simultaneously analyzed using PCA (8 VOCs × 11 PDAs × 3 color values × 3 repeats), which is a statistical technique that can be used to linearly reduce highly dimensional data by generating a new coordinate based on the original dataset. The new coordinate axes are the principal component (PC) scores. The importance of a PC score in explaining the original data set's variance reduces with an increasing PC number. For example, the first PC (PC1) explains the maximum variance of the data, followed by PC2, and so on. Linear dimensionality reduction was done using Singular Value Decomposition (SVD). Fig. 1b and c demonstrates that the 11-array PDA sensors yielded separate clusters which indicate that they could differentiate the 8 VOCs. Examining the PCA plot, the first two principal components (PC1 and PC2 scores) of the array when tested at 60% RH account for 54.96% and 13.35% of the variance, respectively (Fig. 1b). At 90% RH, PC1 is 56.81% and PC2 is 18.98% (Fig. 1c). Greater discriminating capability is achieved in the 60% RH data as shown by the segregated clusters when compared to the 90% RH clusters. However, the two ketones (acetone and 2-butanone) clusters were relatively close to each other when tested at 90% RH, which means they may be slightly hard to distinguish.
LC breath VOCs are still in the discovery phase with limited information on the confirmed biomarkers. VOCs exist in trace amounts in breath and as there is no standardized breath sampling method, the reported biomarkers in studies are highly diverse. Thus, the 5 VOC biomarkers in this work were selected by carefully evaluating prior studies that used analytical techniques (i.e.: GC, PTR, SIFT) to identify potential lung cancer breath biomarkers. Only studies that used human breath samples were evaluated as cancer cells cultured in vitro and animal cells are not representative of human cancer microenvironment, which consequently varies the amount and type of VOCs generated. Furthermore, plastic culture vessels emit alkanes and aromatics, and culture media release background VOCs, which may lead to erroneous conclusions if uncorrected.84,85 The 5 biomarkers selected in this study are ethylbenzene, 2-butanone, 2-ethylhexanol, hexanal, and undecane. Most of these VOCs were selected primarily due to their correlation with smoking history, as cigarette smoking is a well-known risk factor for developing LC and is estimated to account for >70% of LC risk annually.86
Ethylbenzene is important as its elevated levels in LC patients, particularly among smokers, have been consistently reported.57,60,87–89 For example, ethylbenzene concentrations are 88% higher in lung cancer patients compared to healthy non-smokers.60 Ethylbenzene can also distinguish non-small cell lung cancer (NSCLC) and chronic obstructive pulmonary disease (COPD) patients and non-smokers.54 Hexanal is absent in healthy populations,60 but is 3–4 times higher in smokers or ex-smokers. Hexanal also has a high sensitivity (74–100%)16,58 and specificity (72–100%)16,60 to LC. 2-Ethylhexanol is also a prominent LC biomarker, with elevated levels in smokers.56–58 In contrast, 2-butanone is not present in healthy passive smokers,60 but can identify benign nodules and differentiate stage I to stage II–IV NSCLC.57,59–63 Finally, undecane is 80–100% sensitive and 81% specific to LC, although it may coexist with the other two common cancers among smokers which are pharyngeal and oral cancers.16,55,57 Detailed VOC concentrations for healthy individuals and LC patients, along with sensitivity and specificity, are provided in Table S3 (ESI†).
Fig. 2 plots the ΔE and hue values of each PDA and PDA/copolymer in Fig. 1a when exposed to different saturated VOCs at 60% RH and 90% RH. The cut-off ΔE value, which determines when color change can be perceived by the naked eye, is marked by the magenta dashed line. The blue, purple, and red regions indicate the hue values where PDA is of that color. For ease of comparison, the direction of ΔE's change (increase or decrease) of PDA before copolymer addition is presented as categorical data in Table S4 (ESI†). The reference values used are those of Fig. 2. For example, PCDA/PVP10K showed higher ΔE compared to PCDA alone after 2-butanone exposure at 60% RH and is thus marked ^.
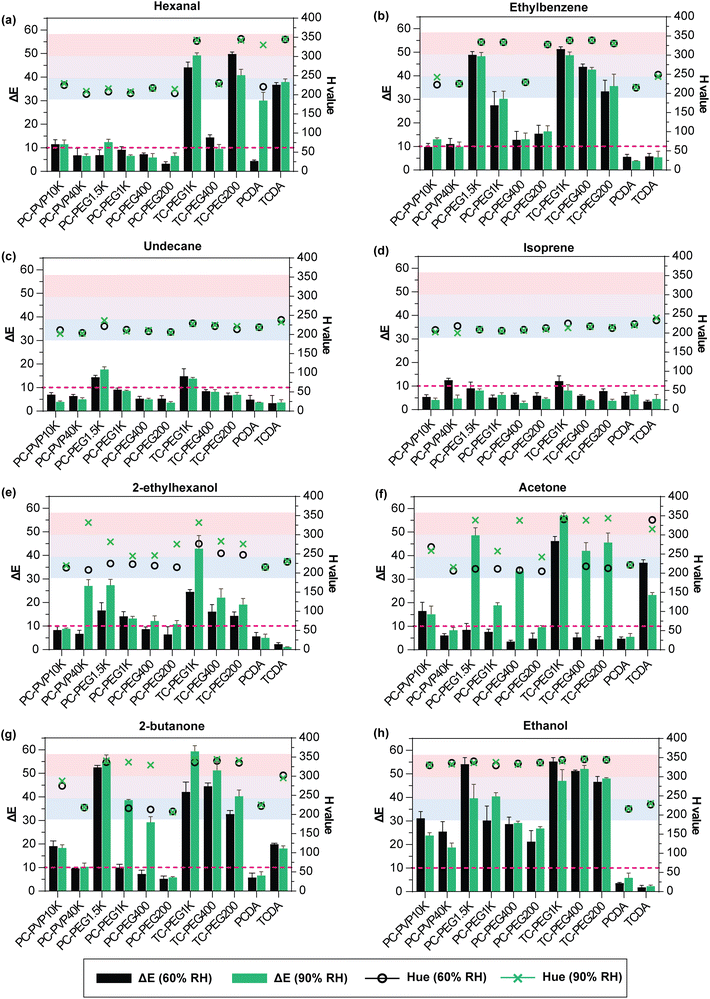 |
| Fig. 2 ΔE and hue values of PDA and PDA/copolymers after 30 minutes of exposure to (a) hexanal, (b) ethylbenzene, (c) undecane, (d) isoprene, (e) 2-ethylhexanol, (f) acetone, (g) 2-butanone, and (h) ethanol at standard breath and relative humidity conditions (35 °C and 60% RH or 90% RH). The blue, purple and red sections highlight the H value where PDA is of that color. The dashed magenta line at ΔE = 10 marks the value when color change can be observed via the naked eye. n = 3, error bars represent standard deviation. | |
Table S4 (ESI†) showed that in most cases, the copolymer addition increased PDA's VOC sensitivity. The change in copolymer type and MW influenced the intercalation process with PDA layers, which altered its chromic responses. PDA's blue-to-red color change mechanism is highly debated. However, the most cited cause is the twisting of the conjugated backbone, which disrupts the p-orbital alignment along the molecule.41 In its blue phase, PDA is planar in shape and its p-orbitals efficiently overlap. The supramolecular structure is held together by three interactions, including hydrogen bonding along the –COOH head group, the π–π interaction within the conjugated backbone, and the dispersion forces along the alkyl tail.40 PDA is amphiphilic and contains both polar and non-polar properties in opposite ends. When an analyte is introduced and causes an imbalance among the 3 forces, the highly ordered structure collapses and the p-orbital overlap is disrupted, causing a shift in light absorbance. Consequently, blue PDA turns into a red PDA.
As previously mentioned in the introduction, incorporating support matrix into PDA is one way to tune its sensitivity and selectivity.47,52Fig. 2 shows that higher MW PEG copolymer yielded a stronger response, whilst the reverse was observed when PVP was added. This is due to the more efficient PEG arrangement into the PDA structure that resulted in greater PDA accessibility to react with the VOCs. Although both PEG and PVP are amphiphilic which enable them to form complexes with PDA via hydrogen bonding, their structural difference (Fig. S1†) alters the location for hydrogen bonding. For example, hydrogen bonding in PEG monomer occurs along its hydroxyl (–OH) group ends, whereas in PVP, hydrogen bonding is through the lone-pair electrons from the nitrogen atom within its repeating N-vinylpyrrolidone units. Additionally, the formation of a thicker PVP layer with increasing MW has previously been reported.90,91 The lower packing efficiency of PVP with PDA and the increasing layer thickness when MW is higher lead to the isolation of the PDAs from the analyte VOCs. Consequently, PDA/PVP reactivity is lower compared to PDA/PEG combinations.
An anomaly was present in the PCDA-containing mixtures after hexanal exposure (Fig. 2a). Increased responses were found when tested at 60% RH after copolymer incorporation. However, the reverse occurred when tested at 90% RH because the control PCDA itself was already red after hexanal exposure (90% RH) and thus, further ΔE is likely not possible. Among the 9 combinations produced, only TCDA/PEG1K and TCDA/PEG200 (Fig. 2a) improved sensitivity to hexanal. The suppressed response to hexanal after copolymer addition was not predicted as hexanal's terminal carbonyl group is usually highly reactive. One possible explanation is that the copolymer addition created a dense layer surrounding the PDA which inhibited hexanal from reacting with the PDA to cause the conformational change along the backbone. When comparing the VOC response of PDA/copolymer mixtures with the controls (PCDA and TCDA only), the highest ΔE increase was observed in the TCDA-containing mixtures. This indicates that TCDA monomer chain lengths along its head group and alkyl tail are favorable for the efficient intercalation of the PVP and PEG. A similar pattern was observed when exposed to ethylbenzene (Fig. 2b). Comparing PCDA-PEG1K and TCDA-PEG1K, TCDA showed 2× higher ΔE compared to PCDA. Ethylbenzene is a monocyclic aromatic hydrocarbon with an ethyl substituent. This ethyl group imparts a small dipole moment which gives ethylbenzene its slight polarity. Herein, the reaction between PDA and ethylbenzene occurred as the ethyl group orients itself into the PDA structure. Ethylbenzene's reactivity with PDA is driven by the van der Waals forces along the alkyl tail and conjugated backbone of the PDA.92 These interactions can disrupt the balance of the three dominating forces within the PDA, leading to a collapse of its structure and a consequent blue-to-red color change.
Reduced sensitivity and selectivity in high humidity conditions is a common problem in sensor technologies due to the competitive binding or adsorption of the water molecules to the binding sites.93,94 Hence, it is imperative to evaluate the effects of relative humidity on PDA's responses. Fig. 2 shows that the colorimetric responses of PDA/copolymers to ethylbenzene (Fig. 2b), undecane (Fig. 2c), and isoprene (Fig. 2d) were not significantly impacted by humidity. On the other hand, humidity-dependent responses were evident when exposed to 2-ethylhexanol (Fig. 2e), acetone (Fig. 2f), and 2-butanone (Fig. 2g). Greater ΔE was found in 9 out of 11 PDAs tested after acetone exposure at 90% RH compared to 60% RH (Fig. 2f). From the same figure, PEG addition increased PCDA and TCDA's response to acetone by 2–9 times and >2 times when tested at 90% RH, respectively. However, when tested at 60% RH, minimal responses were observed, except for when PEG1K was incorporated into TCDA monomer. Increased response to ketones in high humidity is due to the solubility and increased hydrogen bonding with the hydrophilic headgroup of PDA.95Fig. 2f also showed that the addition of PVP approximately doubled PCDA's acetone response, and that greater increase was present in the shorter PVP chain (PVP10K). Lower response in longer PVP chain is likely due to the more rigid PVP crystals and thicker PVP layer which inhibited VOC's accessibility to react with PDA. Improved PDA performance upon the incorporation of low MW PVP (MW = 10
000 Da) was previously reported by Kamphan et al.77
The highest colorimetric response was observed upon ethanol exposure (Fig. 2h), where 9 out of 11 red PDAs were generated. As the controls remained blue in both humidities, the color change was therefore attributed to the addition of the copolymers (PVP and PEG). In contrast, insignificant responses were observed in all PDA composites upon exposure to undecane and isoprene (Fig. 2c and d). The poor response to non-polar and unreactive undecane is as expected as its chain is saturated. No color change was observed when PDAs were exposed to isoprene (Fig. 2d) as isoprene has poor solubility in both PVP and PEG as reflected in the difference in the Hildebrand parameter values (Δδhild). The Hildebrand parameter (δhild) of polymers and organic solvents (Table S1 ESI†) estimates the degree of interaction by accounting the dispersion (δd), polar (δp), and hydrogen (δh) bonds in the molecule.96 Materials with smaller Δδhild indicate greater solubility and miscibility. The Δδhild of isoprene is among the highest compared to the other VOC tested in this study (Table S2 ESI†). For undecane, the Δδhild are 6.1 (PEG) and 7.6 (PVP). On the other hand, isoprene Δδhild are 5.6 (PEG) and 7.1 (PVP). It is worth noting that this poor response is beneficial as isoprene is one of the major breath VOCs (ppmv range) and is a breath interferent. Isoprene is not suitable as a breath biomarker as its concentration is highly dependent on physical activity, age, sampling time, diet, etc.97–101 Overall, the impacted humidity response means that this must be accounted for when testing in real breath samples. For example, by integrating humidity sensor and cross-referencing colorimetric responses based on the humidity readout.
3.4 VOC sensitivity test
For PDAs that turned fully red after VOC exposure in both relative humidities (Fig. 1), we tested their sensitivity to determine their detection limit. To do this testing, we developed a custom-built reactor (Fig. S2 ESI†) to enable controlled dosing of VOC while maintaining standard breath and relative humidity conditions. Herein, PDA paper sensors were suspended using a stainless-steel hook. Immediately adjacent is a temperature and relative humidity probe to ensure that the exposed conditions are consistent with the readout. This is imperative because despite the reactor being a closed system, a temperature and relative humidity gradient inevitably occur in the chamber. Pressure indicator was equipped to maintain atmospheric conditions during dosing. The chamber is heated using a hot plate at the base and water is dosed by injecting pure water. A PID detector capable of quantifying VOCs was included for accurate dosing and VOC was dosed by bubbling liquid solvent with nitrogen. The bubbler may be immersed in a heated water bath to assist VOC vaporization. Of note, no commercial detectors to date can detect all 8 VOCs under standard breath relative humidity and temperature, and the PID detector used in this study is one of the best currently available. Therefore, after accounting both the sensors’ responses in saturated conditions and the PID's capability, the 3 VOCs further evaluated include ethylbenzene, 2-butanone, and ethanol.
Referring to Fig. 1, the 6 PDAs that were tested against ethylbenzene were TCDA/PEG1K, TCDA/PEG400, TCDA/PEG200, PCDA/PEG1.5K, PCDA/PEG1K, and PCDA/PEG200. For 2-butanone, 4 PDAs were evaluated including TCDA/PEG1K, TCDA/PEG400, TCDA/PEG200, and PCDA/PEG1.5K. All 9 PDA/copolymer sensors, including TCDA/PEG1K, TCDA/PEG400, TCDA/PEG200, PCDA/PEG1.5K, PCDA/PEG1K, PCDA/PEG200, PCDA/PVP40K, and PCDA/PVP10K were responsive to ethanol. All these sensors were tested from 25–2500 ppmv VOC at 60% RH. Dosing at 90% RH was not done as significant condensation within the test chamber and severe concentration fluctuations occurred, rendering unreliable results. Additionally, all PDAs were incubated for 15 minutes as our end goal is to produce a rapid test, which is defined as tests with an average turnaround time of 5 to 15 minutes.102 Test time was kept consistent as PDA's response is concentration-time-dependent to avoid false readout.49
Results are shown in Fig. 3. In general, blue-to-red or purple color change increased in a logarithmic trend upon exposure to increasing VOC concentration. In all 3 VOCs tested, the steepest increase occurred between 25–100 ppmv. Among datasets with ΔE value of > 10 (i.e.: color change is visible via the naked eye), a regression line was fitted on the linear range as shown in Fig. 4. The linear range, regression value from the line of best fit (R2), and limit of detection (LOD) for all PDA/copolymer sensors are listed in Table 1. The LOD was calculated with LOD = 3σ/S, where σ is the standard deviation of the blank and S is the slope of the calibration curve.
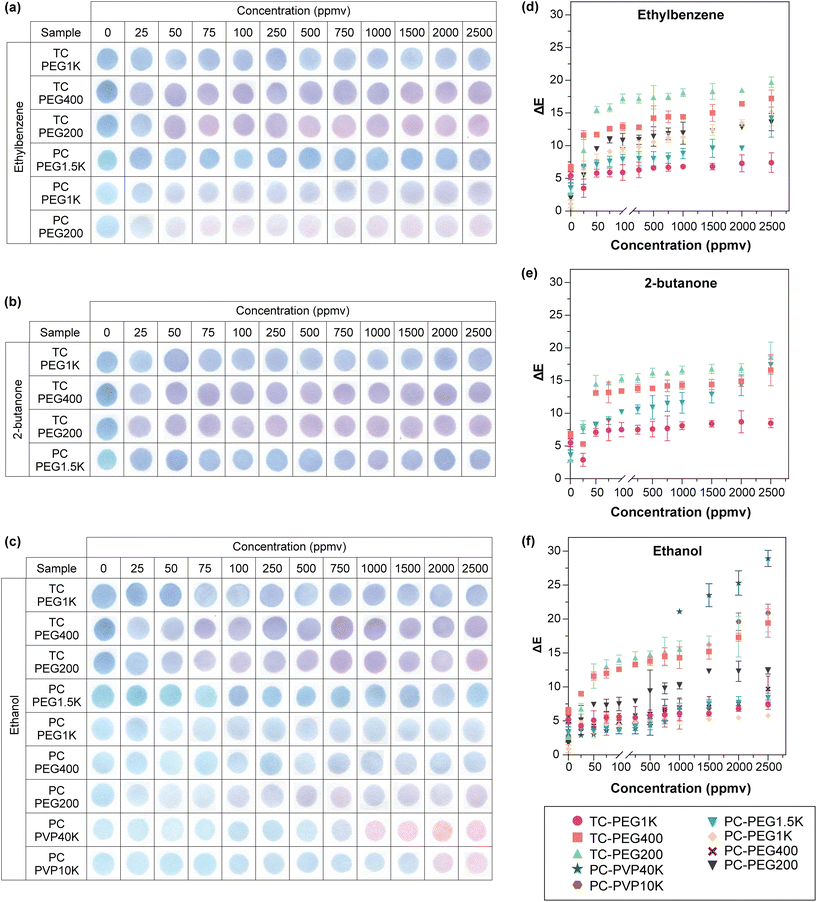 |
| Fig. 3 Colorimetric response of PDA/copolymer sensors after 15 minutes of exposure to (a) ethylbenzene, (b) 2-butanone, and (c) ethanol at various concentrations (25–2500 ppmv), and the corresponding ΔE values (d–f). All sensors were evaluated at 35 °C and 60% RH. n = 3, error bars represent standard deviation. | |
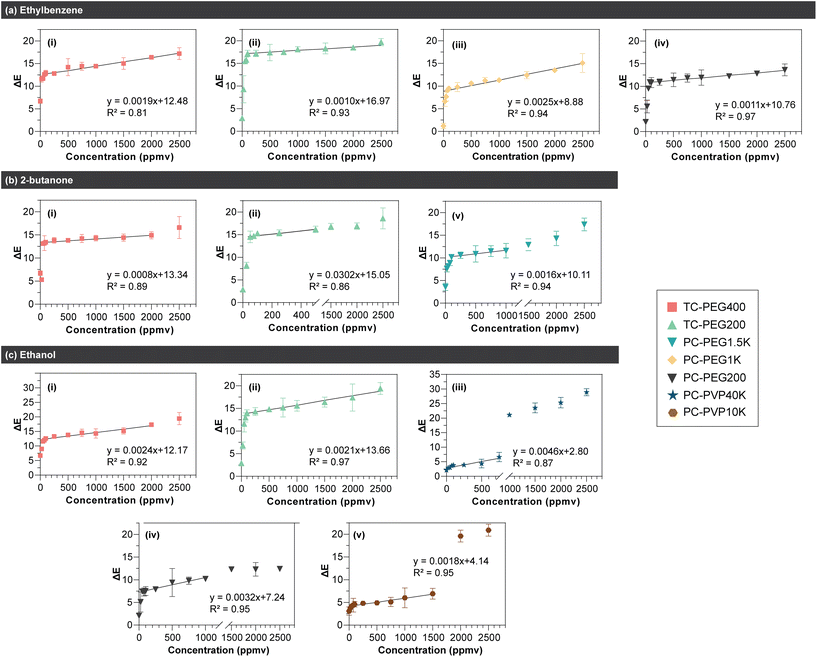 |
| Fig. 4 Regression line fitted on the linear region of PDA/copolymer sensors’ colorimetric response after 15 minutes of exposure to (a) ethylbenzene, (b) 2-butanone, and (c) ethanol. All sensors were evaluated at 35 °C and 60% RH. n = 3, error bars represent standard deviation. The linear range and the LOD values are listed in Table 1. | |
Table 1 The linear range and limit of detection (LOD) of each PDA/copolymer when exposed to ethylbenzene, 2-butanone, and ethanol for 15 minutes at 35 °C and 60% RH. Only datasets with ΔE value of >10 were fitted
Analyte |
PDA/copolymer |
R
2
|
Linear range (ppmv) |
LOD (ppmv) |
Ethylbenzene |
PCDA/PEG200 |
0.97 |
75–2500 |
457 |
PCDA/PEG1K |
0.94 |
50–2500 |
683 |
TCDA/PEG200 |
0.93 |
100–2500 |
771 |
TCDA/PEG400 |
0.81 |
50–1500 |
812 |
2-Butanone |
TCDA/PEG200 |
0.86 |
50–500 |
267 |
PCDA/PEG1.5K |
0.94 |
100–1000 |
320 |
TCDA/PEG400 |
0.89 |
50–2000 |
775 |
Ethanol |
PCDA/PEG200 |
0.95 |
50–1000 |
269 |
PCDA/PVP40K |
0.87 |
0–750 |
333 |
PCDA/PVP10K |
0.95 |
50–1500 |
354 |
TCDA/PEG200 |
0.97 |
100–2500 |
482 |
TCDA/PEG400 |
0.92 |
50–2000 |
644 |
From Table 1, the most suitable PDA/copolymer systems to detect the VOCs without any specialized equipment (i.e.: changes are visible by the naked eye for on-site detection) are PCDA/PEG200 (LOD = 457 ppmv) for ethylbenzene, TCDA/PEG200 (LOD = 267 ppmv) for 2-butanone, and PCDA/PEG200 (LOD = 269 ppmv) for ethanol. PDA/copolymer systems with the widest linear range are PCDA/PEG200 for ethylbenzene (75–2500 ppmv), TCDA/PEG400 (50–2000 ppmv) for butanone, and TCDA/PEG200 for ethanol (100–2500 ppmv). Table 1 shows that when the DA monomer is the same, lower copolymer MW resulted in lower LOD. This is in agreement with results from the previous section, where higher MW creates a thicker layer which isolated PDA from the VOC analytes. The formation of a thicker protective layer with increasing PEG MW is well known and has previously been reported.103–105 Although greater copolymer MW yielded higher LOD, it enabled a wider linear range. This is because color change is more subtle (i.e.: change in ΔE between each data point is less prominent) when a higher VOC concentration is dosed as observed in Fig. 3.
Of note, as these sensors are cross-responsive by nature, it is not possible to quantify VOC concentration in samples containing multiple VOCs without previously identifying them. For example, although PCDA/PEG200 is the best system to detect both ethanol and ethylbenzene, it is not possible to know the exact concentration without first knowing which of the two VOCs is present. Additionally, PCDA/PEG200 alone cannot quantify the concentration if both ethanol and ethylbenzene are present in the same system. In such cases where quantification is crucial, different PDA/copolymer systems must be used. Additionally, it is worth noting that a direct comparison of PDA sensor's LOD is difficult because of the inconsistent reported units between previous studies.41 Unit conversion is not possible due to incomplete test methods and because VOC gaseous concentration is temperature (T), relative humidity (RH), volume and pressure dependent. The standard unit as recommended by the EPA is parts-per-million by volume (ppmv) or by atom (ppma).106 Of all PDA studies in our latest review,41 only one study reported concentration in ppmv unit and these VOCs are none of those evaluated herein.41,46Table 2 compares the sensor's performance from this work to prior studies utilizing PDA for the same purpose. No other studies have used PDA to detect ethylbenzene and 2-butanone. Thus, no comparison is possible.
Table 2 Performance comparison with previous studies that used PDA to detect the same VOC. Only the best performing sensor in this work is listed
VOC |
Ref. |
Assay time |
LOD |
Ethanol |
This work |
15 min |
269 ppmv |
PDA/copolymer on paper |
5 min |
0.08% v/v (ref. 44) |
PDA/clay |
35 min |
0.08% v/v (ref. 43) |
PDA-coated electrodes |
100 s |
30 ppm (ref. 108) |
In this study, PDA/copolymer paper sensors were successfully synthesized using PEG and PVP copolymers. To the best of our knowledge, research on PDA/copolymers as paper sensors for VOC detection is still limited, with the only other notable work reported by Tu et al.44 In their study, copolymers included poly-4-vinylpyridine (P4VP, MW 60 kDa), polyacrylic acid (PAA, MW 160 kDa), PVP (MW 40 kDa), and PEG (MW 8 kDa).44 The best performance was observed with PVP and PEG composites, attributed to the solubility of the VOCs in these copolymers. PDA/copolymer composites as paper sensors are rarely studied, as in most cases, polymer matrices are used as inert scaffolds or supports to house PDA molecules. Typically, the resultant sensors are transparent films rather than in a paper format.46,107 Furthermore, although the selection of the copolymer should be driven by the solubility of the target analyte in the copolymer as indicated by the Hildebrand solubility parameters (δ), our work shows that PDA's polymerizability and sensitivity vary depending on the molecular weight of the copolymer. Therefore, it is essential to evaluate different molecular weights when selecting a polymer with a suitable δ value.
Overall, although the sensitivity of our PDA/copolymer systems needs further improvement before they could be used as breath sensors (relevant application range is in Table S3 ESI†), our results suggest that PDA system can be tuned to optimize its performance for gaseous VOC detection. Furthermore, the proposed PDA/copolymer system is attractive for VOC detection due to its simple synthesis, low cost, and easy to handle paper material compared to existing technologies such as electrochemical, optical assays, and chromatography. Recommendations for future studies include combining multiple copolymers with unmodified and chemically modified PDA to improve its sensing performance.
4. Conclusions
We have developed colorimetric PDA/copolymer composite paper sensors to detect and distinguish breath VOCs indicative of early lung cancer, including ethylbenzene, 2-butanone, hexanal, 2-ethylhexanol, and undecane in standard breath temperature and relative humidity (35 °C, 60% RH and 90% RH). Performance against common breath interferents, such as acetone, ethanol, and isoprene, was also evaluated. Copolymers such as PMMA, PVP, PST, and PEG were incorporated with PDA to facilitate color change based on their chemical affinity and solvating ability. PDA/copolymer system was synthesized by a simple drop-casting method, and a blue-to-red color transition upon reactions with gaseous VOCs was successfully shown. We found that the incorporation of PEG yielded better responses compared to PVP owing to its more efficient integration with PDA via terminal hydrogen bonding. In addition, lower PVP molecular weight is desired to prevent thick layer formation that inhibits PDA-to-VOC accessibility. The colorimetric data (R, G, B values) of the 11 tested PDAs and PDA/copolymers after saturated VOC exposure were simultaneously analyzed using PCA, demonstrating excellent discriminating capability. PDA/copolymers that generated red PDA in saturated VOC conditions were further tested. Sensitivity testing was conducted from 25 to 2500 ppmv VOC, and the LODs for the PDA/copolymer sensors are 457 ppmv for ethylbenzene, 267 ppmv for 2-butanone, and 269 ppmv for ethanol. We found that although higher copolymer molecular weight resulted in higher LOD, it enabled a wider linear range for detection. Thus, the selection of suitable PDA/copolymer sensors should depend on its final application. Our work presents the first study showcasing PDA's potential to detect breath VOC biomarkers. More specifically, it is the first PDA-based system to detect ethylbenzene and 2-butanone VOCs. Further studies to improve sensitivity limits by incorporating chemically modified PDAs and integrating these into different copolymers are recommended.
Author contributions
A. D. T. conceived idea, designed research, developed methodologies, performed experiments, analyzed the data, and wrote and revised the paper. R. C. revised the paper and supervised the project.
Data availability
The authors declare that the data supporting the findings of this study are available within the paper and its ESI.†
Conflicts of interest
The authors declare no conflict of interest.
Acknowledgements
A. D. T. acknowledges the support from the University of New South Wales Scientia PhD Scholarship. R. C. acknowledges the support from the National Health and Medical Research Council Emerging Leadership Investigator Grant (NHMRC APP1173428) and the UNSW Scientia Fellowship.
References
- World Health Organization, Lung Cancer, https://www.who.int/news-room/fact-sheets/detail/lung-cancer, (accessed 1 February 2024).
- J. Huang, Y. Deng, M. S. Tin, V. Lok, C. H. Ngai, L. Zhang, D. E. Lucero-Prisno, W. Xu, Z.-J. Zheng, E. Elcarte, M. Withers and M. C. S. Wong, Chest, 2022, 161, 1101–1111 CrossRef PubMed
.
- R. Sharma, Int. J. Clin. Oncol., 2022, 27, 665–675 CrossRef PubMed
.
- American Cancer Society, Key Statistics for Lung Cancer, https://www.cancer.org/cancer/types/lung-cancer/about/key-statistics.html, (accessed 1 February 2024).
- S. B. Knight, P. A. Crosbie, H. Balata, J. Chudziak, T. Hussell and C. Dive, Open Biol., 2017, 7, 170070 CrossRef PubMed
.
- S. Quadrelli, G. Lyons, H. Colt, D. Chimondeguy and A. Buero, Int. J. Surg. Oncol., 2015, 2015, 287604–287604 CAS
.
- D. E. Jonas, D. S. Reuland, S. M. Reddy, M. Nagle, S. D. Clark, R. P. Weber, C. Enyioha, T. L. Malo, A. T. Brenner, C. Armstrong, M. Coker-Schwimmer, J. C. Middleton, C. Voisin and R. P. Harris, J. Am. Med. Assoc., 2021, 325, 971–987 CrossRef PubMed
.
- CDC Centers for Disease Control and Prevention, Screening for Lung Cancer, https://www.cdc.gov/lung-cancer/screening, (accessed 1 February 2024).
- C. S. Pramesh, R. A. Badwe, N. Bhoo-Pathy, C. M. Booth, G. Chinnaswamy, A. J. Dare, V. P. de Andrade, D. J. Hunter, S. Gopal, M. Gospodarowicz, S. Gunasekera, A. Ilbawi, S. Kapambwe, P. Kingham, T. Kutluk, N. Lamichhane, M. Mutebi, J. Orem, G. Parham, P. Ranganathan, M. Sengar, R. Sullivan, S. Swaminathan, I. F. Tannock, V. Tomar, V. Vanderpuye, C. Varghese and E. Weiderpass, Nat. Med., 2022, 28, 649–657 CrossRef CAS PubMed
.
- B. Lubuzo, T. Ginindza and K. Hlongwana, Pan Afr. Med. J., 2020, 35, 38 Search PubMed
.
- L. Pauling, A. B. Robinson, R. Teranishi and P. Cary, Proc. Natl. Acad. Sci. U. S. A., 1971, 68, 2374–2376 CrossRef CAS PubMed
.
- K. M. Paschke, A. Mashir and R. A. Dweik, F1000 Med. Rep., 2010, 2, 56–56 Search PubMed
.
- M. K. Nakhleh, H. Amal, R. Jeries, Y. Y. Broza, M. Aboud, A. Gharra, H. Ivgi, S. Khatib, S. Badarneh, L. Har-Shai, L. Glass-Marmor, I. Lejbkowicz, A. Miller, S. Badarny, R. Winer, J. Finberg, S. Cohen-Kaminsky, F. Perros, D. Montani, B. Girerd, G. Garcia, G. Simonneau, F. Nakhoul, S. Baram, R. Salim, M. Hakim, M. Gruber, O. Ronen, T. Marshak, I. Doweck, O. Nativ, Z. Bahouth, D.-y. Shi, W. Zhang, Q.-l. Hua, Y.-y. Pan, L. Tao, H. Liu, A. Karban, E. Koifman, T. Rainis, R. Skapars, A. Sivins, G. Ancans, I. Liepniece-Karele, I. Kikuste, I. Lasina, I. Tolmanis, D. Johnson, S. Z. Millstone, J. Fulton, J. W. Wells, L. H. Wilf, M. Humbert, M. Leja, N. Peled and H. Haick, ACS Nano, 2017, 11, 112–125 CrossRef CAS PubMed
.
- M. Phillips, N. Altorki, J. Austin, R. Cameron, R. Cataneo, J. Greenberg, R. Kloss, R. Maxfield, M. I. Munawar, H. Pass, A. Rashid, W. Rom and P. Schmitt, Cancer Biomarkers, 2007, 3, 95–109 CAS
.
- M. Phillips, R. N. Cataneo, A. R. C. Cummin, A. J. Gagliardi, K. Gleeson, J. Greenberg, R. A. Maxfield and W. N. Rom, Chest, 2003, 123, 2115–2123 CrossRef CAS PubMed
.
- M. Phillips, K. Gleeson, J. M. B. Hughes, J. Greenberg, R. N. Cataneo, L. Baker and W. P. McVay, Lancet, 1999, 353, 1930–1933 CrossRef CAS PubMed
.
- M. Phillips, Sci. Am., 1992, 267, 74–79 CrossRef CAS PubMed
.
- A. Amann, B. d. L. Costello, W. Miekisch, J. Schubert, B. Buszewski, J. Pleil, N. Ratcliffe and T. Risby, J. Breath Res., 2014, 8, 034001 CrossRef CAS PubMed
.
- M. Shirasu and K. Touhara, J. Biochem., 2011, 150, 257–266 CrossRef CAS PubMed
.
- R. A. Hand, S. Efstathiou, A. M. Wemyss, M. Grypioti, G. Kirby, T. Barlow, E. C. Tinley, J. Ford, A. Jamieson, J. Reynolds, J. Miller, S. Bates, E. Khoshdel and D. M. Haddleton, RSC Appl. Polym., 2024, 2, 98–104 RSC
.
- S. Das, S. Pal and M. Mitra, J. Med. Biol. Eng., 2016, 36, 605–624 CrossRef PubMed
.
- Z. Jia, A. Patra, V. K. Kutty and T. Venkatesan, Metabolites, 2019, 9, 52 CrossRef CAS PubMed
.
-
T. Rinken and K. Kivirand, Biosensing Technologies for the Detection of Pathogens: A Prospective Way for Rapid Analysis, IntechOpen, 2018 Search PubMed
.
- N. Peled, M. Abud-Hawa, O. Liran, M. Ilouze, N. Gai-Mor, D. Shlomi, A. Ben-Nun, A. Onn, J. Bar, D. Johnson, J. Wells, S. Millstone, P. A. Bunn, Y. E. Miller, R. L. Keith, B. Rikke, F. R. Hirsch and H. Haick, J. Clin. Oncol., 2014, 32, 7560–7560 Search PubMed
.
- J. Pereira, P. Porto-Figueira, C. Cavaco, K. Taunk, S. Rapole, R. Dhakne, H. Nagarajaram and J. S. Câmara, Metabolites, 2015, 5, 3–55 CrossRef PubMed
.
- G. Schomburg, J. High Resolut. Chromatogr., 1979, 2, 461–474 CrossRef CAS
.
- A.-S. Lehnert, T. Behrendt, A. Ruecker, G. Pohnert and S. E. Trumbore, Atmos. Meas. Tech. Discuss., 2019, 1–17 Search PubMed
.
- J. King, P. Mochalski, A. Kupferthaler, K. Unterkofler, H. Koc, W. Filipiak, S. Teschl, H. Hinterhuber and A. Amann, Physiol. Meas., 2010, 31, 1169–1184 CrossRef CAS PubMed
.
- B. Henderson, A. Khodabakhsh, M. Metsälä, I. Ventrillard, F. M. Schmidt, D. Romanini, G. A. D. Ritchie, S. te Lintel Hekkert, R. Briot, T. Risby, N. Marczin, F. J. M. Harren and S. M. Cristescu, Appl. Phys. B: Lasers Opt., 2018, 124, 161 CrossRef PubMed
.
- G. Peng, U. Tisch, O. Adams, M. Hakim, N. Shehada, Y. Y. Broza, S. Billan, R. Abdah-Bortnyak, A. Kuten and H. Haick, Nat. Nanotechnol., 2009, 4, 669–673 CrossRef CAS PubMed
.
-
G. S. Andre, M. Joshua, Y. Walter and M. P. Edmund, Proc. SPIE 4575, Chemical and Biological Early Warning Monitoring for Water, Food, and Ground, 2002, p. 4575 Search PubMed
.
- P. J. Mazzone, X.-F. Wang, S. Lim, H. Choi, J. Jett, A. Vachani, Q. Zhang, M. Beukemann, M. Seeley, R. Martino and P. Rhodes, BMC Cancer, 2015, 15, 1001 CrossRef PubMed
.
- M. O. Kim, M. Q. Khan, A. Ullah, N. P. Duy, C. Zhu, J.-S. Lee and I. S. Kim, Mater. Res. Express, 2019, 6, 105372 CrossRef CAS
.
- P. J. Mazzone, J. Hammel, R. Dweik, J. Na, C. Czich, D. Laskowski and T. Mekhail, Thorax, 2007, 62, 565 CrossRef PubMed
.
- R. Moreddu, J. S. Wolffsohn, D. Vigolo and A. K. Yetisen, Sens. Actuators, B, 2020, 317, 128183 CrossRef CAS
.
- M. Weston, M. A. T. Phan, J. Arcot and R. Chandrawati, Food Chem., 2020, 326, 127017 CrossRef CAS PubMed
.
- M. Weston, R. P. Kuchel, M. Ciftci, C. Boyer and R. Chandrawati, J. Colloid Interface Sci., 2020, 572, 31–38 CrossRef CAS PubMed
.
- N. A. Rakow and K. S. Suslick, Nature, 2000, 406, 710–713 CrossRef CAS PubMed
.
- D.-H. Park, J.-M. Heo, W. Jeong, Y. H. Yoo, B. J. Park and J.-M. Kim, ACS Appl. Mater. Interfaces, 2018, 10, 5014–5021 CrossRef CAS PubMed
.
- A. D. Tjandra, M. Weston, J. Tang, R. P. Kuchel and R. Chandrawati, Colloids Surf., A, 2021, 619, 126497 CrossRef CAS
.
- A. D. Tjandra, A.-H. Pham and R. Chandrawati, Chem. Mater., 2022, 34, 2853–2876 CrossRef CAS
.
- J. Yoon, S. K. Chae and J.-M. Kim, J. Am. Chem. Soc., 2007, 129, 3038–3039 CrossRef CAS PubMed
.
- S. Suklabaidya, S. Chakraborty, S. Sarkar, R. Paul, H. Banik, A. Chakraborty, D. Bhattacharjee, S. Majumdar and S. A. Hussain, J. Phys. Chem. C, 2021, 125, 15976–15986 CrossRef CAS
.
- M.-C. Tu, J. A. Cheema, U. H. Yildiz, A. Palaniappan and B. Liedberg, J. Mater. Chem. C, 2017, 5, 1803–1809 RSC
.
- L. H. Nguyen, S. Naficy, R. McConchie, F. Dehghani and R. Chandrawati, J. Mater. Chem. C, 2019, 7, 1919–1926 RSC
.
- B. W. Davis, A. J. Burris, N. Niamnont, C. D. Hare, C.-Y. Chen, M. Sukwattanasinitt and Q. Cheng, Langmuir, 2014, 30, 9616–9622 CrossRef CAS PubMed
.
- S. Dolai, S. K. Bhunia, S. S. Beglaryan, S. Kolusheva, L. Zeiri and R. Jelinek, ACS Appl. Mater. Interfaces, 2017, 9, 2891–2898 CrossRef CAS PubMed
.
- B. Gao, G. Yuan and L. Ren, J. Mater. Sci., 2018, 53, 6698–6706 CrossRef CAS
.
- M. Weston, A. D. Tjandra and R. Chandrawati, Polym. Chem., 2020, 11, 166–183 RSC
.
- S. Park, G. S. Lee, C. Cui and D. J. Ahn, Macromol. Res., 2016, 24, 380–384 CrossRef CAS
.
- T. Eaidkong, R. Mungkarndee, C. Phollookin, G. Tumcharern, M. Sukwattanasinitt and S. Wacharasindhu, J. Mater. Chem., 2012, 22, 5970–5977 RSC
.
- H. Jiang, Y. Wang, Q. Ye, G. Zou, W. Su and Q. Zhang, Sens. Actuators, B, 2010, 143, 789–794 CrossRef CAS
.
- M. Seo, D.-H. Park, B. J. Park and J.-M. Kim, J. Appl. Polym. Sci., 2017, 134, 44419 CrossRef
.
- D. Poli, P. Carbognani, M. Corradi, M. Goldoni, O. Acampa, B. Balbi, L. Bianchi, M. Rusca and A. Mutti, Respir. Res., 2005, 6, 71 CrossRef PubMed
.
- P. Mochalski, J. King, M. Klieber, K. Unterkofler, H. Hinterhuber, M. Baumann and A. Amann, Analyst, 2013, 138, 2134–2145 RSC
.
- R. Capuano, M. Santonico, G. Pennazza, S. Ghezzi, E. Martinelli, C. Roscioni, G. Lucantoni, G. Galluccio, R. Paolesse, C. Di Natale and A. D'Amico, Sci. Rep., 2015, 5, 16491 CrossRef CAS PubMed
.
- W. Filipiak, V. Ruzsanyi, P. Mochalski, A. Filipiak, A. Bajtarevic, C. Ager, H. Denz, W. Hilbe, H. Jamnig, M. Hackl, A. Dzien and A. Amann, J. Breath Res., 2012, 6, 036008 CrossRef CAS PubMed
.
- J. Rudnicka, T. Kowalkowski and B. Buszewski, Lung Cancer, 2019, 135, 123–129 CrossRef PubMed
.
- X.-A. Fu, M. Li, R. J. Knipp, M. H. Nantz and M. Bousamra, Cancer Med., 2014, 3, 174–181 CrossRef CAS PubMed
.
- A. Ulanowska, T. Kowalkowski, E. Trawińska and B. Buszewski, J. Breath Res., 2011, 5, 046008 CrossRef PubMed
.
- Y. Saalberg, H. Bruhns and M. Wolff, Sensors, 2017, 17, 210 CrossRef PubMed
.
- M. Ligor, T. Ligor, A. Bajtarevic, C. Ager, M. Pienz, M. Klieber, H. Denz, M. Fiegl, W. Hilbe, W. Weiss, P. Lukas, H. Jamnig, M. Hackl, B. Buszewski, W. Miekisch, J. Schubert and A. Amann, Clin. Chem. Lab. Med., 2009, 47, 550–560 CAS
.
- A. Bajtarevic, C. Ager, M. Pienz, M. Klieber, K. Schwarz, M. Ligor, T. Ligor, W. Filipiak, H. Denz, M. Fiegl, W. Hilbe, W. Weiss, P. Lukas, H. Jamnig, M. Hackl, A. Haidenberger, B. Buszewski, W. Miekisch, J. Schubert and A. Amann, BMC Cancer, 2009, 9, 348 CrossRef PubMed
.
- D. Poli, M. Goldoni, M. Corradi, O. Acampa, P. Carbognani, E. Internullo, A. Casalini and A. Mutti, J. Chromatogr. B: Anal. Technol. Biomed. Life Sci., 2010, 878, 2643–2651 CrossRef CAS PubMed
.
- C. Lourenço and C. Turner, Metabolites, 2014, 4, 465–498 CrossRef PubMed
.
- E. Mansour, R. Vishinkin, S. Rihet, W. Saliba, F. Fish, P. Sarfati and H. Haick, Sens. Actuators, B, 2020, 304, 127371 CrossRef CAS
.
-
J. G. Speight, in Natural Water Remediation, ed. J. G. Speight, Butterworth-Heinemann, 2020, pp. 263–303 Search PubMed
.
-
NIST, NIST Chemistry WebBook, 2018, DOI:10.18434/T4D303
.
- S. Jang, S. U. Son, J. Kim, H. Kim, J. Lim, S. B. Seo, B. Kang, T. Kang, J. Jung, S. Seo and E.-K. Lim, Food Chem., 2023, 403, 134317 CrossRef CAS PubMed
.
- A. D. Tjandra, T. Heywood and R. Chandrawati, Biosens. Bioelectron.: X, 2023, 14, 100361 CAS
.
- M. Safdar, G. Cui, Y. J. Kim and M. R. Luo, Opt. Express, 2017, 25, 15131–15151 CrossRef PubMed
.
- W. Mokrzycki and M. Tatol, Mach. Graph. Vis., 2011, 20, 383–411 Search PubMed
.
- C. Gambardella, R. Parente, A. Ciambrone and M. Casbarra, Data, 2021, 6, 108 CrossRef
.
- I. T. Jolliffe and J. Cadima, Philos. Trans. R. Soc., A, 2016, 374, 20150202 CrossRef PubMed
.
-
S. Litman, P. Geddes, M. Edwards, M. Carson Jr. and K. J. Hook, US Pat., 20170096570A1, 2015 Search PubMed
.
-
P. Wallace and O. Morel, WIPO (PCT) Pat., 2020021237A1, 2019 Search PubMed
.
- A. Kamphan, C. Khanantong, N. Traiphol and R. Traiphol, J. Ind. Eng. Chem., 2017, 46, 130–138 CrossRef CAS
.
- J. Lee, S. Balakrishnan, J. Cho, S.-H. Jeon and J.-M. Kim, J. Mater. Chem., 2011, 21, 2648–2655 RSC
.
- J. Lee, H. T. Chang, H. An, S. Ahn, J. Shim and J.-M. Kim, Nat. Commun., 2013, 4, 2461 CrossRef PubMed
.
- T. Ogawa, Prog. Polym. Sci., 1995, 20, 943–985 CrossRef CAS
.
- R. E. Navarro and T. Ogawa, J. Polym. Sci., Part A: Polym. Chem., 1989, 27, 2143–2149 CrossRef CAS
.
- C. Khanantong, N. Charoenthai, T. Phuangkaew, F. Kielar, N. Traiphol and R. Traiphol, Colloids Surf., A, 2018, 553, 337–348 CrossRef CAS
.
- N. Bolourchian, M. M. Mahboobian and S. Dadashzadeh, Iran. J. Pharm. Res., 2013, 12, 11–20 CAS
.
- O. Barash, N. Peled, U. Tisch, P. A. Bunn, F. R. Hirsch and H. Haick, Nanomedicine, 2012, 8, 580–589 CrossRef CAS PubMed
.
- K. Schallschmidt, R. Becker, C. Jung, J. Rolff, I. Fichtner and I. Nehls, J. Chromatogr. B: Anal. Technol. Biomed. Life Sci., 2015, 1006, 158–166 CrossRef CAS PubMed
.
- T. Walser, X. Cui, J. Yanagawa, J. M. Lee, E. Heinrich, G. Lee, S. Sharma and S. M. Dubinett, Proc. Am. Thorac. Soc., 2008, 5, 811–815 CrossRef PubMed
.
- C. Wagner-Struwing, M. A. Munoz-Lucas, J. Jareno-Esteban, C. Civera-Tejuca, J. A. Maldonado-Sanz and L. Callol-Sanchez, Eur. Respir. J., 2014, 44, 1138 Search PubMed
.
- R. B. Jain, Biomarkers, 2016, 21, 342–346 Search PubMed
.
- M. Koureas, D. Kalompatsios, G. D. Amoutzias, C. Hadjichristodoulou, K. Gourgoulianis and A. Tsakalof, Molecules, 2021, 26, 2609 Search PubMed
.
- T. Sato and S. Kohnosu, Colloids Surf., A, 1994, 88, 197–205 CrossRef CAS
.
- M. Namasivayam, M. R. Andersson and J. G. Shapter, Polymers, 2021, 13, 2447 CrossRef CAS PubMed
.
- N. Nugraha, A. G. Saputro, M. K. Agusta, F. T. Akbar and A. D. Pramudya, Molekul, 2019, 14, 37 CrossRef
.
- R. L. Fomekong and B. Saruhan, Chemosensors, 2019, 7, 42 CrossRef CAS
.
- R. Wagner, D. Schönauer-Kamin and R. Moos, J. Electrochem. Soc., 2020, 167, 167516 CrossRef CAS
.
-
J. E. McMurry, Organic Chemistry, Cengage Learning, 2015 Search PubMed
.
- M. Belmares, M. Blanco, W. A. Goddard III, R. B. Ross, G. Caldwell, S. H. Chou, J. Pham, P. M. Olofson and C. Thomas, J. Comput. Chem., 2004, 25, 1814–1826 CrossRef CAS PubMed
.
- J. King, H. Koc, K. Unterkofler, P. Mochalski, A. Kupferthaler, G. Teschl, S. Teschl, H. Hinterhuber and A. Amann, J. Theor. Biol., 2010, 267, 626–637 CrossRef CAS PubMed
.
- I. Kushch, B. Arendacká, S. Stolc, P. Mochalski, W. Filipiak, K. Schwarz, L. Schwentner, A. Schmid, A. Dzien, M. Lechleitner, V. Witkovský, W. Miekisch, J. Schubert, K. Unterkofler and A. Amann, Clin. Chem. Lab. Med., 2008, 46, 1011–1018 CAS
.
- M. Lechner, B. Moser, D. Niederseer, A. Karlseder, B. Holzknecht, M. Fuchs, S. Colvin, H. Tilg and J. Rieder, Respir. Physiol. Neurobiol., 2006, 154, 478–483 CrossRef CAS PubMed
.
- S. Janfaza, B. Khorsand, M. Nikkhah and J. Zahiri, Biol. Methods Protoc., 2019, 4, bpz014 CrossRef CAS PubMed
.
- A. Cailleux, M. Cogny and P. Allain, Biochem. Med. Metab. Biol., 1992, 47, 157–160 CrossRef CAS PubMed
.
- R. F. Louie, Z. Tang, D. G. Shelby and G. J. Kost, Lab. Med., 2000, 31, 402–408 CrossRef
.
- L. Shi, J. Zhang, M. Zhao, S. Tang, X. Cheng, W. Zhang, W. Li, X. Liu, H. Peng and Q. Wang, Nanoscale, 2021, 13, 10748–10764 RSC
.
- E. Padín-González, P. Lancaster, M. Bottini, P. Gasco, L. Tran, B. Fadeel, T. Wilkins and M. P. Monopoli, Front. Bioeng. Biotechnol., 2022, 10, 882363 CrossRef PubMed
.
- H. Lee and R. G. Larson, Biomacromolecules, 2016, 17, 1757–1765 CrossRef CAS PubMed
.
-
NSW EPA, Approved Methods for the Modelling and Assessment of Air Pollutants in New South Wales: Draft, Department of Environment and Conservation (NSW), 2004 Search PubMed
.
- J. P. Yapor, A. Alharby, C. Gentry-Weeks, M. M. Reynolds, A. K. M. M. Alam and Y. V. Li, ACS Omega, 2017, 2, 7334–7342 CrossRef CAS PubMed
.
- V. K. Rao, N. L. Teradal and R. Jelinek, ACS Appl. Mater. Interfaces, 2019, 11, 4470–4479 CrossRef CAS PubMed
.
|
This journal is © The Royal Society of Chemistry 2024 |
Click here to see how this site uses Cookies. View our privacy policy here.