DOI:
10.1039/C9RA06332C
(Paper)
RSC Adv., 2020,
10, 461-473
Comparative metabolomics unveils molecular changes and metabolic networks of syringin against hepatitis B mice by untargeted mass spectrometry†
Received
14th August 2019
, Accepted 9th December 2019
First published on 2nd January 2020
Abstract
Untargeted metabolomics technology was used to discover the metabolic pathways and biomarkers for revealing the potential biological mechanism of syringin on hepatitis B virus. Serum samples were analyzed by ultra-performance liquid chromatography-mass spectrometry (UPLC-MS)-based comparative metabolomics coupled with pattern recognition methods and network pathway. In addition, the histopathology, HBV DNA detection of liver tissue, and biochemical indicators of liver function change were also explored for investigating the antiviral effect of syringin. In comparison to the model group, the metabolic profiles of the turbulence in transgenic mice tended to recover to the same as the control group after syringin therapy. A total of 33 potential biomarkers were determined to explore the metabolic disorders in the hepatitis B animal model, of which 25 were regulated by syringin, and 8 metabolic pathways, such as phenylalanine, tyrosine and tryptophan biosynthesis, phenylalanine metabolism, arachidonic acid metabolism, glyoxylate and dicarboxylate metabolism, were involved. Syringin markedly reduced the liver pathology change, inhibited HBV DNA replication, and improved liver function. Amino acid metabolism is a potential target for the treatment of hepatitis B. The hepatoprotective effect of syringin may contribute to ameliorating oxidative stress and preventing protein and DNA replication. Comparative metabolomics is a promising tool for discovering metabolic pathways and biomarkers of the hepatitis B animal model as targets to reveal the effects and mechanism of syringin, which benefits the development of natural products and advances the treatment of diseases.
1. Introduction
According to the World Health Organization, approximately one-third of the world's inhabitants have experienced hepatitis B virus (HBV) contagion, and more than 350 million individuals are chronically affected with HBV.1–4 To date, there is still no effective drug to completely eliminate the HBV from the body for the millions of chronically infected people. Patients suffering from HBV for a long time may succumb to end-stage liver hardening and hepatocellular cancer.5–7 HBV-infected patients exhibit many negative emotions, such as depression and anxiety disorder, on account of its incurable nature and increasing psychological pressure, such as depression from the misunderstanding of the infectivity of HBV in society.8,9 Developing anti-HBV agents and seeking treatment programs is still a pressing requirement in the field of HBV research.
There is growing enthusiasm to explore medicines with multiple aims or functional products by all experts and scholars in the pharmaceutical industry alongside the advancement of modern science and the increase in social needs.10 Herbal medicines have been applied in treating diverse sicknesses throughout history and can achieve marked multiple-target effects. Natural products that offer the benefits of heterogeneity, renewability, low cost, and low side effects have been utilized in treating various diseases for thousands of years. Ever since Youyou Tu won the “2015 Nobel Prize in Physiology or Medicine,” natural products originating from herbal medicines have realized huge advances.11,12 Syringin, also called eleutheroside B, is a principal bioactive phenolic glycoside isolated from nature13 and has immunomodulatory and anti-inflammatory effects. It attenuates insulin resistance, suppressing low-grade chronic inflammation and ER stress in diabetic mice, and raises insulin secretion by releasing acetylcholine.14–16 Syringin blocks bone loss by the TRAF6-arbitrated suppression of NF-κB and the acceleration of PI3K/AKT in ovariectomized mice, and blocks adjuvant arthritis by modulating the immune function of abnormal cells and the balance of cytokines.17,18 In addition, syringin can help sleep activity through the NOS/NO pathway, impede cardiac hypertrophy caused by pressure overload by the attenuation of autophagy, and inhibit LPS-induced acute lung injury through operating Nrf2 and restraining the NF-κB signaling pathway.19–21 Syringin may reduce cell toxicity in caspase-3 activity and expression, cleave PARP, and reduce DNA fragmentation.22 Syringin is a promising multi-target candidate to fight hepatitis B, but little is known of the latent operational process of syringin on hindering the pathological changes of the hepatitis B animal model at the metabolic level.23
Recently, metabolomics has been increasingly utilized to probe into virus infection, pathogenesis, and treatment.24 As a rapidly emerging omics field after genomics and proteomics, metabolomics concentrates on a quality and purity analysis of endogenic metabolites, which generates holistic information of metabolites and their pathways in various biological processes.25 Metabolites are final downstream products and can be studied to characterize the global and dynamic profiling of disease occurrence and development.26,27 Compared with targeted metabolomics, non-targeted metabolomics were absorbed in an all-sided reportage analysis of metabolome under a supposed generated circumstance.28,29 It is usually applied to detect metabolic pathways of disease to explore the distinguished metabolites by non-targeted metabolic research.30–33 In this study, we took HBV transgenic animals as objects to tentatively identify the potential disturbed metabolic biomarkers and pathways for exploring the antiviral effect of syringin to aid basic research into drug development for HBV treatment.
2. Materials and methods
2.1 Materials and reagents
Analytically pure absolute ethyl ethanol was obtained from Shanghai Chemical Works (Beijing, China). HPLC grade acetonitrile (ACN) and formic acid (FA) were obtained from Merck (Darmstadt, Germany). Water without ions was obtained by using a Milli-Q water ultrapure water system (Millipore, Bedford, MA, USA). Leucineen kephalin was purchased from Invitrogen Life Technologies (Carlsbad, CA, USA). The assay kits for alanine amino-transferase (ALT), aspartate aminotransferase (AST), total bilirubin (TBIL), direct bilirubin (DBIL), hyaluronic acid (HA), laminin (LN), and precollagen III (PC III) were obtained from Sigma-Aldrich (St. Louis, MO, USA). The enzyme-immunoassay (EIA) kits for the detection of hepatitis B surface antigen (HBsAg), hepatitis B envelope antigen (HBeAg), interleukin-10 (IL-10), and interferon-γ (IFN-γ) were all purchased from Axygen Biosciences (Union City, CA, USA). TRIzol extracting solution and the reverse transcription kit were purchased from Omega Bio-Tek, Inc. (Norcross, GA, USA). Pentobarbital sodium was purchased from Shanghai Chemical Reagent Purchasing and Supply Station, China. Sodium chloride injections were obtained from Harbin Sanlian Pharmaceutical Co., Ltd (Heilongjiang, China). Syringin (purity of more than 99%) was purchased from Shanghai Kehua Bio-engineering Co., Ltd (Shanghai, China). All other reagents were of analytical grade.
2.2 Animal models and treatment
A total of 50 HBV transgenic (HBV-Tg) BALB/c mice (male) and 10 healthy C57BL/6 mice (male) aged eight weeks and weighing 18–25 g were purchased from the Shanghai Infectious Disease Center Hospital. All the HBV mice had a solitary copy of the terminal residue, 1.3-genome length copy of the HBV genome, consolidated into their chromosomal DNA, which led to high levels of HBV replication in the livers of the mice. The mice applied in the breeding test were homozygous compared with the HBV transgene. The mice were raised in specific pathogen-free cages with 12 h light/dark cycles from 08:00 to 20:00, controlled temperatures of 22–26 °C, and humidity of 45–55%. After acclimatizing for one week, the 50 HBV-Tg mice were allocated with 10 mice to each group model group and four different syringin-treated groups at 2, 4, 6, 8 weeks. The syringin-treated group was managed by the administration of 10 mg mL−1 syringin medicinal liquid twice a day by gavage. The control group was treated with distilled water in an identical way. The experimental procedures were approved by the Animal Care and Ethics Committee at Heilongjiang University of Chinese Medicine and all the experiments were performed in accordance with the Helsinki declaration.
2.3 Biochemical indicators and histopathology examination
Blood samples from the different syringin-treated groups were respectively collected from aorta abdominalis at 24 h after the final administration of 2, 4, 6, 8 weeks. Meanwhile, blood samples in the healthy and transgenic group were collected in the same way at 8 weeks. Serum samples after processing through centrifugation at 2800 rpm, 4 °C, for 15 min were delivered to the tubes and deposited in liquid nitrogen to await the clinical biochemistry tests and HBV antigen inspection, as surveyed by ELISA kits following the manufacturer's instructions. Animals were sacrificed using an enterocoelia injection of 2% pentobarbital sodium (0.1 mL/100 g, weight) and the livers were removed promptly, with one part utilized for hematoxylin and eosin (H&E) staining resolution and the other utilized for liver HBV DNA replication intermediates analysis. The obtained pictures were analyzed by SPSS 19.0 software. H&E image analysis was performed by Image-Pro Plus 5.0 software (Media Cybernetics, Bethesda, MD, USA).
2.4 Liver HBV DNA replication examination
The liver samples were taken out from the −80 °C refrigerator, and liquid nitrogen was added for grinding them into powder. The total RNA was extracted with TRIzol solution, and the DNA was synthesized by reverse transcription according to the reverse transcription kit specification that was stored at −20 °C until use. The reaction system contained various items, including 2 μL of c DNA, 10 μL of q PCR mix, 1 μL of primer F, and 1 μL of primer. The reaction conditions were set at: 95 °C for 2 min, 94 °C for 20 s, 60 °C for 20 s, and 72 °C for 30 s in 40 cycles.
2.5 Metabolomics study
Sample processing. After thawing at 4 °C, the serum samples were further processed, whereby 100 μL of the sample mixed with 500 μL methanol for protein removal was eddied for 30 s, then, the mixture sample was centrifuged at 12
000 rpm, 4 °C for 15 min. The liquid supernatant were delivered to the new centrifuge tube and was shattered to dryness under nitrogen gas. The dried residue were redissolved in buffer, including 28% acetonitrile, 37% methanol, and 35% water, and centrifuged at 13
000 rpm, 4 °C for 15 min for eliminating any particulates. The gained supernatant was used for metabolomics analysis.
UPLC-MS condition. UPLC was conducted on a Waters ACQUITY UPLC system (Waters Corporation, Milford, MA) equipped with an ACQUITY UPLC HSS T3 column (2.1 mm × 100 mm, 1.8 μm) and Masslynx control system. The injection volume was 3 μL under 0.5 mL min−1 and the column was set at 40 °C. All the samples were held at 4 °C in the sample custody package. The best mobile phase followed a linear procedure of adding 0.1% formic acid in water (A) and 0.1% formic acid in acetonitrile (B), and the parameters were set at: 0–1 min, 1% B; 1–2 min, 1–9% B; 2–5 min, 9–15% B; 5–7 min, 15–35% B; 7–9 min, 35–50% B, 9–10 min 50–99% B. QC sampling was performed six times for ensuring the endurance and persistent of the platform. A needle rinse period was performed to wipe off the residue for preparing the subsequent injection. The exported eluants were immediately transmitted to the mass spectrometer without separation. Nitrogen was regarded as the sprayer with eluant gas at 50 L h−1 and 550 L h−1, respectively.MS was carried out on a Waters Micromass QTOF Synapt High Definition Mass Spectrometer (Manchester, UK) matched with a positive and negative ion mode electrospray source. Under the positive ion mode, the main parameters were selected as: capillary voltage, 3.0 kV; cone voltage, 25 V; cone gas flow, 320 L h; and ion source temperature, 320 °C. Under the negative ion mode, the main parameters were selected as: capillary voltage, 2.4 kV; cone voltage, 20 V; cone gas flow, 320 L h; and ion source temperature, 430 °C. The scan time was regulated at 0.2 s, with 0.1 s halt. In the centroid mode, original data were captured between m/z 100 and 1000 in both modes. The leucine enkephalin was infused at 100 mL min−1 into the instrument for assuring the accuracy, which offers a check for the positive ion mode and negative ion mode. Ten characteristic peaks were picked out for the method validation based on the metabolite polarities and m/z values; meanwhile, the relative standard deviation% (RSD%) of Rt and the peak areas were determined as an evaluation index. The consecutive analyses were performed of six replicates from the QC samples in injection precision tests, in which the RSD% of the retention time and peak area were 0.53% and 2.2%, respectively. The six parallel samples were separately injected into the UPLC-MS for sample preparation repeatability evaluation, in which the RSD% of Rt and the peak area were 0.71% and 2.7%, respectively.
Data processing. Raw data from the UPLC/MS were analyzed by MassLynx V4.1 software (Waters Corporation, Milford, USA), including the height intensities peak detection, isotope masses, and calibration of Rt and the mass (m/z). As a MarkerLynx Application Manager, EZinfo2.0 software was utilized further for the multivariate data analyses, such as through unsupervised principal component analysis (PCA), orthogonal projection to latent structure-discriminant analysis (OPLS-DA), and a variable importance in projection (VIP) score plot for better understanding the similarities and differences in the processed data. The statistical models ranges were predicted and used to predict the metabolic phenotypes and to distinguish the metabolites. For the PCA scores plots, it was indicated that similar metabolic constituents exhibited a small scatter range, while differential metabolites were dispersed. OPLS-DA has the ability to discover the most distinction traits among different groups and the obtained VIP-plots were applied to extract potential biomarkers. The results from the validation test using the goodness of fit (R2 and Q2) of the PLS models were compared with the goodness of fit of 200 Y-permutated models to evaluate the validity of the PLS model. Metabolic peak areas with a VIP exceeding 1 and p-value lower than 0.05 in the Student's t-test were seem as potential biomarkers. RT, precise MS, MS/MS data, and online databases, such as Chempider, HMDB, and KEGG, were used for identification of the metabolites. Biological information of the pathways and networks were analyzed by MetaboAnalyst 4.0 software. All the statistical arrays were dealt with the mean-centered and pared to scale. Data are expressed here as the mean and standard deviation (SD) with the two-tailed, two-sample Student's t-test, with differences valued lower than 0.05 assessed as statistically significant.
3. Results
3.1 Biochemical analysis and histopathological observations
As shown in Fig. S1,† in comparison with the control group, the HBV-Tg mice had a higher concentration of ALT, AST, TBIL, DBIL, LN, HA, PC III, IFN-γ, HBsAg, HBeAg, and HBv-DNA, and a low concentration of IL-10. After the intragastric administration of syringin at different stages, all of the abnormal indicators were gradually relieved and the highlighted differences were found on most of the indexes compared to the model samples, in which syringin had a prominent inhibitory effect on anti-HBV DNA copy from the 2nd week, while TBIL, DBIL, and HBeAg levels from the 4th week were remarkably changed (p < 0.01), and ALT, AST, LN, HA, IL-10, IFN-γ, and HBsAg levels from the 6th week were remarkably changed (p < 0.01). The results indicated that syringin worked against hepatitis B by improving liver function, inhibiting liver fibrosis, enhancing immunity, and impeding viral replication.
Significant differences were shown in ethology, in which the control mice were evaluated in active and normal states, while the mice in the model group were seen to be restless, aggressive, and had malaise and lethargy. HE staining was carried out to examine the hepatic organization pathology from the model, control, and syringin-treated groups, as seen in Fig. S2.† The liver tissue structure of the control group of was normal and no conspicuous abnormalities were seen, such as lipid droplets, protuberance, and inflammatory cell infiltration. Hepatic organization seen in the transgenic mice exhibited unordered hepatocyte cords and hepatic lobule structure, swollen cell morphology, abnormal cell nuclear atrophy, critical greasy deterioration and fibrosis, spotty or burnt gangrene and obvious inflammatory cells soaking. Hemorrhagic gangrene around the fibrosis tissue could also be easily noticed. However, compared with the model group, the treatment group had a significant relieve of the cell swelling, and balloon-like changes, as well as hepatic sinus congestion. It was suggested that syringin could ameliorate hepatitis B injury.
3.2 Metabolic biomarkers analysis
Serum metabolomics was applied to gain an insight into the potential antiviral effect of the syringin hepatitis B animal model. In the positive and negative ion modes, serum metabolic profiling was performed, showing that the clusters of endogenous metabolites were intuitively embodied by the basic approaches consisting of PCA, PLS, and OPLS-DA. In Fig. 1A and 2A, the model and control groups can be evidently seen to be detached, indicating pathological change has taken place at the metabolic level. The 3D OPLS-DA score plots shown in Fig. 1B and 2B indicate the obvious credibility in the model quality evaluation and prediction capability between the control and model groups, with Comp1 R2X (cum) = 0.9128, Comp2 R2X (cum) = 0.9128 in the positive mode and Comp1 R2X (cum) = 0.9844, Comp2 R2X (cum) = 0.9844 in the negative mode. The S-plot and VIP score plots in both ion modes, respectively, emerged in Fig. 1C, D and 2C, D. After multivariate analysis, 33 differentially expressed metabolites meeting the threshold of VIP of more than 1 and a p-value less than 0.05 were selected and identified to distinguish the healthy mice and hepatitis B model mice in Table S1.† Animals in the syringin group brought out different tracks in the metabolic profiles with respect to the model group, which tended to progressively track the control condition with 2, 4, 6, 8 weeks change. The obvious metabolic path of PCA score plots in Fig. 3A and B was suggested that syringin treatment had a protective effect to reverse the serum biochemical disorder resulting from hepatitis B and that the therapeutic response of syringin was most prominent at 8 weeks. The contents of 25 biomarkers were regulated by syringin in the transgenic mice toward normal levels. In Fig. 4A, the interrelation of the correlation matrix of these was shown in a heatmap by Pearson's linear correlation analysis, in which the brightness scale suggests the different degrees of magnitude and the direction in the control, model, and syringin groups after 8 weeks treatment. Compared with the control group, 14 metabolites were increased and 11 metabolites declined, with the relative signal intensities of the serum metabolites presented in Fig. 4B–D.
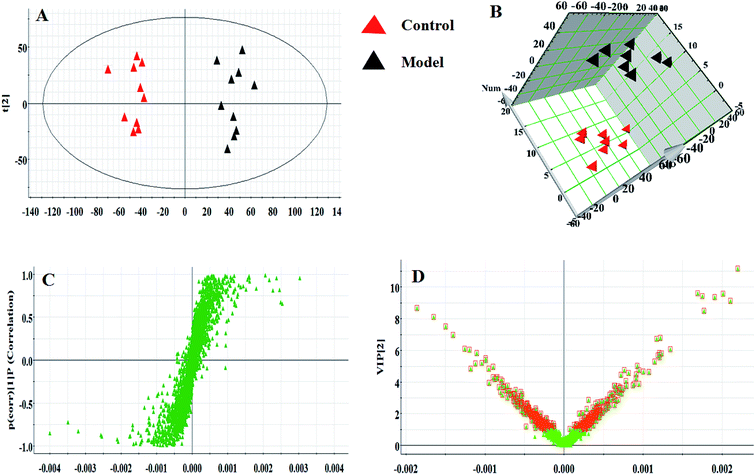 |
| Fig. 1 Multivariate data analysis of the serum samples in the positive ion mode. (A) The PCA score plot between the control and model groups. (B) The OPLS-DA score plot between the control and model groups. S-plot (C) and VIP-score plot (D) of OPLS-DA between the control and model groups. Red symbols in the VIP-score plot indicate the ions with a VIP > 1. | |
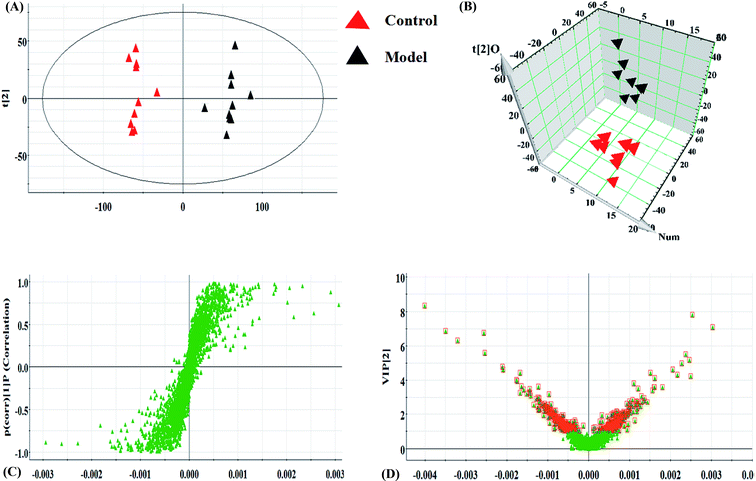 |
| Fig. 2 Multivariate data analysis of the serum samples in the negative ion mode. (A) The PCA score plot between the control and model groups. (B) The OPLS-DA score plot between the control and model groups. S-plot (C) and VIP-score plot (D) of OPLS-DA between the control and model groups. Red symbols in the VIP-score plot indicate the ions with a VIP > 1. | |
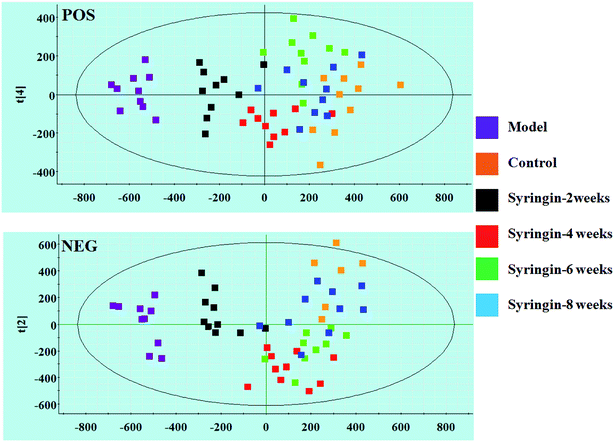 |
| Fig. 3 PCA scores plot of the serum metabolism trajectory among the control, model, and the syringin groups at different time points in positive and negative ion modes. | |
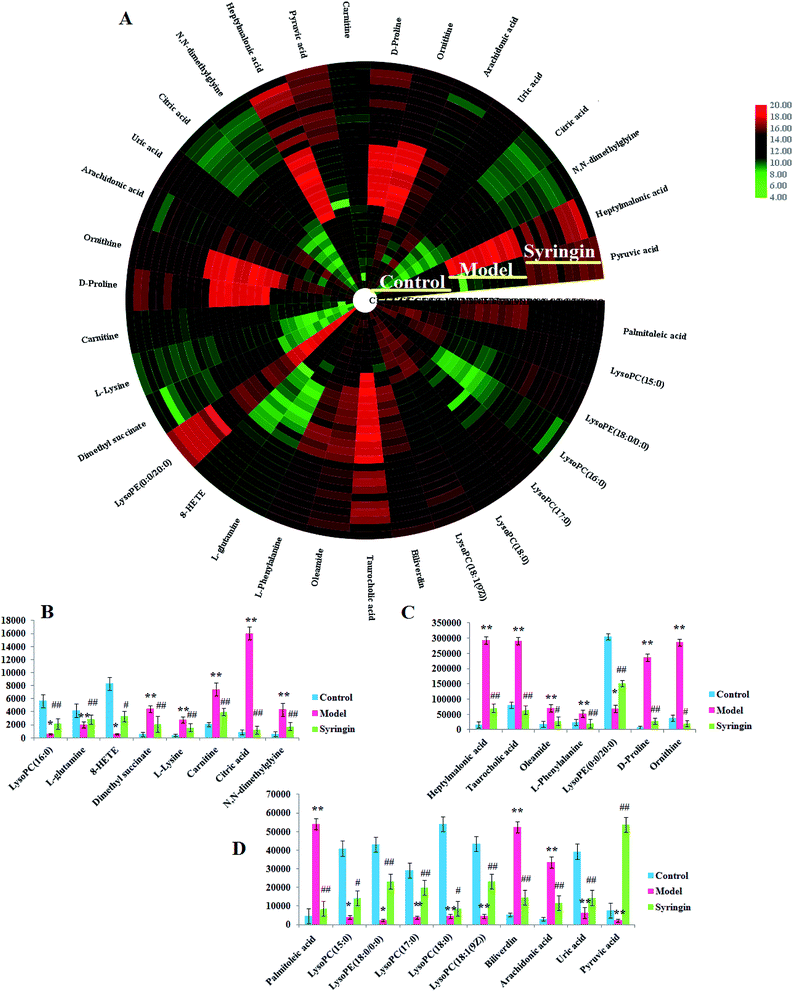 |
| Fig. 4 Hierarchical clustering heat map of the 25 differential metabolites regulated by syringin in the 8th week with the degree of change marked with colors, including upregulation (red) and downregulation (green) (A). The relative intensities of the metabolic biomarkers in the serum sample identified from the control, model, and 8 week syringin groups (B)–(D). Compared with the control group: *p < 0.05, **p < 0.01; compared with the model group, #p < 0.05, ##p < 0.01. | |
3.3 Metabolic pathway and network analysis
MetPA analysis is an unrestrained web-based instrument based on the MetaboAnalyst platform, and it indicated that 25 differentiated metabolites in syringin treatment were mainly involved in phenylalanine, tyrosine and tryptophan biosynthesis, phenylalanine metabolism, arachidonic acid metabolism, glyoxylate and dicarboxylate metabolism, pyruvate metabolism, alanine, aspartate and glutamate metabolism, arginine and proline metabolism, and the citrate cycle. Their pathway impact values were respectively 0.500, 0.407, 0.3296, 0.296, 0.187, 0.149, 0.127, 0.125, as shown in Fig. 5. As shown in Fig. 6A, the KEGG global metabolic network offers the visual exploration of the results for mapping the metabolites and enzymes closely associated with the antiviral effect of syringin, such as L-phenylalanine, ornithine, L-glutamine, citrate and pyruvate in phenylalanine metabolism, D-arginine and D-ornithine metabolism, D-glutamine and D-glutamate metabolism, TCA cycle and alanine, aspartate and glutamate metabolism, which is conducive for integrating metabolomics and metagenomics studies. In Fig. 6B, the potential functional relationships between a wide set of metabolites is highlighted in the metabolite–metabolite interaction network, which mainly refers to pyruvic acid, citric acid, L-lysine, L-glutamine, ornithine, L-phenylalanine, arachidonic acid, uric acid, D-proline, neotrehalose, and palmitoleic acid with similar chemical structures and similar molecular activities. From the gene–metabolite interaction network in Fig. 7B, it can be seen that the antiviral effect of syringin in the hepatitis B animal model is associated with arachidonic acid, citric acid, ornithine, L-lysine, L-glutamine, uric acid, pyruvic acid, L-phenylalanine due to linking the potential therapeutic targets.
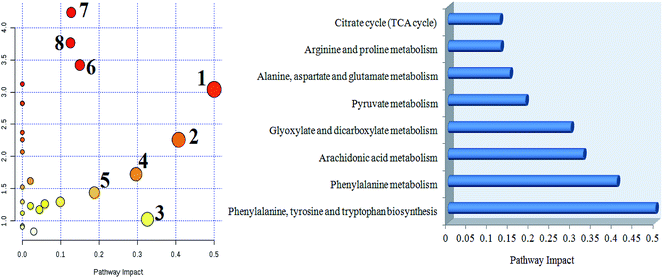 |
| Fig. 5 Pathway analysis of the antiviral effect of syringin. (1) Phenylalanine, tyrosine, and tryptophan biosynthesis; (2) phenylalanine metabolism; (3) arachidonic acid metabolism; (4) glyoxylate and dicarboxylate metabolism; (5) pyruvate metabolism; (6) alanine, aspartate and glutamate metabolism; (7) arginine and proline metabolism; (8) citrate cycle (TCA cycle). The histogram on the right shows the pathway impact value of the eight pathways closely related to the antiviral effect of syringin. | |
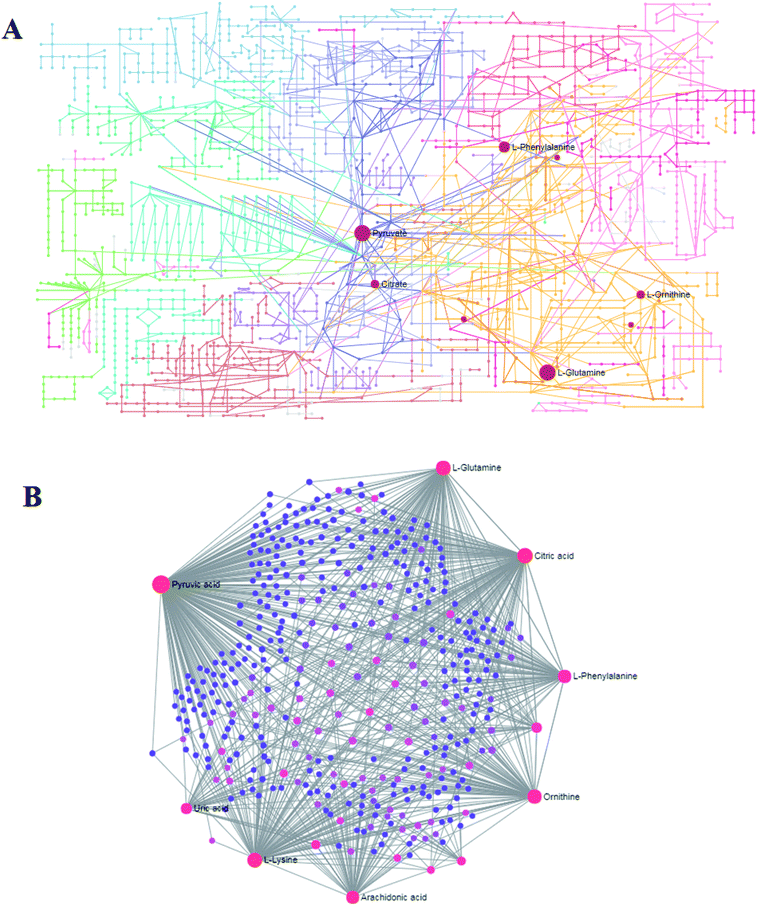 |
| Fig. 6 The global metabolic network associated with the antiviral effect of syringin (A). Metabolite–metabolite interaction network associated with the antiviral effect of (B). | |
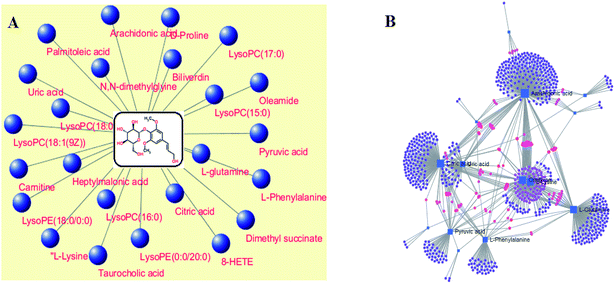 |
| Fig. 7 The relationship between syringin and the influential metabolites in resisting hepatitis B (A); the gene–metabolite interaction network of the antiviral effect of syringin enables the exploration and visualization of the interactions between the functionally related metabolites and genes in hepatitis B treatment (B). | |
4. Discussion
Untargeted metabolomics has been used to discover the metabolite biomarkers of diseases for disease diagnosis,34–41 pathological mechanisms,42–53 and therapeutic effects.54–59 Liquid chromatography-mass spectrometry (LC-MS) is widely used in metabolic analysis.60–71 It can monitor small molecule metabolites changes in biological samples.72–83 In this study, we used the untargeted metabolomics technology to discover the metabolic pathways and biomarkers to reveal the potential biological mechanism of syringin on the hepatitis B virus. The immunohistochemistry results showed that monocytes infiltrated the histopathological analysis and were present in the liver of mice, which was consistent with the pathological changes occurring with chronic hepatitis B.84 In addition, the clinical manifestations and immunological responses of transgenic mice are analogous to those of human hepatitis B virus carriers. Therefore, mice can be used as a model of chronic hepatitis B carrier status to study the infection mechanism of HBV.85 More than 90.2% of chronic carriers have a certain degree of liver tissue lesions, and syringin can regulate the abnormal levels of ALT, AST, TBIL, and DBIL in the blood to close to normal. Clinical studies have found that different degrees of liver fibrosis are involved in the development and outcome of hepatitis B.86–91 After the liver tissue of the model mice was injured, syringin regulated the elevated abnormalities state of LN, HA, and PC in the blood, indicating the trend of liver fibrosis. IFN is an important biological function cytokine with antiviral, anti-proliferative, and immunomodulatory effects in the body. It mainly acts on the JAK–STaT1 pathway, and achieves its antiviral effect by the tyrosine phosphorylation of STAT, nuclear access, and activation of the transcription of interferon-responsive genes. IL-10 is mainly produced by Th2 cells and is an immunosuppressive factor with multi-directional biological activity, which can change the immune response of the body, the expression of MGC-like antigens, and mediates the interaction between Th1 and Th2 cells. If the secretion of the cytokine IFN is inhibited, the body's antiviral immunomodulatory ability is decreased. Compared with the model group, syringin could increase autoimmunity in the model mice by reducing IL-10 levels and increasing IFN levels.92 HBeAg is an indicator of hepatitis B virus replication, in which a positive reaction indicates that hepatitis B virus replication is active and the viral load is high. Syringin can effectively inhibit the increase in the content of HBeAg and HBsAg, and then inhibit the replication of HBV-DNA.93
The decomposition of aromatic amino acids, such as phenylalanine, tryptophan, and tyrosine, is mainly done in the liver. When the liver function is impaired, the decomposition of aromatic amino acids is reduced. This study showed that the content of L-phenylalanine increased in the model group, and syringin could reduce this by regulating phenylalanine, tyrosine, and tryptophan biosynthesis and phenylalanine metabolism activity.94 Arachidonic acid (AA) is an essential fatty acid in the human body. Its metabolites, such as prostaglandins, prostacyclin, and thromboxane, are involved in the regulation of various physiological functions of the liver and gall bladder. They have the ability to cause white blood cells chemotaxis, aggregation, adhesion to vascular endothelial cells, degranulation, and release of oxygen free radicals and lysosomal enzymes. They can also increase vascular permeability and stimulate bronchial mucus secretion. After syringin treatment, the rising level of AA in the model group was decreased, which affected the AA metabolism.95
Pyruvic acid plays a pivotal role in the metabolic linkage of the three major nutrients, whereby it achieves the mutual conversion of sugar, fat, and amino acids in the body using the acetyl CoA and the tricarboxylic acid cycle. Studies have shown that there are HBV particles in the pancreatic tissue of patients with hepatitis.96 It is speculated that HBV can directly damage pancreatic β cells, which leads to pancreatic β cells not secreting active insulin and producing inactive “pseudo-insulin.” Meanwhile, elevated ALT levels can increase the risk of renal tubules. An abnormal sugar threshold can aggravate tubular damage, suggesting that CHB patients are more likely to develop diabetic nephropathy.97 Keeping a close watch on liver enzymes is, therefore, important for preventing the development of diabetes in HBV patients.
Syringin had a tendency to call back the abnormal content of citric acid and pyruvic acid in the control group by regulating glyoxylic acid and dicarboxylic acid metabolism, pyruvate metabolism, and the citric acid cycle in the treatment of hepatitis B. Studies have shown that a decreased glutamate level can directly lead to a rapid decline in the immune system function of patients with hepatitis. The elevated ornithine seen in the model group could affect the urea circulation in the whole body of a patient, and the detoxification function of the body would then be disordered. In living organisms, proline is not only an ideal osmotic adjustment substance, but also acts as a protective substance for membranes and enzymes, which protects against growth under osmotic stress.98 Under osmotic stress, proline plays a protective role by regulating the cytoplasmic osmotic balance when harmful substances, such as free radicals, in the test mice were increased.99 Currently, the detection of serum biomarkers can be used as an indicator to reflect disease states.100–115 Syringin can regulate the levels of proline, ornithine, and glutamate to the level of control group by affecting alanine, aspartate and glutamate metabolism, and arginine and proline metabolism.
5. Conclusion
Using serum metabolism analysis based on high-throughput technologies, we were able to reveal the therapeutic effect of syringin on hepatitis B and to probe into the pivotal antiviral molecular mechanisms. Our results suggested that syringin dramatically perfected a pathological change in hepatitis B by improving liver function, inhibiting liver fibrosis, enhancing immunity, and impeding viral replication, in which 25 metabolites and 8 vital metabolic pathways were involved. Amino acid metabolism was shown to be a potential target for the treatment of hepatitis B. Comparative metabolomics is a promising tool for discovering metabolic pathways and the biomarkers of hepatitis B animal model as targets to reveal the effects and mechanism of syringin, which will benefit the development of natural products and advances the treatment of diseases.
Conflicts of interest
There are no conflicts to declare.
References
- C. Trépo, H. L. Chan and A. Lok, Hepatitis B virus infection, Lancet, 2014, 384(9959), 2053–2063 CrossRef.
- J. N. Zuckerman, Protective efficacy, immunotherapeutic potential and safety of hepatitis B vaccines, J. Med. Virol., 2006, 78, 169–177 CrossRef CAS PubMed.
- A. Bartholomeusz and S. Locarnini, Hepatitis B virus mutations associated with antiviral therapy, J. Med. Virol., 2006, 78(1), S52–S55 CrossRef CAS PubMed.
- M. A. Budzinska, N. A. Shackel and S. Urban, et al., Cellular Genomic Sites of Hepatitis B Virus DNA Integration, Genes, 2018, 9(7), E365 CrossRef PubMed.
- J. Zhang, L. Zong and Y. Wang, et al., Core gene insertion in hepatitis B virus genotype G functions at both the encoded amino acid sequence and RNA structure levels to stimulate core protein expression, Virology, 2019, 526, 203–213 CrossRef CAS PubMed.
- J. Huang, K. Zhang and W. Chen, et al., Switching to PegIFNα-2b leads to HBsAg loss in patients with low HBsAg levels and HBV DNA suppressed by NAs, Sci. Rep., 2017, 7(1), 13383 CrossRef PubMed.
- D. Mu, F. C. Yuan and Y. Chen, et al., Baseline value of intrahepatic HBV DNA over cccDNA predicts patient's response to interferon therapy, Sci. Rep., 2017, 7(1), 5937 CrossRef PubMed.
- H. P. Zhu, Y. R. Gu and G. L. Zhang, et al., Depression in patients with chronic hepatitis B and cirrhosis is closely associated with the severity of liver cirrhosis, Exp. Ther. Med., 2016, 12, 405–409 CrossRef PubMed.
- M. Cornberg, J. Jaroszewicz and M. P. Manns, et al., Treatment of chronic hepatitis B, Minerva Dietol. Gastroenterol., 2010, 56, 451–465 CAS.
- L. Scotti and M. T. Scotti, Multi-Target Drugs Against Metabolic Disorders, Endocr., Metab. Immune Disord.: Drug Targets, 2019, 19(4), 389–390 CrossRef CAS PubMed.
- L. Chen, X. Wang and Y. Liu, et al., Dual-target screening of bioactive components from traditional Chinese medicines by hollow fiber-based ligand fishing combined with liquid chromatography-mass spectrometry, J. Pharm. Biomed. Anal., 2017, 5(143), 269–276 CrossRef.
- W. Liu and Y. Liu, Youyou Tu: significance of winning the 2015 Nobel Prize in Physiology or Medicine, Cardiovasc. Diagn. Ther., 2016, 6(1), 1–2 CrossRef CAS PubMed.
- J. Y. Cho, K. H. Nam and A. R. Kim, et al., In vitro and in vivo immunomodulatory effects of syringin, J. Pharm. Pharmacol., 2001, 53(9), 1287–1294 CrossRef CAS PubMed.
- B. Kim, M. S. Kim and C. K. Hyun, Syringin attenuates insulin resistance via adiponectin-mediated suppression of low-grade chronic inflammation and ER stress in high-fat diet-fed mice, Biochem. Biophys. Res. Commun., 2017, 488(1), 40–45 CrossRef CAS PubMed.
- H. S. Niu, I. M. Liu and J. T. Cheng, et al., Hypoglycemic effect of syringin from Eleutherococcussenticosus in streptozotocin-induced diabetic rats, Planta Med., 2008, 74(2), 109–113 CrossRef CAS PubMed.
- K. Y. Liu, Y. C. Wu and I. M. Liu, et al., Release of acetylcholine by syringin, an active principle of Eleutherococcus senticosus, to raise insulin secretion in Wistar rats, Neurosci. Lett., 2008, 434(2), 195–199 CrossRef CAS PubMed.
- J. Liu, Z. Zhang and Q. Guo, et al., Syringin prevents bone loss in ovariectomized mice via TRAF6 mediated inhibition of NF-κB and stimulation of PI3K/AKT, Phytomedicine, 2018, 15(42), 43–50 Search PubMed.
- Y. Y. Song, Y. Li and H. Q. Zhang, Therapeutic effect of syringin on adjuvant arthritis in rats and its mechanisms, Yaoxue Xuebao, 2010, 45(8), 1006–1011 CAS.
- Y. Cui, Y. Zhang and G. Liu, Syringin may exert sleep-potentiating effects through the NOS/NO pathway, Fundam. Clin. Pharmacol., 2015, 29(2), 178–184 CrossRef CAS PubMed.
- F. Li, N. Zhang and Q. Wu, et al., Syringin prevents cardiac hypertrophy induced by pressure overload through the attenuation of autophagy, Int. J. Mol. Med., 2017, 39(1), 199–207 CrossRef CAS PubMed.
- A. Zhang, Z. Liu and L. Sheng, et al., Protective effects of
syringin against lipopolysaccharide-induced acute lung injury in mice, J. Surg. Res., 2017, 209, 252–257 CrossRef CAS.
- E. J. Yang, S. I. Kim and H. Y. Ku, et al., Syringin from stem bark of Fraxinus rhynchophylla protects Abeta(25-35)-induced toxicity in neuronal cells, Arch. Pharmacal Res., 2010, 33(4), 531–538 CrossRef CAS.
- X. Gong, L. Zhang and R. Jiang, et al., Hepatoprotective effects of syringin on fulminant hepatic failure induced by D-galactosamine and lipopolysaccharide in mice, J. Appl. Toxicol., 2014, 34(3), 265–271 CrossRef CAS.
- X. Wang, A. Zhang and G. Yan, et al., Metabolomics and proteomics annotate therapeutic properties of geniposide: targeting and regulating multiple perturbed pathways, PLoS One, 2013, 8(8), e71403 CrossRef CAS.
- E. B. Graham, A. R. Crump and D. W. Kennedy, et al., Multi 'omics comparison reveals metabolome biochemistry, not microbiome composition or gene expression, corresponds to elevated biogeochemical function in the hyporheic zone, Sci. Total Environ., 2018, 642, 742–753 CrossRef CAS PubMed.
- A. Zhang, H. Sun and P. Wang, et al., Metabonomics for discovering biomarkers of hepatotoxicity and nephrotoxicity, Die Pharmazie, 2012, 67(2), 99–105 CAS.
- H. Mizuno, K. Ueda and Y. Kobayashi, et al., The great importance of normalization of LC-MS data for highly-accurate non-targeted metabolomics, Biomed. Chromatogr., 2017, 31(1), e3864 CrossRef PubMed.
- X. Wang, H. Lv and A. Zhang, et al., Metabolite profiling and pathway analysis of acute hepatitis rats by UPLC-ESI MS combined with pattern recognition methods, Liver Int., 2014, 34(5), 759–770 CrossRef CAS PubMed.
- A. Ribbenstedt, H. Ziarrusta and J. P. Benskin, Development, characterization and comparisons of targeted and non-targeted metabolomicsmethods, PLoS One, 2018, 13(11), e0207082 CrossRef.
- A. Zhang, H. Sun and G. Yan, et al., Urinary metabolic profiling identifies a key role for glycocholic acid in human liver cancer by ultra-performance liquid-chromatography coupled with high-definition mass spectrometry, Clin. Chim. Acta, 2013, 418, 86–90 CrossRef CAS.
- X. Wang, Q. Wang and A. Zhang, et al., Metabolomics study of intervention effects of Wen-Xin-Formula using ultra high-performance liquid chromatography/mass spectrometry coupled with pattern recognition approach, J. Pharm. Biomed. Anal., 2013, 74, 22–30 CrossRef CAS PubMed.
- A. Zhang, H. Sun and X. Wang, Urinary metabolic profiling of rat models revealed protective function of scoparone against alcohol induced hepatotoxicity, Sci. Rep., 2014, 4, 6768 CrossRef PubMed.
- Y. Nan, X. Zhou and Q. Liu, et al., Serum metabolomics strategy for understanding pharmacological effects of ShenQi pill acting on kidney yang deficiency syndrome, J. Chromatogr. B: Anal. Technol. Biomed. Life Sci., 2016, 1026, 217–226 CrossRef CAS PubMed.
- X. N. Li, A. Zhang and M. Wang, et al., Screening the active compounds of Phellodendri Amurensis cortex for treating prostate cancer by high-throughput chinmedomics, Sci. Rep., 2017, 7, 46234 CrossRef CAS PubMed.
- A. Zhang, H. Sun and Y. Han, et al., Urinary metabolic biomarker and pathway study of hepatitis B virus infected patients based on UPLC-MS system, PLoS One, 2013, 8(5), e64381 CrossRef CAS PubMed.
- H. Sun, A. H. Zhang and Q. Song, et al., Functional metabolomics discover pentose and glucuronate interconversion pathways as promising targets for Yang Huang syndrome treatment with Yinchenhao Tang, RSC Adv., 2018, 8, 36831–36839 RSC.
- Y. Zhao, H. Lv and S. Qiu, et al., Plasma metabolic profiling and novel metabolite biomarkers for diagnosing prostate cancer, RSC Adv., 2017, 7(48), 30060–30069 RSC.
- H. Sun, A. Zhang and X. Wang, Potential role of metabolomic approaches for Chinese medicine syndromes and herbal medicine, Phytother. Res., 2012, 26(10), 1466–1471 CAS.
- A. Zhang, H. Sun and P. Wang, et al., Salivary proteomics in biomedical research, Clin. Chim. Acta, 2013, 415, 261–265 CrossRef CAS PubMed.
- H. L. Zhang, A. H. Zhang and X. H. Zhou, et al., High-throughput lipidomics reveal mirabilite regulating lipid metabolism as anticancer therapeutics, RSC Adv., 2018, 8(62), 35600–35610 RSC.
- H. Sun, A. Zhang and L. Yang, et al., High-throughput chinmedomics strategy for discovering the quality-markers and potential targets for Yinchenhao decoction, Phytomedicine, 2019, 54, 328–338 CrossRef CAS.
- H. Fang, A. H. Zhang and H. Sun, et al., High-throughput metabolomics screen coupled with multivariate statistical analysis identifies therapeutic targets in alcoholic liver disease rats using liquid chromatography-mass spectrometry, J. Chromatogr. B: Anal. Technol. Biomed. Life Sci., 2019, 1109, 112–120 CrossRef CAS PubMed.
- H. Sun, A. Zhang and G. Yan, et al., Metabolomic analysis of key regulatory metabolites in hepatitis C virus–infected tree shrews, Mol. Cell. Proteomics, 2013, 12(3), 710–719 CrossRef CAS PubMed.
- H. Xiong, A. H. Zhang and Q. Q. Zhao, et al., Discovery and screening quality-marker ingredients of Panax quinquefolius using chinmedomics approach, Phytomedicine, 2019, 152928 CrossRef.
- A. Zhang, H. Wang and H. Sun, et al., Metabolomics strategy reveals therapeutical assessment of limonin on nonbacterial prostatitis, Food Funct., 2015, 6(11), 3540–3549 RSC.
- Y. Nan, X. Zhou and Q. Liu, et al., Serum metabolomics strategy for understanding pharmacological effects of ShenQi pill acting on kidney yang deficiency syndrome, J. Chromatogr. B: Anal. Technol. Biomed. Life Sci., 2016, 1026, 217–226 CrossRef CAS PubMed.
- A. Zhang, H. Sun and X. Wang, Emerging role and recent applications of metabolomics biomarkers in obesity disease research, RSC Adv., 2017, 7(25), 14966–14973 RSC.
- J. Xie, A. H. Zhang and S. Qiu, Identification of the perturbed metabolic pathways associating with prostate cancer cells and anticancer affects of obacunone, J. Proteomics, 2019, 206, 103447 CrossRef CAS.
- A. Zhang, H. Sun and P. Wang, et al., Future perspectives of personalized medicine in traditional Chinese medicine: a systems biology approach, Complement. Ther. Med., 2012, 20(1–2), 93–99 CrossRef.
- A. Zhang, H. Sun and X. Wang, Potentiating therapeutic effects by enhancing synergism based on active constituents from traditional medicine, Phytother. Res., 2014, 28(4), 526–533 CrossRef PubMed.
- H. Sun, H. L. Zhang and A. H. Zhang, et al., Network pharmacology combined with functional metabolomics discover bile acid metabolism as a promising target for mirabilite against colorectal cancer, RSC Adv., 2018, 8, 30061–30070 RSC.
- Y. Zhang, P. Liu and Y. Li, et al., Exploration of metabolite signatures using high-throughput mass spectrometry coupled with multivariate data analysis, RSC Adv., 2017, 7, 6780–6787 RSC.
- Q. Liang, H. Liu and X. Li, et al., High-throughput metabolomics analysis discovers salivary biomarkers for predicting mild cognitive impairment and Alzheimer's disease, RSC Adv., 2016, 6, 75499–75504 RSC.
- L. Wang, H. Dong and A. H. Zhang, et al., Exploring the detoxification effects and mechanism of Caowu in prescription using liquid chromatography-high-resolution mass spectrometry-based metabolomics, Open Journal of Proteomics and Genomics, 2018, 3(1), 011–023 Search PubMed.
- A. H. Zhang, H. Sun and G. L. Yan, et al., Chinmedomics: A Powerful Approach Integrating Metabolomics with Serum Pharmacochemistry to Evaluate the Efficacy of Traditional Chinese Medicine, Engineering, 2019, 5, 60–68 CrossRef CAS.
- H. Sun, A. H. Zhang and S. B. Liu, et al., Cell metabolomics identify regulatory pathways and targets of magnoline against prostate cancer, J. Chromatogr. B: Anal. Technol. Biomed. Life Sci., 2018, 1102–1103, 143–151 CrossRef CAS PubMed.
- C. C. Feng, A. H. Zhang and J. H. Miao, et al., Recent advances in understanding cross-talk between Bile Acids and Gut Microbiota, Open Journal of Proteomics and Genomics, 2018, 3(1), 024–034 Search PubMed.
- X. J. Wang, J. L. Ren and A. H. Zhang, et al., Novel applications of mass spectrometry-based metabolomics in herbal medicines and its active ingredients: Current evidence, Mass Spectrom. Rev., 2019, 38(4–5), 380–402 CrossRef CAS.
- H. Cao, A. Zhang and H. Zhang, et al., The application of metabolomics in traditional Chinese medicine opens up a dialogue between Chinese and Western medicine, Phytother. Res., 2015, 29(2), 159–166 CrossRef PubMed.
- H. Zhang, A. Zhang and J. Miao, et al., Targeting regulation of tryptophan metabolism for colorectal cancer therapy: a systematic review, RSC Adv., 2019, 9, 3072–3080 RSC.
- A. Zhang, H. Sun and S. Qiu, et al., NMR-based metabolomics coupled with pattern recognition methods in biomarker discovery and disease diagnosis, Magn. Reson. Chem., 2013, 51(9), 549–556 CrossRef CAS.
- X. Liu, A. Zhang and H. Fang, et al., Serum metabolomics strategy for understanding the therapeutic effects of Yin-Chen-Hao-Tang against Yanghuang syndrome, RSC Adv., 2018, 8, 7403–7413 RSC.
- H. Fang, A. Zhang and J. Yu, et al., Insight into the metabolic mechanism of scoparone on biomarkers for inhibiting Yanghuang syndrome, Sci. Rep., 2016, 6, 37519 CrossRef CAS PubMed.
- A. Zhang, H. Sun and G. Yan, et al., Mass spectrometry-based metabolomics: applications to biomarker and metabolic pathway research, Biomed. Chromatogr., 2016, 30(1), 7–12 CrossRef PubMed.
- A. Zhang, H. Sun and Y. Han, et al., Exploratory urinary metabolic biomarkers and pathways using UPLC-Q-TOF-HDMS coupled with pattern recognition approach, Analyst, 2012, 137(18), 4200–4208 RSC.
- X. Li, A. Zhang and H. Sun, et al., Metabolic characterization and pathway analysis of berberine protects against prostate cancer, Oncotarget, 2017, 8, 65022–65041 Search PubMed.
- A. Zhang, H. Sun and G. Yan, et al., Metabolomics in diagnosis and biomarker discovery of colorectal cancer, Cancer Lett., 2014, 345(1), 17–20 CrossRef CAS PubMed.
- H. Chu, A. Zhang and Y. Han, et al., Metabolomics approach to explore the effects of Kai-Xin-San on Alzheimer's disease using UPLC/ESI-Q-TOF mass spectrometry, J. Chromatogr. B: Anal. Technol. Biomed. Life Sci., 2016, 1015, 50–61 CrossRef PubMed.
- H. Sun, X. Li and A. Zhang, et al., Exploring potential biomarkers of coronary heart disease treated by Jing Zhi Guan Xin Pian using high-throughput metabolomics, RSC Adv., 2019, 9(20), 11420–11432 RSC.
- A. Zhang, H. Sun and G. Yan, et al., Serum proteomics in biomedical research: a systematic review, Appl. Biochem. Biotechnol., 2013, 170(4), 774–786 CrossRef CAS PubMed.
- H. Sun, H. Wang and A. Zhang, et al., Berberine ameliorates nonbacterial prostatitis via multi-target metabolic network regulation, OMICS A J. Integr. Biol., 2015, 19(3), 186–195 CrossRef CAS PubMed.
- X. Li, Y. Han and A. Zhang, et al., Mechanistic and Therapeutic Advances in Colon Cancer: A Systematic Review, Open Journal of Proteomics and Genomics, 2019, 4(1), 001–012 CrossRef.
- G. Yan, A. Zhang and H. Sun, et al., An effective method for determining the ingredients of S huanghuanglian formula in blood samples using high-resolution LC-MS coupled with background subtraction and a multiple data processing approach, J. Sep. Sci., 2013, 36(19), 3191–3199 CAS.
- A. Zhang, H. Sun and G. Yan, et al., Metabolomics for biomarker discovery: moving to the clinic, BioMed Res. Int., 2015, 2015, 354671 Search PubMed.
- A. Zhang, H. Sun and S. Qiu, et al., Metabolomics in noninvasive breast cancer, Clin. Chim. Acta, 2013, 424, 3–7 CrossRef CAS PubMed.
- H. Sun, M. Wang and A. Zhang, et al., UPLC-Q-TOF-HDMS Analysis of Constituents in the Root of Two Kinds of Aconitum Using a Metabolomics Approach, Phytochem. Anal., 2013, 24(3), 263–276 CrossRef CAS.
- A. Zhang, H. Sun and X. Wang, Potentiating therapeutic effects by enhancing synergism based on active constituents from traditional medicine, Phytother. Res., 2014, 28(4), 526–533 CrossRef PubMed.
- Y. Zhao, H. Lv and S. Qiu, et al., Plasma metabolic profiling and novel metabolite biomarkers for diagnosing prostate cancer, RSC Adv., 2017, 7(48), 30060–30069 RSC.
- A. Zhang, S. Qiu and H. Xu, et al., Metabolomics in diabetes, Clin. Chim. Acta, 2014, 429, 106–110 CrossRef CAS PubMed.
- A. Zhang, G. Yan and H. Sun, et al., Deciphering the biological effects of acupuncture treatment modulating multiple metabolism pathways, Sci. Rep., 2016, 6, 19942 CrossRef CAS PubMed.
- X. Wang, H. Sun and A. Zhang, et al., Ultra-performance liquid chromatography coupled to mass spectrometry as a sensitive and powerful technology for metabolomic studies, J. Sep. Sci., 2011, 34(24), 3451–3459 CrossRef CAS PubMed.
- X. Wang, H. Sun and A. Zhang, et al., Potential role of metabolomics apporoaches in the area of traditional Chinese medicine: as pillars of the bridge between Chinese and Western medicine, J. Pharm. Biomed. Anal., 2011, 55(5), 859–868 CrossRef CAS PubMed.
- A. Zhang, H. Sun and Z. Wang, et al., Metabolomics: towards understanding traditional Chinese medicine, Planta Med., 2010, 76(17), 2026–2035 CrossRef CAS PubMed.
- L. Yuan, T. Wang and Y. Zhang, et al., An HBV-tolerant immunocompetent model that effectively simulates chronic hepatitis B virusinfection in mice, Exp. Anim., 2016, 65(4), 373–382 CrossRef CAS.
- L. Ye, H. Yu and C. Li, et al., Adeno-Associated Virus Vector Mediated Delivery of the HBV Genome Induces Chronic Hepatitis B Virus Infection and Liver Fibrosis in Mice, PLoS One, 2015, 10(6), e0130052 CrossRef.
- S. F. Wieland, The chimpanzee model for hepatitis B virus infection, Cold Spring Harbor Perspect. Med., 2015, 5(6), a021469 CrossRef PubMed.
- M. Roggendorf, A. D. Kosinska and J. Liu, et al., The Woodchuck, a Nonprimate Model for Immunopathogenesis and Therapeutic Immunomodulation in Chronic Hepatitis B Virus Infection, Cold Spring Harbor Perspect. Med., 2015, 5(12), a021451 CrossRef PubMed.
- A. Funk, M. Mhamdi and H. Will, et al., Avian hepatitis B viruses: molecular and cellular biology, phylogenesis, and host tropism, World J. Gastroenterol., 2007, 13(1), 91–103 CrossRef CAS PubMed.
- Y. Chang, H. Li and H. Ren, et al., Misclassification of chronic hepatitis B natural history phase: Insight from new ALT, AST, AKP, and GGT reference intervals in Chinese children, Clin. Chim. Acta, 2019, 489, 61–67 CrossRef CAS PubMed.
- X. Z. Wu, D. Chen and L. S. Zhao, et al., Early diagnosis of bacterial and fungal infection in chronic cholestatic hepatitis B, World J. Gastroenterol., 2004, 10(15), 2228–2231 CrossRef PubMed.
- Y. M. Wu, X. F. Xu and W. L. Wu, et al., Correlation between levels of liver fibrosis and liver fibrosis biochemical parameters of advanced schistosomiasis patients, Zhongguo Xuexichongbing Fangzhi Zazhi, 2014, 26(1), 65–67 CAS.
- S. de Souza-Cruz, M. B. Victória and A. M. Tarragô, et al., Liver and blood cytokine microenvironment in HCV patients is associated to liver fibrosis score: a proinflammatory cytokine ensemble orchestrated by TNF and tuned by IL-10, BMC Microbiol., 2016, 16, 3 CrossRef PubMed.
- D. W. Zeng, Y. R. Liu and J. Dong, et al., Serum HBsAg and HBeAg levels are associated with liver pathological stages in the immune clearance phase of hepatitis B virus chronic infection, Mol. Med. Rep., 2015, 11(5), 3465–3472 CrossRef CAS.
- C. Y. Chen, C. Crowther and M. C. Kew, et al., A valine to phenylalanine mutation in the precore region of hepatitis B virus causes intracellular retention and impaired secretion of HBe-antigen, Hepatol. Res., 2008, 38(6), 580–592 CrossRef PubMed.
- C. Shan, F. Xu and S. Zhang, et al., Hepatitis B virus X protein promotes liver cell proliferation via a positive cascade loop involving arachidonic acid metabolism and p-ERK1/2, Cell Res., 2010, 20(5), 563–575 CrossRef CAS PubMed.
- R. Desai, U. Patel and S. Sharma, et al., Association Between Hepatitis B Infection and Pancreatic Cancer: A Population-Based Analysis in the United States, Pancreas, 2018, 47(7), 849–855 CrossRef PubMed.
- X. J. Wang, W. Hu and T. Y. Zhang, et al., Irbesartan, an FDA approved drug for hypertension and diabetic nephropathy, is a potent inhibitor for hepatitis B virus entry by disturbing Na(+)-dependent taurocholate cotransporting polypeptide activity, Antiviral Res., 2015, 120, 140–146 CrossRef CAS PubMed.
- X. Lin, Z. M. Ma and X. Yao, et al., Substitution of proline 306 in the reverse transcriptase domain of hepatitis B virus regulates replication, J. Gen. Virol., 2005, 86(1), 85–90 CrossRef CAS PubMed.
- B. Böttcher and M. Nassal, Structure of Mutant Hepatitis B Core Protein Capsids with Premature Secretion Phenotype, J. Mol. Biol., 2018, 430(24), 4941–4954 CrossRef PubMed.
- A. Zhang, H. Sun and X. Wang, Urinary metabolic profiling of rat models revealed protective function of scoparone against alcohol induced hepatotoxicity, Sci. Rep., 2014, 4, 6768 CrossRef PubMed.
- A. Zhang, Z. Ma and H. Sun, et al., High-Throughput Metabolomics Evaluate the Efficacy of Total Lignans From Acanthophanax Senticosus Stem Against Ovariectomized Osteoporosis Rat, Front. Pharmacol., 2019, 10, 553 CrossRef PubMed.
- A. Zhang, H. Sun and G. Yan, et al., Mass spectrometry-based metabolomics: applications to biomarker and metabolic pathway research, Biomed. Chromatogr., 2016, 30(1), 7–12 CrossRef PubMed.
- X. Wang, X. Gao and A. Zhang, et al., High-throughput metabolomics for evaluating the efficacy and discovering the metabolic mechanism of Luozhen capsules from the excessive liver-fire syndrome of hypertension, RSC Adv., 2019, 9(55), 32141–32153 RSC.
- A. Zhang, H. Sun and P. Wang, et al., Modern analytical techniques in metabolomics analysis, Analyst, 2012, 137(2), 293–300 RSC.
- A. H. Zhang, J. B. Yu and H. Sun, et al., Identifying quality-markers from Shengmai San protects against transgenic mouse model of Alzheimer's disease using chinmedomics approach, Phytomedicine, 2018, 45, 84–92 CrossRef CAS PubMed.
- A. Zhang, H. Sun and G. Yan, et al., Metabolomics study of type 2 diabetes using ultra-performance LC-ESI/quadrupole-TOF high-definition MS coupled with pattern recognition methods, J. Physiol. Biochem., 2014, 70(1), 117–128 CrossRef CAS PubMed.
- A. Zhang, H. Sun and S. Qiu, et al., Metabolomics insights into pathophysiological mechanisms of nephrology, Int. Urol. Nephrol., 2014, 46(5), 1025–1030 CrossRef CAS PubMed.
- A. Zhang, H. Sun and G. Yan, et al., Metabolomics in diagnosis and biomarker discovery of colorectal cancer, Cancer Lett., 2014, 345(1), 17–20 CrossRef CAS PubMed.
- H. Dong, A. Zhang and H. Sun, et al., Ingenuity pathways analysis of urine metabolomics phenotypes toxicity of Chuanwu in Wistar rats by UPLC-Q-TOF-HDMS coupled with pattern recognition methods, Mol. BioSyst., 2012, 8(4), 1206–1221 RSC.
- A. Zhang, H. Sun and H. Xu, et al., Cell metabolomics, OMICS A J. Integr. Biol., 2013, 17(10), 495–501 CrossRef CAS PubMed.
- X. Wang, A. Zhang and X. Zhou, et al., An integrated chinmedomics strategy for discovery of effective constituents from traditional herbal medicine, Sci. Rep., 2016, 6, 18997 CrossRef CAS PubMed.
- Y. F. Li, S. Qiu and L. J. Gao, et al., Metabolomic estimation of the diagnosis of hepatocellular carcinoma based on ultrahigh performance liquid chromatography coupled with time-of-flight mass spectrometry, RSC Adv., 2018, 8(17), 9375–9382 RSC.
- X. Wang, J. Li and A. H. Zhang, Urine metabolic phenotypes analysis of extrahepatic cholangiocarcinoma disease using ultra-high performance liquid chromatography-mass spectrometry, RSC Adv., 2016, 6(67), 63049–63057 RSC.
- S. Qiu, A. Zhang and T. Zhang, et al., Dissect new mechanistic insights for geniposide efficacy on the hepatoprotection using multiomics approach, Oncotarget, 2017, 8(65), 108760–108770 Search PubMed.
- H. Wang, G. Yan and A. Zhang, et al., Rapid discovery and global characterization of chemical constituents and rats metabolites of Phellodendri amurensis cortex by ultra-performance liquid chromatography-electrospray ionization/quadrupole-time-of-flight mass spectrometry coupled with pattern recognition approach, Analyst, 2013, 138(11), 3303–3312 RSC.
Footnotes |
† Electronic supplementary information (ESI) available. See DOI: 10.1039/c9ra06332c |
‡ These authors contributed equally. |
|
This journal is © The Royal Society of Chemistry 2020 |
Click here to see how this site uses Cookies. View our privacy policy here.