DOI:
10.1039/D4SM01065E
(Paper)
Soft Matter, 2024,
20, 8909-8923
Mapping deformation dynamics to composition of topologically-active DNA blends†
Received
8th September 2024
, Accepted 27th October 2024
First published on 28th October 2024
Abstract
Blends of circular and linear polymers have fascinated researchers for decades, and the role of topology on their stress response and dynamics remains fervently debated. While linear polymers adopt larger coil sizes and form stronger, more pervasive entanglements than their circular counterparts, threading of circular polymers by linear chains can introduce persistent constraints that dramatically decrease mobility, leading to emergent rheological properties in blends. However, the complex interplay between topology-dependent polymer overlap and threading propensity, along with the large amounts of material required to sample many compositions, has limited the ability to experimentally map stress response to composition with high resolution. Moreover, the role of supercoiling on the response of circular-linear blends remains poorly understood. Here, we leverage in situ enzymatic topological conversion to map the deformation dynamics of DNA blends with over 70 fractions of linear, ring and supercoiled molecules that span the phase space of possible topological compositions. We use OpTiDDM (optical tweezers integrating differential dynamic microscopy) to map strain-induced deformation dynamics to composition, revealing that strain-coupling, quantified by superdiffusive dynamics that are aligned with the strain, is maximized for blends with comparable fractions of ring and linear polymers. Increasing the supercoiled fraction dramatically reduces strain-coupling, while converting rings to linear chains offers more modest coupling reduction. We demonstrate that these results are a direct consequence of the interplay between increasing polymer overlap and decreasing threading probability as circular molecules are converted to linear chains, with a careful balance achieved for blends with ample ring fractions but devoid of supercoiled molecules.
Introduction
Blends of polymers of different topologies are widely used in industry to improve performance metrics such as miscibility and strength-to-weight ratios,1–7 and are leveraged by many biological systems, such as the cell cytoplasm, to enable key mechanical processes.8–13 The advantage of blends over single constituent systems is their expanded breadth of relaxation modes and timescales, as well as intrinsic length scales, allowing for broader dynamic range and enhanced tunability. Cells harness these features to perform numerous distinct processes that occur over decades of spatiotemporal scales.14–17 Biology takes this advantageous design one step further by utilizing enzymes to alter the topologies and lengths of biopolymers, such as DNA, to enable a diversity of mechanical processes and state transitions that are dictated by the varying intra- and inter-polymer interactions.18–22 At the same time, many industrial processes performed on blends may intentionally or unintentionally shear, break or otherwise alter the topologies comprising polymers.23–27 Understanding how the time-varying alteration of blend composition alters the response dynamics of the polymers subject to stresses and strains, remains poorly understood.
Moreover, previous studies have shown that polymeric blends and composites exhibit scale-dependent mechanical properties and dynamics, with the bulk rheological response not directly mapping to the microscale relaxation dynamics;28–33 as well as signatures of dynamic heterogeneities and glassiness.34–37 For example, previous particle-tracking microrheology studies showed that the viscosity of solutions of overlapping circular double-stranded DNA steadily increased as the polymers were enzymatically linearized (i.e., both strands were cleaved at a single location).20 This effect was shown to arise from increased polymer overlap due to the size of the random coil of a linear chain being substantially larger than that of a circular (ring or supercoiled) polymer of equal length. Namely, as the solution composition became a blend of increasing linear fraction, the degree of overlap and entanglements increased, restricting the polymer motion and increasing local viscosity. The rheological response at the bulk scale was shown to be highly distinct from the microscale, with the viscoelastic moduli exhibiting sharp transitions from fluid-like to elastic-like states, rather than steady increase,37 which was shown to arise from cooperative clustering of entangled linear chains.35 This cooperative clustering of the ‘slow’ population in the blend also gave rise to an unexpected decrease in ensemble-averaged DNA mobility as they were enzymatically fragmented into shorter constructs.28
The scale-dependent dynamics of ring-linear blends are further complicated by the ability of ring polymers to become threaded by neighboring linear chains and, to a lesser extent, ring and supercoiled chains.1,5,31,35,36,38–41 In both solutions and melts, threading drastically slows the motion of the rings by essentially pinning them in place until the penetrating linear chains can diffuse out of the ring center and release their constraint. At high enough polymer concentrations and lengths, threading dominates the rheological and dynamical fingerprint of ring-linear blends, leading to an emergent increase in the elastic plateau modulus, viscosity, and relaxation timescales compared to their pure linear and ring counterparts over a range of blend compositions.29,36,38–40,42–44 Threading has also been suggested to lead ring-linear blends to exhibit more pronounced entropic stretching and shear-thinning in response to strain, increased heterogeneities in transport modes, slower diffusion, and more pronounced subdiffusion compared to pure solutions of linear or ring polymers.36,38–40,42,45,46 However, the exact dependence of these effects on the blend composition (i.e., the fraction of each topology) is a topic of debate due to the difficulty in preparing enough different blend compositions to comprehensively map the effect of composition onto dynamics. Moreover, while some of these emergent properties have been observed in both solutions and melts, such as increased viscosity and extended relaxation times,40,46,47 other features, such as an extended rubbery regime,29,48 have only been reported in solutions. These challenges are further complicated by the inherent scale-dependence and heterogeneity of these properties, rendering results from different measurement techniques that probe different scales difficult to couple.
Here, we leverage the enzymatic topological conversion of concentrated solutions of DNA to map the deformation dynamics of DNA solutions with dozens of fractions of linear, ring and supercoiled molecules that span the phase space of possible compositions. We use OpTiDDM (Optical Tweezers integrating Differential Dynamic Microscopy) to measure the polymer dynamics induced by local strains imposed by optically trapped probes; and elucidate how both the alignment of the DNA motion with the imposed strain, as well as the DNA transport properties, depend on composition and distance from the local strain. To determine the deformation dynamics with high resolution in composition and across a range of spatiotemporal scales, we perform measurements during the active cleaving of DNA by enzymes at multiple stoichiometries, to measure dynamics of blends with over 70 different compositions that range from purely circular, with ∼65% rings and ∼35% supercoiled, to purely linear chains of the same length.
Results
Design of topologically-active DNA solutions to span the composition space of blends of ring, supercoiled and linear polymers
We aimed to design a system in which we could perform dozens of measurements over time that each capture a unique topological composition of a single sample, and could span the full range of possible blend compositions. To this end, we start with a solution of 5.9 kbp DNA (Fig. 1a) that comprises ∼65% relaxed circular (ring) and ∼35% supercoiled molecules at a total concentration of c = 6 mg mL−1, corresponding to ∼5.3× above the overlap concentration of c* ≃ 1.14 mg mL−1 for this specific topological composition (see Methods).28,49
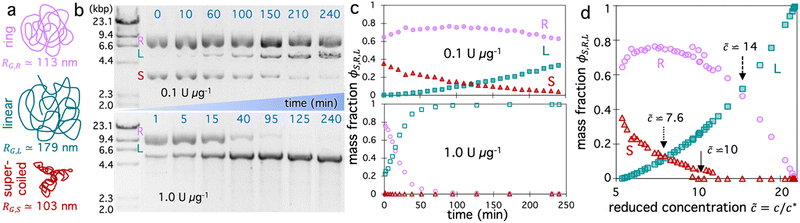 |
| Fig. 1 Mapping the composition space of topological DNA blends. (a) Cartoon of different topologies of 5.9 kbp DNA comprising blends – ring (R), linear (L), and supercoiled (S) –color-coded and ordered as they appear in (b) and with their radius of gyration RG listed. (b) Images of gel electrophoresis of blends of ∼65% ring and ∼35% supercoiled DNA with total mass concentration c = 6 mg mL−1 undergoing linearization by BamHI at a BamHI:DNA stoichiometry of 0.1 U μg−1 (top) or 1 U μg−1 (bottom). Far-left lane is λ-HindIII molecular weight marker with the size of linear DNA corresponding to each band listed. Each subsequent lane shows the topological state of the DNA blend at a distinct time-point during linearization, listed in mins above the corresponding lane. Each band corresponds to a distinct topology (R, L, S) as labeled to the right of the marker, and the relative intensity of each band indicates its relative mass fraction ϕS,R,L = cS,R,L/c. (c) Mass fraction of ring (purple circles), supercoiled (dark red triangles), and linear (teal squares) DNA as functions of digestion time, determined via gel electrophoresis band intensity analysis for 0.1 U μg−1 (top, filled symbols) and 1 U μg−1 (bottom, open symbols). (d) Data shown in (c), with both stoichiometries plotted together as a function of the reduced concentration = c/c*, which provides a measure of the degree of coil overlap for each unique blend composition defined by (ϕR, ϕS, ϕL). Arrows indicate key topological transitions with their values listed: linear fraction surpasses supercoiled fraction (dotted), supercoiled chains are eliminated (double solid), and linear fraction becomes the largest topological fraction (dashed). | |
To vary the blend composition, we introduce a single-site restriction endonuclease, BamHI, that cuts both strands of the DNA in a single location to convert both supercoiled and ring constructs to linear form (Fig. 1a and b).49,50 By using a low stoichiometry of enzyme to DNA we allow digestion kinetics to be slow on the timescale of a single 50-s OpTiDDM measurement, so that the solution can be considered to be in quasi-steady-state,20 and so a high composition resolution can be achieved (Fig. 1b and c). However, to capture the full range of blend compositions, i.e., allowing the enzyme to fully digest (linearize) all of the DNA, we need digestion kinetics to be fast enough to complete digestion before potentially deleterious photobleaching effects or enzymatic star activity occur (after ∼6 hours). Due to the exponential Michaelis–Menten digestion kinetics,20 achieving complete digestion in a limited amount of time requires that the digestion rate is prohibitively high at early times to resolve closely spaced compositions and maintain the quasi-steady-state assumption (Fig. 1b). However, reducing the initial digestion rate to achieve this resolution prohibits reaching complete digestion in the limited time window. Therefore, to achieve these upper and lower bounds on kinetics, we perform measurements with two different stoichiometries that differ by 10-fold, 0.1 U μg−1 and 1 U μg−1. With these two stoichiometries, we are able to accurately capture the full range of compositions from initial to saturating (all linear) conditions while ensuring the quasi-steady-state assumption is valid over each 50-s experiment (Fig. 1a and b).
Fig. 1b shows the fraction of each topology over the course of 4 hours for each stoichiometry, determined from gel electrophoresis band intensity analysis (see Methods), showing thorough sampling of the full composition range. Because the radius of gyration of the DNA molecules are topology-dependent, with values of RG,S ≃ 103 nm, RG,R ≃ 113 nm, and RG,L ≃ 179 nm for supercoiled, ring and linear topologies,50–52 the coil overlap concentration c* = (3/4π)(M/NA)RG−3 varies in time as the fraction of each topology, ϕS,R,L = cS,R,L/c, changes. Specifically, c* decreases as ring and supercoiled constructs are converted to linear topology, according to the expression c* = (3/4π)(M/NA)/(ϕLRG,L3 + ϕRRG,R3 + ϕSRG,S3),20,41 such that the reduced concentration,
= c/c*, which quantifies the degree of overlap, increases (Fig. 1c).
We expect the initial primarily circular blends (ϕL ≃ 0,
≃ 5) to be overlapping but not entangled due to the reduced concentration being below the nominal entanglement concentration
e ≃ 6 (Fig. 1c).20,53 Several studies have also suggested that circular polymers display weaker and less persistent intermolecular interactions compared to canonical linear chain entanglements.35,54–56 Conversely, for the completely digested system, with
≃ 23, we expect the DNA to be classically entangled and their dynamics governed by entanglement tube confinement.20,57 We focus our discussion in the following sections on the compositions that lie between these bounds, for which the dynamics are still poorly understood and expected to be much richer.
Strain-induced DNA dynamics display complex dependence on degree of coil overlap
We now seek to determine how the blend composition impacts the ability of the surrounding molecules to couple their motion to a local strain, and how this coupling decays with distance from the strain. We use OpTiDDM45 to cyclically sweep an optically trapped probe through the DNA solution and simultaneously image fluorescent-labeled DNA molecules in the solution in a field-of-view (FOV) that encompasses the horizontally aligned strain path (Fig. 2a and b) and extends 35 μm vertically above and below the strain path (Fig. 2c). We fix the strain distance (s = 15 μm) and rate (
= 42 s−1) to be large compared to the intrinsic lengthscales and timescales of the system and match previously used parameters that maximize the coupling in similar systems.28,45 Specifically, the strain rate corresponds to Weissenberg numbers of Wi ≈ 11 and Wi ≈ 176 and Deborah numbers of De ≈ 0.8 and De ≈ 12.6 for
≃ 5 and
≃ 23, respectively (see Methods). We are thus well within the nonlinear regime and probing the viscoelastic response of the polymers. We expect the flow field induced by the probe to be primarily shear-dominated,58,59 but also exhibit signatures of extensional flow.42,60
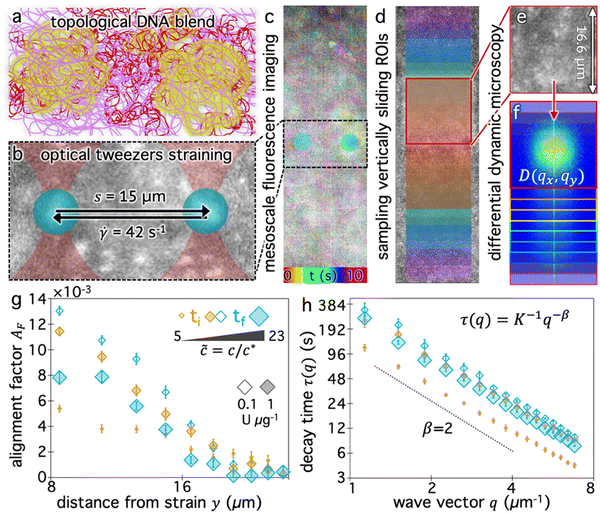 |
| Fig. 2 Spatially and temporally resolving the deformation dynamics of topologically-varying DNA blends using OpTiDDM. (a) Cartoon of initial blend of ∼65% ring (purple) and ∼35% supercoiled (dark red) DNA with a small fraction of labeled DNA indicated by yellow glow. (b) Cartoon of optical tweezers straining of DNA blends doped with fluorescent-labeled molecules (white features) to visualize deformation dynamics. A focused laser beam (red) moves an optically-trapped microsphere probe (blue) of radius r = 2.25 μm back and forth at a constant rate = 42 s−1 over a strain distance s = 15 μm through the blend for a total time of 50 s per measurement, with a 3 s cessation period between each sweep. (c) During each strain, time-series of labeled molecules are collected within a 72 μm × 16.6 μm field-of-view, centered vertically on the strain path and enveloping the strain horizontally, as shown. The temporal color map shows the DNA motion during 3 consecutive sweeps (∼10 s) with colors corresponding to different times relative to the beginning of the first sweep. (d) The FOV is divided into 20 ROIs (translucent squares) of size (16.6 μm)2 centered horizontally with the strain path and centered vertically at 10 ±distances from the strain path. Increasing ±y values follow a rainbow scale from y0 = 8 μm (red) to yf = 27 μm (magenta). (e) and (f) DDM analysis of individual ROIs (e) provides 2D image structure functions D(qx, qy) for each distance y. (g) and (h) OpTiDDM measurements are performed every ∼1–10 minutes from the beginning (ti ⋍ 5 min, orange) to end of the digestion period (tf = 240 min) for stoichiometries (U μg−1) of 0.1 (open symbols) and 1 (translucent filled symbols). The symbol sizes denote the relative reduced concentrations at the beginning and end of each stoichiometric digestion period (see legend). (g) The alignment factor AF, which quantifies the preferential alignment of DNA motion along the strain path, is determined by assessing the anisotropy of D(qx, qy) for a given distance y, and generally decreases with increasing y values. (h) The decay time τ(q), determined by azimuthal averaging and fitting of D(qx, qy, Δt), quantifies the type and rate of motion. τ(q) typically follows power-law scaling τ(q) = K−1q−β. Dashed line denotes β = 2 which is indicative of normal diffusive motion. | |
Fig. 2c qualitatively depicts the strain-induced deformation field for the starting composition (Fig. 2a), showing more pronounced motion near the strain and reduced motion further from the strain. We note that the signal-to-noise is low and single molecules are not easily resolved. This effect is a consequence of including a relatively high fraction of labeled molecules in solution to ensure ample statistics within small spatially resolved region-of-interests (ROIs), and is one of our motivations for using differential dynamic microscopy (DDM), rather than, e.g., particle-tracking or particle-image-velocimetry, to quantify DNA dynamics.
Specifically, we divide the FOV into (16 μm)2 ROIs centered at 20 vertical positions from y0 = 8 μm to yf = 27 μm (Fig. 2d), and perform DDM on each ROI (Fig. 2e–h). As described in Methods, DDM converts a time-series into stacks of image differences which encode information about how correlated two images separated by a given lag time Δt are, which can be analyzed to extract dynamics.16,61–63 In practice, DDM transforms image differences to Fourier space to compute image structure functions
(Fig. 2e and f) that quantify correlations in density fluctuations at a given spatial frequency, or wave vector
, as a function of Δt (Fig. 2f). As described in the following sections, from
we determine the (1) extent to which the direction of DNA motion aligns with the strain direction, which we quantify by the alignment factor AF(y,
) (Fig. 2g), and (2) type and rate of DNA motion, which we quantify by analyzing the q-dependent DDM decay time τ(q, y,
) (Fig. 2h). Fig. 2g and h shows these metrics measured near the beginning and end of each stoichiometric digestion (0.1 and 1 U μg−1), demonstrating that composition generally has a significant effect on the dynamics. We also observe that both metrics display a similar non-monotonic dependence on
, with the strongest alignment and longest decay time occurring at intermediate
values. In the absence of topological conversion, one may expect these metrics to increase monotonically with
, insofar as stronger connectivity and increased steric hindrances are expected to slow motion (increasing τ) and enhance affine response to strain (increasing AF). We investigate the functional form of these non-monotonic dependences and their underlying mechanisms in the remaining sections. We choose to characterize composition primarily by
rather than the mass fraction of linearized molecules ϕL because
accounts for the fraction of all three topologies in a single quantity. Conversely, a single ϕL value could correspond to multiple compositions with varying relative fractions of ring and supercoiled molecules, which we expect to have a marked effect on dynamics due to their differing sizes and propensities for threading.28,64
Strain alignment and propagation are maximized at intermediate reduced concentrations
For isotropic motion, such as Brownian motion,
is radially symmetric, and taking the azimuthal average over
provides a 1D image structure function,
that can be fit to models to determine the type and rate of motion.16,62 However, for motion that has a preferred direction,
is anisotropic, with higher correlations being weighted more heavily along the director axis,45 a feature that we leverage to quantify the degree of alignment. Specifically, we compute the alignment factor AF of the image structure function relative to the qx-axis by computing weighted azimuthal integrals of D(qx, qy, Δt), i.e., integrals over θ where θ = tan−1(qy/qx):
(see Methods, Fig. 2g).65,66AF increases from 0 (for purely isotropic motion) as motion becomes more aligned along the strain direction (x-axis).45 We find that AF decays with increasing distance from the strain y for all compositions, as expected (Fig. 3a). However, the degree of alignment closest to the strain (y0 = 8 μm), and the extent to which alignment persists as y increases, displays a non-monotonic dependence on composition (Fig. 2g and 3).
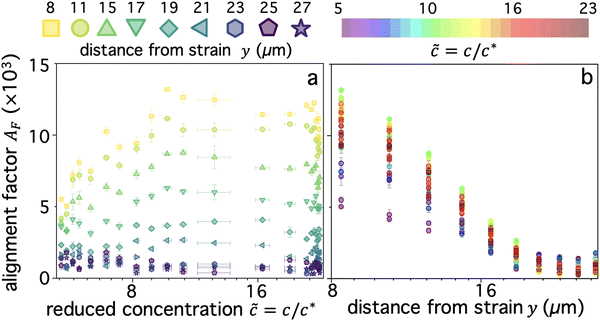 |
| Fig. 3 Strain alignment of DNA motion decays with increasing distance from the strain and displays non-monotonic dependence on reduced concentration. (a) Alignment factor AF as a function reduced concentration measured at 10 vertical distances from the strain, ranging from y0 = 8 μm (yellow squares) to yf = 27 μm (purple stars), as indicated in the legend. Vertical and horizontal error bars are standard error across replicates and consecutive measurements, respectively (see Methods), and data points are means across both. (b) Data shown in (a), plotted as a function of y for each sampled blend composition, characterized by a different denoted by a different color. The rainbow colorscale shows the range of sampled values from i ≈ 5 to f ≈ 23, with increasing values shown as progressively warmer tones. Data points and error bars represent the mean and standard error across replicates. | |
As shown in Fig. 3a, AF(y0) initially increases as
increases from the initial value of
i ≃ 5.4 until
≃ 10 where AF reaches a maximum. As
increases further, the alignment factor becomes largely independent of composition, with AF values remaining substantially higher than that for
i. This behavior persists until the highest
values (
≳ 22), in which the solutions comprise nearly all linear chains (Fig. 1d), at which point we see a subsequent drop in AF. This general non-monotonic dependence of
on AF holds for increasing y values until y ≃ 17 μm, at which point AF becomes increasingly insensitive to composition, and is essentially statistically insignificant for y > 20 μm.
This effect can also be seen clearly in Fig. 3b which shows AF as a function of y for different blend compositions, denoted by the rainbow colorscale. For y < 20 μm, the non-monotonic
dependence is shown by the maximum and minimum values being green (
≃ 10) and purple (
i ≈ 5) while the red tones that denote
≳ 17 reside between these extrema. Two other features that are apparent are: the decrease in composition dependence for y > 20 μm, seen as a much smaller spread in the data compared to y < 20 μm; and the enhanced y dependence for
≃ 10 compared to higher or lower values, with AF dropping by a factor of ∼15 as y increases, versus ∼5 and ∼10 for
i ≈ 5 and
f ≈ 23.
These results suggest that interactions between linear and circular molecules, which are absent for
i and
f (Fig. 1d) provide more effective mechanisms for coupling to the strain compared to linear–linear and circular–circular interactions. As described in the Introduction, numerous previous studies have shown strong evidence of threading of rings by linear chains, which dramatically slows relaxation processes and enhances shear-thinning and viscosity. These features align with the enhanced stretching of threaded rings, as compared to entangled linear or ring polymers, reported by several studies.5,40,45,60 Likewise, we can understand the increased strain alignment at intermediate
values as arising from threaded rings being maximally stretched along the strain direction due to the rigid constraints imposed by the threading chains that strongly resist strain-induced flow. In other words, as the ring is pulled along the strain path, the constraints (threadings) pull against the strain, thereby entropically stretching the threaded ring in the strain direction. A similar effect can occur for entangled linear polymers that are constrained by entanglements from neighboring chains. However, these constraints are expected to be less persistent than threadings, relaxing via reptation versus constraint release, rendering the entropic stretching weaker. We note that due to the nonlinear nature of the straining, we expect convective constraint release (CCR) to play a role in the dynamics for all entangled systems, regardless of the degree of threading.48,57 CCR, which reduces the local entanglement density, likely counteracts the propensity for chains to stretch along the strain direction, thereby dampening the strain-coupling we observe.
Collectively, this topological dependence on stretching is a plausible mechanism for the initial strong increase in alignment as
increases from
i ≃ 5.4 to
max ≃ 10, followed by a modest decrease and greater spread as
increases further to
f ≃ 23. Namely, as the degree of overlap increases and supercoiled molecules are replaced with linear chains (Fig. 1d), the entanglement density increases substantially and threading events become more pervasive. However, further increases in the degree of overlap (beyond
max ≃ 10), a result of rings being converted to linear chains, come at the cost of threading events, so serve to weaken alignment. It is important to note that if increased alignment was primarily a result of increased overlap/entanglement density, then AF should increase monotonically with
. Instead, the non-monotonic dependence on
is direct evidence of topological effects dictating the strength of strain coupling. This conjecture is further supported by the larger drop in alignment that only occurs at the very highest
values (
≳ 22) where the solutions comprise nearly all linear chains (Fig. 1d and 3a).
Finally, we note that the region of composition space in which the degree of alignment is maximized is that in which the fraction of linear chains ϕL surpasses that of supercoiled molecules ϕS (Fig. 1d,
> 7.6) but remains lower than the fraction of rings ϕR (
< 14). We also note that the maximally aligned composition (
max ≃ 10) occurs at the point at which supercoiled molecules are completely eliminated, at which point we expect that all molecules (rings and linear chains) likely participate in threading events.
Strain-induced superdiffusivity is facilitated by rings and maximized for ring-linear blends
The data shown in Fig. 3 suggests that the strain induces athermal directed motion of the DNA, which is maximized at an intermediate overlap of
max ≃ 10 and significant for distances out to y ≈ 20 μm. To shed light on the nature of this athermal motion and the extent to which the strain dynamics can fingerprint onto the DNA, we turn to analyzing the q-dependent decay time τ(q), as described above and in Methods (Fig. 2h). τ(q) typically displays power-law dependence on wave vector q, τ(q) = K−1q−β, where β = 2, 1 < β < 2 and β = 1 denote diffusive, superdiffusive and ballistic dynamics, respectively.16,45,67 The larger τ(q) is for a given q, the slower the dynamics, which is quantified by the generalized transport coefficient K. Higher K values generally indicate faster motion; and for purely diffusive or ballistic motion, K respectively equates to the diffusion coefficient or speed.
We first examine dynamics closest to the strain (y0 = 8 μm), where we observe the most pronounced alignment (Fig. 4a and b). We find that τ(q) curves for all compositions obey power-law scaling that approximately aligns with diffusive behavior (β = 2). However, the magnitude of τ(q) is generally highest for
≈ 10–12 and lowest for
i ≈ 5, following a similar non-monotonic trend as AF. These features can be seen more clearly by examining the functional form of τq2, which is a horizontal line for β = 2 and adopts increasingly positive slopes as β decreases to more superdiffusive exponents (Fig. 4b). The relative rate of motion can also be approximated as the inverse of τq2. All compositions appear to display modest superdiffusivity over at least some region of q space, which appears to be most extreme for intermediate compositions. The mobility also markedly slows as
increases from its initial value to
max ≃ 10.
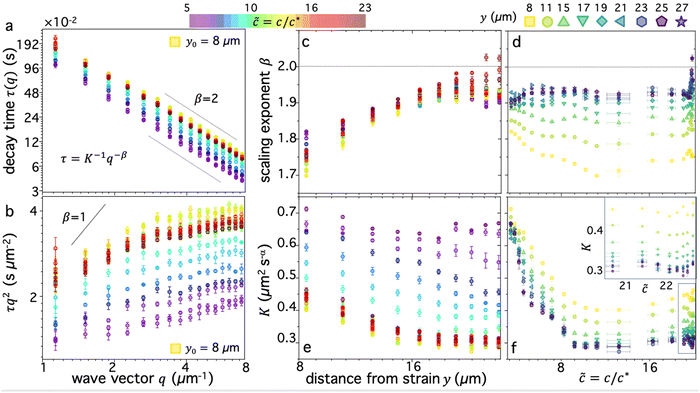 |
| Fig. 4 Digestion of supercoiled DNA enhances strain-coupled superdiffusivity while slowing transport. (a) DDM decay time τ(q) versus wave vector q, evaluated at y0 = 8 μm, for varying blend compositions, characterized by , represented as cool to warm colors for i ≃ 5 (purple) to f ≃ 23 (dark red). Green and yellow data points which are extremal for nearly all q values, correspond to ≈ 10. Dashed scaling bars denote diffusive (β = 2) scaling exponents associated with the expected power-law relation τ(q) = K−1q−β. (b) Data shown in (a) plotted as τq2versus q, which is independent of q for diffusive motion (β = 2) and displays an increasingly positive slope as motion becomes more superdiffusive, bounded above by ballistic scaling β = 1 (solid line). (c) and (d) Scaling exponent β versus (c) distance y and (d) blend composition , determined from fitting τ(q) for each blend composition and distance to τ(q) = K−1q−β. Dashed horizontal line denotes diffusive scaling and data that falls below is superdiffusive. (e) and (f) Transport coefficient K versus (e) distance y and (f) blend composition , determined from the same fits used for (c) and (d). K values are in units of μm2 s−α, where α = 2/β. Inset in (f) is zoom-in of high data enclosed in the main plot, showing increase in K when all circular DNA is digested (highest ). Note that lower scaling exponents β (more superdiffusive) generally correlate nonintuitively with lower transport coefficients K (slower motion). Colors, symbols and error bars are as in Fig. 3. | |
To quantitatively examine these features and determine the extent to which they persist for molecules that are increasingly farther from the strain, we fit the data for each composition and distance to τ(q) = K−1q−β and evaluate β and K as functions of y (Fig. 4c–f). We observe that for all compositions, β increases with increasing distance y, extending from superdiffusive values as low as β ≈ 1.7 at y0 to diffusive scaling at the largest distance. Insofar as superdiffusivity can be taken as an indicator of strain-coupling, which adds a component of directed ballistic motion to the otherwise thermal diffusive motion of the DNA, this result is intuitive and corroborates our alignment factor analysis (Fig. 3). Moreover, β values display a similar non-monotonic dependence on composition, with
≈ 10 exhibiting the most pronounced superdiffusivity for nearly all y values, while
i and
f blends have higher (less superdiffusive) β values. Also mirroring the alignment factor analysis, we observe a significant uptick in β near
f, where we expect blends to be nearly devoid of rings. This non-trivial composition dependence can also be seen clearly by examining β versus
for the different distances (Fig. 4d), which display global minima at
max ≈ 10 for distances out to y ≈ 20 μm, a feature that is most pronounced closest to the strain.
Perhaps less intuitive is the larger effect of composition on β at both small and large distances compared to intermediate y values, which can be seen by the larger spread in the data at the left and right edges (low and high y) of Fig. 4c compared to the middle values. Examination of Fig. 4d reveals that this large spread is due to distinct trends for close versus far distances. Namely, at small y values, the spread is due to the large decrease in β values as
increases to ∼10, while for large distances this spread is from a larger increase in β values at the very highest
values compared to the other distances. The spread in values extends to β > 2, indicative of subdiffusive motion, which has been reported for entangled linear DNA.45 This finding suggests that even a small number of rings in the blend may be sufficient to enhance strain coupling, in accord with recent observations that a small fraction of rings in synthetic ring-linear blends substantially increases the melt viscosity.46
To shed further light on these results, we also examine the dependence of transport coefficients K on
and y (Fig. 4e and f). We observe similar non-monotonic dependence on
as for the scaling exponents (Fig. 4c and d) and alignment factor (Fig. 3), with K decreasing substantially from
i to
max followed by a modest increase as
increases to
f (Fig. 4e and f). Also consistent with the trends of the other metrics, K generally decreases with increasing distance from the strain, and this decrease is more pronounced for higher
values. Finally, at the highest
values (
> 21), we observe a more substantial uptick in K values, similar to the trends observed for β and AF.
While the general trends are consistent across metrics, the relationship between K and β appears complex and perhaps counter to expectations. One may expect that increased superdiffusivity, manifested as lower β values (within the range 2 ≥ β ≥ 1), should result in generally faster motion, described by a higher transport coefficient K. This relation is indeed what we observe for the y dependence: β increases and K decreases with increasing y values (Fig. 4c and e). This dependence suggests that it is the strain-coupling that primarily dictates the expected inverse relationship, with molecules closer to the strain being more strongly stretched along the strain path, resulting in faster and more directed motion. This strain-coupling decays as we move further from the strain site. Conversely, the coupled dependence of K and β on
displays an opposite trend, whereby increasing β values correlate with increasing K values. In other words, as motion becomes ostensibly more superdiffusive (strain-coupled) it actually appears to be slower, i.e., the rate of motion is smaller. This effect can be seen in Fig. 4c and e, where
≈ 10 blends have the lowest values of both β (Fig. 4c) and K (Fig. 4e) among the different compositions; and in Fig. 4d and f which shows that both values generally decrease from
i to
max, followed by a modest increase.
We conjecture that this positive correlation between β and K arises from the slowing of quiescent thermal relaxation modes due to threading events that likewise enhance strain-coupling. Stronger constraints lead to stronger strain-coupling, captured by increased alignment and superdiffusivity. However, they also more strongly suppress thermal motion, which contributes to the transport coefficient in a non-trivial way. When constraints are weaker and/or threading is limited, we expect faster thermal transport (higher K) but less strain-induced motion (higher β, lower AF). Thus, for low
we expect K and β to be generally high and to display the weakest dependence on y, as we see in Fig. 4c–e.
To quantitatively verify this effect, we estimate the diffusion coefficients for the lowest and highest concentration cases as the transport coefficients at the largest y distance, where β values are near 2 (diffusive) and we expect little effect of the strain on dynamics. The values for
≈ 5 and
≈ 23 blends are K ≃ 0.65 μm2 s−1 and K ≃ 0.3 μm2 s−1, respectively. Closest to the strain, these transport coefficients increase to K ≃ 0.7 μm2 s−1.1 and K ≃ 0.45 μm2 s−1.2. The ∼50% increase in K for
≈ 23 is significantly higher than the ∼8% increase for
≈ 5. We can therefore conclude that the correlated decrease in K and β for high
compared to low
blends is a result of, respectively, suppressed diffusion and increased strain-coupling.
Moreover, the speed of the moving probe is v = 45 μm s−1 and the strain distance is s = 15 μm, so the resulting Peclet numbers are Pe ≈ vs/D ≈ 103. As such, if the polymers were completely coupled to the strain, then thermal motion would indeed be negligible. However, the extent to which the polymers couple to the strain depends on the degree to which the polymers are sterically constrained (e.g., entangled, threaded) and the extent to which CCR reduces the density of constraints. For less entangled blends, the coupling is weaker, so thermal motion contributes more to the dynamics on the timescale of the strain.
Transport deviates more strongly from diffusive behavior at larger lengthscales and for blends without supercoiled molecules
In the previous section, we approximated all τ(q) curves as obeying a simple power-law with a single q-independent scaling exponent β. However, closer inspection of τ(q) for varying distances reveals deviations from a single scaling law at small q values (q < 2 μm−1), which are more pronounced closer to the strain (Fig. 5a–c). This weaker scaling, indicative of more pronounced superdiffusivity, suggests that strain-coupling is more pronounced for larger lengthscale dynamics, i.e., at scales λ = 2πq−1 > 3 μm. This effect may indicate the necessity for many molecules to be sterically interacting, via entanglements/constraints, for dynamics to effectively couple to the strain, which we expect to only occur at scales several times larger than the polymer coil sizes,
.57
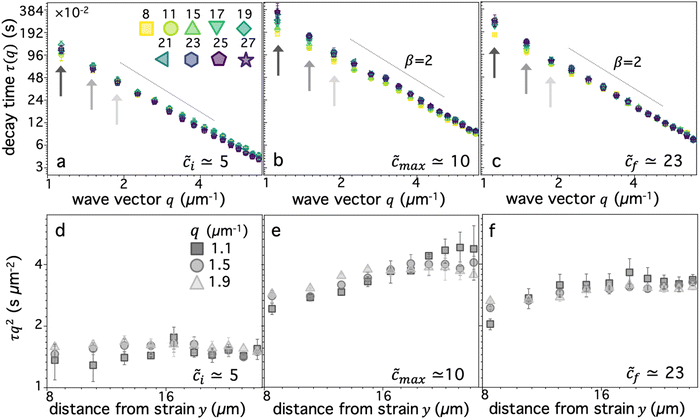 |
| Fig. 5 Transport displays increased distance dependence at larger lengthscales which is maximized at intermediate reduced concentration. (a)–(c) DDM decay time τ(q) versus wave vector q for varying distances y (listed in μm in legend in (a)), evaluated for blend compositions of (a) i ≃ 5, (b) max ≃ 10, and (c) f ≃ 23. (d)–(f) Data indicated by grey arrows in (a)–(c) plotted as τq2versus y, according to the legend shown in (d). All blends display modest increase in τq2 values (slower transport) with increasing distance, with the most pronounced and weakest slowing observed for max ≃ 10 (e) and i ≃ 5 (d), respectively. These trends are coupled with, respectively, the overall slowest and fastest transport (highest and lowest τq2 values). | |
We also find that the deviation towards enhanced superdiffusivity is most pronounced for
max ≃ 10 (Fig. 5b) and weakest for
i ≃ 5 (Fig. 5a). This result is coupled with the slowest and fastest transport, respectively, which can be seen by examining τq2 for the three smallest q values (Fig. 5d–f), where lower/higher values indicate faster/slower transport. These data also clearly show that the maximally strain-coupled composition (
max ≃ 10) is also the one with the largest dependence of mobility on distance from the strain site. Namely, τq2 displays the largest increase with increasing y, signifying the strongest strain-coupling; and this dependence is strongest for the smallest q value. This result corroborates the physical picture that strain-coupling is a many-polymer phenomena that requires numerous constraints and interactions to effectively propagate stress.
Entropic stretching and superdiffusive dynamics are linked effects that drive coupling of DNA dynamics to the strain
The results described in the previous sections suggest a direct linkage between alignment and superdiffusivity, which are both reporters of strain-coupling, while transport coefficients are sensitive to both strain-induced directed motion and quiescent thermal fluctuations. Moreover, the non-monotonic dependence of these metrics on blend composition demonstrates that DNA blends with comparable ring and linear topologies and devoid of supercoiled constructs, occurring at reduced concentrations of
≈ 10–12, exhibit emergent strain-coupling due to pervasive threading events. The dynamics of blends with lower
values, which have non-zero fractions of supercoiled constructs, are highly sensitive to variations in
, with metrics substantially increasing (AF) or decreasing (β, K) with increasing
. Because supercoiled molecules are not expected to participate in threading events,20,28,64 this result corroborates the importance of threading in strain-coupling. The sensitivity is likely amplified by the increasing overlap as smaller supercoiled molecules are replaced with larger linear ones, increasing the entanglement density.
For concentrations above
≃ 10, the dependence on
is much weaker due to competing effects of increasing overlap and entanglements and reducing threading probability. The latter appears to dominate the strain-coupling, resulting in metrics generally decreasing (AF) or increasing (β, K) for
> 10. Again, highlighting the importance of threading, we observe a greater change in all metrics (AF, β, K) for
≳ 22 blends, which have immeasurably low ring content, compared to 10 ≲
< 22 blends that maintain a measurable fraction of rings. To summarize and more closely examine the correlations between the different metrics and their dependence on blend composition and distance, we evaluate pairings of scaling exponents and transport coefficients with their corresponding alignment factor for all distances (Fig. 6a) and compositions (Fig. 6b).
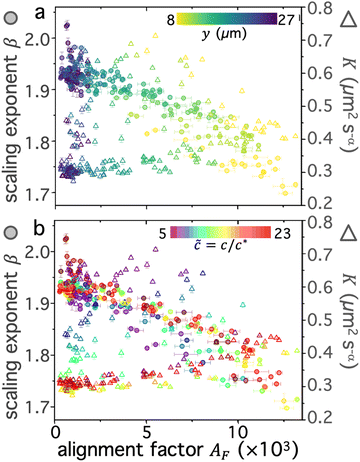 |
| Fig. 6 Superdiffusivity is strongly coupled to alignment while the rate of transport is maximized at intermediate alignment values. Scaling exponents β (left axis, translucent circles) and transport coefficients K (right axis, open triangles) plotted as functions of alignment factor AF for all blend compositions and vertical distances. Data points are colorized by (a) vertical distance y or (b) blend composition according to the corresponding colorscales. | |
As shown in Fig. 6a, the scaling exponent and alignment factor are generally inversely correlated for all distances, with the highest β and lowest AF values occurring at the largest distance yf = 27 μm and the lowest/highest β/AF occurring at y0 = 8 μm. This relation is consistent with the results discussed above. Conversely, K does not appear to be strongly correlated with alignment, but rather exhibits a large spread in values with the highest ones occurring at intermediate alignment.
To understand these results, we examine the dependence of
on correlations between these metrics (Fig. 6b). We find that the large spread in K values at intermediate alignment factors (Fig. 6a) appears to be a signature of low
blends (blue and purple triangles) that are weakly constrained and exhibit the weakest strain-coupling. These compositions likewise generally display higher (less subdiffusive) scaling exponents compared to higher
blends. This behavior is distinct from that for blends with
≳ 10, in which the transport coefficients generally increase and scaling exponents β decrease with increasing alignment. Additionally, we note that while all
≳ 10 blends display similar correlations of metrics,
max ≈ 10 blends have lower values of β and K values than for blends with
≳ 15 across the full range of measured alignment factors. Finally, we find that the large cluster of data points that exhibit diffusive (β ≈ 2), isotropic (AF ≈ 0) motion, which occurs at the largest y values (Fig. 6a), are predominantly from the highest
blends (dark red tones), which are comprised of primarily linear chains. This effect further corroborates our interpretation that the presence of rings, at even a very small fraction, substantially enhance long-range stress propagation and entropic stretching, in line with bulk rheology observations of even low fractions of rings enhancing viscosity of ring-linear blends.46
Conclusions
Topological polymer blends exhibit fascinating emergent properties that continue to be a topic of great interest and debate. The roles that threading of circular polymers by linear chains and other neighboring rings,34,36,38,43,44,60 and steric entanglements between and among circular and linear polymers,31,46,54,68 play in the emergent properties remain poorly understand. The extent to which supercoiling alters these roles has also been scarcely studied.41,64,69 Here, we shed important new light on these open questions by measuring the spatially resolved mechanical response of circular-linear DNA blends with high compositional resolution. We leverage time-controlled enzymatic linearization of circular and supercoiled DNA to resolve over 70 different topological compositions, which we delineate by a unique single quantity, the reduced concentration
, that captures the relative fractions of all three topologies: ring, supercoiled and linear.
We visualize the dynamics of DNA comprising blends in response to local strains, and quantify the extent to which the strain fingerprints onto the DNA dynamics. We identify this coupling as deviations from isotropic Brownian motion, which manifest in our analysis as alignment of DNA motion along the strain path, superdiffusivity, and substantial dependence of metrics on the distance from the strain path. We observe robust non-monotonic dependences of all strain-coupling metrics on blend composition, which show extrema at
≈ 10, which comprises ϕR ≈ 68%, ϕL ≈ 32%, and ϕS ≈ 0. We rationalize this emergent behavior as arising from increased constraints imposed by threadings that facilitate entropic stretching of circular polymers along the strain path.5,43,60,70 Our findings reveal intriguing new information regarding the optimization of topological blend composition for specific performance metrics; and have important implications in the design of materials that can couple efficiently to manufacturing processes via ample stretching and distribution of imposed stresses.
Methods
DNA preparation
We prepare solutions of double-stranded circular DNA of length 5.9 kbp (1.97 μm, N = 19.6) via replication of pYES2 plasmid constructs in Escherichia coli, alkaline lysis, and purification, following well-established protocols described previously.49 Following purification, we resuspend the DNA in nanopure deionized water and concentrate the solution using vacuum rotary evaporation to achieve a mass concentration of c ≃ 12 mg mL−1. Immediately following replication, the solution comprises purely supercoiled molecules (ϕS ≃ 1), but the subsequent purification process introduces nicks into a fraction of the supercoiled constructs, which allow the supercoils to unwind, resulting in relaxed circular (ring) topology. As such, the resulting DNA solution is a topological blend of ∼65% relaxed circular (ring) (ϕR ≃ 0.65), ∼35% supercoiled (ϕS ≃ 0.35), and ∼0% linear (ϕL ≃ 0) molecules (Fig. 1b and c). We quantify c and topological mass fraction ϕR,S,Lvia gel electrophoresis and band intensity analysis using Life Technologies E-Gel Imager and Gel Quant Express software.20
For all measurements, we dilute the stock DNA solution to c ≃ 6 mg mL−1 to match concentrations used in previous works20,28,45 and achieve sufficient degree of polymer coil overlap (Fig. 1d). To determine the degree of overlap we compute the coil overlap concentration by modifying the expression for the overlap concentration for monodisperse polymer solutions, c* = (3/4π)(M/NA)RG−3 where M is the DNA molecular weight, to account for the different coil sizes of the different topologies: c* = (3/4π)(M/NA)/(ϕLRG,L3 + ϕRRG,R3 + ϕSRG,S3).20,41 Using previously reported radius of gyration values of RG,S ≃ 103 nm, RG,R ≃ 113 nm and RG,L ≃ 179 nm for ring, supercoiled and linear topologies (Fig. 1a),20,50,52 we determine an initial overlap concentration of
. The initial reduced concentration
which provides a measure of the degree of polymer overlap, is
i ≈ 5.3.
At this initial concentration, we do not expect the DNA to be entangled, since it is below the nominal entanglement concentration
i ≈ 6.20,53 The longest relaxation timescale in this semidilute unentangled regime is the Rouse time, which we compute to be τR ≈ 2NRG2/πD0 ≈ 261 ms, where N = 19.6 and D0 ≃ 1.53 μm2 s−1 is the tracer diffusion coefficient.50,57 At the highest concentration of
i ≈ 23, in which the polymers are well entangled, the longest relaxation timescale is the disengagement time, which we compute to be τD ≈ 3ZτR ≈ 4.2 s, where Z ≃ (c/ce)5/4 ≃ 5 is the entanglement density.57,71
To image the blends during measurements, we fluorescent-label a small batch of the purified DNA with covalent dye MFP488 (MirusBio) that has excitation/emission peaks at 501/523 nm. We use the manufacturer-supplied Label IT Labeling Kit and corresponding protocols to label molecules at a dye to basepair ratio of 1:5.
Restriction endonuclease
We use the high-fidelity restriction endonuclease BamHI (New England BioLabs) to linearize circular DNA in solution. BamHI cleaves circular pYES2 constructs at a single recognition site, converting each ring and supercoiled molecule to linear topology. To ensure that we can achieve sufficient sampling across the entire composition space (from purely circular to purely linear), we perform measurements with two different BamHI:DNA stoichiometries: 0.1 U μg−1 and 1 U μg−1 (Fig. 1b and c).
Gel electrophoresis
To characterize the topological state of DNA solutions during linearization of circular DNA via BamHI, we use direct current agarose gel electrophoresis to separate the different topologies. Specifically, we prepare 40 μL samples of c ≃ 6 mg mL−1 DNA, 0.1% Tween, and either 0.1 U μg−1 or 1 U μg−1 BamHI, suspended in CutSmart Buffer (0.5 M potassium acetate, 0.2 M Tris–acetate, 0.1 M magnesium acetate; New England BioLabs), We incubate the samples at RT for 240 min, during which we remove a 1 μL aliquot from each reaction in regular intervals and quench the aliquot with TE buffer (100 mM Tris–HCl (pH 8), 1 mM EDTA) and gel loading dye. We load 50 ng of DNA from each ‘kinetic aliquot’ onto a 1% agarose gel prepared with TAE (Tris–acetate–EDTA) buffer.20,37 We run the gel at 5 V cm−1 for 2.5 hours, allowing for separation of the DNA into distinct bands corresponding to ring, supercoiled, and linear topologies (Fig. 1b). To quantify the fraction of each topology at each interval, we perform standard band intensity analysis as described above,20 using intensity as a proxy for mass to determine the relative DNA mass in each band of a given lane.
Sample preparation
For all optical tweezers experiments, we prepare samples as described above, and add 10 μg mL−1 of MFP488-labeled DNA and a trace amount of polystyrene beads of radius r = 2.25 μm (Polysciences, Inc.) (Fig. 2a and b). We coat beads with AlexaFluor594-BSA (ThermoFisher) to prevent DNA adsorption and allow for fluorescence imaging with different excitation/emission spectra than for DNA. Labeling beads and DNA tracers with spectrally distinct fluorophores ensures that the beads do not contribute to the DNA signal used in DDM analysis. To mitigate photobleaching, we add an oxygen-scavenging system comprising 45 μg mL−1 glucose, 43 μg mL−1 glucose oxidase, 7 μg mL−1 catalase, and 5 μg mL−1 β-mercaptoethanol.
We mix the solution by pipetting up and down with a wide-bore pipet tip, and add BamHI last, marking the time at which it is added as t = 0. The buffer conditions and temperature (20 °C) provide good solvent conditions for the DNA.71–75 We construct sample chambers, measuring 20 × 3 × 0.1 mm3, using a microscope glass slide and coverslip, both coated with BSA to prevent DNA adsorption, and separated by two layers of double-sided tape. We introduce DNA samples into chambers via capillary action, using a wide-bore pipette tip, after which we seal chambers with epoxy.
OpTiDDM instrumentation and measurements
We use a custom-built optical trap formed from a 1064 nm Nd:YAG fiber laser (Manlight) focused with a 60× 1.4 NA objective (Olympus) and integrated into an Olympus IX71 epifluorescence microscope, as described previously.58,59 To image the MPF488-labeled DNA and AlexaFluor594-labeled microspheres in the samples we use 490/525 nm and 530/575 nm excitation/emission filter cubes and an ORCA-Flash 4.0 LT+ CMOS camera (Hamamatsu). To impose a local strain in the sample, we use a piezoelectric actuator mirror (PI USA) to move the trap relative to the sample chamber while keeping the 554 × 128 square-pixel (16.6 μm × 72 μm) field-of-view (FOV) that we use for DDM analysis fixed and centered at the resting trap position.45
As shown in Fig. 2b, the microrheological strain program we apply consists of repeatedly sweeping the trapped bead back and forth horizontally (along the x-axis) at constant speed through a strain distance s = 15 μm. We pause between each 15 μm sweep for a fixed cessation time of 3 s to allow the polymers to relax. We perform all measurements at a speed of v = 40 μm s−1, which equates to a strain rate of
= 42 s−1via the relation
.45,76 We chose this speed based on our previous work that showed that this rate allowed for the most pronounced strain coupling in comparable DNA solutions.45 For reference, the corresponding Weissenberg numbers for the initial and final concentrations (
i ≈ 5,
i ≈ 23) are Wi ≈
τR ≈ 11 and Wi ≈
τD ≈ 176. Considering that the time for the probe to complete one 15 μm strain is tp = 0.33 s, the corresponding Deborah numbers for these two extremal concentrations are De = τR/tp ≈ 0.8 and De = τD/tp ≈ 12.6. Therefore, we expect to be in the nonlinear regime and to be probing the viscoelastic response of the polymers.
We perform each oscillatory strain measurement for 50 s, during which we capture a time-series of images of the labeled DNA in the sample at 60 fps (Fig. 2b–e). We perform measurements every ∼1–10 min, depending on the digestion rate, for 4 hours, resulting in >80 measurements that are each performed with a new particle in a new location in the sample chamber, separated by >200 μm from the previous location. All data shown is the average across at least two replicates and three consecutive measurements. Vertical and horizontal error bars are standard error from averaging across replicates and consecutive measurements, respectively.
Differential dynamic microscopy (DDM)
As previously described in detail,45 to determine how the strain-induced DNA dynamics depend on the orthogonal distance y from the strain path, we divide the 554 × 128 square-pixel FOV into 128 × 128 square-pixel (16.6 μm)2 ROIs, centered horizontally at the midpoint of the s = 15 μm strain path and shifted along the ±y direction in 16-pixel increments, with the bottom edge of the first ROI at y = 0 (and its center at y0 = 8 μm) and the farthest ROI centered at yf = 27 μm. We analyze 10 ROIs in each of the +y and −y directions and average the ± data for each y, as they exhibit expectedly statistically indistinguishable dynamics.
We use custom-written scripts (Python) to perform DDM analysis, which takes two-dimensional Fourier transforms of differences between images, separated by a range of lag times Δt, to quantify how the correlation of density fluctuations decays with Δt.67 We quantify this correlation as a function of the 2D wave vector
= (qx, qy) via the image structure function D(
, Δt) (Fig. 2e and f).
To determine the extent to which the DNA dynamics are preferentially aligned along the strain path (x-axis) (Fig. 2 and 3), we compute an alignment factor AF with respect to the strain path (x-axis) by computing weighted azimuthal integrals of D(qx, qy, Δt), i.e., integrals over θ where θ = tan−1(qy/qx):
(Fig. 2g).65,66 Here, θ is defined relative to the x-axis such that isotropic and completely x-aligned dynamics correspond to AF = 0 and AF = A(q)/(A(q) + 2B(q)), respectively, where A(q) and B(q) are amplitude and background terms that we determine from DDM analysis, as described below. Larger AF values indicate more alignment. To obtain a single AF value for each distance y, we average over Δt = 0.17–1 s and q = 1–7 μm−1, where there is no statistically significant dependence of AF on these parameters.
To determine the type and rate of motion of the DNA, we radially average each D(
, Δt) (Fig. 2f) to get a 1D image structure function that can be described by D(q, Δt) = A(q)[1 − f(q, Δt)] + B(q), where f(q, Δt) is the intermediate scattering function (ISF). We model the ISF as a stretched exponential: f(q, Δt) = e−(Δt/τ(q))δ where τ(q) is the decay time and δ the stretching exponent.
By evaluating the functional form of τ(q) determined from fitting the ISF, we analyze the extent to which τ(q) can be described by power-law scaling τ(q) ∼ q−β where the scaling exponent β describes the type of motion (Fig. 2h, 4 and 5). Specifically, β = 2 and β = 1 are indicative of diffusive and ballistic motion, respectively, and 1 < β < 2 indicates superdiffusion.16,77,78
Author contributions
RMRA conceived the project, guided the experiments, interpreted the data, wrote the manuscript and prepared figures. KRP performed the experiments, analyzed and interpreted the data, and prepared figures. RJM developed analysis software and helped guide experiments and analysis and write the manuscript.
Data availability
All data shown in the manuscript is provided in ESI.† Raw data from which the presented data was determined will be made available upon reasonable request to the corresponding author.
Conflicts of interest
The authors declare no competing interests.
Acknowledgements
This research was funded by grants from the Air Force Office of Scientific Research (AFOSR-FA9550-17-1-0249, AFOSR-FA9550-21-1-0361) and National Science Foundation (DMREF DMR 2119663) awarded to RMRA, and National Institutes of Health (R15GM123420, 2R15GM123420-02) awarded to RJM and RMRA.
References
- G. S. Grest, T. Ge, S. J. Plimpton, M. Rubinstein and T. C. O’Connor, Entropic Mixing of Ring/Linear Polymer Blends, ACS Polym. Au, 2023, 3(2), 209–216, DOI:10.1021/acspolymersau.2c00050.
- Y. Kobayashi, Y. Doi, S. S. Abdul Rahman, E. Kim, T.-H. Kim, A. Takano and Y. Matsushita, SANS Study of Ring Topology Effects on the Miscibility of Polymer Blends, Macromolecules, 2018, 51(5), 1885–1893, DOI:10.1021/acs.macromol.7b02359.
-
Z. Bartczak and A. Galeski, Mechanical Properties of Polymer Blends, in Polymer Blends Handbook, ed. L. A. Utracki and C. A. Wilkie, Springer Netherlands, Dordrecht, 2014, pp. 1203–1297 DOI:10.1007/978-94-007-6064-6_13.
-
S. H. Goh, Miscible Polymer Blends, in Polymer Blends Handbook, ed. L. A. Utracki and C. A. Wilkie, Springer Netherlands, Dordrecht, 2014, pp. 1915–2151 DOI:10.1007/978-94-007-6064-6_24.
- T. C. O’Connor, T. Ge and G. S. Grest, Composite Entanglement Topology and Extensional Rheology of Symmetric Ring-Linear Polymer Blends, J. Rheol., 2022, 66(1), 49–65, DOI:10.1122/8.0000319.
- E. Lattuada, T. Pietrangeli and F. Sciortino, Interpenetrating Gels in Binary Suspensions of DNA Nanostars, J. Chem. Phys., 2022, 157(13), 135101, DOI:10.1063/5.0117047.
-
S. Thomas, D. Durand, C. Chassenieux and P. Jyotishkumar, Handbook of Biopolymer-Based Materials: From Blends and Composites to Gels and Complex Networks, John Wiley & Sons, 2013 Search PubMed.
- S. Banerjee, M. L. Gardel and U. S. Schwarz, The Actin Cytoskeleton as an Active Adaptive Material, Annu. Rev. Condens. Matter Phys., 2020, 11(1), 421–439, DOI:10.1146/annurev-conmatphys-031218-013231.
- M. Drechsler, F. Giavazzi, R. Cerbino and I. M. Palacios, Active Diffusion and Advection in the Drosophila Ooplasm Result from the Interplay of the Actin and Microtubule Cytoskeletons, bioRxiv, 2017, 7, 098590, DOI:10.1101/098590.
- D. A. Fletcher and R. D. Mullins, Cell Mechanics and the Cytoskeleton, Nature, 2010, 463(7280), 485–492, DOI:10.1038/nature08908.
- O. Wintner, N. Hirsch-Attas, M. Schlossberg, F. Brofman, R. Friedman, M. Kupervaser, D. Kitsberg and A. Buxboim, A Unified Linear Viscoelastic Model of the Cell Nucleus Defines the Mechanical Contributions of Lamins and Chromatin, Adv. Sci., 2020, 7(8), 1901222, DOI:10.1002/advs.201901222.
- F. Erdel, M. Baum and K. Rippe, The Viscoelastic Properties of Chromatin and the Nucleoplasm Revealed by Scale-Dependent Protein Mobility, J. Phys.: Condens. Matter, 2015, 27(6), 064115, DOI:10.1088/0953-8984/27/6/064115.
- C.-H. Yu, S. Redemann, H.-Y. Wu, R. Kiewisz, T. Y. Yoo, W. Conway, R. Farhadifar, T. Müller-Reichert and D. Needleman, Central-Spindle Microtubules Are Strongly Coupled to Chromosomes during Both Anaphase A and Anaphase B, Mol. Biol. Cell, 2019, 30(19), 2503–2514, DOI:10.1091/mbc.E19-01-0074.
- W. Alt and M. Dembo, Cytoplasm Dynamics and Cell Motion: Two-Phase Flow Models, Math. Biosci., 1999, 156(1), 207–228, DOI:10.1016/S0025-5564(98)10067-6.
- E. H. Barriga and R. Mayor, Adjustable Viscoelasticity Allows for Efficient Collective Cell Migration, Semin. Cell Dev. Biol., 2019, 93, 55–68, DOI:10.1016/j.semcdb.2018.05.027.
- R. Cerbino and P. Cicuta, Perspective: Differential Dynamic Microscopy Extracts Multi-Scale Activity in Complex Fluids and Biological Systems, J. Chem. Phys., 2017, 147(11), 110901, DOI:10.1063/1.5001027.
-
B. Alberts, Molecular Biology of the Cell, Garland, 2004 Search PubMed.
- Y. S. Kim, B. Kundukad, A. Allahverdi, L. Nordensköld, P. S. Doyle and J. R. C. van der Maarel, Gelation of the Genome by Topoisomerase
II Targeting Anticancer Agents, Soft Matter, 2013, 9(5), 1656–1663, 10.1039/C2SM27229F.
- M. Jinek, K. Chylinski, I. Fonfara, M. Hauer, J. A. Doudna and E. Charpentier, A Programmable Dual-RNA–Guided DNA Endonuclease in Adaptive Bacterial Immunity, Science, 2012, 337(6096), 816–821, DOI:10.1126/science.1225829.
- D. Michieletto, P. Neill, S. Weir, D. Evans, N. Crist, V. A. Martinez and R. M. Robertson-Anderson, Topological Digestion Drives Time-Varying Rheology of Entangled DNA Fluids, Nat. Commun., 2022, 13(1), 4389, DOI:10.1038/s41467-022-31828-w.
- A. Pingoud, G. G. Wilson and W. Wende, Type II Restriction Endonucleases—a Historical Perspective and More, Nucleic Acids Res., 2014, 42(12), 7489–7527, DOI:10.1093/nar/gku447.
- O. A. Saleh, B. Jeon and T. Liedl, Enzymatic Degradation of Liquid Droplets of DNA Is Modulated near the Phase Boundary, Proc. Natl. Acad. Sci. U. S. A., 2020, 117(28), 16160–16166, DOI:10.1073/pnas.2001654117.
- L.-Y. Zhou, J. Fu and Y. He, A Review of 3D Printing Technologies for Soft Polymer Materials, Adv. Funct. Mater., 2020, 30(28), 2000187, DOI:10.1002/adfm.202000187.
- D. Kokkinis, F. Bouville and A. R. Studart, 3D Printing of Materials with Tunable Failure via Bioinspired Mechanical Gradients, Adv. Mater., 2018, 30(19), 1705808, DOI:10.1002/adma.201705808.
-
T. G. Mezger, The Rheology Handbook, Vincentz Network, 2006 Search PubMed.
- C. L. C. Chan, J. M. Taylor and E. C. Davidson, Design of Soft Matter for Additive Processing, Nat. Synth., 2022, 1(8), 592–600, DOI:10.1038/s44160-022-00115-3.
- F. M. Haque and S. M. Grayson, The Synthesis, Properties and Potential Applications of Cyclic Polymers, Nat. Chem., 2020, 12(5), 433–444, DOI:10.1038/s41557-020-0440-5.
- P. Neill, N. Crist, R. McGorty and R. Robertson-Anderson, Enzymatic Cleaving of Entangled DNA Rings Drives Scale-Dependent Rheological Trajectories, Soft Matter, 2024, 20(12), 2750–2766, 10.1039/D3SM01641B.
- D. Kong, S. Banik, M. J. San Francisco, M. Lee, R. M. Robertson Anderson, C. M. Schroeder and G. B. McKenna, Rheology of Entangled Solutions of Ring–Linear DNA Blends, Macromolecules, 2022, 55(4), 1205–1217, DOI:10.1021/acs.macromol.1c01672.
- G. B. McKenna, D. Chen, S. C. H. Mangalara, D. Kong and S. Banik, Some Open Challenges in Polymer Physics, Polym. Eng. Sci., 2022, 62(5), 1325–1355, DOI:10.1002/pen.25938.
- M. A. Ubertini, J. Smrek and A. Rosa, Entanglement Length Scale Separates Threading from Branching of Unknotted and Non-Concatenated Ring Polymers in Melts, Macromolecules, 2022, 55(23), 10723–10736, DOI:10.1021/acs.macromol.2c01264.
- W. J. Weigand, A. Messmore, J. Tu, A. Morales-Sanz, D. L. Blair, D. D. Deheyn, J. S. Urbach and R. M. Robertson-Anderson, Active Microrheology Determines Scale-Dependent Material Properties of Chaetopterus Mucus, PLoS One, 2017, 12(5), e0176732, DOI:10.1371/journal.pone.0176732.
- J. Roovers and P. M. Toporowski, Microheterogeneity in Miscible Blends of 1,2-Polybutadiene and 1,4-Polyisoprene, Macromolecules, 1992, 25(13), 3454–3461, DOI:10.1021/ma00039a023.
- D. Michieletto, N. Nahali and A. Rosa, Glassiness and Heterogeneous Dynamics in Dense Solutions of Ring Polymers, Phys. Rev. Lett., 2017, 119(19), 197801, DOI:10.1103/PhysRevLett.119.197801.
- D. Michieletto and T. Sakaue, Dynamical Entanglement and Cooperative Dynamics in Entangled Solutions of Ring and Linear Polymers, ACS Macro Lett., 2021, 10(1), 129–134, DOI:10.1021/acsmacrolett.0c00551.
- Y. Zhou, C. D. Young, M. Lee, S. Banik, D. Kong, G. B. McKenna, R. M. Robertson-Anderson, C. E. Sing and C. M. Schroeder, Dynamics and Rheology of Ring-Linear Blend Semidilute Solutions in Extensional Flow: Single Molecule Experiments, J. Rheol., 2021, 65(4), 729–744, DOI:10.1122/8.0000219.
- J. Marfai, R. J. McGorty and R. M. Robertson-Anderson, Cooperative Rheological State-Switching of Enzymatically-Driven Composites of Circular DNA And Dextran, Adv. Mater., 2023, 35(46), 2305824, DOI:10.1002/adma.202305824.
- J. Smrek, K. Kremer and A. Rosa, Threading of Unconcatenated Ring Polymers at High Concentrations: Double-Folded vs Time-Equilibrated Structures, ACS Macro Lett., 2019, 8(2), 155–160, DOI:10.1021/acsmacrolett.8b00828.
- D. G. Tsalikis and V. G. Mavrantzas, Size and Diffusivity of Polymer Rings in Linear Polymer Matrices: The Key Role of Threading Events, Macromolecules, 2020, 53(3), 803–820, DOI:10.1021/acs.macromol.9b02099.
- D. Parisi, M. Kaliva, S. Costanzo, Q. Huang, P. J. Lutz, J. Ahn, T. Chang, M. Rubinstein and D. Vlassopoulos, Nonlinear Rheometry of Entangled Polymeric Rings and Ring-Linear Blends, J. Rheol., 2021, 65(4), 695–711, DOI:10.1122/8.0000186.
- R. Peddireddy K., M. Lee, Y. Zhou, S. Adalbert, S. Anderson, M. Schroeder C. and M. Robertson-Anderson, R., Unexpected Entanglement Dynamics in Semidilute Blends of Supercoiled and Ring DNA, Soft Matter, 2020, 16(1), 152–161, 10.1039/C9SM01767D.
- K. R. Peddireddy, M. Lee, C. M. Schroeder and R. M. Robertson-Anderson, Viscoelastic Properties of Ring-Linear DNA Blends Exhibit Nonmonotonic Dependence on Blend Composition, Phys. Rev. Res., 2020, 2(2), 023213, DOI:10.1103/PhysRevResearch.2.023213.
- K. R. Peddireddy, R. Clairmont and R. M. Robertson-Anderson, Polymer Threadings and Rigidity Dictate the Viscoelasticity of Entangled Ring-Linear Blends and Their Composites with Rigid Rod Microtubules, J. Rheol., 2023, 67(1), 125–138, DOI:10.1122/8.0000529.
- A. F. Katsarou, A. J. Tsamopoulos, D. G. Tsalikis and V. G. Mavrantzas, Dynamic Heterogeneity in Ring-Linear Polymer Blends, Polymers, 2020, 12(4), 752, DOI:10.3390/polym12040752.
- K. R. Peddireddy, R. Clairmont, P. Neill, R. McGorty and R. M. Robertson-Anderson, Optical-Tweezers-Integrating-Differential-Dynamic-Microscopy Maps the Spatiotemporal Propagation of Nonlinear Strains in Polymer Blends and Composites, Nat. Commun., 2022, 13(1), 5180, DOI:10.1038/s41467-022-32876-y.
- D. Parisi, J. Ahn, T. Chang, D. Vlassopoulos and M. Rubinstein, Stress Relaxation in Symmetric Ring-Linear Polymer Blends at Low Ring Fractions, Macromolecules, 2020, 53(5), 1685–1693, DOI:10.1021/acs.macromol.9b02536.
- J. D. Halverson, G. S. Grest, A. Y. Grosberg and K. Kremer, Rheology of Ring Polymer Melts: From Linear Contaminants to Ring-Linear Blends, Phys. Rev. Lett., 2012, 108(3), 038301, DOI:10.1103/PhysRevLett.108.038301.
- P. Khanal, K. R. Peddireddy, J. Marfai, R. McGorty and R. M. Robertson-Anderson, DNA Topology Dictates Emergent Bulk Elasticity and Hindered Macromolecular Diffusion in DNA-Dextran Composites, J. Rheol., 2022, 66(4), 699–715, DOI:10.1122/8.0000447.
- S. Laib, R. M. Robertson and D. E. Smith, Preparation and Characterization of a Set of Linear DNA Molecules for Polymer Physics and Rheology Studies, Macromolecules, 2006, 39(12), 4115–4119, DOI:10.1021/ma0601464.
- R. M. Robertson, S. Laib and D. E. Smith, Diffusion of Isolated DNA Molecules: Dependence on Length and Topology, Proc. Natl. Acad. Sci. U. S. A., 2006, 103(19), 7310–7314, DOI:10.1073/pnas.0601903103.
- D. E. Smith, T. T. Perkins and S. Chu, Dynamical
Scaling of DNA Diffusion Coefficients, Macromolecules, 1996, 29(4), 1372–1373, DOI:10.1021/ma951455p.
- D. R. Latulippe and A. L. Zydney, Radius of Gyration of Plasmid DNA Isoforms from Static Light Scattering, Biotechnol. Bioeng., 2010, 107(1), 134–142, DOI:10.1002/bit.22787.
- R. M. Robertson and D. E. Smith, Self-Diffusion of Entangled Linear and Circular DNA Molecules: Dependence on Length and Concentration, Macromolecules, 2007, 40(9), 3373–3377 CrossRef CAS.
- T. Ge, S. Panyukov and M. Rubinstein, Self-Similar Conformations and Dynamics in Entangled Melts and Solutions of Nonconcatenated Ring Polymers, Macromolecules, 2016, 49(2), 708–722, DOI:10.1021/acs.macromol.5b02319.
- R. M. Robertson and D. E. Smith, Direct Measurement of the Confining Forces Imposed on a Single Molecule in a Concentrated Solution of Circular Polymers, Macromolecules, 2007, 40(24), 8737–8741, DOI:10.1021/ma071440e.
- A. Y. Grosberg, Annealed Lattice Animal Model and Flory Theory for the Melt of Non-Concatenated Rings: Towards the Physics of Crumpling, Soft Matter, 2013, 10(4), 560–565, 10.1039/C3SM52805G.
-
M. Doi and S. F. Edwards, The Theory of Polymer Dynamics, Clarendon Press, 1988 Search PubMed.
- C. D. Chapman and R. M. Robertson-Anderson, Nonlinear Microrheology Reveals Entanglement-Driven Molecular-Level Viscoelasticity of Concentrated DNA, Phys. Rev. Lett., 2014, 113(9), 098303, DOI:10.1103/PhysRevLett.113.098303.
- R. M. Robertson-Anderson, Optical Tweezers Microrheology: From the Basics to Advanced Techniques and Applications, ACS Macro Lett., 2018, 7(8), 968–975, DOI:10.1021/acsmacrolett.8b00498.
- Q. Huang, J. Ahn, D. Parisi, T. Chang, O. Hassager, S. Panyukov, M. Rubinstein and D. Vlassopoulos, Unexpected Stretching of Entangled Ring Macromolecules, Phys. Rev. Lett., 2019, 122(20), 208001, DOI:10.1103/PhysRevLett.122.208001.
- R. Cerbino, F. Giavazzi and M. E. Helgeson, Differential Dynamic Microscopy for the Characterization of Polymer Systems, J. Polym. Sci., 2022, 60(7), 1079–1089, DOI:10.1002/pol.20210217.
- R. Cerbino and V. Trappe, Differential Dynamic Microscopy: Probing Wave Vector Dependent Dynamics with a Microscope, Phys. Rev. Lett., 2008, 100(18), 188102, DOI:10.1103/PhysRevLett.100.188102.
- D. M. Wulstein, K. E. Regan, R. M. Robertson-Anderson and R. McGorty, Light-Sheet Microscopy with Digital Fourier Analysis Measures Transport Properties over Large Field-of-View, Opt. Express, 2016, 24(18), 20881–20894, DOI:10.1364/OE.24.020881.
- J. Smrek, J. Garamella, R. Robertson-Anderson and D. Michieletto, Topological Tuning of DNA Mobility in Entangled Solutions of Supercoiled Plasmids, Sci. Adv., 2021, 7(20), eabf9260, DOI:10.1126/sciadv.abf9260.
- L. M. Walker and N. J. Wagner, SANS Analysis of the Molecular Order in Poly(γ-Benzyl l-Glutamate)/Deuterated Dimethylformamide (PBLG/d-DMF) under Shear and during Relaxation, Macromolecules, 1996, 29(6), 2298–2301, DOI:10.1021/ma951127p.
- Z. Varga and J. W. Swan, Large Scale Anisotropies in Sheared Colloidal Gels, J. Rheol., 2018, 62(2), 405–418, DOI:10.1122/1.5003364.
- H. N. Verwei, G. Lee, G. Leech, I. I. Petitjean, G. H. Koenderink, R. M. Robertson-Anderson and R. J. McGorty, Quantifying Cytoskeleton Dynamics Using Differential Dynamic Microscopy, JoVE, 2022, 184, e63931, DOI:10.3791/63931.
- J. Wang and T. Ge, Crazing Reveals an Entanglement Network in Glassy Ring Polymers, Macromolecules, 2021, 54(16), 7500–7511, DOI:10.1021/acs.macromol.1c01080.
- B. A. Krajina and A. J. Spakowitz, Large-Scale Conformational Transitions in Supercoiled DNA Revealed by Coarse-Grained Simulation, Biophys. J., 2016, 111(7), 1339–1349, DOI:10.1016/j.bpj.2016.07.045.
- T. C. O’Connor, T. Ge, M. Rubinstein and G. S. Grest, Topological Linking Drives Anomalous Thickening of Ring Polymers in Weak Extensional
Flows, Phys. Rev. Lett., 2020, 124(2), 027801, DOI:10.1103/PhysRevLett.124.027801.
- S. Banik, D. Kong, M. J. San Francisco and G. B. McKenna, Monodisperse Lambda DNA as a Model to Conventional Polymers: A Concentration-Dependent Scaling of the Rheological Properties, Macromolecules, 2021, 54(18), 8632–8654, DOI:10.1021/acs.macromol.0c02537.
- D. Michieletto, P. Neill, S. Weir, D. Evans, N. Crist, V. A. Martinez and R. M. Robertson-Anderson, Topological Digestion Drives Time-Varying Rheology of Entangled DNA Fluids, Nat. Commun., 2022, 13(1), 4389, DOI:10.1038/s41467-022-31828-w.
- R. M. Robertson, S. Laib and D. E. Smith, Diffusion of Isolated DNA Molecules: Dependence on Length and Topology, Proc. Natl. Acad. Sci. U. S. A., 2006, 103(19), 7310–7314, DOI:10.1073/pnas.0601903103.
- S. Pan, D. At Nguyen, T. Sridhar, P. Sunthar and J. Ravi Prakash, Universal Solvent Quality Crossover of the Zero Shear Rate Viscosity of Semidilute DNA Solutions, J. Rheol., 2014, 58(2), 339–368, DOI:10.1122/1.4861072.
- J. R. Prakash, Universal Dynamics of Dilute and Semidilute Solutions of Flexible Linear Polymers, Curr. Opin. Colloid Interface Sci., 2019, 43, 63–79, DOI:10.1016/j.cocis.2019.03.001.
- T. M. Squires and T. G. Mason, Fluid Mechanics of Microrheology, Annu. Rev. Fluid Mech., 2010, 42(1), 413–438, DOI:10.1146/annurev-fluid-121108-145608.
- Y. Gao, J. Kim and M. E. Helgeson, Microdynamics and Arrest of Coarsening during Spinodal Decomposition in Thermoreversible Colloidal Gels, Soft Matter, 2015, 11(32), 6360–6370, 10.1039/C5SM00851D.
- D. Germain, M. Leocmach and T. Gibaud, Differential Dynamic Microscopy to Characterize Brownian Motion and Bacteria Motility, Am. J. Phys., 2016, 84(3), 202–210, DOI:10.1119/1.4939516.
|
This journal is © The Royal Society of Chemistry 2024 |
Click here to see how this site uses Cookies. View our privacy policy here.