DOI:
10.1039/D2RA01420C
(Paper)
RSC Adv., 2022,
12, 14167-14174
A new insight into the transfer and delivery of anti-SARS-CoV-2 drug Carmofur with the assistance of graphene oxide quantum dot as a highly efficient nanovector toward COVID-19 by molecular dynamics simulation†
Received
3rd March 2022
, Accepted 25th April 2022
First published on 11th May 2022
Abstract
Currently, a preventive and curative treatment for COVID-19 is an urgent global issue. According to the fact that nanomaterial-based drug delivery systems as risk-free approaches for successful therapeutic strategies may led to immunization against COVID-19 pandemic, the delivery of Carmofur as a potential drug for the SARS-CoV-2 treatment via graphene oxide quantum dots (GOQDs) was investigated in silico using molecular dynamics (MD) simulation. MD simulation showed that π–π stacking together with hydrogen bonding played vital roles in the stability of the Carmofur–GOQD complex. Spontaneous attraction of GOQDs loaded with Carmofur toward the binding pocket of the main protease (Mpro) resulted in the penetration of Carmofur into the active catalytic region. It was found that the presence of GOQD as an effective carrier in the loading and delivery of Carmofur inhibitor affected the structural conformation of Mpro. Higher RMSF values of the key residues of the active site indicated their greater displacement to adopt Carmofur. These results suggested that the binding pocket of Mpro is not stable during the interaction with the Carmofur–GOQD complex. This study provided insights into the potential application of graphene oxide quantum dots as an effective Carmofur drug delivery system for the treatment of COVID-19.
Introduction
The emergence of a new type of coronavirus, severe acute respiratory coronavirus (SARS-CoV-2),1–3 infects and threatens the lives of a large human population around the world. This new discovered type is the third documented highly pathogenic pneumonia coronavirus,4,5 which belongs to the Nidovirales order of the Coronaviridae family. SARS-CoV-2 is a positive-sense single stranded ribonucleic acid (RNA) virus that infects vertebrates. The main protease (Mpro) of SARS-CoV as a key enzyme in the life cycle of the coronaviruses6 is enrolled in the maturation cleavage events of translated polyproteins to convert the non-structural proteins to polypeptides required for virus replication.7–9 Accordingly, Mpro is a very pivotal element for the replication and transcription of coronaviruses and its inhabitation activity would block viral replication.10
Currently, a preventive and curative treatment for this urgent global issue has projected tremendous research interest. To date, numerous Food and Drug Administration (FDA) approved drugs are considered as potent inhibitors in targeting SARS-CoV-2.11–15
With the rapid development of nanotechnology, various nanomaterials with different composition and biological properties have been extensively studied as drug delivery systems. Drug delivery systems in nano-scaled demonstrated improved treatment efficiency and bioavailability by delivering the therapeutic agents with lower doses to the required sites. Drug delivery applications of polymeric, inorganic and carbon-based nanomaterials were approved by FDA.16–20 Recently, two dimensional (2D) materials such as graphene and graphene oxide due to the tunable physical and chemical properties have investigated in medicine application such as drug loading and transportation, theranostics,21 antimicrobial applications,22 biosensing23 and tissue engineering.24
Graphene oxide is one of the newest members of carbon nanostructures with random distribution of the oxygenated functional groups. It is known that graphene oxide have efficacy against particular bacteria, fungi, and viruses.25–30 In addition, the antimicrobial and antiviral efficiency of graphene and its derivatives are approved.31–33 Although, the toxicity of graphene oxide is its major limitation factor, the tunability of the size can cause these nanomaterials nontoxic and biocompatible.34 An important proposed mechanism underlying the nanotoxicity of graphene-based materials is generation of reactive oxygen species (ROS).35 Overproduction of ROS can induce oxidative stress, resulting in cell failing to maintain normal physiological redox-regulated functions. This in turn leads to DNA damage, unregulated cell signaling, change in cell motility, cytotoxicity, apoptosis and cancer initiation.36
Recently, graphene oxide quantum dots (GOQDs) due to low toxicity as well as their hydrophilicity37 have drawn the research attention in biological applications as drug delivery systems.38–40 GOQDs have a single atom layered structure with lateral dimension ranging from 2 to 20 nm. Li et al.41 assessed a systematic investigation of six graphene quantum dots (GQDs) with different surface modification, size, and redox form to compare the toxicity in cell and animal experiments. The results showed that graphene quantum dots, GQDs, functionalized with amine and carboxyl groups (GQD–NH2 and GQD–COOH) and GOQDs displayed less toxic than graphene oxide and reduced graphene oxide. This study showed that GOQDs could be safely used for drug delivery applications. Furthermore, it is known that graphene oxide in smaller size (i.e., GOQD) efficiently reduce oxidative stress, ROS, cell toxicity, apoptosis and inhibit neurotoxicity in vitro and in vivo through catalase-like activity and metabolic regulation.42
With the COVID-19 pandemic, some studies on the effectiveness of graphene-based materials in the detection and treatment of SARS-CoV-2 infections were applied.43–47 For example, the infection capability of SARS-CoV-2 can reduce by the application of antiviral properties of graphene and graphene oxide on the facial masks.48 Seo et al. introduced graphene sheets as highly sensitive biosensors for SARS-CoV-2 detection by coating anti-spike protein antibodies on their surface.49 A research by Yilmazer50 revealed that graphene oxide did not show any significant toxicity up to a concentration of 100 μg mL−1 in vitro during the investigation of this material against SARS-CoV-2 proteins. A research by Khedri et al. showed the efficiency of 2D structures to reduce the transmissibility and infectivity of SARS-CoV-2 by the deformation of the spike protein and inhibiting the Mpro.51
Among the vast range of therapeutic compounds examined, Carmofur drug appears to be a promising treatment for SARS-CoV-2 by a half-maximal effective concentration (EC50) value of 24.30 μM. In addition, cytotoxicity assays for Carmofur showed low toxicity in Vero E6 cells with a half-maximum cytotoxic concentration (CC50) value of >133 μM.52
The use of the computational methods helped researchers by enriching knowledge regarding the nature of the interaction of potential inhibitors and their targets in the safe environment. Therefore, we investigated the inhibitor activity of Carmofur drug and graphene oxide quantum dots using molecular docking and molecular dynamics (MD) simulation. Our computational results showed that Carmofur has hydrophobic interaction with the catalytic Cys145 of the enzyme. Also, the binding free energy analysis approved Carmofur has a strong complex with hydrophobic active site of Mpro from COVID-19 with the dominance of the van der Waals energy. The dramatic structural change of the main protease protein during interaction with GOQDs was also observed. These computational results are in good agreement with the experimental data.50,52 Jin et al.52 reported that the antineoplastic drug Carmofur inhibits viral replication in cells and is effective against SARS-CoV-2 main protease. A research by Yilmazer et al.50 experimentally demonstrated that graphene oxide could offer a platform to effectively interact and potentially transport other molecules to inactive SARS-CoV-2.
According to the fact that nanomaterial-based drug delivery systems as risk-free approaches for decreasing the side effects and enhancing the therapeutic efficiency of therapeutic molecules that may led to immunization against COVID-19 pandemic, graphene oxide quantum dot as a proper delivery system of Carmofur was investigated by MD simulation. Since in silico methods helped scientists to improve the quality of healthcare studies by providing high quality predictions, we tested our approach on the delivery process of Carmofur assisted by GOQD in the binding pocket of the Mpro enzyme using molecular dynamics simulation. Thus, the present study can be helpful to researchers for further in vitro experimental validation and development of graphene oxide quantum dots as a drug delivery vehicle by targeting COVID-19.
Computational details
System preparation
The initial 3D structure of COVID-19 main protease with a high resolution of 1.31 Å (PDB ID: 5R82) was extracted from RCBS Protein Data Bank (PDB) [https://www.rcsb.org/structure]. The native co-crystallized ligand present in the crystal structure of Mpro is 6-(ethylamino)pyridine-3-carbonitrile. After the removal of the native co-crystallized ligand, the structure of the Mpro enzyme was prepared and minimized using Dock preparation tool of UCSF-Chimera software.53 The 3D structures for Carmofur drug and native co-crystallized ligand were downloaded from PubChem database [https://pubchem.ncbi.nlm.nih.gov/]. The structural geometry optimization for these molecules was performed by density functional theory (DFT) calculations with B3LYP/6-31G(d, p) level of theory using Gaussian 16 software.54 The finite graphene oxide quantum dots were constructed with randomly decorated the graphene model in the dimension of 20 × 20 Å2 with hydroxyl and epoxide groups on the basal plane and carboxyl groups at the edges. Graphene oxide nanosheets consisted of 200 carbon atoms and 63 oxygen atoms. According to Hasselbalch–Henderson equation,55 the deprotonated/protonated sites of GOQD/Carmofur in physiological pH 7.4 were calculated. The structure of Carmofur, native ligand and GOQD are presented in Fig. 1.
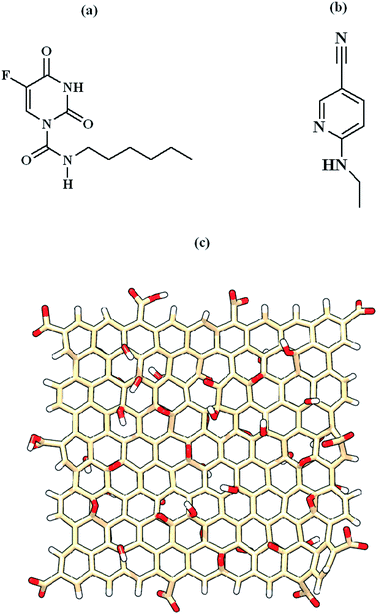 |
| Fig. 1 Chemical structures of (a) Carmofur drug, (b) native ligand and (c) graphene oxide quantum dots. | |
Molecular docking
The corresponding input files for molecular docking were prepared using AutoDockTools (ADT) V.1.5.7 suite.56 The polar hydrogen atoms were added to the amino acid residues and the partial atomic charges for Mpro and ligands were assigned using Kollman united atom57 and Gasteiger–Marsili58 methods, respectively. The native ligand and Carmofur drug were docked in the active binding site of Mpro enzyme by AutoDock Vina software59 with ligand binding pose coordinates X; 11.470, Y; −0.690, Z; 23.940. Docking calculations were carried out using the Lamarckian genetic algorithm (LGA) with a grid-point spacing of 0.375 Å. The structure of docked models was visualized using PyMol software.60
Molecular dynamics simulations
Six systems were considered for MD simulations: (a) Mpro enzyme; (b) Carmofur–Mpro; (c) native ligand–Mpro; (d) Carmofur–GOQD; (e) GOQD–Mpro and (f) Carmofur–GOQD complex with Mpro. It is noted that the best-scored poses of Carmofur–Mpro and native ligand–Mpro complexes obtained after molecular docking were used for MD simulations. The topology of Carmofur and native ligand were determined based on the CHARMM36 force field using CHARMM-GUI server.61 All unoxidized carbon atoms of graphene oxide were treated as uncharged Lennard-Jones (LJ) particles.62 The atomic partial charges of the functional groups and their anchoring carbons were taken from Tang et al.63,64 All simulation systems were immersed into a TIP3P cubic periodic box. The ionic concentration of the simulation systems was set to be 0.15 M sodium chloride (NaCl) according to the physiological ion concentration. The details of the simulation systems were presented in Table S1, ESI.† Molecular dynamics simulation was performed using Groningen machine for chemical simulations (GROMACS) software in force field CHARMM36 (ref. 65) with a time step of 2 fs.
Results and discussion
In order to ascertain the inhibitor activity of Carmofur drug as well as delivery process of Carmofur assisted by graphene oxide quantum dots in the binding pocket of Mpro enzyme, the computational methods such as molecular docking and molecular dynamics simulation were performed.
Docking results
To evaluate the inhibitor activity of Carmofur drug and native ligand of the Mpro enzyme, 6-(ethylamino)pyridine-3-carbonitrile, in the active site of target protein, the molecular docking study was performed. Fig. 2 and 3 show the 3D/2D schematics of the native ligand/Carmofur drug with Mpro protein in the active pocket of the enzyme. It was demonstrated that Carmofur drug has the highest docking score (−5.4 kcal mol−1) when compared with native ligand (−4.7 kcal mol−1). In the active site of Mpro, residues His41, Cys145 and Glu166 are as key species of the SARS-CoV-2 (ref. 10) and residues involved in the binding of ligand co-crystallized with Mpro include His41, Met49, Cys145, His164, Met165, Glu166, Arg188, Gln 189 and Thr190 (see Fig. 3).
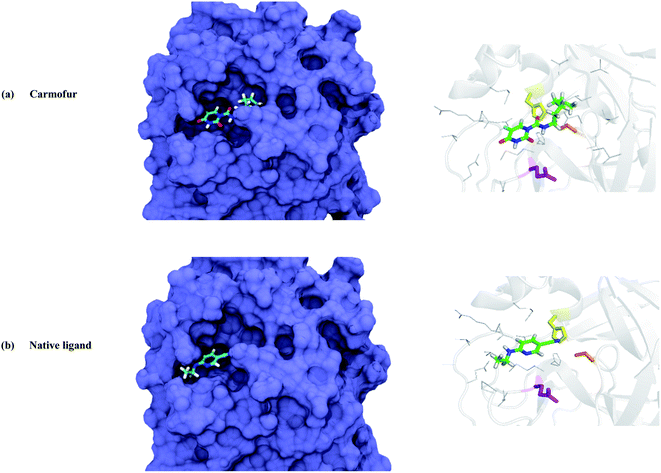 |
| Fig. 2 The surface (left) and 3D positioning (right) of the best docked complexes of (a) Carmofur and (b) native ligand within the binding pocket of COVID-19 main protease (color codes: His41: yellow, Cys145: orange and Glu166: magenta). | |
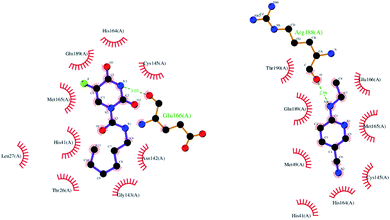 |
| Fig. 3 2D interaction of Carmofur (left) and native ligand (right) with COVID-19 Mpro protein. | |
From Fig. 3, Thr26, Leu27, His41, Asn142, Gly143, Cys145, His164, Met165 and Gln189 residues participated in the hydrophobic interaction with Carmofur drug. Besides, oxygen atom of the carbonyl group in Glu166 residue formed one hydrogen bond (H-bond length: 3.03 Å) with Carmofur drug. The interaction of Carmofur drug with catalytic dyad residues (e.g., His41, Cys145 and Glu166) played essential role for blocking the enzymatic activity of Mpro.
ADME and toxicity prediction
ADME/toxicity properties of Carmofur drug were computed using the SwissADME and pkCSM-pharmacokinetics servers. The predicted drug-likeness descriptors selected using Lipinski rules with SwissADME revealed good adsorption or permeation of Carmofur drug. Molecular weight, octanol–water partition coefficient (log
Po/w), number of hydrogen bond acceptors (HBAs), number of rotatable bonds (NBR) and polar surface area (PSA) of Carmofur were within the recognized values of less than 500, 5, 10, 10 and 140, respectively. The gastrointestinal (GI) absorption ability for Carmofur was high; this compound was unable to cross the blood–brain barrier (BBB). Furthermore, the Carmofur molecule was the substrate of P-glycoprotein (P-gp) and not inhibited the cytochrome P450 2D6 (CYP2D6) enzyme. The distribution of the Silicos-IT log
S value of soluble Carmofur was −3.34. Besides, the toxicity results of pkCSM-pharmacokinetics suggested that Carmofur is non-mutagenic and non-carcinogen as well as non-human ether-a-go-go related gene (hERG) blockers. It was found that Carmofur with log
VDss (volume of distribution at steady state) value of −0.24 is distributed in the plasma. Also, Carmofur showed no hepatotoxic and no skin sensitization. The pharmacokinetics and toxicity results approved the safety of the Carmofur molecule as a potential drug of SARS-CoV-2 treatment.
Analysis of the Carmofur–Mpro complex simulation
To determine the conformational change and the stability of protein target during the interaction with screened compounds, the root mean square deviation (RMSD) values of C-alpha (Cα) atoms were analyzed and the obtained results are presented in Fig. S1, ESI.† As can be seen from Fig. S1,† Carmofur compound bounded Mpro showed small fluctuation compared with native ligand. The average RMSD values of Mpro, Carmofur–Mpro, and native ligand–Mpro were found to be 0.193, 0.175 and 0.184 nm, respectively. Fig. S1† also revealed the stability of the considered systems during MD trajectories. In addition, the lowering of the solvent accessible surface area (SASA) value of Mpro protein (149.250 nm2) upon Carmofur (148.863 nm2) and native ligand (146.422 nm2) binding indicated the significant structure stability of Mpro enzyme after the interaction of the mentioned compounds to the active site of the target protein.
To characterize the overall structural change of Mpro enzyme, the root mean square fluctuations (RMSFs) for each amino acid residues of protein in the absence and presence of screened compounds are plotted in Fig. S1.† In general, the RMSF of the amino acid residues located in the N-terminal and C-terminal regions of the Mpro showed notable deviations owing to their high flexibility. As observed, lower RMSF values of the key residues in the active site region of Mpro interacting with Carmofur drug were indicative of higher stability in protein during the simulation.
To explore the binding stability of the Carmofur drug/native ligand and Mpro enzyme, the binding free energy analysis was performed through the molecular mechanics Poisson–Boltzmann surface area (MM/PBSA) approach66 and the obtained results are tabulated in Table S2, ESI.† In addition, the intermolecular hydrogen bonds (HBs) between the Carmofur/native ligand with SARS-CoV-2 Mpro were analyzed, as presented in Fig. S1.† Here, HBs are defined as those formed when the distance between donor and acceptor is 0.35 nm and the angle of donor-H-acceptor is less than 30.67
The MM/PBSA results suggested that Carmofur drug depicted a strong complex with Mpro enzyme with respect to the co-crystalize ligand, which could have the best inhibitory activity toward the inhibition site of Mpro. Also, the most favorable contribution values of van der Waals (EvdW) and electrostatic (Eelec) energies were observed for the Carmofur–Mpro complex. It is noted that the vdW interaction energy occupied the main driving force for the interaction of the Carmofur drug with hydrophobic active site of Mpro from COVID-19. The notable of the average hydrogen bonds between the Carmofur drug and Mpro confirmed its greater stability with respect to the native ligand during the simulation (see Fig. S1†).
The molecular docking and MD simulation results showed that the Carmofur drug stands out as a potential inhibitor of Mpro.
Carmofur adsorption on GOQD nanovehicle
Molecular dynamics simulation was performed to evaluate the capability of graphene oxide quantum dots to load the Carmofur drug in physiological media. The equilibrated configuration of the adsorbed drug on the nanosheet surface is shown in Fig. S2, ESI.† It was observed that the Carmofur drug was completely adsorbed on the nanosheet in such a way that its aromatic ring was close to the GOQD surface with almost parallel orientation. π–π stacking interaction as an attractive non-covalent interaction between π systems that contain aromatic moieties plays an important role in the stabilization of the formed complex. To validate the presence of π–π stacking interaction in the simulated system, we calculated the probability of finding the Carmofur drug from graphene oxide surface, which named radial distribution functions (RDF), as shown in Fig. S3, ESI.† From this figure, it was observed that the Carmofur drug molecule is positioned away from the nanosheet surface with the maximum distribution function at a distance of 0.370 nm. Location of the most intense peak in this region is well accordance with reported π–π distances in the considered conjugated complexes.68,69 Besides π–π stacking interaction, the stability of the formed complex was contributed to the formation of the hydrogen bonds between the adsorbed drug and oxygen-containing functional groups of GOQD, as shown in Fig. S3.†
The profile of the van der Waals and electrostatic interaction energy between Carmofur drug and GOQD versus simulation time was shown in Fig. S3.† Obviously, EvdW interaction energy was the main driving force in the adsorption of Carmofur on GOQD. It was found that graphene oxide quantum dot is a proper nanovehicle for delivery of Carmofur drug.
The interaction mechanism of GOQD/Carmofur–GOQD complex with Mpro enzyme
In this section, the mechanism of graphene oxide quantum dot-assisted delivery of Carmofur drug into the active site of main protease of SARS-CoV-2 was studied. Here, GOQD as a drug delivery system as well as an additional inhibitor facilitated binding of Carmofur drug within the active site of Mpro. For those, Carmofur–GOQD complex/GOQD and target protein were separated with the initial distance about 2.0 nm, measured from the binding pocket of Mpro and the basal plane of the nanosheets, as shown in Fig. 4 and S4, ESI.†
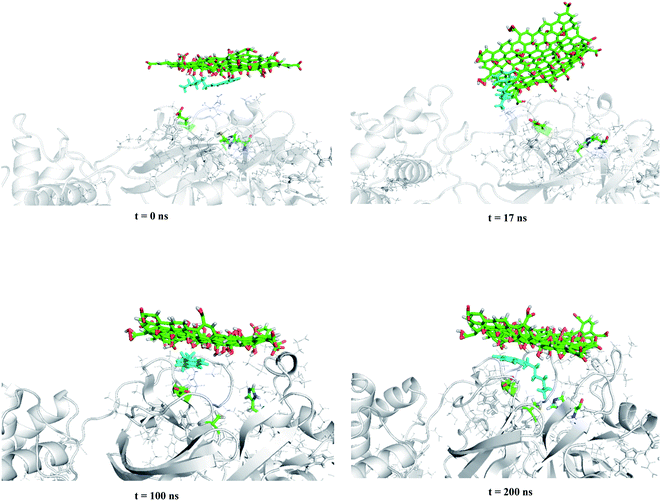 |
| Fig. 4 Trajectory snapshots ranging from 0 to 200 ns for the interaction of the Carmofur–GOQD complex with the binding pocket of the Mpro enzyme (water and ions molecules are not shown for clarity). | |
Within the simulation, it was observed that graphene oxide diffuses rapidly toward Mpro enzyme in such a way the edge of the nanosheet was pulled closer to the entrance of the binding pocket (i.e., t ∼ 17 ns). At this time, the interaction energy of GOQD with Mpro was rapidly decreased to −330 kJ mol−1, as shown in Fig. 5(a). Afterward, graphene oxide nanosheet fluctuated around the entrance of the binding pocket until the nanosheet was orientated almost parallel above the binding pocket. In this situation, Carmofur drug can penetrate into the active site of Mpro as there was a sudden dip in the interaction energy of Carmofur with Mpro enzyme (Fig. 5(b) at t = ∼50 ns). On the other hand, a sharp fall in the centre of mass (COM) distance between Carmofur drug and the residues of the catalytic site was also observed (see Fig. 5(c) at t = ∼50 ns). With deep penetration of Carmofur drug into the binding pocket, the gradually decreasing trend of the interaction energy between the drug and Mpro was found that was associated with a decrease in the average position of Carmofur with respect to the residues of the binding pocket (see Fig. 5, panels (b) and (c)).
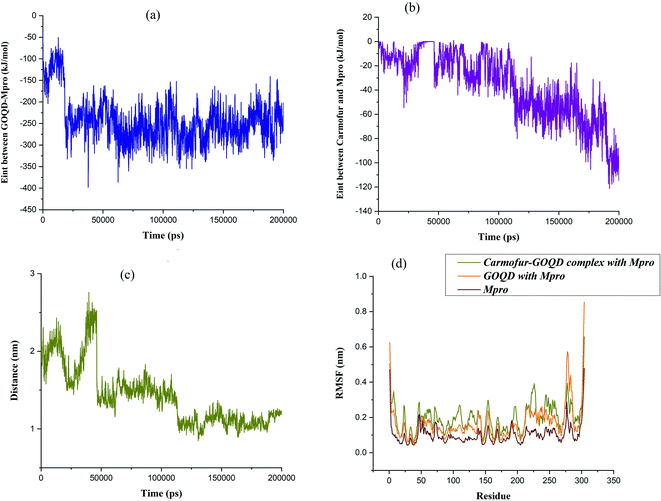 |
| Fig. 5 Time evaluation of (a) the interaction energy between GOQD and Mpro, (b) the interaction energy between Carmofur and Mpro, (c) the center of mass distance between the Carmofur drug molecule and the residues of the catalytic site and (d) RMSF for Cα atoms of main protease with the Carmofur–GOQD/GOQD complex. | |
It was found that Carmofur drug is at the closest interacting distance from Gly143 (1.22 nm), Met165 (1 nm), and Gln166 (1.37 nm) residues of Mpro in the equilibrated state. It is noted that the adsorbed drug still remained on the surface of the nanosheet and the position of the nanosheet did not significantly change until the end of the simulation (see Fig. 4). The conformational changes in the structure of the main protease protein during the interaction with Carmofur–GOQD complex/GOQD was analyzed using RMSD analysis. In addition, the protein stability after the possible interaction with Carmofur–GOQD complex/GOQD was examined by calculating the RMSF of Cα atoms and is shown in Fig. 5(d).
The average RMSD values of GOQD and Carmofur–GOQD complexes with Mpro were found to be 0.242 and 0.314 nm, respectively. As observed, the dramatic structural change of the main protease protein was found during the interaction with simulated complexes, especially with Carmofur–GOQD. In other words, the interaction of the Mpro enzyme with the Carmofur–GOQD complex significantly reduced its stability. Furthermore, the higher RMSF values of Fig. 5(d) confirmed greater flexibility and higher conformational changes of the residues interacting with Carmofur–GOQD complex/GOQD compared with the free form of Mpro enzyme. A close inspection of Fig. 5(d) reveals that the penetration of Carmofur drug with the assistance of GOQDs into the active site of Mpro resulted in a larger flexibility of the key residues. The flexibility of His41, Cys145 and Glu166 key residues significantly increased from 0.0585, 0.0493 and 0.0800 nm in free Mpro to 0.0895, 0.0824 and 0.1234 nm in the Carmofur–GOQD complex. This result suggested that the delivery of Carmofur drug by graphene oxide quantum dot nanovehicles into the active site of Mpro led to the instability and inactivation of the Mpro enzyme. Overall, MD simulation data show the potential usage of graphene oxide quantum dot as a Mpro inhibitor as well as an effective strategy for the delivery of Carmofur into the active site of the main protease to combat COVID-19.
At the equilibrated state, graphene oxide nanosheets interacted with hydrophobic amino acids Met49, Leu50, Pro168, Ala191 and hydrophilic residues Thr24, Ser46, Tyr118, Asn142, Gln189 and Thr190 (see Fig. S5, ESI†). In addition, graphene oxide formed multi-hydrogen bond interactions with the hydroxyl group of Thr190 residue of the protein. Also, multi hydrogen bonds were found between Carmofur drug and Tyr118 and Asn142 residues of Mpro, in which the hydroxyl group of Tyr118 aromatic main chain and Asn 142 amide side chain were involved. In addition, several hydrophobic interactions between Carmofur and Thr 26, Leu27, Leu141, Gly143 and Cys145 residues of Mpro were observed (see Fig. S5†).
Conclusions
In the present study, the mechanism of the loading and delivery of Carmofur drug using graphene oxide quantum dot against COVID-19 main protease as a therapeutic target was investigated using MD simulation. Also, the molecular docking and MD simulation approved more inhibitor activity of Carmofur drug in comparison with co-crystallized native ligand inhibitor of Mpro protein. The stability of the Carmofur–GOQD complex was attributed to π–π stacking interaction together with the hydrogen bonding between the adsorbed drug and oxygen-containing functional groups of the nanosheet. It was observed that graphene oxide quantum dots facilitate the Carmofur delivery in the vicinity of the catalytic region of the COVID-19 protein and further the penetration of drug loaded within the target site was observed.
Conflicts of interest
There are no conflicts to declare.
Notes and references
- R. Hussin, et al., J. Autoimmun., 2020, 109, 102433 CrossRef PubMed.
- N. Zhu, et al., N. Engl. J. Med., 2020, 382, 727–733 CrossRef CAS PubMed.
- C. Liu, et al., ACS Cent. Sci., 2020, 6, 315–331 CrossRef CAS PubMed.
- M. Hoffmann, H. Kleine-Weber, S. Schroeder, N. Kruger, T. Herrler, S. Erichsen, T. S. Schiergens, G. Herrler, N. H. Wu and A. Nitsche, et al., Cell, 2020, 181, 271–280 CrossRef CAS PubMed.
- M. Letko, A. Marzi and V. Munster, Nat. Microbiol., 2020, 5, 562–569 CrossRef CAS PubMed.
- W. Dai, B. Zhang, X. M. Jiang, H. Su, J. Li and Y. Zhao, et al., Science, 2020, 368(6497), 1331–1335 CrossRef CAS PubMed.
- K. Anand, EMBO J., 2002, 21, 3213–3224 CrossRef CAS PubMed.
- H. Yang, et al., Proc. Natl. Acad. Sci. U. S. A., 2003, 100, 13190–13195 CrossRef CAS PubMed.
- R. Hilgenfeld, FEBS J., 2014, 281, 4085–4096 CrossRef CAS PubMed.
- H. L. Nguyen, N. Q. Thai, D. T. Truong and M. S. Li, J. Phys. Chem. B, 2020, 124, 11337–11348 CrossRef CAS PubMed.
- H. L. T., N. Q. Nguyen, D. T. Truong and M. S. Li, J. Phys. Chem. B, 2020, 124, 11337–11348 CrossRef PubMed.
- K. El Bairi, D. Trapani, A. Petrillo and C. Le Page, et al., Eur. J. Cancer, 2020, 141, 40–61 CrossRef CAS PubMed.
- G. Ciliberto, R. Mancini and M. G. Paggi, J. Exp. Clin. Cancer Res., 2020, 39, 1–9 CrossRef PubMed.
- N. Borcherding, Y. Jethava and P. Vikas, Drug Des., Dev. Ther., 2020, 14, 5045 CrossRef CAS PubMed.
- L. Caly, J. D. Druce, M. G. Catton, D. A. Jans and K. M. Wagstaff, Antiviral Res., 2020, 178, 104787 CrossRef CAS PubMed.
- P. Esfandfar, M. Falahati and A. Saboury, J. Biomol. Struct. Dyn., 2016, 34, 1962–1968 CrossRef CAS PubMed.
- G. Hajsalimi, S. Taheri, F. Shahi, L. Pishkar, F. Attar, H. Ahmadi and M. Falahati, J. Biomol. Struct. Dyn., 2018, 36, 928–937 CrossRef CAS PubMed.
- V. Jafari Azad, S. Kasravi, H. Alizadeh Zeinabad, M. Memar Bashi Aval, A. A. Saboury and M. Falahati, J. Biomol. Struct. Dyn., 2017, 35, 2565–2577 CrossRef CAS PubMed.
- J. Mariam, S. Sivakami and P. M. Dongre, J. Biomol. Struct. Dyn., 2017, 35, 368–379 CrossRef CAS PubMed.
- S. Skariyachan, A. Parveen and S. Garka, J. Biomol. Struct. Dyn., 2017, 35, 3449–3468 CrossRef CAS PubMed.
- H. Shen, L. Zhang, M. Liu and Z. Zhang, Theranostics, 2012, 2, 283–294 CrossRef CAS PubMed.
- N. Fatima, U. Y. Qazi, A. Mansha, I. A. Bhatti, R. Javaid, Q. Abbas and M. Zahid, J. Ind. Eng. Chem., 2021, 100, 40–58 CrossRef CAS.
- M. Pumera, Mater. Today, 2011, 14, 308–315 CrossRef CAS.
- L. Shang, Y. Qi, H. Lu, H. Pei, Y. Li, L. Qu and W. Zhang, Graphene and graphene oxide for tissue
engineering and regeneration, in Theranostic Bionanomaterials, Elsevier, 2019, pp. 165–185 Search PubMed.
- J. He, X. Zhu, Z. Qi, C. Wang, X. Mao, C. Zhu, Z. He, M. Li and Z. Tang, ACS Appl. Mater. Interfaces, 2015, 7, 5605–5611 CrossRef CAS PubMed.
- J. Xie, Z. Ming, H. Li, H. Yang, B. Yu, R. Wu, X. Liu, Y. Bai and S. T. Yang, Chemosphere, 2016, 151, 324–331 CrossRef CAS PubMed.
- M. Sametband, I. Kalt, A. Gedanken and R. Sarid, ACS Appl. Mater. Interfaces, 2014, 6, 1228–1235 CrossRef CAS PubMed.
- S. Ye, K. Shao, Z. Li, N. Guo, Y. Zuo, Q. Li, Z. Lu, L. Chen, Q. He and H. Han, ACS Appl. Mater. Interfaces, 2015, 7, 21571–21579 CrossRef CAS PubMed.
- T. Du, J. Lu, L. Liu, N. Dong, L. Fang, S. Xiao and H. Han, ACS Appl. Bio Mater., 2018, 1, 1286–1293 CrossRef CAS PubMed.
- A. R. Deokar, A. P. Nagvenkar, I. Kalt, L. Shani, Y. Yeshurun, A. Gedanken and R. Sarid, Bioconjugate Chem., 2017, 28, 1115–1122 CrossRef CAS PubMed.
- O. Akhavan, M. Choobtashani and E. Ghaderi, J. Phys. Chem. C, 2012, 116, 9653–9659 CrossRef CAS.
- V. Palmieri and M. Papi, Nano Today, 2020, 33, 100883 CrossRef CAS PubMed.
- S. Bhattacharjee, R. Joshi, A. A. Chughtai and C. R. Macintyre, Adv. Mater. Interfaces, 2019, 6, 1900622 CrossRef CAS PubMed.
- k. k. Santhosh, M. D. Modak and P. Paik, J. Nanomed. Res., 2017, 5(6), 1–6 Search PubMed.
- P. P. Fu, Q. Xia, H. M. Hwang, P. C. Ray and H. Yu, J. Food Drug Anal., 2014, 22(1), 64–75 CrossRef CAS PubMed.
- L. Gonzalez, D. Lison and M. Kirsch-Volders, Nanotoxicology, 2008, 2, 252–273 CrossRef.
- Y. Shi, A. Pramanik, C. Tchounwou, F. Pedraza, R. A. Crouch, S. R. Chavva, A. Vangara, S. S. Sinha, S. Jones, D. Sardar and C. Hawker, ACS Appl. Mater. Interfaces, 2015, 7(20), 10935–10943 CrossRef CAS PubMed.
- Y. Xu, X. Hu, P. Guan, C. Du, Y. Tian, S. Ding, Z. Li and C. Yan, J. Mater. Sci., 2019, 54(12), 9124–9139 CrossRef CAS.
- S. Zeng, Y. Ji, Y. Shen, R. Zhu, X. Wang, L. Chen and J. Chen, RSC Adv., 2020, 10(15), 8744–8750 RSC.
- S. De, K. Patra, D. Ghosh, K. Dutta, A. Dey, G. Sarkar, J. Maiti, A. Basu, D. Rana and D. Chattopadhyay, ACS Biomater. Sci. Eng., 2018, 4(2), 514–531 CrossRef CAS PubMed.
- J. Li, X. Zhang, J. Jiang, Y. Wang, H. Jiang, J. Zhang and X. Nie, Toxicol. Sci., 2019, 167(1), 269–281 CrossRef CAS PubMed.
- C. Ren, X. Hu and Q. Zhou, Adv. Sci., 2018, 5(5), 1700595 CrossRef PubMed.
- A. K. Srivastava, N. Dwivedi, C. Dhand, R. Khan, N. Sathish and M. K. Gupta, et al., Mater. Today Chem., 2020, 18, 100385 CrossRef CAS PubMed.
- V. Palmieri and M. Papi, Nano Today, 2020, 33, 100883 CrossRef CAS PubMed.
- Z. Jiang, B. Feng, J. Xu, T. Qing, P. Zhang and Z. Qingb, Biosens. Bioelectron., 2020, 166, 112471 CrossRef CAS PubMed.
- M. A. B. F. Unal, H. Nazir, O. Besbinar, C. Gurcan, N. Lozano, L. Arellano, S. Yalcin, O. Panatli, D. Celik, D. Alkaya, A. Agan, L. Fusco, S. Suzuk Yildiz, L. G. Delogu, K. Akcali, K. Kostarelos and A. Yilmazer, Small, 2021, 17, 2101483 CrossRef CAS PubMed.
- P. Kumar Raghav and S. Mohanty, Med. Hypotheses, 2020, 144, 110031 CrossRef CAS PubMed.
- F. Maio De, V. Palmieri, G. Babini, A. Augello, I. Palucci and G. Perini, et al., iScience, 2020, 24(7), 102788 CrossRef PubMed.
- G. Seo, G. Lee, M. J. Kim, S. Baek, M. Choi and K. B. Ku, et al., ACS Nano, 2020, 14, 5135–5142 CrossRef CAS PubMed.
- M. A. Unal, F. Bayrakdar, H. Nazir, O. Besbinar, C. Gurcan, N. Lozano and A. Yilmazer, Small, 2021, 2101483 CrossRef CAS PubMed.
- M. Khedri, R. Maleki, M. Dahri, M. M. Sadeghi, S. Rezvantalab, H. A. Santos and M. A. Shahbazi, Drug Delivery Transl. Res., 2021, 12, 1408–1422 CrossRef PubMed.
- Z. Jin, Y. Zhao, Y. Sun, B. Zhang, H. Wang, Y. Wu and Z. Rao, Nat. Struct. Mol. Biol., 2020, 27, 529–532 CrossRef CAS PubMed.
- E. F. Pettersen, T. D. Goddard, C. C. Huang, G. S. Couch, D. M. Greenblatt, E. C. Meng and T. E. Ferrin, J. Comput. Chem., 2004, 25, 1605–1612 CrossRef CAS PubMed.
- M. J. Frisch, et al., Gaussian 16, Rev. C.01, Gaussian, Inc., Wallingford CT, 2016 Search PubMed.
- H. N. Po and N. M. Senozan, J. Chem. Educ., 2001, 78, 1499 CrossRef CAS.
- G. M. Morris, R. Huey, W. Lindstrom, M. F. Sanner, R. K. Belew, D. S. Goodsell and A. J. Olson, J. Comput. Chem., 2009, 30, 2785–2791 CrossRef CAS PubMed.
- S. J. Weiner, P. A. Kollman, D. T. Nguyen and D. A. Case, J. Comput. Chem., 1986, 7, 230–252 CrossRef CAS PubMed.
- J. Gasteiger and M. Marsili, Tetrahedron, 1980, 36, 3219–3228 CrossRef CAS.
- O. Trott and A. J. Olson, J. Comput. Chem., 2010, 31, 455–461 CAS.
- W. L. Delano, The PyMOL Molecular Graphics System, Version 1.8. Schrö dinger LLC, vol. 10, 2002 Search PubMed.
- S. Jo, T. Kim, V. G. Iyer and W. Im, J. Comput. Chem., 2008, 29, 1859–1865 CrossRef CAS PubMed.
- X. J. Gong, J. Y. Li, H. J. Lu, R. Z. Wan, J. C. Li, J. Hu and H. P. Fang, Nat. Nanotechnol., 2007, 2, 709–712 CrossRef CAS PubMed.
- H. Tang, D. Liu, Y. Zhao, X. Yang, J. Lu and F. Cui, J. Phys. Chem. C, 2015, 119, 26712–26718 CrossRef CAS.
- H. Tang, Y. Zhao, S. Shan, X. Yang, D. Liu, F. Cui and B. Xing, Environ. Sci. Technol., 2018, 52, 7689–7697 CrossRef CAS PubMed.
- J. Huang and A. D. MacKerell, J. Comput. Chem., 2013, 34, 2135–2145 CrossRef CAS PubMed.
- R. Kumari, R. Kumar and A. G. Lynn, J. Chem. Inf. Model., 2014, 54, 1951–1962 CrossRef CAS PubMed.
- D. Van der Spoel, E. Lindahl and B. Hess, et al., User Manual Version, 3, 2004, available from: https://www.gromacs.org Search PubMed.
- C. Janiak, J. Chem. Soc., Dalton Trans., 2000, 21, 3885–3896 RSC.
- H. Hashemzadeh and H. Raissi, J. Phys. D: Appl. Phys., 2018, 51(34), 345401 CrossRef.
|
This journal is © The Royal Society of Chemistry 2022 |
Click here to see how this site uses Cookies. View our privacy policy here.