DOI:
10.1039/C7RA12725A
(Paper)
RSC Adv., 2018,
8, 3760-3767
Identifying the molecular targets of Salvia miltiorrhiza (SM) in ox-LDL induced macrophage-derived foam cells based on the integration of metabolomics and network pharmacology
Received
23rd November 2017
, Accepted 23rd December 2017
First published on 22nd January 2018
Abstract
The identification of network targets is one of the core issues used to reveal the molecular mechanism of traditional Chinese medicine (TCM) and is also the grand challenge of modernization of TCM. In this study, a protein–protein interaction (PPI) network was constructed based on the integration of network pharmacology and metabolomics, which was used as an effective approach to elucidate the relationship between disease pathway proteins and the targets of active small-molecule compounds. The intermolecular transfer process of the drug effect of active compounds in Salvia miltiorrhiza (SM) was revealed and visualized using the PPI network. Our study indicates that PTGS2 was the most important disease protein regulated by the active compounds in SM. Furthermore, the drug targets that can be linked to PTGS2 were regarded as direct targets and the direct targets of the active compounds were identified, respectively. Western blot and co-immuno precipitation (Co-IP) were used to verify the results of the network analysis and reveal the intermolecular transfer process of the effect of Tan IIA. Biological validation revealed that Tan IIA-EDN1-PTGS2-anandamide was a major intervention way of Tan IIA on early atherosclerosis (AS). This work provides a new perspective for the discovery of drug targets and the specific approaches regulated by the active compounds in SM on disease pathway proteins, which is beneficial for understanding the mechanism of action of bioactive compounds and expanding their clinical applications.
Introduction
Atherosclerosis (AS) is one of the leading causes of disability and death worldwide. Recent studies have demonstrated that oxidized modification of low-density lipoprotein (LDL) is the link or key factor in triggering foamy macrophage formation, which is considered as an important initial event at the onset of AS.1,2 Salvia miltiorrhiza (SM) is a herb widely used in traditional Chinese medicine (TCM) for the treatment of cardiovascular disease.3,4 The aqueous extract, ethanol extract and the major water-soluble constituents, including danshensu, salvianolic acid A (Sal. A) and salvianolic acid B (Sal. B) have an inhibitory effect on LDL oxidation and demonstrate an obviously advantage in the prevention and cure of AS.5–7 However, the mechanisms of action have not been well studied, which has limited their clinical applications. At present, most pharmacology studies based on the holistic view of system theory has been limited to the obscure description of the overall outline of the biological system before and after drug intervention. Whereas, how the bioactive components affect the disease metabolic network still remains unclear. Therefore, perfecting the research strategy on the whole metabolic network is beneficial towards elucidating the scientific essence of TCM and identifying the network targets, which have vital practical significance for accelerating the internationalization of TCM.
Systems biology techniques, such as omics and bioinformatics have facilitated the analysis of complicated systems and relational networks. In view of the advantages of their techniques, metabolomics and network pharmacology have become important platforms for highlighting the pharmacological bioactivity and biochemical mechanism of TCM.8–12 Metabolomics is considered as the “biochemical phenotype” of the whole functional state of an organism, which can reflect the perturbations of endogenous metabolites instantly and sensitively. The main analytical methods used in metabolomics include NMR and UPLC, which have been widely applied towards metabolite identification in vitro or in vivo.13–15 The development of analytical techniques has broadened the application of metabolomics; a typical research area is identifying drug(s)-affected pathways.16,17 However, the proteins in the drug(s)-affected pathways obtained by metabolomics are mainly downstream disease-related proteins. Network pharmacology has been proven successful in target identification, however, it lacks the detailed representation of the regulation processes on specific metabolic pathways or molecular networks, resulting in the weak disease specificity of the target networks. Identifying the relevance between the disease pathway and drug target network followed by elucidating the transfer process of drug action from upstream drug targets to downstream disease proteins systematically is critical towards revealing the mechanism of drug action.
In our previous study, global metabolomics was applied to reveal the metabolic perturbation in ox-LDL induced foamy macrophages, which was then used to identify the potential biomarkers and disease pathways in early AS.18 Herein, a protein–protein interaction (PPI) network was built based on the integration of metabolomics and network pharmacology to explore the regulatory mechanism of SM on disease pathway proteins. This work provides valuable methodological guidance towards visualizing the interaction of drug targets and disease proteins, which is beneficial for understanding the complex mechanisms of multi-target therapy.
Data source and methods
Chemicals and reagents
High-glucose Dulbecco's modified eagle medium (DMEM) and fetal bovine serum (FBS) were purchased from HyClone for cell culture. Trypsin/EDTA solution and dimethylsulfoxide (DMSO) and phosphate buffered saline (PBS) were obtained from Solarbio (Beijing, China). Ox-LDL was obtained from Yiyuan Biotechnologies (Guangzhou, China). PTGS2 (Abcam, ab15191), EDN-1 (Abcam, ab2786) and β-actin (Santa, Sc-47778) were used in the Western blot and co-immuno precipitation (Co-IP) analyses. Tan IIA and Sal. A were purchased from Chengdu Herbpurify Co., Ltd (Chengdu, China).
Data sources
The disease pathway network of early AS was constructed using an ox-LDL induced foamy macrophages model based on untargeted metabolomics in our previous study.18 The proteins involved in the disease pathway network were collected as the disease pathway proteins for further study. Both the lipid-soluble and water-soluble components of SM showed significant activity on AS; the components with a high content and activity were absorbed as candidates in this article.19–21 As a result, tanshinone IIA (Tan IIA), cryptotanshinone, salvianolic acid A (Sal. A), salvianolic acid B (Sal. B), danshensu and protocatechualdehyde were selected as the major bioactive components for further target research. The target proteins of six active components in SM were collected via two data mining methods using the chemical composition database ChEMBL22 (https://www.ebi.ac.uk/chembl/#) and protein interaction database STITCH 3.1 (ref. 23) (http://stitch.embl.de/) in 2017. The names of each active component were entered into ChEMBL as the search keywords for data mining. The target information of six active components in SM was provided in the “compound target summary” of the database. In the STITCH database, the interaction score revealed the binding degree between the components and proteins. A higher score means the higher the reliability of the data. The active interaction sources included many items: text mining, experiments, databases, co-expression, neighborhood, gene fusion, co-occurrence and predictions. The target proteins with a score higher than 0.70 (high confidence) were included for the PPI network construction.
Pathway enrichment analysis
Protein enrichment analysis was carried out in PANTHER™ Version 12.0 (ref. 24) (http://www.pantherdb.org/) for the disease pathway proteins and drug target proteins, respectively. The results of the biological process, molecular function and pathway were obtained using enrichment analysis.
Network construction
Firstly, the target PPI network of each active component was built using Cytoscape 3.2.1 based on the STRING score values (higher than 0.70 with high confidence) and the target proteins without the combination of any other protein at high confidence were ignored in our further study. The disease PPI network was also constructed in a similar way. Then, the target PPI network and disease PPI network were merged together to identify the core targets, which can be connected to the disease pathway proteins discovered using metabolomics. The detailed regulatory process of the active components in SM were obtained and visualized based on the integrated PPI network.
Biological validation
Macrophages (Raw 264.7) were provided by the National infrastructure of cell line resource (Beijing, China). In this experiment, the cell samples were divided into four groups: the control group, model group, Tan IIA-treated group (50 μmol L−1) and Sal. A-treated group (80 μmol L−1). The cell culture was referred to the previous procedure.18 Cell samples of the different groups were harvested for western blotting analysis and Co-IP.
PTGS2 and EDN-1 were chosen to validate the expression patterns in the different groups. A standard western blotting procedure was performed as described previously.25 The blots were probed with PTGS2 (Abcam, ab15191; 1
:
1000 dilution) and EDN-1 (Abcam, ab2786; 1
:
1000 dilution) with β-actin (Santa, Sc-47778; 1
:
1000 dilution) used as an internal reference. The bands of the western blot were quantified by measuring the band intensity. The results were expressed as the fold change upon normalizing to the control group. Co-IP method can directly investigate the combination of disease pathway proteins and drug target proteins. In this work, PTGS2 was identified as the most important disease pathway protein. The PPI network result of Tan IIA and Sal. A showed the interaction of PTGS2 and EDN-1, which indicated PTGS2 can be regulated by Tan IIA and Sal. A through EDN-1. Therefore, PTGS2 and EDN-1 were selected for experimental validation using Co-IP. Standard Co-IP analysis was performed as described previously.26 The protein extracts were incubated with agarose-conjugated antibodies in lysis buffer at 4 °C for 3 h and precipitated via centrifugation. The precipitate was washed 4 times and then boiled for 5 min in 1× loading buffer. The supernatant was run on an SDS-PAGE gel after centrifugation, followed by western blotting.
Results and discussions
Analysis of the disease pathway proteins
In our previous study, five metabolites were identified to discriminate the foamy macrophages of the control group and six metabolic pathways related to early AS were also discovered based on untargeted metabolomics.18 The proteins involved in the disease pathway network were used in the enrichment analysis and drug target study. Detailed information on the disease pathway proteins is shown in Table 1 including the critical metabolites influenced by specific disease proteins. The metabolomics results indicate that the anandamide-related pathway plays a major role in foam cell formation and the metabolic disturbances of anandamide may be an important feature of foamy macrophages. There are three anandamide-related proteins, namely prostaglandin-endoperoxide synthase (PTGS2), arachidonate 12-lipoxygenase (A12LOX) and arachidonate 15-lipoxygenase (A15LOX), which should be meaningful for drug target discovery and mechanistic studies. Recently, chronic inflammation has been considered as an important pathologic event in early AS and arachidonic acid-derived lipid molecules have been reported to be related to inflammation.27,28 Notably, PTGS2 is a key catalytic enzyme in arachidonic acid metabolism and is reasonable as a potential target for the prevention and treatment of AS. Furthermore, gene ontology (GO) enrichment analysis was applied to clarify the biological processes, molecular functions and pathways. Functional classification of the disease proteins is shown in Fig. 1. Among the six pathways, inflammation mediated by the chemokine and cytokine signaling pathway (P00031) was generally recognized as the initial step in the pathogenesis of AS.29 Androgen/estrogen/progesterone biosynthesis (P02727) also has a direct impact on AS and progesterone or its analogue used in the hormone replacement therapy (HRT) may attenuate the cardio protective effects of estrogen.30 The downstream pathway proteins can regulate endogenous metabolites directly, which are more sensitive for drug target discovery and mechanistic studies.
Table 1 Information of disease pathway proteinsa
Protein name |
Gene symbol |
Metabolites |
The critical metabolites influenced by specific disease protein are identified in bold. |
3-β-Hydroxy-delta-(5)-steroid dehydrogenase |
HSD3B2 |
Pregnenolone; progesterone |
3-α-Hydroxysteroid dehydrogenase |
Akr1c9 |
5α-Androstane-3,17-dione; androsterone |
Arachidonate 12-lipoxygenase |
ALOX12 |
Anandamide; 12(S)-hydroxyarachidonylethanolamide |
Arachidonate 15-lipoxygenase |
ALOX15 |
Anandamide; hydroxy arachidonylethanolamide |
Cholesterol monooxygenase |
CYP11A1 |
Pregnenolone; cholesterol; 20α,22β-dihydroxycholesterol |
Prostaglandin-endoperoxide synthase |
PTGS2 |
Anandamide; prostaglandin E2 ethanolamide |
Steroid 21-monooxygenase |
CYP21A2 |
Pregnenolone; 21-hydroxypregneolone |
Steroid 17-α-monooxygenase |
CYP17A1 |
Pregnenolone; 7α-hydroxypregnenolone |
3-Oxo-5-α-steroid 4-dehydrogenase |
SRD5A2 |
5α-Androstane-3,17-dione; androst-4-ene-3,17-dione |
Estrone sulfotransferase |
CLF_112599 |
Pregnenolone; pregnenolone sulfate |
Steryl-sulfatase |
STS |
Pregnenolone; pregnenolone sulfate |
5-Oxoprolinase (ATP-hydrolyzing) |
OPLAH |
5-Oxoproline; L-glutamate |
Steroid b-isomerase |
ksi |
Pregnenolone; progesterone |
Bile acid-CoA: amino acid N-acyltransferase |
BAAT |
Glycocholate; choloyl-CoA; glycine |
γ-Glutamylcyclotransferase |
GGCT |
5-Oxoproline |
Soluble epoxide hydrolase |
Ephx2 |
5,6-DHET; 5,6-EET |
Microsomal epoxide hydrolase |
EPHX1 |
5,6-DHET; 5,6-EET |
Long-chain-fatty-acid-CoA ligase |
ACSL1 |
(9Z,12Z,15Z)-Octadecatrienoic acid; α-linolenoyl-CoA |
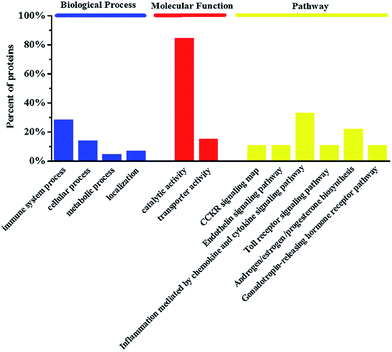 |
| Fig. 1 The enrichment analysis in biological processes, molecular functions and pathways of disease proteins involved in foamy macrophages. | |
Identification of targets of active components in SM
In this paper, Tan IIA, cryptotanshinone, Sal. A, Sal. B, danshensu and protocatechualdehyde are regarded as the active components of SM used for drug target identification. The target proteins of the six active components in SM were collected from the database and the detailed results shown in Table 2. The protein–protein interactions of the target proteins in each active component were analysed, respectively, removing the proteins without the interaction of others. To facilitate the biological function of the potential targets identified, GO enrichment analysis was performed and the results of the biological process displayed in Fig. 2A. Only the target of protocatechualdehyde met the requirements, thus the enrichment analysis result was ignored. The biological process analysis revealed that Tan IIA, Sal. A and Sal. B participated in several important metabolic processes in the organisms giving rise to a prospective therapeutic effect. Additionally, seven common biological processes were discovered in the three active components in SM, including the response to stimulus, developmental process, cellular process, metabolic process, biological regulation, cellular component organization or biogenesis and immune system process, which constitute the main biological processes of SM (Fig. 2B). In addition, ALOX12 as a specific protein exists in both disease pathway proteins and target proteins of Tan IIA (Fig. 3E). Lipoxygenases (LOXs) play a critical role in many diseases by participating in the metabolism of polyunsaturated fatty acids. ALOX12 is involved in many metabolic diseases, such as inflammatory responses, neurodegenerative disorders and cardiovascular disease.31,32 Recently, studies have indicated ALOX12 as an activity mediator of inflammation, expressed by the early events leading to AS.33 Searching for specific LOX inhibitors has been an avenue for targeted drug development. Thus, it is reasonable to believe that Tan IIA may perform therapeutic action via ALOX12 directly.
Table 2 Information on the target proteins of six active components in SM
Compound |
Gene symbol |
Source |
Pathway |
Cryptotanshinone |
STAT3 |
|
|
|
STRING |
Gonadotropin-releasing hormone receptor pathway; JAK/STAT signaling pathway; interleukin signaling pathway; angiogenesis; p53 pathway; inflammation mediated by chemokine and cytokine signaling pathway; CCKR signaling map; PDGF signaling pathway; EGF receptor signaling pathway; RAS Pathway |
|
POLB, RECQL, POLI, SMAD3 |
PTPN6, PTPN1, POLK, TDP1 |
CES2, FEN1, PTPN2, CES1 |
TERT, WRN, PTPN11 POLH |
ChEMBL |
Salvianolic acid A |
STAT3 |
EDN1 |
NR1I2 |
|
STRING |
ALP23B signaling pathway; JAK/STAT signaling pathway; angiogenesis; interleukin signaling pathway; interferon-gamma signaling pathway; inflammation mediated by chemokine and cytokine signaling pathway; EGF receptor signaling pathway; gonadotropin-releasing hormone receptor pathway; vitamin D metabolism and pathway; PDGF signaling pathway; MYO signaling pathway; RAS pathway; Notch signaling pathway; Cadherin signaling pathway; BMP/activin signaling pathway-drosophila; B-cell activation; activin beta signaling pathway; CCKR signaling map; p53 pathway; TGF-beta signaling pathway; FGF signaling pathway |
|
FEN1, POLI, PTPN2, POLH, KAT2A, GLP1R |
WRN, TERT, PTPN1, RECQL, THRB, PTPRF |
PTPN6, TDP1, PTPN11, CES1, VDR, ABCB1 |
POLB, CES2, POLK, SMAD3, NPSR1 |
ChEMBL |
Protocatechualdehyde |
GLS |
|
|
|
ChEMBL |
No |
Danshensu |
HMOX1 |
|
|
|
STRING |
Oxidative stress response; CCKR signaling map; FAS signaling pathway; apoptosis signaling pathway; Huntington disease; p53 pathway |
|
CASP3 |
BCL2 |
BAX |
|
PubChem bioassay |
Salvianolic acid B |
MAPK8 |
IL4 |
IL15 |
LCK |
STRING |
Apoptosis signaling pathway; angiogenesis; interferon-gamma signaling pathway; Alzheimer's disease-amyloid secretase pathway; integrin signalling pathway; EGF receptor signaling pathway; Parkinson's disease; gonadotropin-releasing hormone receptor pathway; PDGF signaling pathway; oxidative stress response; RAS pathway; Cadherin signaling pathway; B-cell activation; CCKR signaling map; Toll receptor signaling pathway; T-cell activation; TGF-beta signaling pathway; FGF signaling pathway; FAS signaling pathway |
|
STAT3, IL2 |
APP, SRC |
STAT1 |
STAT5B, |
ChEMBL |
Tanshinone IIA |
MMP2, ATF3 |
MMP9 |
CCND1 |
EDN1 |
STRING |
Wnt signaling pathway; P53 pathway feedback loops 1; apoptosis signaling pathway; Huntington disease's; p53 pathway feedback loops 2; p53 pathway by glucose deprivation; p53 pathway |
|
CES1, CES2, PTPN6, MGLL, POLH, SMAD3, TP53, MAPT |
MAPK1, TDP1, KAT2A, EHMT2, RECQL, GNAS, POLB, AKR1B1 |
FEN1, POLI, POLK, PGR, APAF1, VDR, NR3C1, AR |
TERT, HPGD, PLK1, PTPN11, AKR1B1, ALOX12, L3MBT, L1 |
ChEMBL |
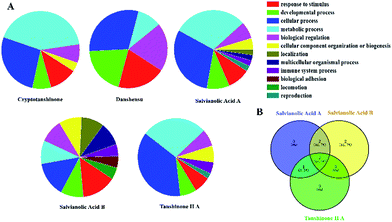 |
| Fig. 2 The enrichment analysis of active components in SM. (A) The results of biological processes of target proteins; (B) venn diagram. | |
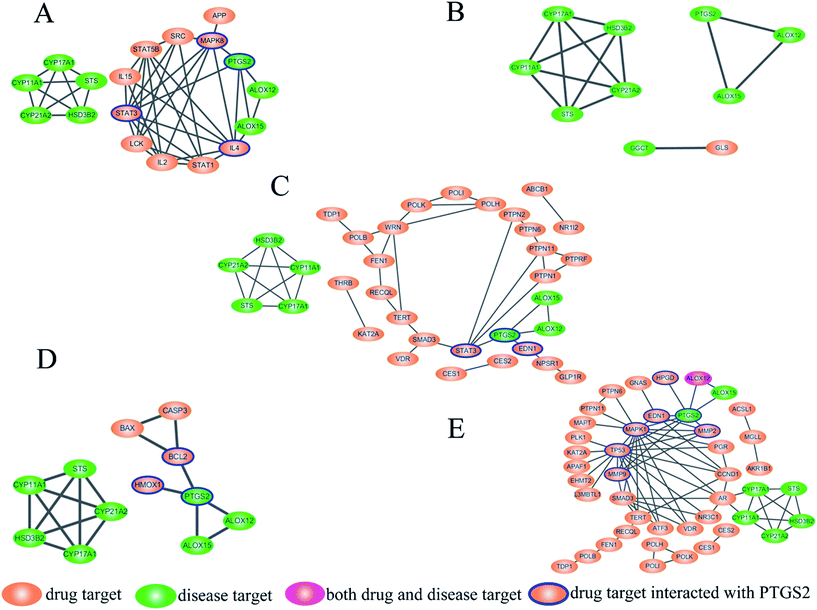 |
| Fig. 3 Disease pathway protein–target protein interaction (PPI) network of the active components in SM: (A) salvianolic acid B (Sal. B); (B) protocatechualdehyde; (C) salvianolic acid A (Sal. A); (D) danshensu; (E) tanshinone IIA (Tan IIA). Green ellipsoids represent the disease pathway proteins, while pink ellipsoids represent the target proteins of the active components in SM. ALOX12 has both the disease pathway protein and target protein of Tan IIA colored in red. | |
Interaction of disease pathway proteins and target proteins
The interaction of disease pathway proteins and target proteins were obtained by STRING and the PPI networks were merged in Cytoscape (Fig. 3A–E). In the PPI networks, the orange ellipsoids represent the disease pathway proteins and the green ellipsoids are the target proteins of the active components in SM. ALOX12 is both a disease pathway protein and target protein of Tan IIA and colored in pink. There were no interactions between the target proteins of cryptotanshinone with any disease pathway protein. The disease pathway network contains two parts, one comprised of PTGS2, ALOX12 and ALOX15, while another was cytochrome P450 family 17 subfamily A member 1 (CYP17A1), hydroxy-delta-5-steroid dehydrogenase (HSD3B2), CYP21A2, CYP11A1 and steroid sulfatase (STS). For most of the active components, the target proteins affecting the disease pathway through PTGS2, only Tan IIA showed a regulatory function on both parts, which may be a reason for its marketable pharmacological effects. The PPI network results revealed PTGS2 was the foremost disease pathway protein regulated by the drug targets, which was consistent with our metabolomics results and the physiological function of PTGS2. Therefore, the target proteins that can be connected with PTGS2 were regarded as the direct targets of the active components in SM, which were highlighted with a blue line. In this way, the direct targets of the active components were identified as follows. Endothelin 1 (EDN-1), signal transducer and activator of transcription 3 (STAT3) were found as the direct targets of Sal. A while mitogen-activated protein kinase 8 (MAPK8), interleukin 4 (IL4) and STAT3 were the direct targets of Sal. B. In the network of danshensu, B-cell leukemia/lymphoma 2 (BCL2) and heme oxygenase 1 (HMOX1) can be connected with PTGS2 directly, which have been identified as direct targets. The network of Tan IIA was the most complicated and seven direct targets were discovered, namely MAPK1, tumor protein p53 (TP53), EDN-1, matrix metallopeptidase 2 (MMP2), hydroxyprostaglandin dehydrogenase 15 (HPGD), KRAS proto-oncogene, GTPase (KRAS) and MMP9. It is noteworthy that EDN-1 was the direct target for both Tan IIA and Sal. A, which may be a crucial target for SM and valuable to study in depth. The PPI network plays a crucial role in visualizing the specific interactions between the drug targets and disease pathway proteins, which manifests promising advantages towards revealing the correlation between drugs and diseases.
Biological validation
PPI network analysis is a useful tool to reveal the mechanism of a drug in a multi-target system. Clarification of the critical disease proteins and drug targets is the foundation for further elucidating the correlation between drugs and disease. Herein, western blot and Co-IP analyses were used to verify the results of the PPI network. The validation experiments investigated two aspects, target protein identification and their interaction. PTGS2 was the most critical disease pathway protein while EDN-1 was a direct target protein related by Tan II A and Sal. A, which were most suitable for biological validation. Western blotting analysis and co-immuno precipitation were used to validate the protein expression and protein–protein interactions.
The biological validation results are presented in Fig. 4. The western blot results showed the higher expression of PTGS2 in the model group and after treatment with Sal. A, the content decreased (Fig. 4A). This infers that PTGS2 is a key disease protein and Sal. A displayed an obvious regulatory effect, which was consistent with the results of the PPI network. The results for EDN-1 also supported the discovery in the PPI network (Fig. 4B). EDN-1 was predicted as a drug target of Tan IIA and there was a similar protein expression in the control and model groups. While after treatment with Tan IIA, the expression level of EDN-1 increased when compared with the control and model groups, which provided evidence that Tan IIA displayed a regulatory effect on EDN-1 expression. This indicated that EDN-1 was the common target of Sal. A and Tan IIA (Fig. 3C and E), and the drug performed its action through the protein–protein interaction of PTGS2 and EDN-1. Co-IP was applied to represent the interaction between the disease pathway protein and drug target protein, and this interaction was enhanced when treated with Tan IIA and Sal. A (Fig. 4C). The biological validation results support the conclusion of the network pharmacology in which PTGS2 and EDN-1 are the disease protein and drug target, respectively. Their interaction plays a crucial role in the prevention and treatment of early AS.
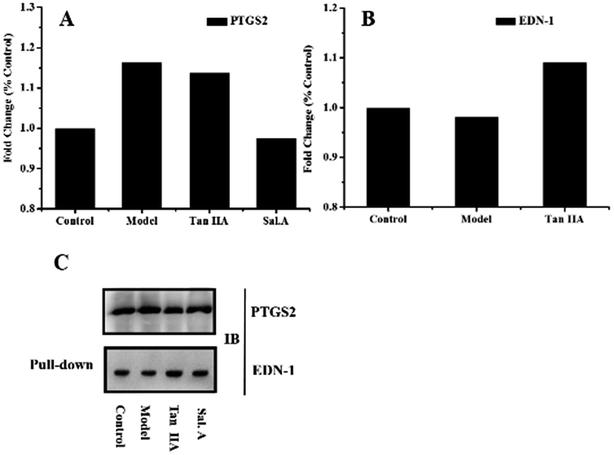 |
| Fig. 4 Biological validation results. (A) and (B) Western blot results obtained for PTGS2 and EDN-1 in different groups; (C) co-immuno precipitation results obtained for PTGS2 and EDN-1. | |
Intermolecular transfer process of drug effect
Interpreting the relevance of components, efficacy and discovering new drug targets have been the hot topics and challenges of TCM modernization. The unclear relationship between the components and the target is a great barrier for TCM research and also restricts its clinical applications. Metabolomics and network pharmacology are important platforms for the pharmacological study of TCM, which can explain the overall outline of the biological system from a macroscopical view and also depict dynamic process of specific metabolic pathways on a micro-level. The curative effect of a drug is transferred from upstream drug targets to the downstream disease pathway proteins, which then regulates the metabolic state of an organism. Elucidating the intermolecular transfer process of the drug effect based on a drug target proteins-disease pathway proteins network is beneficial towards revealing the specific way of drug intervention. In this article, Tan IIA-EDN1-PTGS2-anandamide was identified as a major intervention pathway of Tan IIA on early AS based on the strategy of the integration of metabolomics and network pharmacology. Clarifying the effective association of the component-target and disease–drug via decomposing the complex target network into several transfer processes of drug effect can help simplify the complex system and guide its clinical application effectively.
Conclusions
In summary, a strategy based on the integration of metabolomics and network pharmacology was established in this article to reveal the molecular mechanism of TCM and intermolecular transfer process of drug effects. The PPI networks of disease pathway proteins and target proteins of the active components in SM were constructed, respectively. The typical intermolecular transfer process of Tan IIA (Tan IIA-EDN1-PTGS2-anandamide) was identified and validated using a biological approach. This work provided a practical way for elucidating the relevance of the ingredients and their therapeutic effect, which is a breakthrough towards revealing the specific pathways regulated by the ingredients.
Author contributions
H. B. Xiao and A. Liu designed the research. W. J. Xu conducted the experiments, analyzed the data and drafted the manuscript. L. M. Chen conducted the network analysis. Z. Y. Wei and J. J. Dong performed the cell model and sample preparation. P. Q. Wang, Z. X. Jia and J. Liu discussed the data. J. Yang helped to conduct the cell model. Z. C. Ma and R. B. Su co-worked on the reagents, materials and prepared figures. All the authors approved the final manuscript.
Conflicts of interest
There are no conflicts to declare.
Acknowledgements
This work was financially supported by the National Natural Science Foundation of China (No. 81703947 and 81573839) and China Postdoctoral Science Foundation (2016M591362).
References
- C. Maziere and J. Maziere, Free Radical Biol. Med., 2009, 46, 127–137 CrossRef CAS PubMed.
- G. Maiolino, G. Rossitto, P. Caielli, V. Bisogni, G. P. Rossi and L. A. Calò, Mediators Inflammation, 2013, 714653 Search PubMed.
- W. Chen and G. Chen, Curr. Pharm. Des., 2017 DOI:10.2174/1381612823666170822101112.
- S. Yu, B. Zhong, M. Zheng, F. Xiao, Z. Dong and H. Zhang, J. Altern. Complement. Med., 2009, 15, 557–565 CrossRef PubMed.
- Y. J. Wu, C. Y. Hong, S. J. Lin, P. Wu and M. S. Shiao, Arterioscler., Thromb., Vasc. Biol., 1998, 18, 481–486 CrossRef CAS.
- Q. Liu, J. Li, A. Hartstonerose, J. Wang, J. Li, J. S. Janicki and D. Fan, J. Evidence-Based Complementary Altern. Med., 2015, 752610 Search PubMed.
- T. L. Yang, F. Y. Lin, Y. H. Chen, J. J. Chiu, M. S. Shiao, C. S. Tsai, S. J. Lin and Y. L. Chen, J. Sci. Food Agric., 2011, 91, 134–141 CrossRef CAS PubMed.
- X. Liu, W. Y. Wu, B. H. Jiang, M. Yang and D. A. Guo, Trends Pharmacol. Sci., 2013, 34, 620–628 CrossRef CAS PubMed.
- J. Shi, B. Cao, X. W. Wang, J. Y. Aa, J. A. Duan, X. X. Zhu, G. J. Wang and C. X. Liu, J. Chromatogr. B, 2016, 1026, 204 CrossRef CAS PubMed.
- M. Wang, L. Chen, D. Liu, H. Chen, D. D. Tang and Y. Y. Zhao, Chem.-Biol. Interact., 2017, 273, 133–141 CrossRef CAS PubMed.
- Y. Y. Zhao and R. C. Lin, Chem.-Biol. Interact., 2014, 215, 7–16 CrossRef CAS PubMed.
- Y. Q. Liu, L. B. Zhan, T. G. Liu, M. C. Cheng, X. Y. Liu and H. B. Xiao, J. Ethnopharmacol., 2014, 157, 206–211 CrossRef CAS PubMed.
- Y. Y. Zhao, S. P. Wu, S. M. Liu, Y. M. Zhang and R. C. Lin, Chem.-Biol. Interact., 2014, 220, 181–192 CrossRef CAS PubMed.
- Y. Y. Zhao, X. L. Cheng, N. D. Vaziri, S. M. Liu and R. C. Lin, Clin. Biochem., 2014, 15, 16–26 CrossRef PubMed.
- Y. Y. Zhao, X. L. Cheng, F. Wei, X. Bai, X. J. Tan, R. C. Lin and Q. B. Mei, J. Proteome Res., 2013, 12, 692–703 CrossRef CAS PubMed.
- Z. Fang, B. Lu, M. Liu, M. Zhang, Z. Yi, C. Wen and T. Shi, PLoS One, 2013, 8, e72334 CAS.
- Y. Xu, D. Dou, X. Ran, C. Liu and J. Chen, J. Chromatogr. A, 2015, 1416, 103–111 CrossRef CAS PubMed.
- W. J. Xu, Z. Y. Wei, J. J. Dong, F. P. Duan, K. K. Chen, C. Chen, J. Liu, X. W. Yang, L. M. Chen, H. B. Xiao and A. Liu, Front. Pharmacol., 2017, 8, 586 CrossRef PubMed.
- M. S. Shiao, J. J. Chiu, B. W. Chang, J. Wang, W. P. Jen, Y. J. Wu and Y. L. Chen, Biofactors, 2008, 34, 147–157 CrossRef CAS PubMed.
- Y. G. Li, L. Song, M. Liu, H. Zhi Bi and Z. T. Wang, J. Chromatogr. A, 2009, 1216, 1941–1953 CrossRef CAS PubMed.
- S. Gao, Z. Liu, H. Li, P. J. Little, P. Liu and S. Xu, Atherosclerosis, 2012, 220, 3–10 CrossRef CAS PubMed.
- G. Papadatos and J. P. Overington, Futures, 2014, 6, 361–364 CAS.
- M. Kuhn, D. Szklarczyk, A. Franceschini, C. Von Mering, L. J. Jensen and P. Bork, Nucleic Acids Res., 2011, 40, D876–D880 CrossRef PubMed.
- H. Mi, X. Huang, A. Muruganujan, H. Tang, C. Mills, D. Kang and P. D. Thomas, Nucleic Acids Res., 2017, 45, D183–D189 CrossRef PubMed.
- Y. Y. Chen, F. Y. Meng, H. Fang, Y. N. Yu, J. Liu, Z. W. Jing, A. P. Lv, Z. Wang and Y. Y. Wang, CNS Neurol. Disord.: Drug Targets, 2013, 12, 882–893 CAS.
- O. D. Iancu, D. Oberbeck, P. Darakjian, S. Kawane, J. Erk, S. McWeeney and R. Hitzemann, PLoS One, 2013, 8, e58951 CAS.
- H. Gardener, A. Beecham, D. Cabral, D. Yanuck, S. Slifer, L. Wang, S. H. Blanton, R. L. Sacco, S. H. H. Juo and T. Rundek, Stroke, 2011, 42, 889–896 CrossRef PubMed.
- K. M. Borow, J. R. Nelson and R. P. Mason, Atherosclerosis, 2015, 242, 357–366 CrossRef CAS PubMed.
- T. W. H. Pols, M. Nomura, T. Harach, G. L. Sasso, M. H. Oosterveer, C. Thomas, G. Rizzo, A. Gioiello, L. Adorini, R. Pellicciari, J. Auwerx and K. Schoonjans, Cell Metab., 2011, 14, 747–757 CrossRef CAS PubMed.
- X. X. Yang, W. W. Zhang, Y. L. Chen, Y. Li, L. Sun, Y. Liu, M. Y. Liu, M. Yu, X. J. Li, J. H. Han and Y. J. Duan, J. Biol. Chem., 2016, 291, 15108 CrossRef CAS PubMed.
- E. S. Jankun, J. Jankun and A. Al-Senaidy, Curr. Med. Chem., 2012, 19, 5122–5127 CrossRef.
- J. Z. Haeggström and C. D. Funk, Chem. Rev., 2011, 111, 5866–5898 CrossRef PubMed.
- K. B. Reilly, S. Srinivasan, M. E. Hatley, M. K. Patricia, J. Lannigan, D. T. Bolick, G. Vandenhoff, H. Pei, R. Natarajan, J. L. Nadler and C. C. Hedrick, J. Biol. Chem., 2004, 279, 9440–9450 CrossRef CAS PubMed.
|
This journal is © The Royal Society of Chemistry 2018 |
Click here to see how this site uses Cookies. View our privacy policy here.