DOI:
10.1039/D1SC05852E
(Edge Article)
Chem. Sci., 2022,
13, 3216-3226
Multiplex metal-detection based assay (MMDA) for COVID-19 diagnosis and identification of disease severity biomarkers†‡
Received
24th October 2021
, Accepted 14th February 2022
First published on 14th February 2022
Abstract
The ongoing COVID-19 pandemic caused by SARS-CoV-2 highlights the urgent need to develop sensitive methods for diagnosis and prognosis. To achieve this, multidimensional detection of SARS-CoV-2 related parameters including virus loads, immune response, and inflammation factors is crucial. Herein, by using metal-tagged antibodies as reporting probes, we developed a multiplex metal-detection based assay (MMDA) method as a general multiplex assay strategy for biofluids. This strategy provides extremely high multiplexing capability (theoretically over 100) compared with other reported biofluid assay methods. As a proof-of-concept, MMDA was used for serologic profiling of anti-SARS-CoV-2 antibodies. The MMDA exhibits significantly higher sensitivity and specificity than ELISA for the detection of anti-SARS-CoV-2 antibodies. By integrating the high dimensional data exploration/visualization tool (tSNE) and machine learning algorithms with in-depth analysis of multiplex data, we classified COVID-19 patients into different subgroups based on their distinct antibody landscape. We unbiasedly identified anti-SARS-CoV-2-nucleocapsid IgG and IgA as the most potently induced types of antibodies for COVID-19 diagnosis, and anti-SARS-CoV-2-spike IgA as a biomarker for disease severity stratification. MMDA represents a more accurate method for the diagnosis and disease severity stratification of the ongoing COVID-19 pandemic, as well as for biomarker discovery of other diseases.
Introduction
The ongoing coronavirus disease 2019 (COVID-19) pandemic caused by the novel severe acute respiratory syndrome coronavirus 2 (SARS-CoV-2) poses a huge threat to public health worldwide.1–3 Early diagnosis is essential for the control of this highly transmissible disease.4 Laboratory diagnostics for COVID-19 can be achieved through either direct detection of viral components (RNA or proteins)5,6 or indirect detection of anti-SARS-CoV-2 antibodies by serological assays.7,8 Although the methods for direct virus quantification are preferred for early diagnosis of COVID-19, assessment of serum anti-SARS-CoV-2 antibody levels (e.g. IgG, IgA and IgM) is still essential for retrospective diagnosis, immune response monitoring, contact tracing, seroprevalence studies, and possible determination of vaccination intervals.9–12 Most reported COVID-19 serological assays are monoplex assays that detect total immunoglobulin (Ig), IgM, and/or IgG against the SARS-CoV-2 spike (S) or nucleocapsid (N) protein. Simultaneous quantitation of different anti-SARS-CoV-2 Ig isotypes remains a major challenge.13,14
Inductively coupled plasma mass spectrometry (ICP-MS) is a robust platform for accurate quantification of elements, especially metals at ultratrace levels. Given the unique advantages of ICP-MS for metal detection, metal tags are often introduced in ICP-MS-based detection platforms for the quantification of biomolecules (nucleic acids and proteins).15–17 In recent years, with the advent of mass cytometry,18 ICP-MS-based single-cell analysis has emerged as a state-of-the-art technology with extremely high multiplexing capability (theoretically ≥100) that may potentially be applied in clinical laboratories for the diagnosis of various diseases.19
Compared with fluorophore tags, the most commonly used reporter molecules, metal (lanthanides) tags in mass cytometry are superior in terms of their sensitivity, dynamic range, signal overlap and background. The advantages of metal tags over fluorophores have been well addressed in previous studies which compared the performance of flow cytometry and mass cytometry,20 the two representative single cell techniques using fluorophores and metals as reporters, respectively.
Herein, by utilizing metal-tagged antibodies as reporting probes, we report a novel ICP-MS-based MMDA for COVID-19 diagnosis, which provides to date the highest multiplexed potentiality for quantification of the biofluid proteome. Furthermore, by integration with the high-dimensional data exploration/visualization tool (tSNE) with machine learning algorithms, the MMDA is applicable to different biofluid (plasma, serum, saliva, urine, etc.) multiplex assays for disease diagnosis, patient stratification and biomarker development. Using COVID-19 as a showcase, we validated the robustness of MMDA by serologic profiling of SARS-CoV-2-specific antibodies, including anti-SARS-CoV-2-spike (S) and -nucleocapsid (N) IgM, IgG, and IgA. We characterized the serum antibody landscape of COVID-19 patients and identified a unique correlation between their antibody profiles and clinical severity.
Results
Development of an ICP-MS-based method for diagnosis of COVID-19
As a proof-of-concept, we first established the multiplex metal-detection based assay (MMDA) and validated it for SARS-CoV-2-specific antibody detection by ICP-MS using metal-tagged antibodies as reporting probes. To capture and enrich the targeted antibodies in the biofluids, including SARS-CoV-2-specific IgM, IgG, and IgA, the magnetic beads were functionalized with SARS-CoV-2-S or -N proteins and the lanthanide tag (175Lu, 167Er, 149Sm) labeled anti-human secondary antibodies (anti-IgM, IgG, and IgA) were used to differentiate and quantify these antibodies. Each lanthanide tag was conjugated to specific secondary antibodies (175Lu-IgG, 167Er-IgM, 149Sm-IgA) through a reaction with thiol (–SH) groups in the side-chain of cysteine. The extremely high sensitivity and wide dynamic range of ICP-MS for lanthanide quantification and the low background of lanthanides in biological samples endow MMDA with unique advantages for biomolecule analysis in real specimens. After the biofluids were incubated with functionalized magnetic beads, the captured targets were magnetically separated and interacted with probe antibodies. The formed immune complexes on magnetic beads were then eluted to the supernatant under acidic conditions, and the released tagged metals were subsequently quantified by ICP-MS for the quantification of antibodies against S proteins or N proteins (Scheme 1).
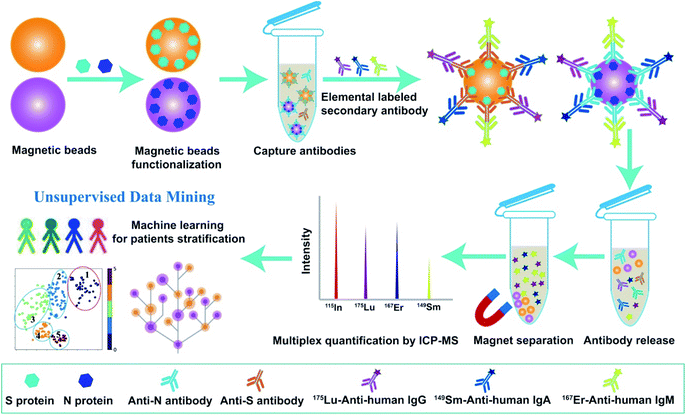 |
| Scheme 1 Schematic chart of the MMDA platform for multiplex anti-SARS-CoV-2 serological assay. | |
Feasibility of MMDA for multiplexed determination of COVID-19 antibodies
We examined the feasibility of MMDA for quantification of SARS-CoV-2 antibodies by comparing the intensity of the labeled metals (167Er and 175Lu) in the presence and absence of S protein specific IgM and IgG antibodies (1 mg L−1) (Fig. 1A). Evident increases in the signals (27- and 36-fold increase for IgG and IgM, respectively) were observed in the presence of target molecules, indicating the feasibility of MMDA for quantification of COVID-19 antibodies. We further evaluated the specificity of MMDA for quantification of different targets. As two isotypes of immunoglobulin, IgM and IgG show a high tendency to cross-react with each other, we examined the specificity of MMDA for IgM and IgG detection by comparing the signal intensities of IgM and IgG in the presence and absence of the same amount of IgG or IgM. As shown in Fig. 1B, the signal-to-background ratios of IgG and IgM were maintained at the same level before and after introducing IgM and IgG into the detection system, indicating the high specificity of MMDA for the quantification of different targets. More importantly, the metal signal intensity (167Er/175Lu) and concentrations of target molecules (S protein specific IgM and IgG antibodies) exhibit good linearity with correlation equations of y = 22.19x + 1375 (R2 = 0.9857) and y = 78.4x + 2030 (R2 = 0.9973) for the quantification of IgM and IgG antibodies, respectively, and the corresponding limits of detection (LOD, 3σ/k) were calculated to be 21.78 and 6.3 ppb (Fig. 1C).
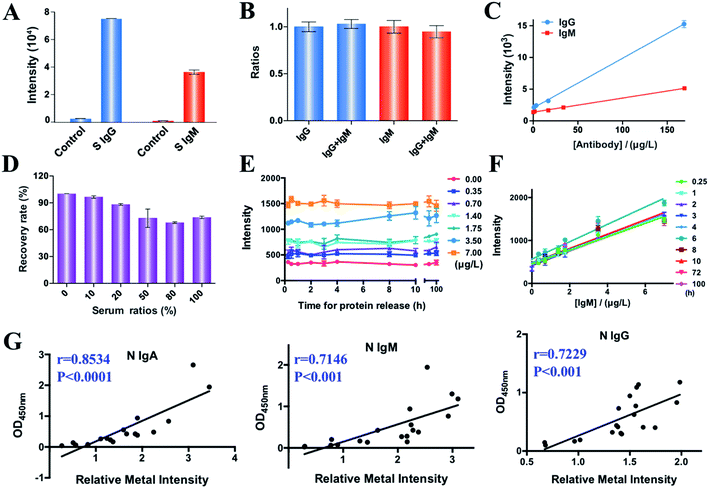 |
| Fig. 1 (A) Metal signal intensity in the presence and absence of IgG and IgM antibodies against the S protein. An evident increase in the metal signal intensity was observed after introducing the target molecules. (B) Comparison of normalized signal-to-background ratios between IgG or IgM detection in the presence and absence of the same amount of IgM or IgG (1 mg L−1), respectively. Minor interference was observed between different targets. (C) Linear relationship between concentrations of IgG/IgM antibody responses to the S protein and intensities of 175Lu and 167Er. A good correlation was obtained between the intensity of reporter metals and the concentration of the target molecules. (D) Comparison of the recovery rates for quantification of IgM in different ratios of serum. (E) Variation of the 167Er signal response to different concentrations of the IgM antibody (0–7 μg L−1) analyzed at different time points after adding the elution solution. Minor variation was shown among samples analyzed at different time points. (F) Linear relationship between concentrations of the IgM antibody and the intensity of 167Er analyzed at different time points. Consistent good linearity between the IgM concentration and 167Er intensity was obtained. (G) Correlation of the ELISA and MMDA results for antibody quantification. The intensities of N protein specific IgA, IgG and IgM from ELISA and MMDA are well correlated. Pearson correlation coefficients (r) are depicted in plots. P values were calculated by the two-sided t-test. | |
We also investigated the complex matrix effects on the detection results of MMDA in biological samples by monitoring the recovery rate (signal intensity of samples containing different concentrations of serum/sample without serum) by comparing the signal intensity in the presence of different concentrations of serum (0%, 10%, 20%, 50%, 80%, and 100%). As shown in Fig. 1D, the signal intensity was maintained at almost the same level in the presence of different serum concentrations, and the recovery rates for samples containing 50%, 80% and 100% serum were 73%, 68% and 74%, respectively, indicating the good tolerance of MMDA towards the complex biological matrix. Given that less than 10% serum is contained in most of the serum assay systems (1
:
10 dilution), the biological matrix effects could be neglected for MMDA.
Unlike fluorescent molecules, which suffer from low stability due to photobleaching, the metal tags are stable, which permits their reliable quantification even after extensive periods following the initial staining. Fig. 1E shows the metal intensity (167Er) of a series of samples collected at different time points (15 min to 100 h) after release from the targets (IgM antibody, 0–7 μg L−1). We only observed minor variations of metal signals with time for samples with different concentrations of targets. The consistent good linearity between the IgM concentration and 167Er intensity further indicates the rapid release (as short as 15 min) of the captured target molecules into the elution solution (1% HNO3) and the long-term stability of reporter molecules (Fig. 1F). Taken together, we demonstrate the feasibility of MMDA for multiplex quantification of SARS-CoV-2 antibodies and the long-term sample stability of MMDA, which allows retrospective analysis without the requirement for further staining and additional preservation steps and direct quantitative comparison between patient samples collected at different stages.
Higher accuracy of MMDA for the quantification of COVID-19 antibodies than ELISA
We next comprehensively profiled specific anti-SARS-CoV-2-S and -N antibodies (IgM, IgG, IgA) in 217 serum samples, including 107 serum samples from COVID-19 patients and 110 serum samples from non-SARS-CoV-2-infected controls. The clinical characteristics, including gender, age, sample collection date, and infection status are summarized in Table S1.‡
To validate the accuracy of multiplex antibody profiling results by MMDA, the intensities of N specific IgA, IgM and IgG of 19 COVID-19 patients were compared with those by enzyme-linked immunosorbent assay (ELISA),21 the clinically used method for COVID-19 antibody quantification. The results show a high correlation between ELISA and MMDA with Pearson correlation coefficients of 0.85, 0.71, and 0.72 for IgA, IgM and IgG, respectively (Fig. 1G), indicating the reliability of MMDA for COVID-19 antibody profiling. Furthermore, to compare the sensitivity of MMDA and ELISA for COVID-19 diagnosis, 7 mild COVID-19 patients with lower antibody levels among 107 patients and 5 non-SARS-CoV-2-infected controls with higher antibody background signals among 110 healthy controls were selected given their higher potential to cause false positive and false negative results, and the anti-SARS-CoV-2-S and -N IgM, IgG, and IgA were quantified by both MMDA and ELISA. For the antibody profiling of these 12 samples, the ELISA assay exhibited high false-positive rates (ratios of non-infected samples with antibodies higher than the 95% percentile for non-infected serum samples) for the detection of antibodies against the SARS-CoV-2-S protein (28.6% IgM, 71.4% IgG, 100% IgA) and also high false-negative rates (ratios of patients with antibodies lower than the 95% percentile for non-infected serum samples) based on the levels of SARS-CoV-2-S antibodies (40% IgM, 80% IgG, and 60% IgA) (Table S2‡). In contrast, MMDA had significantly lower false-negative rates (p = 0.0172), with no false positive and negative results for anti-SARS-CoV-2-S and -N IgG. Taken together, we demonstrate the higher sensitivity and specificity of MMDA than ELISA for COVID-19 antibody profiling.
Diagnosis of COVID-19 and host anti-SARS-CoV-2 antibody response profiling by MMDA
We further compared the antibody levels in 107 COVID-19 patients with the control serum samples (110 healthy controls), and the serum samples of COVID-19 patients showed significant elevation of all types of antibodies, including IgM, IgG, and IgA against SARS-CoV-2-S and -N proteins (Fig. 2A). By using the cut-off thresholds as reported previously22 (95% percentile for 110 non-infected serum samples corresponding to 95% specificity), the percentages of positive seroconversion of COVID-19 patients were 59.8%/59.8%, 91.6%/94.4%, and 85.0%/88.8%, for IgM, IgG, and IgA to SARS-CoV-2-S and -N proteins, respectively. The varied positive rates and relatively low IgM positivity may be caused by the different collection times of the samples and the high ratios of mild or asymptomatic infection among the COVID-19 patients in the study.
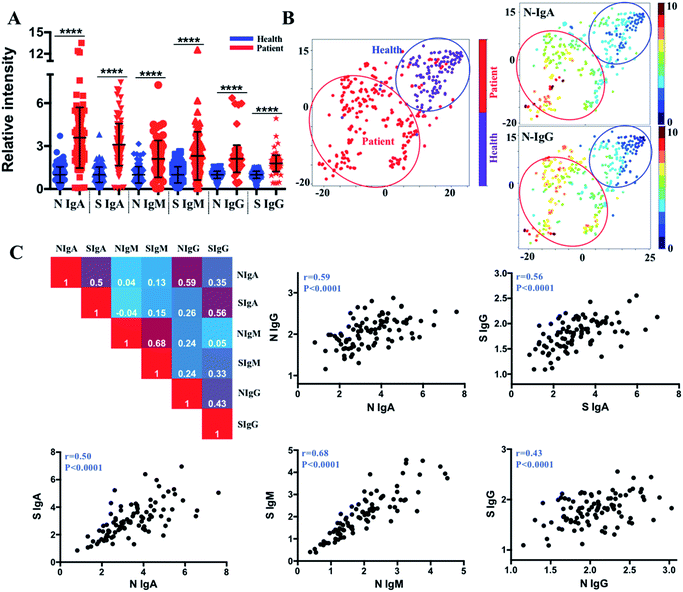 |
| Fig. 2 Multiplexed serological profiling of IgA, IgM and IgG responses to SARS-CoV-2 S and N proteins. (A) Comparison of antibody intensity between COVID-19 patients and non-infected individuals. Antibody signals are normalized to the median value of the corresponding antibody levels of 110 healthy controls. Significant increases in IgA, IgG and IgM antibodies were found in serum samples of COVID-19 patients compared with non-infected subjects. Solid bars denote the median value of each antibody across all samples used in the plot. (B) tSNE map generated by the intensity of different types of antibodies for COVID-19 diagnosis (the red and blue circles represent the gates for the discrimination of COVID-19 patients and healthy controls). The x- and y-axes of each tSNE figure represent t-SNE dimension 1 and dimension 2, respectively. The distribution of N protein specific IgA and IgG shows the highest correlation with the distribution of COVID-19 patients and healthy controls in the tSNE map. (C) Correlation metrics among antibodies against different antigens and different antibody isotypes against the same antigen. IgA, IgG and IgM against S and N antigens and S and N protein specific IgA and IgG antibodies are well correlated. Pearson correlation coefficients (r) are depicted in plots. P values were calculated by the two-sided t-test. | |
We then aimed to exploit these data to develop a stratification strategy for COVID-19 patients into different subgroups based on their antibody profiles by incorporating tSNE,23 an efficient high-dimensional data visualization approach that is widely applied in single-cell studies. With tSNE, each subject is shown as a single point in high-dimensional space, within which the location reflects the information of different antibody intensities. The t-SNE map was generated based on six antibody variables, i.e., IgA, IgM and IgG antibodies against S and N proteins, by an open-source machine learning library scikit-learn in Python 3.6. Antibody intensities were transformed using the sigmoid function. The iterations of 1000, perplexity parameter of 30 and trade-off θ of 0.5 were used to project the high-dimensional data into low-dimensional space for visualization. The tSNE map in two dimensions is formed using an optimization algorithm to determine the pairwise distances between each point based on the similarity of the antibody pattern. Each subject can be visualized by incorporating colour as a third dimension in the tSNE map through changing different channels (IgA, IgM, IgG, infection status). As shown in Fig. 2B, tSNE automatically arranged COVID-19 patients (red dots) in one region (red circled area) that is distinct from the non-infected controls (purple dots, blue circled area) with approximately 95.3% sensitivity (102 of 107 COVID-19 patients fall into the red circled area) and 94.5% specificity (104 of 110 non-infected controls fall into the blue circled area). By incorporating the status of infection with different antibody types (Fig. S1‡), the most efficient parameters for COVID-19 diagnosis were found to be IgG and IgA responses to the SARS-CoV-2-N protein, considering the relatively higher positive rate in the patient group, the higher negative rate in the non-infected group, and the higher increased median value between the patients and non-infected group. This finding provides the basis for the selection of antibody types in future serological assays.
As multiple functions or features of antibodies may simultaneously contribute to controlling the infection, correlation matrices were constructed to examine the relationships between different antibody isotypes (IgM, IgG and IgA) and the same antibody isotype responding to different antigens (N and S proteins). By plotting the antibody values against each other, an overall high correlation between anti-SARS-CoV-2-S and -N antibodies was observed, with Pearson correlation coefficients of 0.50, 0.68, and 0.43 for IgA, IgM and IgG, respectively (Fig. 2C). Importantly, the IgA and IgG responses to both antigens were found to be positively correlated, indicating the potential interaction between IgA and IgG during infection.
To further evaluate the antibody profile characteristics in different population subsets, the COVID-19 patients were categorized into different subgroups based on gender, age, sampling time, and symptomatic status, and their serum antibody profiles were compared (Fig. 3). Interestingly, we found a generally higher level of anti-SARS-CoV-2-N IgM in females (n = 48) than in males (n = 46) (p = 0.0509), while no difference in the anti-SARS-CoV-2-S and -N IgG levels was observed (Fig. 3A). To investigate whether the antibody profile differs with age, the COVID-19 patients were split into three groups: 0–18 (n = 4), 18–60 (n = 60), and >60 (n = 30) years. As shown in Fig. 3B, higher anti-SARS-CoV-2-S and -N IgA levels were found in older patients. Although not statistically significant, patients older than 60 years also exhibited higher anti-SARS-CoV-2-N IgG levels, while the anti-SARS-CoV-2-S IgG levels showed no observable difference among these three groups. To evaluate whether the antibody response varied with different sampling times, the antibody levels were compared between patients whose serum samples were collected within two weeks after symptom onsets (0–14) (n = 49) and those whose serum samples were collected after two weeks (14–37) (n = 12). The results showed an overall decreasing trend in terms of the median values of all the antibodies, although the reduction was not significantly different among patients with a sampling time of less than 37 days (Fig. 3C). To investigate whether a specific antibody response was shown in asymptomatic patients (n = 31), their antibody levels were compared with those of symptomatic patients (n = 45). No observable difference was found in the levels of any of the antibody isotypes to SARS-CoV-2-S and -N antigens between asymptomatic and symptomatic patients (Fig. 3D).
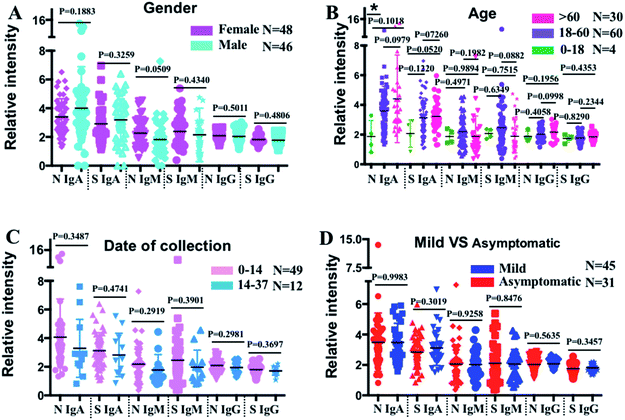 |
| Fig. 3 Cross-sectional SARS-CoV-2 antibody responses. IgM, IgG, and IgA antibody responses against N and S antigens in patients with different genders (A), ages (B), sampling times (C) and disease status (D). Comparisons between groups were made by the two-sided t-test. The solid line denotes the median value of each antibody. The data are presented as relative intensity by dividing the median values of 110 negative controls. (*) P < 0.05. | |
Defining antibody features associated with different disease severities
Although substantial progress has been made to understand the immune response to SARS-CoV-2,24–26 the serological response in patients with different clinical severities remains unclear. We analyzed the association between anti-SARS-CoV-2-S and -N antibodies and disease severity in our patient cohort. The COVID-19 patients included in this study were split into three categories according to their disease severity: mild (n = 76), moderate (n = 14), and severe or critical (n = 17). The mild, moderate, severe and critical COVID-19 were defined according to the COVID-19 Treatment Guidelines by the National Institutes of Health (https://www.covid19treatmentguidelines.nih.gov/overview/clinical-spectrum/). The levels of anti-SARS-CoV-2-S and -N IgA, anti-SARS-CoV-2-S IgM, and anti-SARS-CoV-2-S and -N IgG were significantly higher in severe than in mild patients (Fig. 4A), suggesting a potential association between these antibodies and disease severity. To conduct further in-depth analysis, the patients were clustered into five subgroups by tSNE based on their IgM, IgG, and IgA levels against SARS-CoV-2-S and -N proteins (Fig. 4B left and S2‡), and the distribution of mild (n = 76) and non-mild (moderate and severe/critical) patients (n = 31) in different subgroups was annotated in the tSNE map by purple and red dots (Fig. 4B right). We found that more non-mild patients are distributed in the region of group 1 and a significantly higher ratio of severe patients in group 1 was observed compared with the other four subgroups (Fig. 4C). To uncover the antibody characteristics of severe patients, each antibody level among the five subgroups was compared as shown in Fig. 4D and S3.‡ For group 1, the patients exhibited evident higher IgA levels against SARS-CoV-2-S and -N proteins. The higher expression level of these two types of antibodies for patients of group 1 could be further noted from the tSNE map of Fig. 4E, which shows an obvious higher intensity (red color) in the group 1 region compared with other regions (blue to yellow color). The fold changes of median values of S protein and N protein specific IgA were 2.7 and 2.1 when compared with the group with the corresponding lowest antibody level. The most evident higher value of IgA response to the S protein indicates the potential of anti-SARS-CoV-2-S IgA as a biomarker of moderate to severe/critical COVID-19.
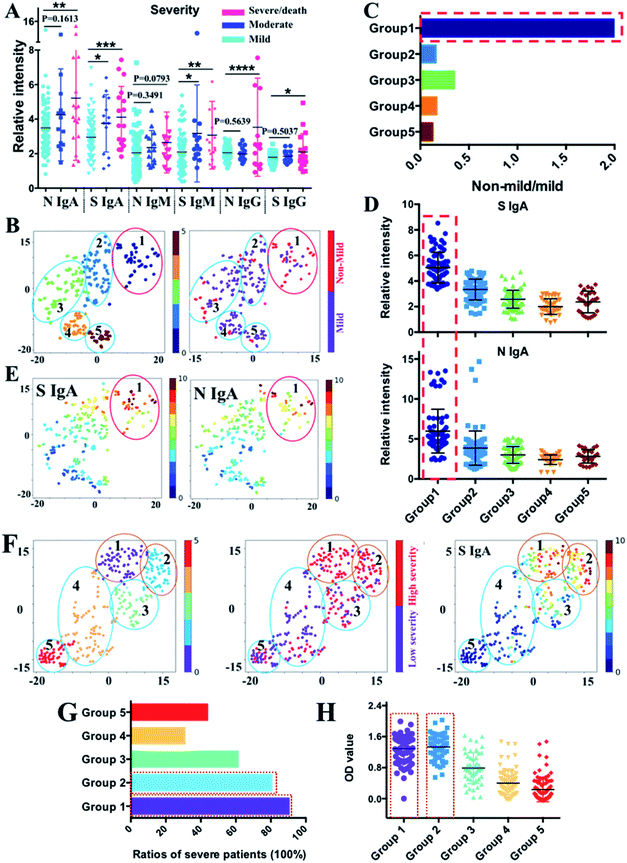 |
| Fig. 4 Correlation between antibody pattern and disease severity. (A) Comparison of IgA, IgM and IgG antibody responses to N and S proteins between different patient groups with different levels of severity (mild, moderate and severe/death). Comparisons between groups were made by the two-sided t-test. Each dot indicates one serum sample either from the mild group (n = 76), moderate group (n = 14) or the severe group (n = 17). The solid line denotes the median value of each antibody. (*) P < 0.05, (**) < 0.01, (***) < 0.001, and (****) < 0.0001. (B) tSNE map for patient classification based on the antibody features (left) with distribution information of mild (purple dots) and non-mild individuals (red dots) in each subpopulation (right). Different subgroups were differentiated by different colours. (C) Components of non-mild and mild patients in each group. Evident higher ratios of non-mild patients were displayed in group 1. (D) Comparison of IgA responses to S and N proteins between different subgroups classified by tSNE. (E) tSNE map shows the intensity distribution of S and N protein specific IgA across all the five subpopulations classified by tSNE. (F) tSNE map for patient classification based on antibody features from published ELISA data27 (left); distribution information of patients in low severity (purple dots) and high severity (red dots) in each subpopulation (middle); the tSNE map shows the intensity distribution of S protein specific IgA across all five subpopulations classified by tSNE (right). (G) Comparison of the ratios of patients in severe conditions among different subgroups. Higher ratios of patients with higher severity levels were displayed in group 1 and 2. (H) Comparison of IgA level responses to the S protein between different subgroups. The x- and y-axes of each tSNE figure represent t-SNE dimension 1 and dimension 2, respectively. | |
To validate this, we used the high dimensional visualization algorithm to further analyze 302 samples with antibodies profiled by ELISA published recently.27 The optical density (OD) corresponding to anti-SARS-CoV-2-S and -N IgA, IgM, and IgG was used to generate the tSNE map for subpopulation classification. As shown in Fig. 4F (left), the patients could be classified into 5 subgroups based on their antibody profiles. By incorporating the information on severity into the tSNE map (Fig. 4F, middle), the association between antibody intensity and disease severity could be elicited. The highest anti-SARS-CoV-2-S IgA level was found in groups 1 and 2 (Fig. 4F, right and 4H), among which the highest ratio of patients with severe/critical COVID-19 was observed (Fig. 4G), further confirming the elevated IgA response to the S protein as a common immune response feature for more severe COVID-19 patients. Collectively, our findings demonstrate the association between anti-SARS-CoV-2-S IgA and disease severity, further indicating its potential role as a biomarker for severity stratification.
Machine learning-based decision tree classifier indicates anti-SARS-CoV-2-S IgA as the first criterion for disease severity stratification
As one of the most popular and powerful machine learning algorithms, the decision tree has been widely used for both classification and regression tasks with an easily understandable tree model. To further uncover an optimized classification criterion for stratification of COVID-19 disease severity, a decision tree model was built based on the levels of six types of antibodies in 107 COVID-19 patients i.e., anti-SARS-CoV-2-S and -N IgA, IgM, and IgG (Fig. 5). The decision tree was generated based on six antibody variables, i.e., IgA, IgM and IgG antibodies against S and N proteins, by an open-source machine learning library scikit-learn in Python 3.6. The tree has a depth of 3 and the samples at each node were classified based on the splitting criterion shown in each node. By using only six decision nodes (anti-SARS-CoV-2-S IgA and IgG, and anti-SARS-CoV-2-N IgA, IgM and IgG), 89% accuracy could be achieved for the classification of mild (n = 76) and non-mild (n = 31) patients. Using this prediction model, 100% of mild COVID-19 patients (76/76) could be discriminated from all the cases included in the study (n = 107). Importantly, the results indicate that the anti-SARS-CoV-2-S IgA level was the first significant predictor for differentiating mild from non-mild disease. A cutoff value of 4.478 (IgA intensity against S protein/mean value of the 110 negative controls) was applied as the first splitting condition in the root of the tree structure, and 92% (70/76) mild patients could be identified based on this criterion, further confirming the importance of IgA response to the S protein for severity stratification of COVID-19 patients. This model could be further optimized by an increase in the patient data sets for model construction to further improve the accuracy in stratifying patients. This machine learning approach may facilitate decision-making in clinics for the prediction of disease severity and patient stratification in the future. Furthermore, in agreement with our findings, the decision tree model generated by the published ELISA data26 also strongly suggests anti-SARS-CoV-2-S IgA as the first criterion for disease severity stratification (Fig. S4‡).
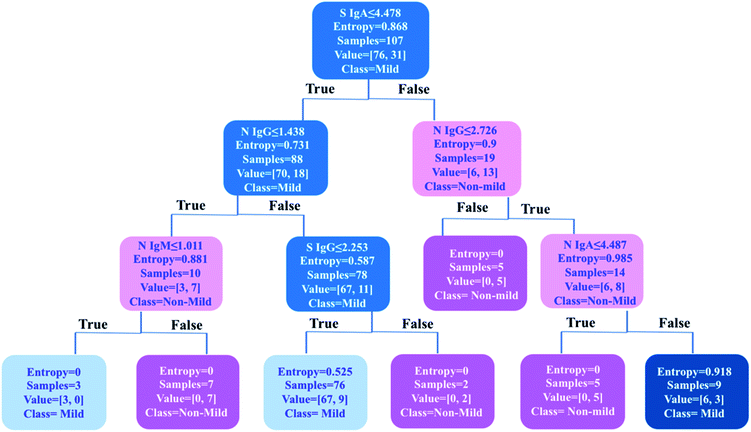 |
| Fig. 5 Graphical representation of the decision tree model based on antibody features for disease severity classification. The colour of the nodes indicates the severity level: blue = mild and pink = non-mild (moderate/severe/death). 95 cases (107 cases in total) are correctly classified (accuracy = 89%). The shades of colour indicate the proportion of mild or non-mild patients included in the indicated class. Entropy indicated in the decision tree stands for homogeneity in each classified subgroup. | |
Discussion
Serological antibody assays could provide important information that is not found in molecular tests, including past-exposure to the virus, immune status, and response to vaccination. Although different strategies have been reported for serum antibody profiling in COVID-19 patients,9,10,28 multiplex antibody quantification assays remain under-developed. The limitations of monoplex antibody assays include the higher demand of time and sample volume, and the lack of correlation among different types of antibodies. In this study, by taking advantage of the high sensitivity of ICP-MS for quantification of lanthanides and target molecule enrichment by using magnetic beads, a novel and highly sensitive MMDA platform was established for COVID-19 diagnosis and patient stratification. The MMDA exhibits significantly higher accuracy than the widely used ELISA. Given the minimal interference among different metals when quantified by ICP-MS, we performed multiplex antibody profiling on the serum samples of 107 COVID-19 patients and 110 controls. A significant increase in the levels of all the evaluated antibodies was observed among individuals with COVID-19 compared with the controls. Interestingly, we found that 40.2% of the COVID-19 patients did not exhibit positive seroconversion in terms of IgM levels, which was likely caused by the gradual decrease of IgM levels with time after symptom onset.
Efforts have been devoted to understanding the dynamic change of immune response with the time after symptom onset.7,26,29–31 We have previously shown that,32 for COVID-19 patients with serum samples available for two weeks or longer after symptom onset, the rates of seropositivity for anti-NP IgG, anti-NP IgM, anti-RBD IgG and anti-RBD IgM were 94%, 88%, 100% and 94%, respectively. Another study demonstrated that anti-SARS-CoV-2 IgG was detected in 100% of the patients within 19 days after symptom onset and the peak value was obtained 3–4 weeks post-infection.8 Although anti-SARS-CoV-2 IgG levels remain relatively stable for up to 3 months, the rapid decline in IgA and IgM levels is well recognized.7 Our MMDA platform allows multiplex antibody profiling to simultaneously detect and quantify IgG, IgA, and IgM antibody levels. Using tSNE for individual classification, high sensitivity (95.3%) and specificity (94.5%) were achieved even for our patient cohort in which the majority of patients were asymptomatic or mildly symptomatic (71%, 76/107). Importantly, our findings indicated that anti-SARS-CoV-2-N IgG and IgA were amongst the most efficient antibodies for COVID-19 diagnosis. This may be due to the more abundant expression of SARS-CoV-2-N proteins than S proteins during viral replication.33 It has recently been reported that anti-SARS-CoV-2-N antibody response appears earlier than the anti-SARS-CoV-2-S antibody response,22,34 which makes the detection of the anti-SARS-CoV-2-N antibody a useful strategy for earlier diagnosis of COVID-19. As for IgG and IgA, the time it takes for a positive IgA response to be apparent is markedly shorter than that required for an IgG response.35 The simultaneous detection of both IgA and IgG may help to minimize false-negative results caused by the dynamic changes of these antibodies at different time points after SARS-CoV-2 infection. Furthermore, it has been shown that a positive correlation exists between antibody responses from paired serum and saliva samples,7 and so the simultaneous monitoring of viral load and immune response to SARS-CoV-2 infection in saliva may be an earlier and efficient surrogate method for diagnosing COVID-19.
The correlation between different types of antibodies and disease severity remains unclear.36 In this study, we evaluated the antibody responses of COVID-19 patients with different disease severities by comprehensively comparing each antibody intensity across different subpopulations. Our findings showed that a higher anti-SARS-CoV-2-S IgA level was found in severe COVID-19 patients.
Using a machine learning-based decision tree algorithm, we further demonstrated that anti-SARS-CoV-2-S IgA was the first significant predictor for differentiating mild and non-mild COVID-19 patients, suggesting the potential role of IgA as a biomarker of disease severity. Our results are in line with recent reports that higher levels of anti-SARS-CoV-2-S IgA were observed in severe COVID-19 patients.27,36–38 Moreover, it has also been reported that individuals with severe disease exhibit a delayed, but eventually very strong and broad IgA response.39 Neutralizing antibodies have been demonstrated to play a critical role in preventing virus reinfection, and the SARS-CoV-2-S protein is an important target for neutralizing antibodies owing to its capability of preventing viral entry into host cells.13,40 A recent systematic study on the acute humoral responses to SARS-CoV-2 found that IgA, particularly anti-S-RBD IgA, contributes more to serum neutralization than IgG does in the early phase of the infection. It, however, remains unclear whether a high level of IgA in the early phase of infection has a potentially positive or negative influence on disease outcome.35
Several studies have tried to explore the relationship between the extent of the neutralizing immune response and disease severity, and they proposed that asymptomatic individuals with COVID-19 mount a neutralizing humoral response that is lower than that observed in symptomatic or hospitalized COVID-19 patients with COVID-19.41,42 These results indicate that asymptomatic or mild-symptomatic COVID-19 patients generally exhibit a weaker immune response to SARS-CoV-2 infection, especially for neutralizing antibody response. Given the S protein specific IgA as the potential major neutralizing antibody,35,43 our finding that individuals with non-mild symptoms exhibited higher IgA response to the S protein than mildly ill patients provides further evidence that mildly symptomatic patients exhibit weaker neutralizing antibody responses.
Conclusions
In summary, we have developed a MMDA platform as a general strategy for multiplex biofluid assay. The high sensitivity and minimal interference among different metal signals (i.e., masses) endow MMDA with an extremely high multiplexing capability (theoretically higher than 100 metals with different masses) for biofluid assay. MMDA serves as a promising alternative method to the currently widely used fluorescence-based method.13 The main limitation of MMDA is the possible influence of cross-interactions between different captured antibodies or antigens, which is commonly suffered by all multiplexed assays. Therefore, the cross-reactivity of antibodies needs to be evaluated with optimized concentrations to minimize the side effects caused by cross-interactions. Using COVID-19 as a showcase, we applied MMDA for serological profiling of the COVID-19 disease. Superior performance for COVID-19 diagnosis is achieved for MMDA compared to ELISA in terms of accuracy. The incorporation of more parameters into our detection system (e.g. more antibodies of different isotypes or different classes, inflammation factors, etc.) for serological profiling will provide more invaluable information to interrogate the mechanism of COVID-19 progression. Further assessment of the antibody profile using a high dimensional visualization tool and machine learning algorithm enables more potent biomarkers for COVID-19 diagnosis (N protein specific IgG and IgA) and disease severity classification (S protein specific IgA) to be uncovered, providing valuable information for COVID-19 patient stratification. Given that MMDA is a metal detection based method, it is readily applicable to all the instruments with metal quantification capability. More importantly, the machine learning method proposed in this work is suitable for the current commercially available multiplex methods (e.g. Luminex, cytometric bead array assay, etc.). Such a strategy of combining a high dimensional data analysis tool with a multiplexed assay provides a generalized streamlined approach for the discovery of biomarkers in different emerging diseases.
Data availability
Most important data to get the major conclusion in the paper are present in the ESI file.‡ Additional data related to this paper could be requested from the authors.
Author contributions
Y. Z., J. F.-W. C., S. Y. and H. S. designed the experiments. Y. Z. and X. X. performed ICP-MS assay. S. Y., J.-P. C. and C. L. performed ELISA assay. K. K.-W. T., I. F.-N. H., K.-H. C., K.-Y. Y. and J. F.-W. C. collected the clinical samples and provided the clinical information. Y. Z. analyzed all the data. Y. Z. wrote the manuscript with modifications and further inputs from S. Y., H. L., K.-Y. Y., Y. L., J. F.-W. C. and H. S. J. F.-W. C. and H. S. supervised the project.
Conflicts of interest
There are no conflicts to declare.
Acknowledgements
This study was partly supported by funding from Health@InnoHK, Innovation and Technology Commission (ITS/278/20), the Government of the Hong Kong SAR; the General Research Fund (17119821), the Senior Research Fellow Scheme (2122-7S04) and Theme-Based Research Scheme (T11-709/21-N) of the Research Grants Council of Hong Kong SAR; the Consultancy Service for Enhancing Laboratory Surveillance of Emerging Infectious Diseases and Research Capability on Antimicrobial Resistance for Department of Health of the Hong Kong SAR; Sanming Project of Medicine in Shenzhen, China (SZSM201911014); and High Level Hospital Program, Health Commission of Guangdong Province, China; the Research Project of Hainan Academician Innovation Platform (YSPTZX202004); the University of Hong Kong Outstanding Young Researcher Award; and the University of Hong Kong Research Output Prize (Li Ka Shing Faculty of Medicine); and donations from the Shaw Foundation Hong Kong, Norman & Cecilia Foundation (HKU), Richard Yu and Carol Yu, Michael Seak-Kan Tong, May Tam Mak Mei Yin, Lee Wan Keung Charity Foundation Limited, Hong Kong Sanatorium & Hospital, Hui Ming, Hui Hoy and Chow Sin Lan Charity Fund Limited, Chan Yin Chuen Memorial Charitable Foundation, Marina Man Wai Lee, the Hong Kong Hainan Commercial Association South China Microbiology Research Fund, the Jessie & George Ho Charitable Foundation, Perfect Shape Medical Limited, Kai Chong Tong, Tse Kam Ming Laurence, Foo Oi Foundation Limited, Betty Hing-Chu Lee, Ping Cham So, and Lo Ying Shek Chi Wai Foundation. The funding sources had no role in the study design, data collection, analysis, interpretation, or writing of the report.
References
- F. Wu, S. Zhao, B. Yu, Y. M. Chen, W. Wang, Z. G. Song, Y. Hu, Z. W. Tao, J. H. Tian and Y. Y. Pei, Nature, 2020, 579, 265–269 CrossRef CAS PubMed.
- P. Zhou, X. L. Yang, X. G. Wang, B. Hu, L. Zhang, W. Zhang, H. R. Si, Y. Zhu, B. Li and C. L. Huang, Nature, 2020, 579, 270–273 CrossRef CAS PubMed.
- J. F. W. Chan, S. Yuan, K. H. Kok, K. K. W. To, H. Chu, J. F. Yang, F. Xing, J. Liu, C. C. Y. Yip and R. W. S. Poon, Lancet, 2020, 395, 514–523 CrossRef CAS.
- X. Li, J. F. W. Chan, K. K. W. Li, E. Y. K. Tso, C. C. Y. Yip, S. Sridhar, T. W. H. Chung, K. H. Y. Chiu, D. L. L. Hung and A. K. L. Wu, Infection, 2021, 49, 257–265 CrossRef CAS PubMed.
- W. Feng, A. M. Newbigging, C. Le, B. Pang, H. Peng, Y. Cao, J. Wu, G. Abbas, J. Song and D. B. Wang, Anal. Chem., 2020, 92, 10196–10209 CrossRef CAS PubMed.
- Y. Xu, X. Li, B. Zhu, H. Liang, C. Fang, Y. Gong, Q. Guo, X. Sun, D. Zhao and J. Shen, Nat. Med., 2020, 26, 502–505 CrossRef CAS PubMed.
- B. Isho, K. T. Abe, M. Zuo, A. J. Jamal, B. Rathod, J. H. Wang, Z. Li, G. Chao, O. L. Rojas and Y. M. Bang, Sci. Immunol., 2020, 5, eabe5511 CrossRef PubMed.
- Q. X. Long, B. Z. Liu, H. J. Deng, G. C. Wu, K. Deng, Y. K. Chen, P. Liao, J. F. Qiu, Y. Lin and X. F. Cai, Nat. Med., 2020, 26, 845–848 CrossRef CAS PubMed.
- C. H. GeurtsvanKessel, N. M. Okba, Z. Igloi, S. Bogers, C. W. Embregts, B. M. Laksono, L. Leijten, C. Rokx, B. Rijnders and J. Rahamat-Langendoen, Nat. Commun., 2020, 11, 1–5 Search PubMed.
- H. W. Jiang, Y. Li, H. N. Zhang, W. Wang, X. Yang, H. Qi, H. Li, D. Men, J. Zhou and S. C. Tao, Nat. Commun., 2020, 11, 1–11 Search PubMed.
- R. Wölfel, V. M. Corman, W. Guggemos, M. Seilmaier, S. Zange, M. A. Müller, D. Niemeyer, T. C. Jones, P. Vollmar and C. Rothe, Nature, 2020, 581, 465–469 CrossRef PubMed.
- F. Krammer and V. Simon, Science, 2020, 368, 1060–1061 CrossRef CAS PubMed.
- M. Norman, T. Gilboa, A. F. Ogata, A. M. Maley, L. Cohen, E. L. Busch, R. Lazarovits, C. P. Mao, Y. Cai and J. Zhang, Nat. Biomed. Eng., 2020, 4, 1180–1187 CrossRef CAS PubMed.
- O. Vandenberg, D. Martiny, O. Rochas, A. van Belkum and Z. Kozlakidis, Nat. Rev. Microbiol., 2021, 19, 171–183 CrossRef CAS PubMed.
- G. Han, S. Zhang, Z. Xing and X. Zhang, Angew. Chem., Int. Ed., 2013, 125, 1506–1511 CrossRef PubMed.
- X. Yan, Y. Luo, Z. Zhang, Z. Li, Q. Luo, L. Yang, B. Zhang, H. Chen, P. Bai and Q. Wang, Angew. Chem., Int. Ed., 2012, 51, 3358–3363 CrossRef CAS PubMed.
- P. Zhang, S. Misra, Z. Guo, M. Rehkämper and E. Valsami-Jones, Nat. Protoc., 2019, 14, 2878–2899 CrossRef CAS PubMed.
- S. C. Bendall, E. F. Simonds, P. Qiu, D. A. El-ad, P. O. Krutzik, R. Finck, R. V. Bruggner, R. Melamed, A. Trejo and O. I. Ornatsky, Science, 2011, 332, 687–696 CrossRef CAS PubMed.
- S. C. Wilschefski and M. R. Baxter, Clin. Biochem. Rev., 2019, 40, 115 Search PubMed.
- M. H. Spitzer and G. P. Nolan, Cell, 2016, 165, 780–791 CrossRef CAS PubMed.
- D. S. Ong, S. de Man, F. A. Lindeboom and J. G. Koeleman, Clin. Microbiol. Infect., 2020, 26(1094), e7–1094 Search PubMed.
- L. Grzelak, S. Temmam, C. Planchais, C. Demeret, L. Tondeur, C. Huon, F. Guivel-Benhassine, I. Staropoli, M. Chazal and J. Dufloo, Sci. Transl. Med., 2020, 12, eabc3103 CrossRef CAS PubMed.
- D. Kobak and G. C. Linderman, Nat. Biotechnol., 2021, 39, 156–157 CrossRef CAS PubMed.
- Y. Su, D. Chen, D. Yuan, C. Lausted, J. Choi, C. L. Dai, V. Voillet, V. R. Duvvuri, K. Scherler and P. Troisch, Cell, 2020, 183, 1479–1495 CrossRef CAS PubMed.
- Y. Zuo, S. K. Estes, R. A. Ali, A. A. Gandhi, S. Ya-lavarthi, H. Shi, G. Sule, K. Gockman, J. A. Madison and M. Zuo, Sci. Transl. Med., 2020, 12, eabd3876 CrossRef CAS PubMed.
- S. P. Weisberg, T. J. Connors, Y. Zhu, M. R. Baldwin, W. H. Lin, S. Wontakal, P. A. Szabo, S. B. Wells, P. Dogra and J. Gray, Nat. Immunol., 2021, 22, 25–31 CrossRef PubMed.
- J. Seow, C. Graham, B. Merrick, S. Acors, S. Pickering, K. J. Steel, O. Hemmings, A. O'Byrne, N. Kouphou and R. P. Galao, Nat. Microbiol., 2020, 5, 1598–1607 CrossRef CAS PubMed.
- A. N. Grossberg, L. A. Koza, A. Ledreux, C. Prusmack, H. K. Krishnamurthy, V. Jayaraman, A. C. Granholm and D. A. Linseman, Nat. Commun., 2021, 12, 1–11 CrossRef PubMed.
- K. Röltgen, A. E. Powell, O. F. Wirz, B. A. Stevens, C. A. Hogan, J. Najeeb, M. Hunter, H. Wang, M. K. Sahoo and C. Huang, Sci. Immunol., 2020, 5, eabe0240 CrossRef PubMed.
- A. S. Iyer, F. K. Jones, A. Nodoushani, M. Kelly, M. Becker, D. Slater, R. Mills, E. Teng, M. Kamruzzaman and W. F. Garcia-Beltran, Sci. Immunol., 2020, 5, eabe0367 CrossRef PubMed.
- C. Lucas, P. Wong, J. Klein, T. B. Castro, J. Silva, M. Sundaram, M. K. Ellingson, T. Mao, J. E. Oh and B. Israelow, Nature, 2020, 584, 463–469 CrossRef CAS PubMed.
- K. K. W. To, O. T. Y. Tsang, W. S. Leung, A. R. Tam, T. C. Wu, D. C. Lung, C. C. Y. Yip, J. P. Cai, J. M. C. Chan and T. S. H. Chik, Lancet Infect. Dis., 2020, 20, 565–574 CrossRef CAS PubMed.
- C. Atyeo, S. Fischinger, T. Zohar, M. D. Slein, J. Burke, C. Loos, D. J. McCulloch, K. L. Newman, C. Wolf and J. Yu, Immunity, 2020, 53, 524–532 CrossRef CAS PubMed.
- L. Guo, L. Ren, S. Yang, M. Xiao, D. Chang, F. Yang, C. S. Dela Cruz, Y. Wang, C. Wu and Y. Xiao, Clin. Infect. Dis., 2020, 71, 778–785 CrossRef CAS PubMed.
- D. Sterlin, A. Mathian, M. Miyara, A. Mohr, F. Anna, L. Claër, P. Quentric, J. Fadlallah, H. Devilliers and P. Ghillani, Sci. Transl. Med., 2021, 13, eabd2223 CrossRef CAS PubMed.
- S. Ravichandran, Y. Lee, G. Grubbs, E. M. Coyle, L. Klenow, O. Akasaka, M. Koga, E. Adachi, M. Saito and I. Nakachi, Sci. Adv., 2021, 7, eabf2467 CrossRef PubMed.
- H. Q. Yu, B. Q. Sun, Z. F. Fang, J. C. Zhao, X. Y. Liu, Y. M. Li, X. Z. Sun, H. F. Liang, B. Zhong and Z. F. Huang, Eur. Respir. J., 2020, 56, 2001526 CrossRef CAS PubMed.
- J. Tang, S. Ravichandran, Y. Lee, G. Grubbs, E. M. Coyle, L. Klenow, H. Genser, H. Golding and S. Khurana, Nat. Commun., 2021, 12, 1–13 CrossRef PubMed.
- C. Dahlke, J. Heidepriem, R. Kobbe, R. Santer, T. Koch, A. Fathi, M. L. Ly, S. Schmiedel, P. H. Seeberger and M. M. Addo, Pathogens, 2021, 10, 438 CrossRef PubMed.
- M. Letko, A. Marzi and V. Munster, Nat. Microbiol., 2020, 5, 562–569 CrossRef CAS PubMed.
- D. F. Robbiani, D. F. Gaebler, C. Muecksch, F. Lorenzi, J. C. Wang, Z. Cho, A. Agudelo, M. Barnes, C. O. Gazumyan and A. Finkin, Nature, 2020, 584, 437–442 CrossRef CAS PubMed.
- Q. X. Long, X. J. Tang, Q. L. Shi, Q. Li, H. J. Deng, J. Yuan, J. L. Hu, W. Xu, Y. Zhang and F. J. Lv, Nat. Med., 2020, 26, 1200–1204 CrossRef CAS PubMed.
- W. Zeng, H. Ma, C. Ding, Y. Yang, Y. Sun, X. Huang, W. He, Y. Xiang and Y. Gao, Signal Transduction Targeted Ther., 2021, 6, 35 CrossRef CAS PubMed.
Footnotes |
† Dedicated to Prof. Zhifang Chai on the occasion of his 80th birthday. |
‡ Electronic supplementary information (ESI) available. See DOI: 10.1039/d1sc05852e |
|
This journal is © The Royal Society of Chemistry 2022 |
Click here to see how this site uses Cookies. View our privacy policy here.