DOI:
10.1039/D4RA02310B
(Paper)
RSC Adv., 2024,
14, 23392-23403
Low-cost precision agriculture for sustainable farming using paper-based analytical devices†
Received
26th March 2024
, Accepted 11th July 2024
First published on 25th July 2024
Abstract
The United Nations estimates that by 2030, agricultural production must increase by 70% to meet food demand. Precision agriculture (PA) optimizes production through efficient resource use, with soil fertility being crucial for nutrient supply. Traditional nutrient quantification methods are costly and time-consuming. This study introduces a rapid (15 min), user-friendly, paper-based platform for determining four essential macronutrients—nitrate, magnesium, calcium, and ammonium—using colorimetric methods and a smartphone for data reading and storage. The sensor effectively detects typical soil nutrient concentrations, showing strong linearity and adequate detection limits. For nitrate, the RGB method resulted in an R2 of 0.992, a detection range of 0.5 to 10.0 mmol L−1, and an LOD of 0.299 mmol L−1. Calcium quantification using grayscale displayed an R2 of 0.993, a detection range of 2.0 to 6.0 mmol L−1, and an LOD of 0.595 mmol L−1. Magnesium was best quantified using the hue color space, with an R2 of 0.999, a detection range of 1.0 to 6.0 mmol L−1, and an LOD of 0.144 mmol L−1. Similarly, ammonium detection using the hue color space had an R2 of 0.988, a range of 0.5 to 2.5 mmol L−1, and an LOD of 0.170 mmol L−1. This device enhances soil fertility assessment accessibility, supporting PA implementation and higher food production.
1 Introduction
The United Nations (UN) projects a global population surge to 9.7 billion people by 2050 – a considerable increase from current numbers.1 This population growth will require a 70% increase in food production until 2050 to meet the world's demand.2 This herculean challenge is aggravated by the continuous decrease in arable landing per capita, resource scarcity, and climate change impacts, capable of reducing the agricultural productivity by 40%.2,3 Therefore, traditional agricultural expansion is no longer sufficient, intensifying the need for sustainable methods to enhance food security without compromising environmental integrity or the future generation demands.4–6
Precision agriculture (PA), as defined by The International Society of Precision Agriculture, utilizes detailed data collection and analysis to optimize resource use, increase productivity, and ensure sustainability.7 Given that smallholders and family farms produce approximately 70–80% of the world's food,8 precision agriculture can lead these farmers in improving their food production capacity by offering a better tool for agricultural decisions and output sustainably.7
Notwithstanding, spatial and temporal soil variability is a critical parameter addressed by precision agriculture measurements.9 Soil monitoring can improve crop yield and the capacity of farmers to cultivate nutritious food.10 Soil formation involves complex biochemical and physical processes, giving rise to different horizons according to its composition.11,12 Indeed, the soil is a dynamic natural system composed of minerals, organic matter, living organisms, gas, and water.11 The water retained in the pores or empty spaces of the soil is called soil solution and is a source of nutrient uptake for plant roots.13 Therefore, the concentration of nutrients in the soil solution is critical for the supply of nutrients to plant roots.
Conventional soil analysis involves complex protocols and requires many chemicals, expensive instruments, and trained personnel, is time-consuming, and can generate divergent results depending on the chosen method.7,14,15 Researchers have been developing electrochemical and optical on-the-go soil sensors to measure chemical properties. These sensors monitor soil pH and individual ions (nitrate, phosphate, and potassium) but require external equipment and electricity.16,17 To date, there are few commercially available portable sensors for the analysis of nutrients in the soil and these usually require specific equipment for measurements.18,19
Paper-based analytical devices (μPADs) provide a user-friendly and portable platform for in-field analysis. These devices require lower sample volumes, in the order of microliters, and can be easily disposed by incineration or recycled.20,21 One common way to detect analytes within this platform is by colorimetry, which relies on measuring the color intensity produced by a reaction between the analyte and a specific molecule.22–25 The color intensity of the product then correlates to the concentration of the analyte.24 This detection method provides rapid and straightforward results, being appropriate to use in remote areas.26 Digital imaging has been widely used in the reading of point-of-care tests. Devices such as scanners, cameras, and smartphones can register and process optical signals, enabling the quantification of colored images and providing a fast, intuitive, and cost-effective platform for real-time analysis.27,28 This commonly available technology can provide more accurate and reproducible results for precision agriculture, and its connectivity allows storing and sharing of data, which enables real-time mapping of the soil conditions.6,29,30
This work presents a low-cost platform for the semi-quantification of essential nutrients in the soil. We developed a paper-based microfluidic device containing hydrophobic patterns made with wax to create different channels for the simultaneous detection of analytes. The analytes were detected through colorimetry and quantified using a smartphone. Here, we aim to offer a simple platform for farmers and agronomists to monitor soil micronutrients (ammonium, calcium, magnesium, and nitrogen) to address the plant's needs and support real-time decisions precisely.
2 Materials and methods
2.1 Materials
Chromatography paper Whatman® #1 (Whatman, United Kingdom), wax printer Xerox® Phaser ColorQube 8570 (Xerox Corporation, United States), Cartridge-free Colorqube black ink (8870 series, Xerox Corporation, United States), oven 404-3D (Nova Ética, Brazil). Ultrapure water Milli-Q®, smartphone iPhone 8 Plus (Apple Inc., United States). The chemicals sulfanilamide, N-(1-naphthyl) ethylenediamine hydrochloride, (NED) hydrochloric acid 37%, citric acid, metallic zinc in powder, sodium nitrate, murexide, calcium chloride dihydrate, xylidyl blue, ethylene-bis(oxyethylenenitrilo)tetraacetic acid (EGTA), sodium hydroxide, eriochrome black T (EBT) indicator, magnesium chloride hexahydrate, hypochlorite, sodium salicylate, sodium nitroprusside, ammonium chloride were purchased from Sigma-Aldrich (Brazil).
2.2 Design and fabrication of microfluidic paper-based analytical device (μPAD)
Circles with 5 mm diameter were created in AutoCAD 2021 software (Autodesk, United States). The patterns were printed on chromatographic paper by the wax printing method. Then, the printed papers were baked in an oven at 110 °C for 1 min (Fig. 1A). It is important to notice that the tests were designed to be independent so that each final user can determine the ideal combination of analysis for their application. The use of the methods described here with subsequent designs have the potential to join 2 or more macronutrient analysis with no significant interference.
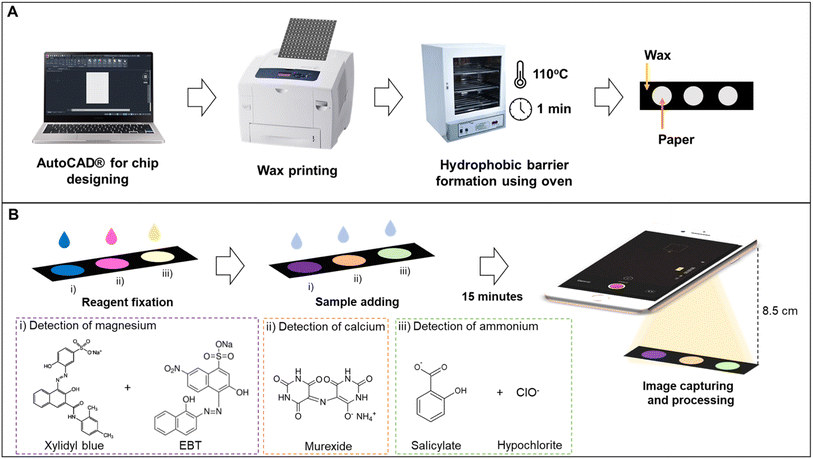 |
| Fig. 1 μPAD manufacturing process. (A) μPAD fabrication: patterns were drawn in AutoCAD software. The wax was then deposited on Whatman #1 chromatographic paper by the wax printing method. When placed in an oven at 110 °C for 1 min, the printed paper allows the wax to melt and permeate the paper. (B) Reagents immobilization: firstly, the reagents for magnesium (xylidyl blue and EBT), calcium (murexide), and ammonium (salicylate and hypochlorite) detection were added to the paper. Then the sample was added to each spot and filmed 15 min later. | |
2.3 Image acquisition and treatment
The detection zones were captured with an iPhone 8 (Apple Inc., United States) in video mode using the smartphone's flash as a light source. A fixed distance of 8.5 cm was used between the smartphone and the device. The images of the detection zones were extracted from the videos and analyzed in the open-source software ColorScan.31 The intensity values of each component of the RGB, HSV, CIELab color spaces, and grayscale were analyzed. It is essential to notice that the images were always obtained with the detection zones placed in the upper left side of the video, the region where the flash is more intense.
The correlation between the intensity of the individual components of the RGB, HSV, and CIELab color spaces and the concentration of each analyte generated the analytical curves for each analyte. For RGB, the magnitude of the vector between the white coordinates (Ro, Go, Bo) and the test coordinate containing the analyte (Rt, Gt, Bt) was also considered, as shown in eqn (1).32
|
 | (1) |
2.4 Nutrient detection
2.4.1 Calcium. A 0.2% solution of murexide was prepared in Milli-Q® water. To prepare the device, 1 μL of the murexide solution was added to the detection zone and allowed to dry for 5 min.33 A 20 mmol L−1 calcium stock solution was prepared by dissolving calcium chloride dihydrate in Milli-Q® water. The solutions were prepared by diluting standards to concentrations ranging from 0.1 to 5.0 mmol L−1. For detection, 1.4 μL of the sample was added to the detection zone and allowed to react for 15 min prior video acquisition (Fig. 1B). All experiments were performed in triplicate.
2.4.2 Magnesium. Two indicators were used to detect magnesium (Fig. 1B); the first consisted of a solution containing 110 μmol L−1 of xylidyl blue, 160 μmol L−1 of EGTA, and 1 mol L−1 of ethanolamine, prepared in Milli-Q® water (solution AMg). Then, the volume taken was adjusted to achieve the desired final concentration. The second indicator consisted of a solution of EBT, at 20 mg mL−1, prepared in ethanol (solution BMg).34A 20 mmol L−1 stock solution of magnesium was prepared by dissolving magnesium chloride hexahydrate in Milli-Q® water. The working range for preparing the dilutions was 0.1 to 5.0 mmol L−1. For the immobilization of reagents, 0.4 μL of solution AMg and 0.1 μL of solution BMg were added to the detection zone, waiting for the complete drying of the first solution before adding the second. For the test, 1.4 μL of the sample was added, and 15 min later, the videos were acquired (Fig. 1B). All experiments were performed in triplicate.
2.4.3 Ammonium. Ammonium was detected through the salicylate method.35 A solution was prepared to contain 0.5 mL of hypochlorite (11.1 mol L−1) and 0.25 g (0.125 mol L−1) of sodium hydroxide in Milli-Q® water for a final volume of 50 mL (solution ANH4). A second solution was prepared to contain 5 g of sodium salicylate (0.62 mol L−1), 20 mg of sodium nitroprusside (1.34 mol L−1), 0.25 g of sodium hydroxide (0.125 mol L−1) and Milli-Q® water to make up the volume of a 50 mL flask (solution BNH4). A 20 mmol L−1 ammonium chloride stock solution was prepared in Milli-Q® water. The working concentration interval ranged from 0.1 to 2 mmol L−1, and 4 μL of solution ANH4 and 4 μL of solution BNH4 were added on paper. Then, 1 μL of the sample was added while starting the video recording after 20 min (Fig. 1B).
2.4.4 Nitrate. The Griess method36 detected nitrate in the samples. For that, two solutions were prepared: sulfanilamide 100 mmol L−1 in a 1
:
10 mixture of 37% hydrochloric acid and Milli-Q® water (solution ANO3), and N-(1-naphthyl) ethylenediamine hydrochloride (NED) 20 mmol L−1 and citric acid 660 mmol L−1 in Milli-Q® water (solution BNO3). A zinc suspension was prepared by dissolving 50 mg of powdered metallic zinc in 1 mL of water.In the preparation of the device (Fig. 2), 8 μL of the zinc suspension was added to the reduction channel in the bottom layer of the device. After drying completely, the channel was covered with adhesive tape to ensure zinc fixation. Then, 0.5 μL of solution ANO3 and 0.5 μL of solution BNO3 were added in separate fluidic layers and allowed to dry completely. Finally, to assemble the 3D device, the layers were folded and fixed with a paper binder.
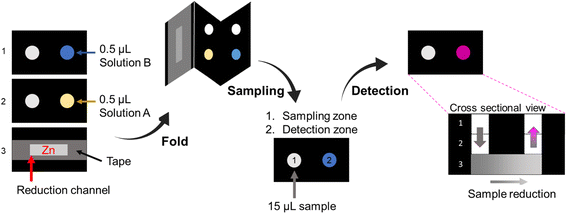 |
| Fig. 2 Representation of the device assembly for the nitrate test. The sulfanilamide and NED solutions were deposited in distinct fluidic layers. Zinc was added to the reduction channel and immobilized with adhesive tape. After the reagents were dried, the device was folded. The sample was added to the sampling zone and allowed to react for 15 min for color development. The cross-sectional view shows the sample path (indicated by the gray-to-pink arrows): sample was added onto fluidic layer 1 on the left side via sampling zone 1 and percolated through layer 2, arriving at the reduction channel on layer 3 and then returned to layer 1 on the right, emerging on the detection zone 2. The black region represents the hydrophobic wax barrier, and the white part represents the hydrophilic paper forming the path through which the sample percolates. The dotted black line only indicates the different layers. Dimensions: 5 mm diameter circles and reduction channel 15 mm length × 4 mm width. | |
The sodium nitrate stock solution at 10 mmol L−1 concentration was prepared in Milli-Q® water. The dilutions were prepared from the nitrate stock solution with concentrations ranging from 1.0 to 5.0 mmol L−1. For the detection test, 15 μL of the sample was applied in the sampling zone, and for the blank, 15 μL of Milli-Q® water was added. Video of the detection zone was acquired after 15 min. All experiments were performed in triplicate.
2.4.5 Interference study. The concentrations for the studied analyte and the interferents were fixed based on the average concentration of nutrients found in the soil solution (nitrate: 3.0 mmol L−1; ammonium: 1.0 mmol L−1; magnesium: 3.0 mmol L−1; calcium: 3.0 mmol L−1).37,38 The solutions containing the studied macronutrient and one or two interferents were prepared.
2.4.6 Analytical parameters. To obtain the LOD for each method, five measurements of the blank were made – that means the detection zone containing the reagents and the added sample was only purified water. Images were captured and treated as described in session 2.3. Then, the LOD was calculated using a 3 × standard deviation of the blank/slope of the calibration curve. The same procedure yielded the LOQ, and the calculation is given by the 10 × standard deviation of the blank/slope of the calibration curve. In turn, intermediate precision was assumed to be the standard deviation divided by the average value of the five blank measurements for each colorimetric method. Ten measures of the same concentration in the middle of the analytical range were made to calculate repeatability. Since we recorded the detection zone as videos, a way to obtain ten measurements is to evaluate different video frames. Finally, the standard deviation of these ten measurements was divided by the average value to obtain repeatability.
3 Results and discussion
3.1 Image acquisition
Images captured by smartphones are recorded as a two-dimensional array – in matrix format – where each element of this array is a pixel. For colored images, each pixel carries three intensity values, represented by the intensities of the components of the color spaces – proposals for the specific organization of colors in the space.39 A minimum of three parameters must describe a color space since human vision is based on trichromatic perception. Some of the most well-known color spaces are RGB (red, green, and blue), CIELab (L: lightness, a: green–red axis, b: blue–yellow axis), and HSV (hue, saturation, and value).40–42 Thus, the combination of the components of the three-dimensional models describes a color in space.39,43 Colored images can be converted to grayscale to decrease the space needed for storage, taking up a third of the space initially required. Furthermore, as the grayscale image is more straightforward than a color image, developing an algorithm for processing this image may be more objective.44
The video mode was chosen to ensure continuous lighting in the detection zone, avoiding variations due to flash firing in a photo capture.45 In addition to eliminating external equipment, one of the advantages of working with the smartphone's built-in flash as the dominant light source is canceling variations in ambient light. As the device is intended for field application, the light conditions must remain constant, as variations in luminosity can generate poor reproducibility. If the outdoor light intensity is equal to or greater than the smartphone camera LED, the effect of ambient light becomes essential. For example, brightness can reach 30
000 lux in direct sunlight on a sunny day, while smartphones can compensate only for up to 1000 lux. An application that evaluates the brightness of the environment46 and provides color corrections or alerts the user if it is suitable for reading the tests could overcome this limitation.
Using the ColorScan software for image analysis becomes advantageous over ImageJ, as the software recognizes the limits of the hydrophobic barrier. In ImageJ, the analyst must select the region of interest and manually delimit the boundary between the test zone and its surroundings. In addition, this zone must also be centered to avoid non-uniform signal capture. This Python-based program automatically identifies and measures zones containing signals of any geometry or colors from paper-based devices, enabling automated analysis of colorimetric signals on μPADs.31
Overall, we did not observe the coffee-ring effect during our measurements. We hypothesized that the quality of the paper – known for its uniform capillary action and minimal surface roughness –, the diameter of the of the spots, the small volume of reagents and sample, and the fast-drying time have corroborated with the even distribution of the components. This likely minimized the formation of concentrated rings of analytes along the edges of the dried spots. Additionally, to mitigate potential variability, we used the ColorScan software to precisely delimit the region of interest (ROI) for all measurements, ensuring uniform sizing and exclusion of border effects.
3.2 Colorimetric detection of analytes
3.2.1 Calcium. Among the reactions for identifying calcium are those with ammonium carbonate solution, sulfuric acid, ammonium oxalate, potassium hexacyanoferrate(II), or picrolonic acid. However, these reactions produce white products, making it difficult to quantify them on paper because they do not offer a contrast with the paper background.47 One of the methods for detecting calcium that produce bright colors is using the murexide indicator (Fig. 3B).48 In the presence of calcium, murexide changes its color from yellow to orange-red. This is because the Ca2+ ion forms a complex with the metallochromic indicator in a 1
:
1 ratio that absorbs light in a region of the electromagnetic spectrum (480 nm). At neutral pH, murexide is a monovalent anion, and its charge is distributed between the nitrogen atom and the four oxygen atoms that contribute to metal coordination.49,50
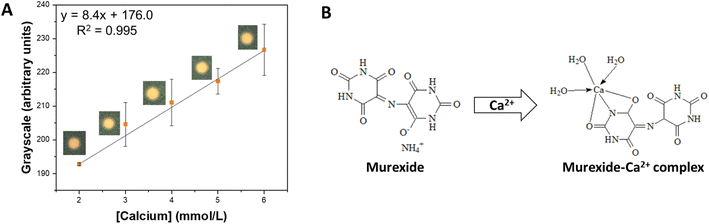 |
| Fig. 3 Detection of calcium in the proposed μPAD. (A) Calibration curve obtained for Ca2+ in concentrations ranging from 2.0 to 6.0 mmol L−1. (B) Structure of murexide and the murexide–Ca2+ complex. | |
The typical calcium concentration in soil solution can range from 1.0 to 5.0 mmol L−1. For the analytical curves, concentrations ranging from 0.1 to 6.0 mmol L−1 were tested, evaluating the response in the RGB, HSV, CIELab, and grayscale color spaces (Fig. S1†). The grayscale and ΔRGB displayed good sensitivity (7.74 and 9.74 a.u. L mol−1, respectively) and linearity (R2 = 0.993 and 0.973, respectively), being further investigated (Table S1†). Following, the limit of detection (LOD), the limit of quantification (LOQ), repeatability, and intermediate precision were compared for both color spaces (Table S2†). Although the grayscale displayed lower LOD (0.595 mmol L−1), LOQ (1.984 mmol L−1), and the best intermediate precision (3%), it stood out for its excellent reproducibility (4%), being chosen for subsequent experiments. The obtained values suggest that calcium can be detected in most of the typical range it is found in soil (from 1.0 to 5.0 mmol L−1), which was confirmed in the analytical curve (ranged from 2.0 to 6.0 mmol L−1) (Fig. 3A). Last, an interference test was performed with each reagent and with a mixture. The addition of the individual ions in concentrations typically found in soil did promote the underestimation of calcium concentrations (≤10%) (Table S3†). Still, the mixtures did not interfere significantly with the observed signal (≤1%) (Table S4†). It is also important to notice that although murexide can react with several other metals (e.g., cadmium, lead(II), copper), their typical concentrations in soil are much lower than calcium.51–53 Therefore, we do not expect significant interferences in actual soil samples.
3.2.2 Magnesium. The xylidyl blue dye is known to be sensitive and selective for magnesium, forming a red complex with the Mg2+ ion in an alkaline medium in a 1
:
1 ratio.54,55 EBT is a tridentate ligand that complexes with divalent metal ions in a 1
:
1 ratio. The indicator complexes with Mg2+ ions in an alkaline medium, changing colors from blue to violet.56 The use of two complexing agents for the detection of Mg2+ ions causes a mixture of colors that allows better identification of the compound (Fig. 4B).48
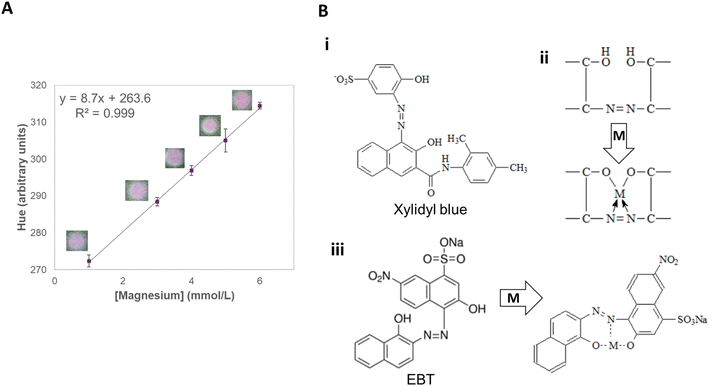 |
| Fig. 4 Detection of magnesium in the proposed μPAD. (A) Calibration curve obtained for Mg2+ in concentrations ranging from 1.0 to 6.0 mmol L−1. (B) Structure of (i) Xylidyl blue before the interaction with the analyte; (ii) the region of Xylidyl blue responsible for binding to Mg2+ before and after the interaction; and (iii) EBT before and after binding to Mg2+. | |
Both xylidyl blue and EBT are not specific for magnesium, reacting with other cations, especially Ca2+. EGTA, a chelating agent, is then used to mask the presence of Ca2+ ions, as this reagent has a greater affinity for Ca2+ ions than for Mg2+ ions.55,56 Furthermore, ethanolamine helps in the dissolution of metallic salts, such as xylidyl blue, which has low solubility in ethanol and is partially soluble in water, as the solvent is a bidentate ligand that has an amine group and a hydroxyl group available for coordination with metals.57
The parameter H (hue) of the HSV color space was the one that showed a linear trend (R2 = 0.999) with increasing magnesium concentrations from 1.0 to 6.0 mmol L−1, which comprises its typical concentrations in soil (from 1.0 to 5.0 mmol L−1)37 (Fig. 4A). The color of the paper changed gradually from blue to pink when magnesium was added to the sampling zone. Since hue indicates color gradient (ranging from 0 to 360), it was the best parameter to quantify the presence of this macronutrient (Fig. S2†). The method displayed good sensitivity (8.37 a.u. L mmol−1) and great intermediate precision (0.2%), and repeatability (1.0%), with all the evaluated analytical parameters being shown in Table S5.† Last, no significant signal change was observed both when studying the interference of other nutrients individually or in mixtures (≤1.6%), showing the great potential of applicability of the method to actual samples (Tables S6, and S7†). Fig. 4B shows the binding of Mg2+ to the applied reagents.56
3.2.3 Ammonium. The Berthelot reaction, or indophenol method, was first employed in 1859 and is one of the most widely used methods for detecting ammonium in seawater. However, this method generates volatile compounds hazardous to human health (ortho-chlorophenol).58,59 The salicylate method for ammonium detection is an alternative, and it was first introduced in soil science in 1983. The procedure uses sodium salicylate – to avoid working with phenolic compounds – and sodium nitroprusside as a catalyst.60 The salicylate method can detect low ammonium concentrations, which is another advantage, as ammonium concentrations in the soil solution are low (0.1–2.0 mmol L−1).35,61,62 For detection, the ammonium ion reacts with hypochlorite to form monochloramine, which then reacts with salicylate to form the 5-aminosalicylate compound. The 5-aminosalicylate is then oxidized in the presence of the nitroprusside catalyst forming the blue-colored indosalicylate compound (Fig. 5B).35,58,63
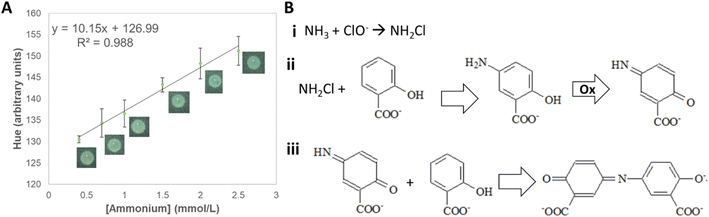 |
| Fig. 5 Detection of ammonium in the proposed μPAD. (A) Calibration curve obtained for ammonium in concentrations ranging from 0.5 to 2.5 mmol L−1. (B) Reaction between (i) ammonium and hypochlorite, forming monochloramine; (ii) monochloroamine and salicylate, forming 5-aminosalicylate and (iii) 5-aminosalicylate being oxidized in the presence of nitroprusside catalyst forming the blue colored indosalicylate compound. | |
Among the analyzed color spaces, the parameter that showed linearity (R2 = 0.988) with ammonium concentration (from 0.5 to 2.5 mmol L−1) was hue (Fig. 5A, and S3†). Although the final compound (indosalicylate) is blue, the resulting color is green due to the excess reagent, which is yellow. Thus, with the increasing ammonium concentration, the hue changed gradually from yellow to green. The method displayed good sensitivity (10.15 a.u. L mmol−1) and excellent repeatability (0.4%), and intermediate precision (0.8%). The calculated LOD is slightly above the lower concentrations typically found in soil (0.181 mmol L−1), suggesting that most samples can be analyzed using the device. The analytical parameters for the detection of ammonium are summarized in Table S8.† The influence of other nutrients on the quantification of ammonium was not significant both individually (≤3%) and in mixtures (≤5%) (Tables S9 and S10†), confirming its potential applicability to soil samples.
3.2.4 Nitrate. The Griess reagent is a classic method for the detection of nitrite. It is based on the reaction of nitrite with an aromatic primary amine, sulfanilamide, in an acidic medium, forming a diazo compound. This compound then reacts with NED, an aromatic compound containing an amino group, forming an azo dye that absorbs light in the region of 545 nm and, therefore, has a red-purple color (Fig. 6B).64 However, it is essential to notice that nitrate does not react with Griess reagent and needs to be reduced to nitrite to be detected. Reagents such as zinc, cadmium, hydrazine copper, cadmium with copper, and enzymes have been used to reduce nitrate to nitrite. Some of these reagents produce relatively large amounts of toxic or carcinogenic residues, making them unsuitable for use in-field. Zinc is a satisfactory alternative for the conversion of nitrate to nitrite. It eliminates the generation of toxic products associated with the use of cadmium – a metal commonly used in the determination of nitrate.65 The reagents sulfanilamide and NED compete for the reaction with nitrite. For this reason, each component of the Griess reagent was added in separate fluidic layers to ensure the analyte would react sequentially and to increase the stability and lifetime of the device.66,67 Device time life can be extended to 30 days if vacuum sealed and refrigerated in the freezer at −20 °C.68
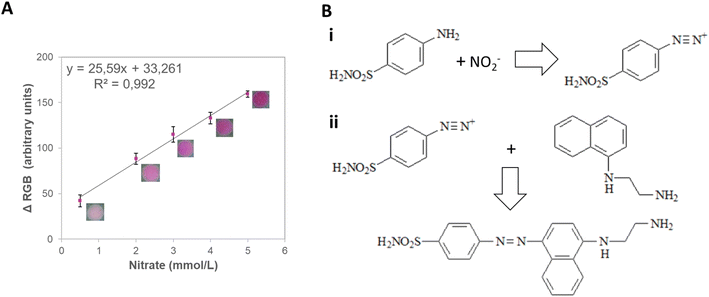 |
| Fig. 6 Detection of nitrate in the proposed μPAD. (A) Calibration curve obtained for ammonium in concentrations ranging from 0.5 to 10.0 mmol L−1. (B) Reaction between (i) nitrite and sulfanilamide, forming a diazo compound; (ii) diazo compound and NED, forming an azo dye with a red-purple color, allowing the quantification of nitrate. | |
In agricultural soils, the nitrate concentration can vary from 1 to 5 mmol L−1.38 Therefore, a concentration range from 0.5 to 10.0 mmol L−1 was tested and correlated with the signal intensity from different color spaces (RGB, CIELab, and HSV) (Fig. S4†). The ΔRGB and the greyscale presented good sensitivity (25.6 and 16.6 a.u. L mmol−1, respectively) and linearity (R2 = 0.992 and 0.997, respectively), being chosen for further investigation (Table S11†). Next, the LOD, LOQ, intermediate precision, and repeatability of the method were assessed (Table S12†). The ΔRGB method displayed lower LOD (0.229 mmol L−1) and LOQ (0.996 mmol L−1) while still maintaining good repeatability (4%) and being, therefore, chosen for the detection of this nutrient (Fig. 6A) due to its potential applicability to actual soil samples.
The analysis of individual interferents showed negligible signal variations for most nutrients (≤4%) except for calcium (7%) (Table S13†). It is essential to notice that the mixture of nutrients showed significant suppression of signal in the presence of calcium and magnesium (22%), showing the need for separation or the addition of masking agents – chelating agents such as EGDTA – to mitigate interference and to achieve reliable quantification (Table S14†).
Table 1 compares the detection range and LOD of developed devices to some of the most relevant devices found on the literature. Potentially portable sensors that could detect the analytes in aqueous solutions were preferably selected for assembling the table. It can be observed that most of the developed devices listed rely on electricity and external equipment to detect the desired analytes. Therefore, although these commonly present wider detection ranges and lower LODs than the devices developed by us, these are associated with higher costs and are more complicated to perform. The devices created here are, in general, easier to transport and use and present lower associated costs while still maintaining adequate analytical performance for soil analysis.
Table 1 Comparison of detection range and LOD between the developed devices and the literature
Macronutrient |
Sensor description |
Detection range |
LOD |
Ref. |
Calcium |
Potentiometry with ion-selective electrode based on diacrylated polyurethane |
5 × 10−3 to 80 mmol L−1 |
5 × 10−3 mmol L−1 |
69 |
Calcium |
Potentiometry with ion-selective electrode based on poly(vinyl chloride) (PVC) membranes with α-furildioxime(I) |
2.56 × 10−4 to 100 mmol L−1 |
1.25 × 10−4 mmol L−1 |
70 |
Calcium |
Electrochemical sensor based on flexible interdigitated electrodes composed of multi-walled carbon nanotubes and polydimethylsiloxane |
2.5 × 10−2 to 5.0 mmol L−1 |
2.5 × 10−2 mmol L−1 |
71 |
Calcium |
Potentiometric sensor based on PVC and {calcium bis[4-(1,1,3,3-tetramethylbutyl)phenylphosphate]} |
10−2 to 100 mmol L−1 |
10−2 mmol L−1 |
72 |
Calcium |
Paper-based colorimetric device based on murexide |
0.1 to 6.0 mmol L−1 |
0.595 mmol L−1 |
This work |
Magnesium |
Fluorimetric detection based on diaza-18-crown-6 8-hydroxy-quinoline and PVC |
4.6 × 10−4 to 22 mmol L−1 |
4.6 × 10−4 mmol L−1 |
73 |
Magnesium |
Electrochemical sensor based on flexible interdigitated electrodes composed of multi-walled carbon nanotubes and polydimethylsiloxane |
4.1 × 10−2 to 8.2 mmol L−1 |
4.1 × 10−2 mmol L−1 |
71 |
Magnesium |
Microfluidic paper-based analytical device based on cis-1,4-polyisoprene, EDTA eriochrome black T |
1.0 to 8.2 mmol L−1 |
1.0 mmol L−1 |
74 |
Magnesium |
Potentiometry with ion selective electrode based on PVC and methyl phenyl semicarbazone |
1 × 10−5 to 100 mmol L−1 |
1.7 × 10−6 mmol L−1 |
75 |
Magnesium |
Paper-based colorimetric device based on xylidyl blue and EBT |
1.0 to 6.0 mmol L−1 |
0.144 mmol L−1 |
This work |
Ammonium |
Laser-induced graphene electrodes for electrochemical ion-selective sensing of plant-available nitrogen |
0.01 to 100 mmol L−1 |
28.2 ± 25.0 × 10−3 mmol L−1 |
76 |
Ammonium |
Ion selective electrodes with phosphonium-based ionic liquid and poly(methyl methacrylate)/poly(decyl methacrylate) (MMA–DMA) |
5.0 × 10−3 to 1.0 mmol L−1 |
1.2 × 10−3 mmol L−1 |
77 |
Ammonium |
Colorimetric detection with o-phthalaldehyde/K2SO3 based sensor |
18 × 10−6 mmol L−1 |
0–250 × 10−3 mmol L−1 |
78 |
Ammonium |
Near-infrared transmission spectroscopy and partial least squares regression (PLSR) |
n/a |
0.0776 to 0.145 mmol L−1 |
79 |
Ammonium |
Paper-based colorimetric device based on the salicylate method |
0.5 to 2.5 mmol L−1 |
0.181 mmol L−1 |
This work |
Nitrate |
Ion selective electrodes with phosphonium-based ionic liquid and poly(methyl methacrylate)/poly(decyl methacrylate) (MMA–DMA) |
5.0 × 10−5 to 5.0 × 10−2 mol L−1 |
11.3 × 10−3 mmol L−1 |
77 |
Nitrate |
Laser-induced graphene electrodes for electrochemical ion-selective sensing of plant-available nitrogen |
0.01 to 100 mmol L−1 |
20.6 ± 14.8 × 10−3 mmol L−1 |
76 |
Nitrate |
Graphene oxide aerogel based solid-contact ion-selective electrodes |
10−5 to 100 mmol L−1 |
0.76 mmol L−1 |
80 |
Nitrate |
Ion sensitive field effect transistor |
2.3 × 10−5 to 6 × 10−2 mol L−1 |
1 × 10−2 mmol L−1 |
81 |
Nitrate |
Digital microfluidics combined with spectrometer and a 3D-printed microfluidic chip |
1.5 × 10−3 to 6.5 × 10−2 mmol L−1 |
5.1 × 10−3 mmol L−1 |
82 |
Nitrate |
Paper-based colorimetric device based on the Griess method |
0.5 to 10.0 mmol L−1 |
0.229 mmol L−1 |
This work |
4 Conclusions
Using a single device, the proposed colorimetric paper-based platform showed promising results for quantifying four different macronutrients in agricultural soils (nitrate, calcium, magnesium, and ammonium). The μPAD fabrication is simple, fast, and favors scaling, besides being portable, enabling field analysis. The range of concentrations in which each macronutrient was quantified is mostly within its typical range in soil. All macronutrients displayed a linear correlation with a colorimetric parameter, allowing its precise quantification in an automated setup. Furthermore, in most cases, no significant interference was observed in the presence of other macronutrients. The exception is the nitrate quantification, which showed a variation of −22% in the measured concentration when calcium and magnesium ions were present in the solution, indicating the need for masking agents to minimize signal suppression.
The challenge remains to find a protocol that can be applied to any soil type. Soils around the world can assume ultra-acidic pH (<3.5) or strongly alkaline (>9.0). In this work, we focused on neutral soils as most crops in Brazil are cultivated in soils with a pH ranging from 6–7. In truth, most plants are cultured in pH around 5.5–7.5; however, some grow outside this range.83,84 Thus, new extraction methods should be explored considering the variability of soil pH to convey the absolute bioavailability.
For future research, we aim to gather the tests in a single microfluidic device containing only one sample spot. The challenge in this is to control the flow inside a three-dimensional device. Also, each macronutrient was quantified by different color spaces, and that should be considered when developing a smartphone app for real-time analysis. It would also be interesting to include detecting other macronutrients such as potassium, phosphorus, sulfur, or micronutrients. However, an important point to consider is developing quick colorimetric tests that match the acquisition time for the other nutrients. Besides, finding colorimetric tests with low detection limits can be challenging, given the low concentration of nutrients in most soils.
Data availability
Data for this article, including the videos used in the analysis, are available at Mendeley Data at https://doi.org/10.17632/hxjcwstkgd.1.
Conflicts of interest
The authors have no competing interests to declare that are relevant to the content of this article.
Acknowledgements
The research leading to these results received funding from São Paulo Research Foundation (FAPESP) under Grant Agreement No. 2018/19750-3 and National Council for Scientific and Technological Development (CNPq) (Grant Agreement 130518/2016-6, 134389/2019-0 and 308835/2019-0). This study was also founded by Research Partnership for Technological Innovation - PITE (https://bv.fapesp.br/en/4/university-industry-cooperative-research-pite/) IBM-FAPESP, FAPESP (Grant number 17/19413-4), and INCTBio (grants FAPESP #2014/40867-3 and CNPq #465389/2014-7). The São Paulo Research Foundation supported this work - FAPESP (Grant number 2018/19750-3 and research grant Research Partnership for Technological Innovation - PITE IBM-FAPESP, Grant number 17/19413-4), INCTBio (grants FAPESP #2014/40867-3 and CNPq #465389/2014-7), and The National Council for Scientific and Technological Development (CNPq) (fellowships 130518/2016-6, 134389/2019-0 and 308835/2019-0).
References
- United Nations, Department of Economic and Social Affaris, The World Population Prospects 2019, 2019, https://reliefweb.int/sites/reliefweb.int/files/resources/WPP2019_Highlights.pdf Search PubMed
. - Food and Agriculture Organization, Global Agriculture towards 2050, 2009 Search PubMed
. - B. Sultan, Global warming threatens agricultural productivity in Africa and South Asia, Environ. Res. Lett., 2012, 7, 041001 CrossRef
. - S. Brodt, J. Six, G. Feenstra, C. Ingels and D. Campbell, Sustainable Agriculture, Nature Education Knowledge, 2011, vol. 3, p. 1 Search PubMed
. - S. Umesha, H. M. G. Manukumar, and B. Chandrasekhar, Chapter 3 - Sustainable Agriculture and Food Security, in Biotechnology for Sustainable Agriculture, ed. R. L. Singh and S. Mondal, Woodhead Publishing, 2018, pp. 67–92, DOI:10.1016/B978-0-12-812160-3.00003-9
. - H. Benyezza, M. Bouhedda, R. Kara and S. Rebouh, Smart platform based on IoT and WSN for monitoring and control of a greenhouse in the context of precision agriculture, Internet of Things, 2023, 23, 100830 CrossRef
. - S. T. Far and K. Rezaei-Moghaddam, Impacts of the precision agricultural technologies in Iran: An analysis experts' perception & their determinants, Inf. Process. Agric., 2018, 5, 173–184 Search PubMed
. - Food and Agriculture Organization of the United States, Food and Agriculture Organization of the United States, 2022, https://www.fao.org/home/en/ Search PubMed
. - D. J. Mulla, in Spatial Variability in Precision Agriculture BT - Encyclopedia of GIS, ed. S. Shekhar, H. Xiong and X. Zhou, Springer International Publishing, Cham, 2015, pp. 1–8, DOI:10.1007/978-3-319-23519-6_1652-1
. - H. R. El-Ramady, et al., in Soil Quality and Plant Nutrition BT - Sustainable Agriculture Reviews 14: Agroecology and Global Change, ed. H. Ozier-Lafontaine and M. Lesueur-Jannoyer, Springer International Publishing, Cham, 2014, pp. 345–447, DOI:10.1007/978-3-319-06016-3_11
. - B. A. Needelman, What Are Soils?, Nature Education Knowledge, 2013, vol. 4, p. 2 Search PubMed
. - Solo fértil SP Comercial Agrícola, Nutrição Foliar. solofertil.Com, 2019 Search PubMed.
- F. J. Maathuis, Physiological functions of mineral macronutrients, Curr. Opin. Plant Biol., 2009, 12, 250–258 CrossRef CAS PubMed
. - H.-J. Kim, K. A. Sudduth and J. W. Hummel, Soil macronutrient sensing for precision agriculture, J. Environ. Monit., 2009, 11, 1810–1824 RSC
. - Instituto agronômico, Instituto agronômico, http://www.iac.sp.gov.br/.
- V. I. Adamchuk, J. W. Hummel, M. T. Morgan and S. K. Upadhyaya, On-the-go soil sensors for precision agriculture, Comput. Electron. Agric., 2004, 44, 71–91 CrossRef
. - L. Burton, K. Jayachandran and S. Bhansali, Review—The ‘Real-Time’ Revolution for In situ Soil Nutrient Sensing, J. Electrochem. Soc., 2020, 167, 037569 CrossRef CAS
. - Imacimus, Portable Nutrients Analyzer, https://www.imacimus.com/, 2024 Search PubMed.
- Renke, Soil Analyzer, https://www.renkeer.com/product/portable-soil-analyzer/, 2024 Search PubMed.
- T. Ozer, C. McMahon and C. S. Henry, Advances in Paper-Based Analytical Devices, Annu. Rev. Anal. Chem., 2020, 13, 85–109 CrossRef PubMed
. - M. I. G. S. Almeida, B. M. Jayawardane, S. D. Kolev and I. D. McKelvie, Developments of microfluidic paper-based analytical devices (μPADs) for water analysis: A review, Talanta, 2018, 177, 176–190 CrossRef CAS PubMed
. - A. Gilchrist and J. Nobbs, Colorimetry, Theory, in Encyclopedia of Spectroscopy and Spectrometry, ed. J. C. Lindon, G. E. Tranter, and D. Koppenaal, Academic Press, Oxford, 3rd edn, 2017, pp. 328–333, DOI:10.1016/B978-0-12-803224-4.00124-2
. - Y. Fan, J. Li, Y. Guo, L. Xie and G. Zhang, Digital image colorimetry on smartphone for chemical analysis: A review, Measurement, 2021, 171, 108829 CrossRef
. - G. M. Fernandes, et al., Novel approaches for colorimetric measurements in analytical chemistry – A review, Anal. Chim. Acta, 2020, 1135, 187–203 CrossRef CAS PubMed
. - H. A. Silva-Neto, L. R. Sousa and W. K. T. Coltro, Chapter 4 – Colorimetric Paper-Based Analytical Devices, in Paper-based Analytical Devices for Chemical Analysis and Diagnostics, ed. W. R. de Araujo and T. R. L. C. Paixão, Elsevier, 2022, pp. 59–79, DOI:10.1016/B978-0-12-820534-1.00009-8
. - Y. Yang, et al., Paper-Based Microfluidic Devices: Emerging Themes and Applications, Anal. Chem., 2017, 89, 71–91 CrossRef CAS PubMed
. - L.-M. Fu and Y.-N. Wang, Detection methods and applications of microfluidic paper-based analytical devices, TrAC, Trends Anal. Chem., 2018, 107, 196–211 CrossRef CAS
. - H. J. Chun, Y. M. Park, Y. D. Han, Y. H. Jang and H. C. Yoon, Paper-based glucose biosensing system utilizing a smartphone as a signal reader, BioChip J., 2014, 8, 218–226 CrossRef CAS
. - C. Carrell, et al., Beyond the lateral flow assay: A review of paper-based microfluidics, Microelectron. Eng., 2019, 206, 45–54 CrossRef CAS
. - M. Dhanaraju, P. Chenniappan, K. Ramalingam, S. Pazhanivelan and R. Kaliaperumal, Smart Farming: Internet of Things (IoT)-Based Sustainable Agriculture, Agriculture, 2022, 12, 1745 CrossRef
. - R. W. Parker, D. J. Wilson and C. R. Mace, Open software platform for automated analysis of paper-based microfluidic devices, Sci. Rep., 2020, 10, 11284 CrossRef CAS PubMed
. - T. Kong, et al., Accessory-free quantitative smartphone imaging of colorimetric paper-based assays, Lab Chip, 2019, 19, 1991–1999 RSC
. - D. Xu, X. Huang, J. Guo and X. Ma, Automatic smartphone-based microfluidic biosensor system at the point of care, Biosens. Bioelectron., 2018, 110, 78–88 CrossRef CAS PubMed
. - GENPLANT - Grupo de Estudos em Nutrição de Plantas da UNESP, Nutrição de plantas, http://www.nutricaodeplantas.agr.br/site/equipe.php, 2022 Search PubMed.
- J. J. Giner-Sanz, G. M. Leverick, V. Pérez-Herranz and S.-H. Y, Salicylate Method for Ammonia Quantification in Nitrogen Electroreduction Experiments: The Correction of Iron III Interference, J. Electrochem. Soc., 2020, 167, 134519 CrossRef CAS
. - P. Griess, “Bemerkungen zu der Abhandlung der HH. Weselsky und Benedikt „Ueber einige Azoverbindungen”, Ber. Dtsch. Chem. Ges., 1879, 12, 426–428 CrossRef
. - S. A. Barber, Soil Nutrient Bioavailability: A Mechanistic Approach, John Wiley, New York, 1995 Search PubMed
. - P. J. White, Chapter 2 – Ion Uptake Mechanisms of Individual Cells and Roots: Short-Distance Transport, in Marschner's Mineral Nutrition of Higher Plants (Third Edition), ed. P. B. T.-M. Marschner, Academic Press, San Diego, 3rd edn, 2012, pp. 7–47, DOI:10.1016/B978-0-12-384905-2.00002-9
. - A. Bovik Introduction to digital image processing. in The Essential Guide to Image Processing 1–21, Academic Press, Austin, 2009 Search PubMed
. - Z. A. C. Shogah, D. S. Bolshakov and V. G. Amelin, Using Smartphones in Chemical Analysis, J. Anal. Chem., 2023, 78, 426–449 CrossRef CAS
. - S. Soares, G. M. Fernandes and F. R. P. Rocha, Smartphone-based digital images in analytical chemistry: Why, when, and how to use, TrAC, Trends Anal. Chem., 2023, 168, 117284 CrossRef CAS
. - S. Balasubramanian, et al., Digital colorimetric analysis for estimation of iron in water with smartphone-assisted microfluidic paper-based analytical devices, Int. J. Environ. Anal. Chem., 2023, 103, 2480–2497 CrossRef CAS
. - R. Lind Open source software for image processing and analysis: picture this with imageJ. in Open Source Software in Life Science Research, ed. L. Harland and M. Forster, Woodhead publishing, 2012, pp. 131–149 Search PubMed
. - L. Tan & J. Jiang Image processing basics. in Digital Signal Processing: Fundamentals and Application, ed. L. Tan and J. Jiang, Academic press, New York, 2019, pp. 679–725 Search PubMed
. - G. M. S. Ross, et al., Best practices and current implementation of emerging smartphone-based (bio)sensors – Part 1: Data handling and ethics, TrAC, Trends Anal. Chem., 2023, 158, 116863 CrossRef CAS
. - Google Play, Lux Meter (Light Meter), 2022 Search PubMed.
- A. I. Vogel and G. Svehla, Vogel's Qualitative Inorganic Analysis, Prentice Hall, Hoboken, 1996 Search PubMed
. - M. A. Ostad, A. Hajinia and T. Heidari, A novel direct and cost effective method for fabricating paper-based microfluidic device by commercial eye pencil and its application for determining simultaneous calcium and magnesium, Microchem. J., 2017, 133, 545–550 CrossRef CAS
. - A. Scarpa, Spectrophotometric measurement of calcium by murexide, in Photosynthesis and Nitrogen Fixation Part B, Academic Press, 1972, vol. 24, pp. 343–351 Search PubMed
. - S. Kashanian, M. B. Gholivand, S. Madaeni, A. Nikrahi and M. Shamsipur, Spectrophotometric study of the complexation reactions between alkaline earth cations and murexide in some non-aqueous solutions, Polyhedron, 1988, 7, 1227–1230 CrossRef CAS
. - UMass Extension, Soil Lead: Testing, Interpretation and Recommendations, https://ag.umass.edu/soil-plant-nutrient-testing-laboratory/fact-sheets/soil-lead-fact-sheet#:%7E:text=Lead-is-naturally-presentin,levels-to-several-thousand-ppm, 2020 Search PubMed.
- A. Kubier, R. T. Wilkin and T. Pichler, Cadmium in soils and groundwater: A review, Appl. Geochem., 2019, 108, 104388 CrossRef CAS PubMed
. - Cropnuts, Interpreting Your Soil Test Results, https://cropnuts.helpscoutdocs.com/article/829-interpreting-your-soil-test-results#:%7E:text=Calcium-(Ca)-2C-is-an,levels-of-430-2D540-20ppm, 2020 Search PubMed.
- E. E. Ludwig and C. R. Johnson, Spectrophotometric Determination of Magnesium by Titan Yellow, Ind. Eng. Chem., Anal. Ed., 1942, 14, 895–897 CrossRef CAS
. - M. F. Ryan and H. Barbour, Magnesium Measurement in Routine Clinical Practice, Ann. Clin. Biochem., 1998, 35, 449–459 CrossRef CAS PubMed
. - A. J. dos Santos, M. D. de Lima, D. R. da Silva, S. Garcia-Segura and C. A. Martínez-Huitle, Influence of the water hardness on the performance of electro-Fenton approach: Decolorization and mineralization of Eriochrome Black T, Electrochim. Acta, 2016, 208, 156–163 CrossRef
. - Z. Chen, et al., Ethanolamine-assisted synthesis of size-controlled indium tin oxide nanoinks for low temperature solution deposited transparent conductive films, J. Mater. Chem. C, 2015, 3, 11464–11470 RSC
. - Y. Zhu, et al., Development of analytical methods for ammonium determination in seawater over the last two decades, TrAC, Trends Anal. Chem., 2019, 119, 115627 CrossRef CAS
. - Y. B. Cho, S. H. Jeong, H. Chun and Y. S. Kim, Selective colorimetric detection of dissolved ammonia in water via modified Berthelot's reaction on porous paper, Sens. Actuators, B, 2018, 256, 167–175 CrossRef CAS
. - A. J. Kempers and A. Zweers, Ammonium determination in soil extracts by the salicylate method, Commun. Soil Sci. Plant Anal., 1986, 17, 715–723 CrossRef CAS
. - P. Marschner and Z. Rengel, Chapter 12 – Nutrient Availability in Soils, in Marschner's Mineral Nutrition of Higher Plants (Third Edition), ed. P. Marschner, Academic Press, San Diego, 3rd edn, 2012, pp. 315–330, DOI:10.1016/B978-0-12-384905-2.00012-1
. - M. A. Grusak, M. R. Broadley and P. J. White, Plant Macro- and Micronutrient Minerals, eLS, pp. 1–6, 2016, DOI:10.1002/9780470015902.a0001306.pub2
. - H. Hwang, et al., Lab-on-a-Disc for Simultaneous Determination of Nutrients in Water, Anal. Chem., 2013, 85, 2954–2960 CrossRef CAS PubMed
. - D. Giustarini, R. Rossi, A. Milzani and I. B. T.-M. Dalle-Donne, in E. Nitrite and Nitrate Measurement by Griess Reagent in Human Plasma: Evaluation of Interferences and Standardization. In Nitric Oxide, Part F, Academic Press, 2008, vol. 440, pp. 361–380 Search PubMed
. - N. O. Oladosu, et al., Online zinc reduction-sequential injection analysis for the determination of nitrogen species in extracts of riverine sediment, J. Anal. Sci. Technol., 2017, 8, 5 CrossRef
. - Z.-H. Wang and S.-X. Li, Chapter Three – Nitrate N Loss by Leaching and Surface Runoff in Agricultural Land: A Global Issue (A Review), in Advances in Agronomy, ed. D. L. Sparks, Academic Press, 2019, vol. 156, pp. 159–217 Search PubMed
. - G. A. Ermakova, S. Y. Skachilova, V. G. Voronin and Z. I. Shramova, Factors influencing the quality of sulfanilamide preparations (Review), Chem. Pharm. J., 1983, 17, 963–972 CAS
. - B. M. Jayawardane, S. Wei, I. D. McKelvie and S. D. Kolev, Microfluidic Paper-Based Analytical Device for the Determination of Nitrite and Nitrate, Anal. Chem., 2014, 86, 7274–7279 CrossRef CAS PubMed
. - J. Artigas, A. Beltran, C. Jiménez, J. Bartrolí and J. Alonso, Development of a photopolymerisable membrane for calcium ion sensors, Anal. Chim. Acta, 2001, 426, 3–10 CrossRef CAS
. - A. K. Singh and S. Mehtab, Calcium(II)-selective potentiometric sensor based on α-furildioxime as neutral carrier, Sens. Actuators, B, 2007, 123, 429–436 CrossRef CAS
. - F. Akhter, A. Nag, M. E. E. Alahi, H. Liu and S. C. Mukhopadhyay, Electrochemical detection of calcium and magnesium in water bodies, Sens. Actuators, A, 2020, 305, 111949 CrossRef CAS
. - S. G. Lemos, A. R. A. Nogueira, A. Torre-Neto, A. Parra and J. Alonso, Soil Calcium and pH Monitoring Sensor System, J. Agric. Food Chem., 2007, 55, 4658–4663 CrossRef CAS PubMed
. - L. Lvova, et al., Systematic approach in Mg2+ ions analysis with a combination of tailored fluorophore design, Anal. Chim. Acta, 2017, 988, 96–103 CrossRef CAS PubMed
. - P. Jarujamrus, et al., Screen-printed microfluidic paper-based analytical device (μPAD) as a barcode sensor for magnesium detection using rubber latex waste as a novel hydrophobic reagent, Anal. Chim. Acta, 2019, 1082, 66–77 CrossRef CAS
. - S. Chandra, K. Sharma and A. Kumar, Mg(II) Selective PVC Membrane Electrode Based on Methyl Phenyl Semicarbazone as an Ionophore, J. Chem., 2013, 1–7 Search PubMed
. - N. T. Garland, et al., Flexible Laser-Induced Graphene for Nitrogen Sensing in Soil, ACS Appl. Mater. Interfaces, 2018, 10, 39124–39133 CrossRef CAS PubMed
. - J. Choosang, et al., Simultaneous Detection of Ammonium and Nitrate in Environmental Samples Using on Ion-Selective Electrode and Comparison with Portable Colorimetric Assays, Sensors, 2018, 18, 3555 CrossRef PubMed
. - J. Cao, et al., Portable smartphone platform utilizing dual-sensing signals for visual determination of wide concentration ammonium in real samples, Chem. Eng. J., 2023, 456, 141085 CrossRef CAS
. - R. Yupiter, S. Arnon, E. Yeshno, I. Visoly-Fisher and O. Dahan, Real-time detection of ammonium in soil pore water, npj Clean Water, 2023, 6, 25 CrossRef CAS
. - M.-Y. Kim, et al., Highly stable potentiometric sensor with reduced graphene oxide aerogel as a solid contact for detection of nitrate and calcium ions, J. Electroanal. Chem., 2021, 897, 115553 CrossRef CAS
. - J. Artigas, et al., Application of ion sensitive field effect transistor based sensors to soil analysis, Comput. Electron. Agric., 2001, 31, 281–293 CrossRef
. - Y. Hong, et al., Multi-Sample Detection of Soil Nitrate Nitrogen Using a Digital Microfluidic Platform, Agriculture, 2023, 13, 2226 CrossRef CAS
. - International Plant Nutrition Institute, Manual Internacional de Fertilidade Do Solo, Associação Basileira para Pesquisa da potassa e do fosfato, Piracicaba, 1998 Search PubMed
. - S. O. Oshunsanya, Relevance of soil pH to agriculture, in Soil pH for Nutrient Availability and Crop Performance, ed. S. Oshunsanya, Intechopen, 2018 Search PubMed
.
|
This journal is © The Royal Society of Chemistry 2024 |
Click here to see how this site uses Cookies. View our privacy policy here.