Why genetic modification of lignin leads to low-recalcitrance biomass†
Received
30th October 2014
, Accepted 31st October 2014
First published on 11th November 2014
Abstract
Genetic modification of plants via down-regulation of cinnamyl alcohol dehydrogenase leads to incorporation of aldehyde groups in the lignin polymer. The resulting lignocellulosic biomass has increased bioethanol yield. However, a molecular-scale explanation of this finding is currently lacking. Here, we perform molecular dynamics simulation of the copolymer with hemicellulose of wild type and the genetically modified lignin, in aqueous solution. We find that the non-covalent association with hemicellulose of lignin containing aldehyde groups is reduced compared to the wild-type. This phase separation may increase the cell wall porosity in the mutant plants, thus explaining their easier deconstruction to biofuels. The thermodynamic origin of the reduced lignin-hemicellulose association is found to be a more favorable self-interaction energy and less favorable interaction with hemicellulose for the mutant lignin. Furthermore, reduced hydration water density fluctuations are found for the mutant lignin, implying a more hydrophobic lignin surface. The results provide a detailed description of how aldehyde incorporation makes lignin more hydrophobic and reduces its association with hemicellulose, thus suggesting that increased lignin hydrophobicity may be an optimal characteristic required for improved biofuel production.
Introduction
Plant biomass is composed of cellulose fibers embedded in a matrix of lignin and hemicellulose polymers.1 Lignins are hydrophobic heteropolymers, which can be branched, that provide plants with mechanical rigidity and help regulate water conduction.2–5 They are composed of three monomers or monolignols: syringyl (S), guaiacyl (G), and p-hydroxyphenyl (H), radically coupled to form a lignin polymer chain.5 The polymerization of lignin is random, resulting in differences between lignins among taxa and species as well as cell types in the same plant.5
Lignin protects plants against enzymatic degradation by forming a covalently bonded network with hemicellulose that blocks enzymatic access to cellulose.2,5,6 A biological route of cellulosic biofuel production involves enzymatic break down of cellulose into fermentable sugars.7,8 Thermochemical pretreatment of biomass increases the effectiveness of subsequent cellulose hydrolysis by increasing access of cellulolytic enzymes to cellulose. This is achieved by either partial removal of lignin and hemicellulose or by phase separation of those two polymers.7,9 However, currently, pretreatment is energy intensive, time consuming, and costly. Therefore, improving the pretreatment process is an essential step in making plant biomass a more economically viable biofuel source.
Recent advances in genetic engineering have shown that precursors along the biosynthetic pathway of monolignols can be incorporated into lignin, sometimes without detrimental effects on plant growth.2,5,10,11 For example, down-regulation of cinnamyl alcohol dehydrogenase (CAD), which catalyzes the final step in biosynthesis of monolignols, leads to incorporation of cinnamic aldehydes into the lignin polymer.4,5,12–15 Hydroxycinnamyl and cinnamic aldehydes differ from wild type monolignols by the presence of aldehyde groups on the allyl chain, which is thus more hydrophobic than with the equivalent wild type hydroxyl groups.
Reduction in CAD activity leads to improved lignocellulosic biofuel feedstocks due to reduced lignin content and/or modified lignin structure.16 For example, mutants with reduced CAD activity are more susceptible to enzymatic hydrolysis (saccharification) than wild-type plants in Brachypodium16 and switchgrass.17 Another study showed down-regulation of CAD to improve ethanol yield in maize.18 However, a molecular-level understanding of how aldehyde precursor inclusion may lead to less recalcitrant biomass is lacking.
Here, we conduct atomistic molecular dynamics (MD) simulations to characterize how aldehyde precursor inclusion in lignin affects its association with hemicellulose. We find that the increase in hydrophobicity of lignin from incorporation of the aldehyde groups reduces its association with hemicellulose, with more favorable intra-lignin interactions and less favorable interactions with hemicellulose. This work illustrates how MD simulations can be a useful tool for predicting the effects of changes in plant genotype to cell wall phenotype in efforts to enhance the production of biofuels or other bioproducts.
Methods
Lignin and hemicellulose models
A previously-built crosslinked lignin–hemicellulose copolymer model,9 constructed based on experimental data of the composition of Aspen lignin and hemicellulose, was modified for use in this study. A structural model of a covalently linked lignin–hemicellulose copolymer was generated by using available experimental information on the average chemical composition of Aspen lignin and hemicellulose.19 Aspen lignin is composed primarily of guaiacyl (G) and syringyl (S) monomeric units, in the ratio S/G ∼ 1.86, connected by various linkages. Here, a linear lignin polymer was constructed that was composed of 11 G and 20 S units connected via 22 β-O-4 (β-aryl ether), four 4-O-5 (bithenyl ether), two β–β (pinoresinol) and two β-1 linkages, similar to the experimentally determined average inter-linkage composition of Aspen.19 Hemicelluloses are branched polymers composed of sugar residues. Here, the hemicellulose polymer consisted of a (1 → 4) linked backbone consisting of 28 β-xylose and four β-mannose monomers. Four 4-O-methyl-glucuronic acid (4-O-MeGlcA) side-chain monomers were bonded to the xylose backbone via a (1 → 2) link. The lignin and hemicellulose polymers were connected end-to-end via an ether bond between the γ carbon of the lignin and the O1 oxygen of the hemicellulose, resembling a ferulate lignin-hemicellulose crosslink. The lignin-hemicellulose polymer was then hydrated in a cubic box and four Na+ ions added to neutralize the system (4-O-MeGlcA, with pKa value of 3, was deprotonated). The system is shown in Fig. 1.
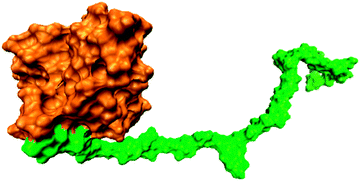 |
| Fig. 1 Snapshot of the simulated lignin (orange)–hemicellulose (green) copolymer. | |
Here, hydrophobicity is defined by the magnitude and distribution of the partial charges of functional groups on monolignols.. The partial charge on the oxygen atom of the hydroxyl OH group (−0.66) is more negative than that of the methoxy MeO (−0.28) and aldehyde groups (−0.40) respectively. The variance of the distribution of the atomistic partial charges of three functional groups (which contain four, three, and two atoms, respectively), Cq is 0.02, 0.13 and 0.22 for the methoxy, aldehyde and hydroxyl groups, respectively. Cq provides a quantitative measure of the distribution of the magnitude of partial charges across atoms of an overall neutral functional group. A large Cq indicates a high degree of polarization and stronger electrostatic dipole interactions.
Three models of lignin are considered here, constructed by altering partial charges of specific functional groups on S and G (Fig. 2). The following modifications were made on these models.
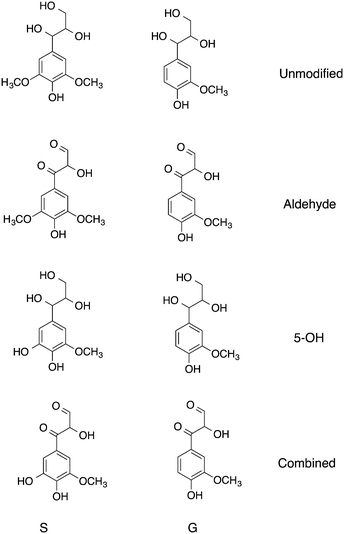 |
| Fig. 2 Monomers of the lignin polymers simulated here, S are syringyl-like and G guaiacyl-like. The full simulated system is shown in Fig. 1. | |
5-OH model.
A more hydrophilic 5-OH group replaces the 5-MeO group at position 5 present on wild-type S lignin. The oxygen atom of the 5-OH group has its partial charge decreased from −0.28 to −0.54, which is the charge of a phenolic oxygen in the CHARMM force field.20,21 Accordingly, the carbon atom at position 4 on the phenyl ring has its partial charge increased by 0.07 to 0.11. This modification makes the phenol ring of S lignin less hydrophobic than in wild type plants, and reflects 5-hydroxy-guaiacyl lignin found in mutant plants.22,23
Aldehyde model.
OH groups on the α, β, and γ carbon atoms of the allyl of both S and G lignin have their partial charges modified in order to model the presence of aldehyde functional groups. The partial charges of the oxygen atom of the allyl are increased from −0.66 for a hydroxyl oxygen to −0.40 for an aldehyde oxygen in the CHARMM General force field.24 The carbon and hydrogen partial charges are also taken from the aldehyde groups in CHARMM General force field.24 This modification makes the allyl chain of lignin more hydrophobic than in wild type plants and reflects coniferaldehyde and sinapaldehyde lignin found in mutant plants.12,13 A PDB file of the model is provided in the ESI.†
Combined model.
For the final model, the two modifications discussed above were combined to form a lignin with a 5-OH substitution and aldehyde groups on the allyl chain that reflects, 5-hydroxyconiferaldehyde, a lignin precursor that could be incorporated into lignin.2
For all models, β-O-4 (β-aryl ether) and β–β (pinoresinol) linkages were modified in order to preserve overall charge neutrality. Comparison is made to the unmodified. Wild-type model of ref. 9.
Molecular dynamics simulation
MD simulations were performed using the NAMD 2.9 software25 by employing the CHARMM carbohydrate26,27 and lignin21 force fields and the TIP3P water model.28 The Particle Mesh Ewald method29,30 was used with a grid spacing of 1 Å and a force-switching function to smoothly transition Leonard Jones forces to zero over the range of 9–10 Å. Multiple time steps of 2 fs were used for bonded and short-range non-bond forces, and 6 fs for long-range electrostatic forces. The neighbor list was updated every 10 steps with a pair-list distance of 11 Å. Constant temperature was maintained by using the Langevin dynamics algorithm with a damping coefficient of 5 ps−1. The pressure was maintained at 1 atm using the Nose–Hoover Langevin piston algorithm31,32 that employed a piston oscillation period of 200 fs and a piston damping decay time of 100 fs.
All models were solvated in a water box of size (126 × 126 × 125) Å. The solvated systems were then ionized in order to maintain the system charge neutral. The 5-OH, aldehyde, and combined lignin models were simulated for 388 ns, 340 ns, and 361 ns respectively at temperature T = 298 K. All calculations were performed on the Titan Cray XK7 supercomputer at the Oak Ridge Leadership Computing Facility.
Analysis of all MD trajectories was performed with the VMD software33 by using local scripts. An atomic contact was defined as two atoms separated by less than 3 Å. An atom is defined as hydrophobic when having a partial charge |q| < 0.3 and hydrophilic otherwise. In comparison, the partial charges of apolar moieties in molecular mechanics force fields of proteins have |q| < 0.4.34 A sphere radius of 1.4 Å was employed in the computation of the solvent-accessible surface area (SASA). The surface water density, ρ is given by:
|  | (1) |
where
N is the number of water molecules at a distance less than 4.5 Å from the lignin,
S is the solvent accessible surface area of lignin, and
d is the thickness of the hydration shell, which is taken as 4.5 Å.
35 The surface energy density
γ is given by:
|  | (2) |
where
E is the interaction energy between lignin and water. Lastly, the number of hydrogen bonds was calculated over the last 10 ns of simulation time of the aldehyde and combined models at intervals of 1 ps. Here, the cutoff distance between donor and acceptor is 3.5 Å and the angle formed by donor, hydrogen, and acceptor must be less than 30°. This geometric definition has been shown to roughly reproduce experimental water hydrogen bond activation energies
36 and to match experimentally determined water hydrogen bond geometries.
37
Results
Structure and contacts
The radius of gyration (Rg) of each model is shown as a function of simulation time in Fig. 3. The aldehyde model initially expands slightly from its initial structure, then relaxes into a more compact state after about 60 ns (Fig. 3, blue) and on average is the most compact of the models and has the smallest fluctuations of Rg (Table 2). The 5-OH model displays considerably larger variations in Rg than the others and elongates near the end of the simulation to a greater extent (Fig. 3, red). Since the shape of the 5-OH model did not converge in simulation, it is not discussed further here. The combined model shows a slightly greater Rg than the aldehyde model, and has a more extended and variable structure (Fig. 3, purple). Comparison with the wild-type (unmodified) model (Table 1) indicates that inclusion of aldehyde groups reduces the size of the lignin.
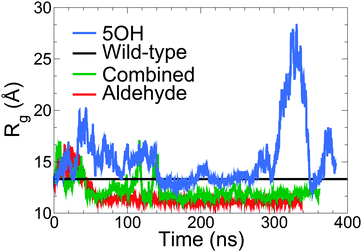 |
| Fig. 3 Radius of gyration of the lignin polymers (Rg) as a function of time for each model of lignin. The black line represents the average Rg of the wild-type (unmodified) model of lignin from ref. 9. | |
Table 1 Average radius of gyration and percentage of lignin atoms in contact with hemicellulose for each lignin model averaged over the last 150 ns of each trajectory
Model |
R
g (Å) |
Contacts (%) |
Wild-type |
13.3 ± 0.2 |
10.1 ± 1.3 |
Aldehyde |
11.1 ± 0.1 |
2.4 ± 0.7 |
Combined |
11.8 ± 0.3 |
2.8 ± 1.4 |
Table 2 Normalized variance of water density fluctuations of each lignin model. Values are an average of five 10 ns segments calculated over the last 50 ns of the simulation
Model |
Var(ρ) (×10−4) |
Wild-type |
0.04 ± 0.02 |
Aldehyde |
0.13 ± 0.05 |
Combined |
0.13 ± 0.06 |
Fig. 4 quantifies the proportion of lignin contacts with hemicellulose, defined here as the percentage of lignin atoms at a distance less than 3 Å from the hemicellulose. Compared to the wild-type model, in the aldehyde-containing models contacts with hemicellulose are reduced over the course of the simulation.
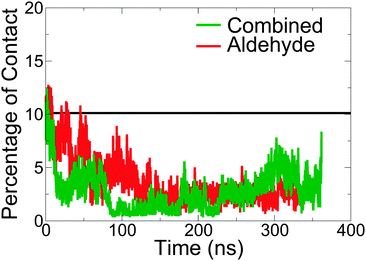 |
| Fig. 4 Percentage of lignin atoms forming contacts with hemicellulose, defined here as the number of atoms of lignin within 3 Å of hemicellulose divided by the total number of lignin atoms. The black line is for the wild-type (unmodified) model from ref. 9. | |
The ratio of lignin-hemicellulose hydrogen bonds of the combined model to the aldehyde model over the last 10 ns of simulation time is 2.4 ± 1.3. In comparison, the ratio of the total number of possible hydrogen-bond acceptors/donors on a monolignol monomer between the aldehyde and combined models is 1
:
1.25. Therefore, there is a significantly greater amount of hydrogen-bond saturation in the combined than in the aldehyde model.
Surface polarity
Ratios of solvent accessible hydrophilic to hydrophobic surface areas given by r, |  | (3) |
are shown in Fig. 5. The ratios are given for the lignin polymer (LI), an isolated syringyl monomer (SYR), and the hemicellulose polymer (HE). The ratio r is higher for LI than for SYR for all models, reflecting the preferential exposure of hydrophilic groups to the solvent in the lignin polymer.35 For SYR, the wild-type model has a higher r than the aldehyde model (Fig. 5, black and blue) because of the larger number of hydrophobic moieties introduced in the latter. However, the ratio for LI is the same for the wild-type and aldehyde polymers, indicating that the addition of aldehyde groups does not change the overall polarity of the lignin surface. The values of r for the combined model of both SYR and LI are larger than the other models, reflecting the addition of hydrophilic moieties, and indicates an increase in the overall polarity of the lignin surface (Fig. 5, purple). The r for hemicellulose is the same for all models.
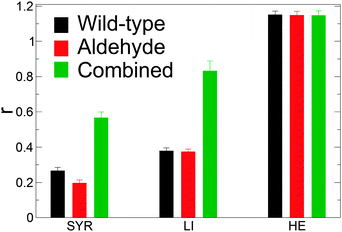 |
| Fig. 5 Ratio of average solvent-accessible hydrophilic surface area to hydrophobic surface area, r, for each model of lignin (LI), hemicellulose (HE), and syringyl (SYR) monomer. Data averaged over the last 150 ns. | |
Surface hydrophobicity
The normalized variance of the water density, Var(ρ), was used to quantitatively compare the hydrophobicity of the surface of each lignin model (Table 2). Here, |  | (4) |
where σρ is the standard deviation and 〈ρ〉 is the mean of the water density over a period of 10 ns. The higher Var(ρ), i.e. the higher the density fluctuations in the hydration shell, the more hydrophobic is the solute surface.38,39 Var(ρ) for the aldehyde and combined models are statistically similar (Table 2). However, the wild-type model has a lower value for Var(ρ), which indicates that the hydrophobicity of the lignin surface is increased by the presence of the aldehyde groups.
Interaction energies
Non-bonded interaction energies are listed in Table 3. Both the combined and the aldehyde models have more favorable lignin-to-lignin (LI–LI) interactions and less favorable lignin-to-hemicellulose (LI–HE) interactions compared to the wild-type model (Table 3). The aldehyde model has the energetically least favorable interaction with hemicellulose, which reflects the smaller LI–HE contact reported in Fig. 5.
Table 3 Average interaction energies of each lignin model with lignin (LI–LI) and hemicellulose (LI–HE). Data averaged over the last 10 ns of the simulation
Model |
LI–LI (kcal mol−1) |
LI–HE (kcal mol−1) |
Wild-type |
1083 ± 22 |
−136 ± 16 |
Aldehyde |
687 ± 21 |
−27 ± 7 |
Combined |
685 ± 21 |
−48 ± 13 |
Both the aldehyde and the combined models interact less favorably with water (Table 4). Values for the lignin–water surface energy density γ, given by eqn (2), are shown in Table 4. The highest values for surface energy density are for the aldehyde and combined models.
Table 4 Average surface energy density of each lignin model during the last 10 ns of simulation time. Obtained as the lignin–water interaction energy divided by the lignin SASA
Model |
γ (kcal mol−1 Å−2) |
Wild-type |
−0.27 ± 0.08 |
Aldehyde |
−0.05 ± 0.06 |
Combined |
−0.06 ± 0.07 |
Discussion
The recalcitrance of lignocellulose biomass to hydrolysis into fermentable sugars is the main barrier to economically viable second-generation biofuel production. It has been shown that plants with reduced activity of the CAD enzyme, which catalyzes the last step of monolignol biosynthesis, integrally incorporate aldehydes into lignin12 and also show improved yields of ethanol from biomass compared to wild-type.17,18 However, a molecular-scale explanation of this improvement in biomass conversion to ethanol has not been provided until now. Decreasing the non-covalent association between lignin and hemicellulose is a potential explanation for the increased cellulose hydrolysis, by increasing the accessibility to cellulose of cellulolytic enzymes.9
Inclusion of the hydrophobic aldehyde groups in lignin reduces lignin-hemicellulose contact (Table 1). Generalizing this finding, we suggest that any genetic modification that renders lignin more hydrophobic may impact favorably biomass conversion to biofuel. A thermodynamic explanation of this finding is obtained by considering changes in lignin interaction with itself and with hemicellulose. The average interaction energies (Table 3) imply that the presence of aldehyde moieties leads to a variant lignin that interacts more favorably with itself than does wild-type lignin. Additionally, the lignin-hemicellulose interaction energy per contact is more favorable for wild-type lignin (−13.5 kcal mol−1) than for the aldehyde model (−11.3 kcal mol−1). Finally, lignin:water interaction energy densities (Table 4) show the wild-type model to interact more favorably with water, which is chemically more similar to the hydrophilic hemicellulose than the hydrophobic lignin. Therefore, compared to wild-type lignin, inclusion of aldehydes in the variant lignin thermodynamically promotes self-association over non-covalent contact with hemicellulose.
A quantitative measure of the hydrophobicity of a surface is the magnitude of the density fluctuations of its hydration water, Var(ρ): the greater the fluctuations, the more hydrophobic the surface.38 Var(ρ) at the surface of the wild-type model is lower than for the aldehyde and combined models, (Table 2) thus suggesting that the genetic modification introduced by downregulation of CAD increases the hydrophobicity of the lignin surface. This is consistent with the differences in the water-lignin surface energy density. Although the surface energy density differs between the wild-type and aldehyde models, their respective SASA ratios are similar; this may be due to sharp cut-off used here to differentiate between a hydrophilic and hydrophobic atom. The ratio of the hydrophobic to hydrophilic SASA of the lignin polymer (LI) is higher than that of an isolated syringyl monomer (SYR). This implies that lignin adopts conformations that maximize exposure of its hydrophilic components.
Previous computational studies have focused on wild type lignin.3 Quantum chemical studies have examined bond dissociation enthalpies relevant to lignin deconstruction,40–44 radical coupling reactions in lignin biosynthesis45–47 and dilignol interaction energies with ionic liquid ions.48 MD simulations of wild-type lignin have probed its shape and configurations35,49,50 as well as its interaction with cellulose51–53 and hemicellulose.9 The present study shows that mutating lignin in silico can yield macromolecular properties that are consistent with the phenotype of mutant plants. Therefore, the use of MD simulation as a predictive tool for the effect of genetic modifications on lignin structure could be further pursued in future work.
Conclusions
Effects of down regulation of the CAD enzyme in plants were modelled by introducing aldehyde functional groups in a lignin:hemicellulose copolymer. MD simulations of the model show the modified lignin to be more hydrophobic and to associate less with hemicellulose than does wild-type lignin. The looser coupling of lignin and hemicellulose could lead to increased accessibility of cellulose in plant biomass. Therefore, the results of this study explain why plants with reduced CAD activity are more easily deconstructed for biofuel production. This work suggests the potential for using MD simulation as a tool to connect genotype to phenotype in genetic modifications to plants.
Acknowledgements
We thank Drs Brian H. Davison and Amandeep Sangha for useful discussions. This research is funded by the BioEnergy Science Center, a U.S. Department of Energy (DOE) Bioenergy Research Center supported by the Office of Biological and Environmental Research in the DOE Office of Science. This research used resources of the Oak Ridge Leadership Computing Facility at the Oak Ridge National Laboratory, which is supported by the Office of Science of the U.S. Department of Energy under Contract No. DE-AC05-00OR22725.
Notes and references
- D. J. Cosgrove and M. C. Jarvis, Front. Plant Sci., 2012, 3, 204 CrossRef PubMed.
- A. J. Ragauskas, G. T. Beckham, M. J. Biddy, R. Chandra, F. Chen, M. F. Davis, B. H. Davison, R. A. Dixon, P. Gilna, M. Keller, P. Langan, A. K. Naskar, J. N. Saddler, T. J. Tschaplinski, G. A. Tuskan and C. E. Wyman, Science, 2014, 344, 1246843 CrossRef PubMed.
- A. K. Sangha, L. Petridis, J. C. Smith, A. Ziebell and J. M. Parks, Environ. Prog. Sustainable Energy, 2012, 31, 47–54 CrossRef CAS.
- W. Boerjan, J. Ralph and M. Baucher, Annu. Rev. Plant Biol., 2003, 54, 519–546 CrossRef CAS PubMed.
- R. Vanholme, B. Demedts, K. Morreel, J. Ralph and W. Boerjan, Plant Physiol., 2010, 153, 895–905 CrossRef CAS PubMed.
- P. Langan, B. R. Evans, M. Foston, W. T. Heller, H. O'Neill, L. Petridis, S. V. Pingali, A. J. Ragauskas, J. C. Smith and V. S. Urban, Ind. Biotechnol., 2012, 8, 209–216 CrossRef CAS.
- H. Jorgensen, J. B. Kristensen and C. Felby, Biofuels, Bioprod. Biorefin., 2007, 1, 119–134 CrossRef.
- M. E. Himmel, S. Y. Ding, D. K. Johnson, W. S. Adney, M. R. Nimlos, J. W. Brady and T. D. Foust, Science, 2007, 315, 804–807 CrossRef CAS PubMed.
- P. Langan, L. Petridis, H. M. O'Neill, S. V. Pingali, M. Foston, Y. Nishiyama, R. Schulz, B. Lindner, B. L. Hanson, S. Harton, W. T. Heller, V. Urban, B. R. Evans, S. Gnanakaran, A. J. Ragauskas, J. C. Smith and B. H. Davison, Green Chem., 2014, 16, 63–68 RSC.
- N. D. Bonawitz, J. I. Kim, Y. Tobimatsu, P. N. Ciesielski, N. A. Anderson, E. Ximenes, J. Maeda, J. Ralph, B. S. Donohoe, M. Ladisch and C. Chapple, Nature, 2014, 509, 376–380 CrossRef CAS PubMed.
- P. N. Ciesielski, M. G. Resch, B. Hewetson, J. P. Killgore, A. Curtin, N. Anderson, A. N. Chiaramonti, D. C. Hurley, A. Sanders, M. E. Himmel, C. Chapple, N. Mosier and B. S. Donohoe, Green Chem., 2014, 16, 2627–2635 RSC.
- Q. Zhao, Y. Tobimatsu, R. Zhou, S. Pattathil, L. Gallego-Giraldo, C. Fu, L. A. Jackson, M. G. Hahn, H. Kim, F. Chen, J. Ralph and R. A. Dixon, Proc. Natl. Acad. Sci. U. S. A., 2013, 110, 13660–13665 CrossRef CAS PubMed.
- X. Li, J. K. Weng and C. Chapple, Plant J., 2008, 54, 569–581 CrossRef CAS PubMed.
- H. Kim, J. Ralph, F. C. Lu, S. A. Ralph, A. M. Boudet, J. J. MacKay, R. R. Sederoff, T. Ito, S. Kawai, H. Ohashi and T. Higuchi, Org. Biomol. Chem., 2003, 1, 268–281 CAS.
- H. Kim, J. Ralph, N. Yahiaoui, M. Pean and A. M. Boudet, Org. Lett., 2000, 2, 2197–2200 CrossRef CAS PubMed.
- C. R. Poovaiah, M. Nageswara-Rao, J. R. Soneji, H. L. Baxter and C. N. Stewart, Plant Biotechnol. J., 2014 DOI:10.1111/pbi.12225.
- C. X. Fu, X. R. Xiao, Y. J. Xi, Y. X. Ge, F. Chen, J. Bouton, R. A. Dixon and Z. Y. Wang, BioEnergy Res., 2011, 4, 153–164 CrossRef.
- S. Fornale, M. Capellades, A. Encina, K. Wang, S. Irar, C. Lapierre, K. Ruel, J. P. Joseleau, J. Berenguer, P. Puigdomenech, J. Rigau and D. Caparros-Ruiz, Mol. Plant, 2012, 5, 817–830 CrossRef CAS PubMed.
- P. Sannigrahi, A. J. Ragauskas and G. A. Tuskan, Biofuels, Bioprod. Biorefin., 2010, 4, 209–226 CrossRef CAS.
- A. D. MacKerell, D. Bashford, M. Bellott, R. L. Dunbrack, J. D. Evanseck, M. J. Field, S. Fischer, J. Gao, H. Guo, S. Ha, D. Joseph-McCarthy, L. Kuchnir, K. Kuczera, F. T. K. Lau, C. Mattos, S. Michnick, T. Ngo, D. T. Nguyen, B. Prodhom, W. E. Reiher, B. Roux, M. Schlenkrich, J. C. Smith, R. Stote, J. Straub, M. Watanabe, J. Wiorkiewicz-Kuczera, D. Yin and M. Karplus, J. Phys. Chem. B, 1998, 102, 3586–3616 CrossRef CAS PubMed.
- L. Petridis and J. C. Smith, J. Comput. Chem., 2009, 30, 457–467 CrossRef CAS PubMed.
- J.-K. Weng, H. Mo and C. Chapple, Plant J., 2010, 64, 898–911 CrossRef CAS PubMed.
- R. Vanholme, J. Ralph, T. Akiyama, F. Lu, J. R. Pazo, H. Kim, J. H. Christensen, B. Van Reusel, V. Storme, R. De Rycke, A. Rohde, K. Morreel and W. Boerjan, Plant J., 2010, 64, 885–897 CrossRef CAS PubMed.
- K. Vanommeslaeghe, E. Hatcher, C. Acharya, S. Kundu, S. Zhong, J. Shim, E. Darian, O. Guvench, P. Lopes, I. Vorobyov and A. D. MacKerell, J. Comput. Chem., 2010, 31, 671–690 CAS.
- J. C. Phillips, R. Braun, W. Wang, J. Gumbart, E. Tajkhorshid, E. Villa, C. Chipot, R. D. Skeel, L. Kale and K. Schulten, J. Comput. Chem., 2005, 26, 1781–1802 CrossRef CAS PubMed.
- O. Guvench, S. N. Greene, G. Kamath, J. W. Brady, R. M. Venable, R. W. Pastor and A. D. Mackerell, J. Comput. Chem., 2008, 29, 2543–2564 CrossRef CAS PubMed.
- O. Guvench, E. Hatcher, R. M. Venable, R. W. Pastor and A. D. MacKerell, J. Chem. Theory Comput., 2009, 5, 2353–2370 CrossRef CAS PubMed.
- W. L. Jorgensen, J. Chandrasekhar, J. D. Madura, R. W. Impey and M. L. Klein, J. Chem. Phys., 1983, 79, 926–935 CrossRef CAS PubMed.
- T. Darden, D. York and L. Pedersen, J. Chem. Phys., 1993, 98, 10089–10092 CrossRef CAS PubMed.
- U. Essmann, L. Perera, M. L. Berkowitz, T. Darden, H. Lee and L. G. Pedersen, J. Chem. Phys., 1995, 103, 8577–8593 CrossRef CAS PubMed.
- G. J. Martyna, D. J. Tobias and M. L. Klein, J. Chem. Phys., 1994, 101, 4177–4189 CrossRef CAS PubMed.
- S. E. Feller, Y. H. Zhang, R. W. Pastor and B. R. Brooks, J. Chem. Phys., 1995, 103, 4613–4621 CrossRef CAS PubMed.
- W. Humphrey, A. Dalke and K. Schulten, J. Mol. Graphics, 1996, 14, 33–38 CrossRef CAS.
- D. S. Cerutti, J. E. Rice, W. C. Swope and D. A. Case, J. Phys. Chem. B, 2013, 117, 2328–2338 CrossRef CAS PubMed.
- L. Petridis, R. Schulz and J. C. Smith, J. Am. Chem. Soc., 2011, 133, 20277–20287 CrossRef CAS PubMed.
- F. W. Starr, F. Sciortino and H. E. Stanley, Phys. Rev. E: Stat. Phys., Plasmas, Fluids, Relat. Interdiscip. Top., 1999, 60, 6757–6768 CrossRef CAS.
- D. van der Spoel, P. J. van Maaren, P. Larsson and N. Timneanu, J. Phys. Chem. B, 2006, 110, 4393–4398 CrossRef CAS PubMed.
- S. N. Jamadagni, R. Godawat and S. Garde, Annu. Rev. Chem. Biomol. Eng., 2011, 2, 147–171 CrossRef CAS PubMed.
- S. Sarupria and S. Garde, Phys. Rev. Lett., 2009, 103, 037803 CrossRef.
- A. Beste, J. Phys. Chem. A, 2014, 118, 803–814 CrossRef CAS PubMed.
- A. Beste and A. C. Buchanan, J. Org. Chem., 2009, 74, 2837–2841 CrossRef CAS PubMed.
- A. Beste and A. C. Buchanan, J. Org. Chem., 2011, 76, 2195–2203 CrossRef CAS PubMed.
- S. Kim, S. C. Chmely, M. R. Nimlos, Y. J. Bomble, T. D. Foust, R. S. Paton and G. T. Beckham, J. Phys. Chem. Lett., 2011, 2, 2846–2852 CrossRef CAS.
- R. Parthasarathi, R. A. Romero, A. Redondo and S. Gnanakaran, J. Phys. Chem. Lett., 2011, 2, 2660–2666 CrossRef CAS.
- A. K. Sangha, B. H. Davison, R. F. Standaert, M. F. Davis, J. C. Smith and J. M. Parks, J. Phys. Chem. B, 2014, 118, 164–170 CrossRef CAS PubMed.
- A. K. Sangha, J. M. Parks, R. F. Standaert, A. Ziebell, M. Davis and J. C. Smith, J. Phys. Chem. B, 2012, 116, 4760–4768 CrossRef CAS PubMed.
- T. J. Tschaplinski, R. F. Standaert, N. L. Engle, M. Z. Martin, A. K. Sangha, J. M. Parks, J. C. Smith, R. Samuel, N. Jiang, Y. Q. Pu, A. J. Ragauskas, C. Y. Hamilton, C. X. Fu, Z. Y. Wang, B. H. Davison, R. A. Dixon and J. R. Mielenz, Biotechnol. Biofuels, 2012, 5, 71 CrossRef CAS PubMed.
- N. Sun, R. Parthasarathi, A. M. Socha, J. Shi, S. Zhang, V. Stavila, K. L. Sale, B. A. Simmons and S. Singh, Green Chem., 2014, 16, 2546–2557 RSC.
- L. Petridis, S. V. Pingali, V. Urban, W. T. Heller, H. M. O'Neil, M. Foston, A. Ragauskas and J. C. Smith, Phys. Rev. E: Stat., Nonlinear, Soft Matter Phys., 2011, 83, 061911 CrossRef.
- S. Besombes and K. Mazeau, Biopolymers, 2004, 73, 301–315 CrossRef CAS PubMed.
- S. Besombes and K. Mazeau, Plant Physiol. Biochem., 2005, 43, 299–308 CrossRef CAS PubMed.
- S. Besombes and K. Mazeau, Plant Physiol. Biochem., 2005, 43, 277–286 CrossRef CAS PubMed.
- B. Lindner, L. Petridis, R. Schulz and J. C. Smith, Biomacromolecules, 2013, 14, 3390–3398 CrossRef CAS PubMed.
Footnote |
† Electronic supplementary information (ESI) available. See DOI: 10.1039/c4cp05004e |
|
This journal is © the Owner Societies 2015 |
Click here to see how this site uses Cookies. View our privacy policy here.