DOI:
10.1039/D2SC03326G
(Edge Article)
Chem. Sci., 2022,
13, 12403-12418
Highly effective identification of drug targets at the proteome level by pH-dependent protein precipitation†
Received
14th June 2022
, Accepted 20th September 2022
First published on 30th September 2022
Abstract
Fully understanding the target spaces of drugs is essential for investigating the mechanism of drug action and side effects, as well as for drug discovery and repurposing. In this study, we present an energetics-based approach, termed pH-dependent protein precipitation (pHDPP), to probe the ligand-induced protein stability shift for proteome-wide drug target identification. We demonstrate that pHDPP works for a diverse array of ligands, including a folate derivative, an ATP analog, a CDK inhibitor and an immunosuppressant, enabling highly specific identification of target proteins from total cell lysates. This approach is compared to thermal and solvent-induced denaturation approaches with a pan-kinase inhibitor as the model drug, demonstrating its high sensitivity and high complementarity to other approaches. Dihydroartemisinin (DHA), a dominant derivative of artemisinin to treat malaria, is known to have an extraordinary effect on the treatment of various cancers. However, the anti-tumor mechanisms remain unknown. pHDPP was applied to reveal the target space of DHA and 45 potential target proteins were identified. Pathway analysis indicated that these target proteins were mainly involved in metabolism and apoptosis pathways. Two cancer-related target proteins, ALDH7A1 and HMGB1, were validated by structural simulation and AI-based target prediction methods. And they were further validated to have strong affinity to DHA by using cellular thermal shift assay (CETSA). In summary, pHDPP is a powerful tool to construct the target protein space to reveal the mechanism of drug action and would have broad application in drug discovery studies.
Introduction
Fully understanding the target spaces of drugs is essential for investigating the mechanism of drug action and side effects, as well as for drug discovery and repurposing.1–3 In the past few years, modification-free approaches emerged as innovative approaches to decipher drug–protein interactions,4–6 which are usually based on the conformational change of target proteins induced by binding with ligands. These modification-free approaches overcome the disadvantages of traditional drug-modified methods such as activity-based protein profiling (ABPP) and affinity chromatography, which require chemical modification or immobilization of compounds,7,8 thereby reducing the risk of non-specific binding and affinity variations.
Till now, different physicochemical stresses including temperature, proteolysis, oxidation, organic solvent and mechanical force have been exploited to distinguish the stability shift or structural change between the ligand-bound protein and the free protein.9–13 Among them, a temperature based protein precipitation method, i.e., thermal proteome profiling (TPP) approach,14,15 is the prevailing method. The TPP method, which combines cellular thermal shift assay (CETSA)16 with mass spectrometry-based proteomics, is based on the principle that the ligand binding protein has higher resistance to heating induced precipitation than the free protein.17 It has been successfully applied to identify targets or off-targets for some drugs such as antihistamine clemastine18 and panobinostat.19 Another protein precipitation-based approach for target identification, solvent-induced protein precipitation approach (SIP), was developed by us.12 The SIP approach was successfully employed to screen the target proteins of the naphthoquinone natural product Shikonin (SHK) and revealed that SHK binds with the NEMO/IKKβ complex.20 Recently, solvent proteome profiling (SPP) and solvent proteome integral solubility alteration (solvent-PISA) approaches were established by combining SIP with modern quantitative proteomics to monitor target engagement.21
Although the two precipitation based modification-free approaches provide novel solutions for target identification, they still have the coverage problem. For instance, TPP is unable to identify target proteins that are not responsive to temperature.17,21 The cognate target BCR-ABL did not yield significant stability shifts upon binding with the BCR-ABL inhibitor dasatinib in the TPP approach.17 Furthermore, the thermal shifts of some proteins could be captured only under extreme temperature conditions; for example, the temperature windows of thermal stability shifts of DCK22 and MetAP2 (ref. 23) are within the range of 60–85 °C, which made the identification of those targets a failure in the conventional TPP method within the range of 37–60 °C. Similarly, SIP is unable to cover the entire target landscape of a ligand either. Therefore, alternative and complementary approaches are required to improve the target protein identification.
Proteins can be unfolded and precipitated under different denaturation conditions. Each denaturation condition may have a different effect on protein unfolding. The different sensitivity of proteins to various denaturation conditions results in different protein solubility, which ultimately leads to the identification of different target proteins when those denaturation methods are used to precipitate proteins for target protein screening. In addition to heating and organic solvent treatment, changing pH values could also denature proteins. For instance, decreasing pH will break the hydrogen bond of the protein, and at the same time will make the protein positively charged, and thus form an insoluble complex with the acid reagent anion.24,25 It is reasonable to think that the proteins that are insensitive to thermal and solvent denaturation may be sensitive to pH-induced denaturation.
Herein, we proposed a pH-dependent protein precipitation (pHDPP) approach for proteome-wide drug target identification and demonstrated its effectiveness by applying this approach to a series of model drugs. Ascorbic acid (Vc) and citric acid (CA) are finally developed from various acid agents as denaturation stresses for target identification of ligands. The known targets for all tested compounds were successfully identified by Vc and CA-based pHDPP. Surprisingly, pHDPP was observed with higher sensitivity in target identification as compared with TPP and SIP, which may be attributed to acidic agent treatment that more potently affected the stability shifts of target proteins than heating and solvent treatment. We demonstrated that the targets identified by pHDPP, TPP and SIP approaches were strongly complementary. Therefore, the integrated drug target identification platform by combining approaches with different mechanisms would allow more comprehensive target identification. Finally, pHDPP was applied to identify the targets of dihydroartemisinin (DHA), revealing unknown protein targets that could explain the anti-tumor effect of DHA. Meanwhile, the structural simulation, network pharmacology and AI-based target prediction methods were applied to validate the identification results of pHDPP for DHA. Taken together, we anticipate that pHDPP would become a powerful tool to reveal ligand–protein interactomes.
Results and discussion
The strategy of pHDPP for proteome-wide identification of drug targets
Proteins can be gradually denatured and precipitated by the decrease of the pH value. Similar to high temperature in TPP and organic solvent in SIP, we reason that acidic agents could also be used to precipitate proteins to monitor the target engagement. This method was termed the pH-dependent protein precipitation (pHDPP) approach in this study. In a typical pHDPP profiling (Fig. 1A), cell lysates were incubated with and without a ligand, and then divided into several aliquots, respectively. These aliquots were treated with increasing concentrations of acidic agent to decrease the pH value to initiate protein denaturation. Proteins subsequently aggregate with increasing concentrations of acidic agent, resulting in a gradual decrease in their abundances in the soluble fractions and a gradual increase in their abundances in the insoluble fractions. The soluble fractions were separated from precipitates by centrifugation, and the equal volumes of supernatants or precipitates in the ligand and vehicle groups were digested into tryptic peptides and then were subjected to isotopic labeling with different labeling strategies such as dimethyl labeling and neutron-encoded isobaric tandem mass tags (TMT 10-plex) prior to LC-MS/MS. In the case of the dimethyl labeling strategy, the stability shifts of proteins induced by a ligand can be detected by measuring the fold changes in the protein abundance with or without a ligand. In the case of the TMT 10-plex labeling strategy, vehicle and drug-induced samples were treated with 5 concentrations of acidic agent, and the resulting 10 supernatants were digested by using trypsin, and isotopically labeled with one set of TMT 10-plex. After labeling, the 10 samples were pooled together and fractionated by high pH RPLC before LC-MS/MS analysis.
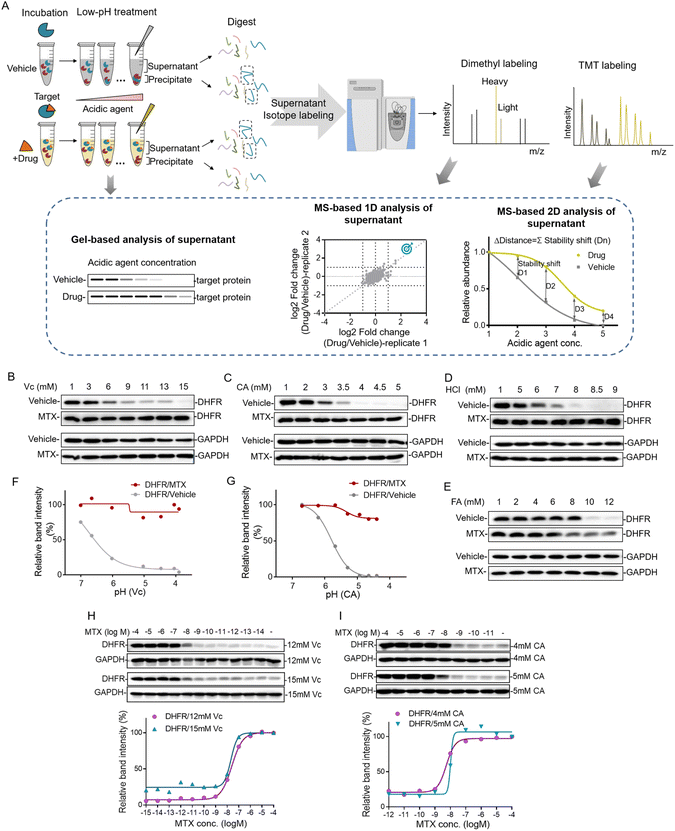 |
| Fig. 1 Workflow of the pH dependent protein precipitation (pHDPP) approach and the investigation of the acidic agents for drug target identification. (A) Schematic representation of the pHDPP approach for target protein identification. Cell lysate was incubated with and without a ligand, and then divided into several aliquots, each of which was subjected to treatment with an acidic agent with a concentration gradient. Soluble fractions treated with a ligand or vehicle were separated from the aggregate by centrifugation. The abundances of proteins in the supernatant or precipitate were detected by antibody-based immunoblotting for target protein verification, or dimethyl and TMT 10 label-based quantitative proteomics for target protein identification. Western blotting confirmed that MTX stabilized the known target DHFR in 293T cell lysate after treating with concentration gradients of (B) Vc (ascorbic acid), (C) CA (citric acid), (D) HCl (hydrochloric acid) and (E) FA (formic acid). The relative band intensities of DHFR in Vc and CA experiments were quantified based on the above western blotting, and they were shown as a function of the solution pH (F and G). Drug dose-dependent profile of the target protein DHFR for MTX in the 293T cell lysate treated with (H) 12 mM and 15 mM Vc and (I) 4 mM and 5 mM CA. The relative band intensities of DHFR were quantified based on the above western blotting. | |
The target engagement was determined by the calculation of Δdistance (abundance change) between the drug and vehicle for each quantified protein across 5 acidic agent concentrations in pHDPP. Therefore, only one set of TMT 10-plex per experiment is required. The Δdistance reflects the stability shift of a protein upon ligand binding. The higher the Δdistance, the greater the stability shift of a protein after the binding of a ligand. If the Δdistance value of a protein is positive, it means that the protein is stabilized. The stabilized proteins are likely to bind ligands directly in most cases, but indirectly in rare cases. In contrast, a negative Δdistance indicates that the protein is destabilized after incubation with a ligand. For this case, the ligand may indirectly bind to the protein. The destabilization of a protein could be because it is dissociated from a protein complex after the ligand binds to another protein in the protein complex.17 The identified candidate targets can also be confirmed by detecting the abundance change of proteins in the supernatant of the ligand and vehicle groups by antibody-based immunoblotting.
Investigating the acidic agents for probing the drug–target interactions
The decrease of the pH value in the buffer will cause protein gradual denaturation and precipitation. In order to screen suitable denaturation agents for target protein identification, 4 commonly used acidic agents including ascorbic acid (Vc), citric acid (CA), hydrochloric acid (HCl) and formic acid (FA) were investigated. The concentration window of the acid agent to precipitate proteins in the cell lysate was a key parameter. For this purpose, we profiled the impact of increasing concentrations of different acidic agents on the proteome of K562 cells. For this purpose, the K562 cell lysate was treated with increasing concentrations of acidic agents (Vc was from 0–19 mM, CA was from 0–5.8 mM, HCl was from 0–13 mM and FA was from 0–13 mM). The soluble fractions were separated from aggregates by centrifugation and analyzed by SDS–PAGE. As shown in Fig. S1,† the majority of the proteome were denatured and precipitated at high acidic agent concentrations. The corresponding pH values for the tested concentrations of Vc, CA, HCl and FA in 10 mM PBS buffer were in the range of 3–7 (Table S1†).
Next, we investigated the feasibility of using acidic agents including Vc, CA, HCl and FA to probe the stabilization shift induced by ligand binding. Methotrexate (MTX), a folate derivative inhibiting dihydrofolate reductase (DHFR), was used as the model drug for this investigation. Increasing concentrations of Vc, CA, HCl and FA were applied to precipitate aliquots of 293T cell lysate treated with either MTX or DMSO, and then the supernatants were separated from the precipitate by centrifugation. The abundances of known target proteins in drug and vehicle groups were determined by immunoblotting. In the experiments treated with Vc and CA, we found that MTX protected its target protein DHFR from unfolding, while the abundance of free DHFR showed a more profound decrease in the supernatant with the increasing concentrations of acidic agents (Fig. 1B and C). In addition to Vc and CA, HCl and FA also displayed good performance in probing ligand induced stabilization (Fig. 1D and E). However, due to the low buffering capability and high volatility of these agents, they were not chosen for the subsequent investigation. Furthermore, we plotted the fitting curves of DHFR based on its intensity in the western blotting as a function of the solution pH. The results showed that free DHFR was more easily unfolded and precipitated as pH decreases, which demonstrated that pH was indeed the main factor affecting target protein denaturation/precipitation (Fig. 1F and G). The optimal Vc and CA concentration windows for target identification are within the range of 3–15 mM and 1–5 mM, respectively. The higher molar concentration of Vc for protein precipitation than CA is because the pKa of Vc (4.04) is higher than CA (3.13). However, the concentration range might need to be slightly adjusted according to the proteins of interest to yield sufficient stability shift.
The efficacy of a drug is closely related to its binding affinity with the target proteins.16 To investigate the feasibility of the Vc or CA-based pHDPP approach for the determination of binding affinity, we exploited a dose-dependent experiment to measure the affinity between DHFR and MTX. The stability shift of the target protein will increase with the increase of drug concentration. And it will reach the maximum signal response value at the plateau stage when all target binding sites are occupied by the drug. The drug concentration occupied half of the target (the half-saturation point), which is approximate to the Kd value, is roughly considered as the binding affinity. The dose-dependent response assay is performed at a defined acid agent concentration, across a range of compound concentrations. The 293T cell lysate exposed to different MTX concentrations was treated with 12 and 15 mM Vc, and the fitting curves of DHFR abundance in the soluble fraction showed that the half-saturation point of DHFR to MTX was at around 10−8 M, which was in line with the well-known Kd values (Fig. 1H). Furthermore, similar to Vc, CA was also used to test the ability of profiling affinity of drug–target interaction by our approach. Similarly, after treatment with 4 and 5 mM CA, the half-saturation point of DHFR to MTX was also at around 10−8 M based on the fitting curves of DHFR abundance in the soluble fraction (Fig. 1I). Thus, the above data indicated that our approach can determine the binding affinity of a specific target protein in the total cell lysate using western blotting readout. Large scale assessment of the affinity of a drug for the protein targets in a cell lysate could be achieved by coupling this method with the quantitative proteomic approach.
Identifying target proteins of ligands from the total cell lysate by using dimethyl labeling
Western blotting can only be used to confirm the target proteins, but was unable to reveal unknown target proteins. We then used the quantitative proteomics technique to identify target proteins from complex biological samples. Dimethyl stable isotope labeling has several advantages including fast and complete labeling and cost effectiveness.26 To further verify the feasibility of Vc to identify target proteins in the total cell lysate, we profiled the stability shifts of proteins treated with ligands by quantitative proteomics using the dimethyl labeling strategy. The immunoblotting in Fig. 1B indicated that the stability shift of DHFR by binding with MTX could be obviously observed in 6 and 9 mM Vc-treated samples. The two pairs of samples were then analyzed by quantitative proteomics for the identification of target proteins. The equal volumes of supernatants incubated with and without MTX were digested, dimethyl labeled and analyzed by LC/MS-MS. In total, 1213 and 1092 proteins were quantified from 6 and 9 mM Vc-treated samples, respectively. As expected, quantitative proteomics revealed that DHFR was the top hit in both 6 and 9 mM Vc-treated samples, which produced the most significant stability shift after interacting with MTX (Fig. 2A). Next, we applied this Vc-induced protein precipitation approach to profile the target proteins of the small molecule AMP-PNP, a non-hydrolysable analog of ATP. The 293T cell lysate was incubated with AMP-PNP or ddH2O and then treated with Vc with different concentrations. The western blotting analysis of CDK9, the known target of AMP-PNP, in the supernatant indicated that it was stabilized with the addition of AMP-PNP (Fig. 2B). Because the observed stability shift started from the 3 mM-treated samples based on the immunoblotting readout and the missing values may be generated in the proteomics data of 12 and 15 mM Vc-treated samples where the majority of proteins were almost precipitated, the 3, 6 and 9 mM Vc-treated samples in the vehicle and AMP-PNP groups were selected for quantitative proteomics analysis. The three pairs of supernatant samples, i.e. the samples with and without drug treatment for the three Vc concentrations, were digested, dimethyl labeled, and analyzed by LC-MS/MS. Overall, 2317, 2210 and 1732 proteins were quantified from the three pairs of samples. When the threshold was set as a log2 fold change of 1.5, 7, 7 and 63 AMP-PNP stabilized proteins were identified in the three samples (Fig. S2A†), and a total of 67 AMP-PNP-stabilized proteins were obtained after removing redundantly identified proteins. Most target proteins were identified in the 9 mM Vc treated sample, indicating that more proteins were sensitive at this Vc concentration and thus produced significant stability shifts. To evaluate the reliability of the pHDPP approach, we investigated the percentage of the ATP-binding proteins among the AMP-PNP stabilized proteins. It was found that this percentage in our approach (46.3%) was much higher than that of the 2D-TPP approach (41.8%) (Fig. 2C). Although 23.2% of AMP-PNP stabilized proteins were not annotated as ATP-binding proteins using the DAVID online tool, they were nucleotide and RNA binding proteins. Moreover, we observed that 20.3% non-ATP binding subunits of complexes were also stabilized by AMP-PNP. For instance, some ATP-binding PSMC subunits of 19S regulatory particles from proteasome were stabilized by AMP-PNP, and the non-ATP-binding PSMD subunits also exhibited a stabilized effect (Table S2†). Additionally, we found that the ATP-binding MYH9 subunit was stabilized by AMP-PNP, and non-ATP binding MYL1 and MYL6 subunits of myosin were also simultaneously identified as AMP-PNP-induced stabilized proteins. The stabilization of non-ATP binding MYL1 and MYL6 subunits by AMP-PNP demonstrates that these two proteins are not dissociated from the complex and the stability generated from the ATP-binding subunit was likely to propagate to the nearby non-ATP binding subunits (Fig. 2D) because they present in the same protein complex. This finding was consistent with a previous report,27 which also hints that the stabilizing effect produced by at least one subunit can be propagated to subunits in physical proximity.
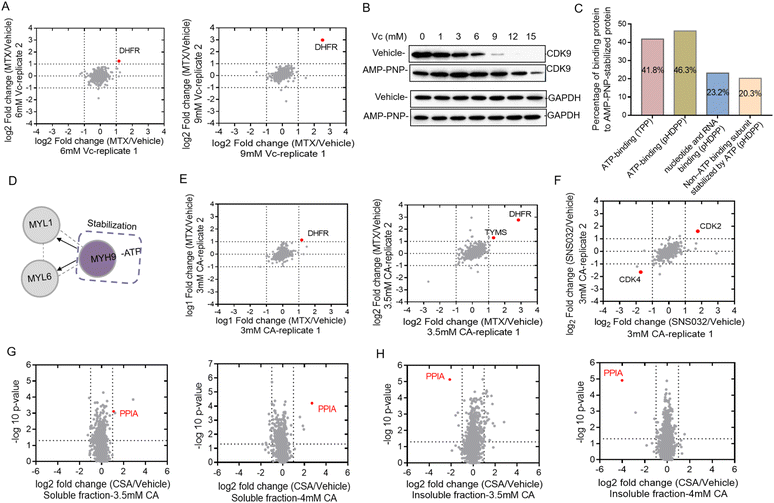 |
| Fig. 2 Vc and CA-based pHDPP approach to identify the targets of small molecules from the total cell lysate by using dimethyl labeling based quantitative proteomics. (A) The known target DHFR of MTX was identified in 6 mM and 9 mM Vc-treated samples. The target hits were obtained by LC-MS/MS data from two replicate runs. (B) Western blotting confirmed that the known target CDK9 was stabilized by AMP-PNP in the 293T cell lysate after treating with the Vc concentration gradient. (C) The distribution of binding proteins of AMP-PNP-induced stabilized targets identified by quantitative proteomics. (D) An example where the stability of the ATP-binding subunit induced by AMP-PNP was propagated to the non-ATP-binding subunit. (E) The known target DHFR of MTX was identified in 3 mM and 3.5 mM CA-treated samples by quantitative proteomics. The target hits were obtained from LC-MS/MS data from two replicate runs. (F) The known target CDK2 of SNS-032 was identified in 3 mM CA-treated samples by quantitative proteomics. The target hits were obtained from LC-MS/MS data from two replicate runs. Quantitative proteomics result confirmed that the known target protein PPIA of CSA was identified in both (G) soluble and (H) precipitate fractions of the Hela cell lysate by the CA-based pHDPP approach. | |
In addition to Vc, CA can also be used to precipitate proteins to probe the ligand binding induced stabilization. Next, we used compounds MTX, kinase inhibitor SNS-032 and cyclosporin A (CSA) to evaluate the ability of the pHDPP approach using CA-based pHDPP, for identifying target proteins from the total cell lysate. To verify the stability shift of DHFR by MTX in the CA treatment experiment, the supernatants in 3 and 3.5 mM CA-treated samples were analyzed by quantitative proteomics. As shown in Fig. 1C, a significant stability shift of DHFR after binding with MTX was observed in 3 and 3.5 mM CA-treated samples. The two pairs of supernatants were analyzed by quantitative proteomics. Totally, 1389 and 1341 proteins were quantified in the samples treated with 3 and 3.5 mM CA, respectively. Similar to the VC treated sample, DHFR was also identified as the target protein for both CA concentrations (Fig. 2E). Moreover, in the 3.5 mM CA-treated sample, it was found that DHFR was identified as the top 1 hit and human thymidylate synthase (TYMS), another known target of MTX, also showed significant stability shift (Fig. 2E).
We further investigated the performance of CA-pHDPP to identify the target proteins of the kinase inhibitor SNS-032. Western blotting analysis indicated that the abundance of CDK9, a known target protein of SNS-032, in the vehicle group decreased more rapidly (Fig. S2B†), which revealed that SNS-032 protected CDK9 from precipitation. As an obvious stability shift was observed, 3.5 mM CA-treated samples in the presence and absence of SNS-032 groups were subjected to dimethyl labeling and LC-MS/MS analysis. Although CDK9 was not identified in the quantitative proteomics data, another known target CDK2 was determined as the top stabilized hit with a significant abundance change (Fig. 2F). Interestingly, CDK4 was identified as the top destabilized protein. These results indicated that both Vc and CA can be used in pHDPP to identify drug target proteins from complex protein samples.
In the above experiments, the supernatants were submitted to quantitative proteomics to identify target proteins. We believe that the counterpart of the supernatant, the precipitate, can also be used to identify target proteins. To prove this, we analyzed the stability shift of protein targets upon binding with cyclosporin A (CSA) in both the supernatant and precipitate of Hela cell lysates treated with 3.5 and 4 mM CA. CSA is a cyclic undecapeptide with an immunosuppressive effect and widely used for allogeneic transplantation. After quantitative proteomics analysis, the known target protein PPIA was both identified in the supernatant (Fig. 2G) and precipitation fractions (Fig. 2H). Interestingly, we also found that the identification of the target protein PPIA in the precipitate showed higher specificity compared to the supernatant, presumably due to the higher proportion of the target protein in the precipitation fraction than that in the supernatant. Therefore, these results demonstrated that the target proteins of a ligand could be dually confirmed by our approach through the simultaneous analysis of the supernatant and precipitation fractions. More importantly, for low abundant proteins, the detection of protein abundance changes in the precipitate may be more sensitive and accurate.
Constructing the target protein space of staurosporine by the TMT labeling strategy
In the majority of cases of the above pHDPP experiments, the known target proteins were identified as the top hits among over 1000 quantified proteins, implying the high specificity of this approach. However, the dimethyl labeling based quantitative proteomics with 1D RPLC-MS/MS analysis can only identify a limited number of proteins, and the single-point sample treated with an acidic agent can only capture the ligand induced stability shift for limited targets. To further increase the target identification coverage, a multiplexed isobaric labeling method (e.g., TMT 10-plex) in conjunction with high pH-low pH 2D RPLC-MS/MS was applied to analyze the ligand induced stability shift treated with 5 CA concentrations. Staurosporine, a pan specific protein kinase inhibitor, was used as a model drug to investigate the performance of the developed approach. The Hela cell lysate was exposed to staurosporine and the vehicle, and then treated with CA concentration gradients of 1.5, 2, 2.5, 3, 3.5, 4 and 4.5 mM, respectively. It can be affirmed from the western blotting results that staurosporine protected the known targets IRSK4 and CDK5 from unfolding (Fig. 3A). The supernatants obtained from the above staurosporine/vehicle-exposed samples were then digested and labeled with the TMT 10-plex agent followed by proteomic analysis.
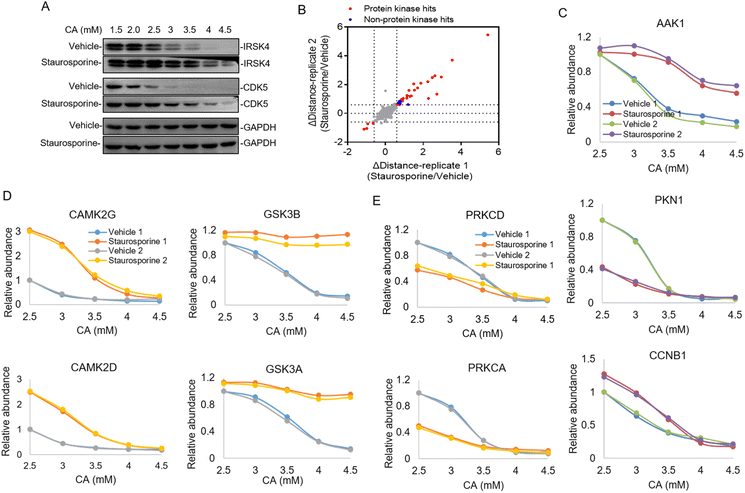 |
| Fig. 3 Identification of the protein kinase of a pan-kinase inhibitor staurosporine by pHDPP in the Hela cell lysate by using the TMT 10-plex strategy. (A) Western blotting confirmed that the known target IRSK4 and CDK5 were stabilized by staurosporine in the Hela cell lysate after treating with the CA concentration gradient. (B) The scatter plot of target proteins with staurosporine-induced stability shift identified by pHDPP in the Hela cell lysate with a Δdistance cutoff of ±0.6. (C) Examples of stability shift curves for AAK1, PKN1 and CCNB1 in the presence or absence of staurosporine in pHDPP. (D) Examples of stability shift curves for two stabilized subunits of CAMK2 and GSK3 protein complexes and (E) two destabilized subunits of the PRKC protein complex induced by staurosporine. | |
Around 3700 proteins were quantified from the above samples across five concentration gradients of CA treatment. Next, Δdistance was used as the measurement of protein stability shifts as in our previous report.28 In total, 36 proteins with significant stability shift were detected when the Δdistance threshold of two replicates was set ±0.6 in this experiment, of which 31 hits were protein kinases and the stability shifts of 5 non-protein kinases were small, indicating high specificity of this approach (Fig. 3B and Table S3†). The stability curves of proteins with significant stability shifts were plotted across a range of CA concentrations using normalized protein abundance in the supernatant fraction. While the majority of protein kinases with stability shifts were stabilized by staurosporine (such as AAK1), 4 protein kinases such as PKN1 displayed destabilization, which may have resulted from indirect binding (Fig. 3C and Table S3†). In addition to protein kinases, it was also found that non-protein kinases such as CCNB1, ADK and PMPCA displayed substantial stability shifts (Fig. 3C and S3†). Some non-protein kinases are identified as targets of staurosporine as observed in previous reports.9,17 The above results highlighted that our pHDPP approach enabled highly specific target identification of small molecules by using the TMT labeling strategy.
Looking specifically into the protein complex of staurosporine-induced targets with apparent stability shifts, we found that the subunits of a complex showed similar melting curve behaviors. Specifically, taking CAMPK2, GSK3 and PRKC complexes as examples, it was obvious that their two subunits exhibited similar melting curves in the staurosporine and vehicle-treated samples (Fig. 3D and E). This is consistent with the recent finding that interacting proteins can lead to similar solubility across different temperatures.29 These similar stability shift curves across acidic agent concentration gradients are presumably due to the coaggregation for subunit pairs of each protein complex upon CA-induced denaturation. As a consequence, our approach may provide an alternative and complementary approach to explore the protein–protein interactome on the proteome scale.
Comparison of pHDPP with TPP and SIP
We then set out to compare the performance of the three approaches, pHDPP, in-house TPP and SIP, for target protein identification. To make parallel comparison of the three approaches, the same drug of staurosporine and the aliquot of the K562 cell lysate were used. After the K562 cell lysate was exposed to staurosporine and DMSO, they were treated with three types of denaturation methods with 5 different strengths. Then the samples were centrifuged and the supernatants containing soluble proteins were digested to tryptic peptides. The 10 peptide samples from each denaturation method were labeled with one set of TMT 10-plex and the pooled samples were fractionated by high-pH reversed-phase chromatography and analyzed by nanoLC-MS/MS.
After proteomic analysis, in total, 5233, 5592 and 5537 proteins were quantified in the pHDPP, in-house TPP and SIP strategies, respectively (Table S4†). Similar numbers of quantified proteins from these three methods indicated equivalent proteomics coverage for analysis. If we arbitrarily used a Δdistance cutoff of 0.6 to screen the target proteins with stability shift, the pHDPP, in-house TPP and SIP approaches identified 49, 51 and 49 stabilized proteins (Fig. 4A and B), indicating similar numbers of potential targets were identified in each method. However, when we looked into the data, pHDPP yielded the maximal number (42) of protein kinases, while in-house TPP and SIP only yield 36 and 34 protein kinases. The percentage of non-protein kinases in pHDPP (14%) was lower than that in in-house TPP (29%) and SIP (31%), and it was comparable with that of Savitski-TPP (15%) (Fig. 4B).17
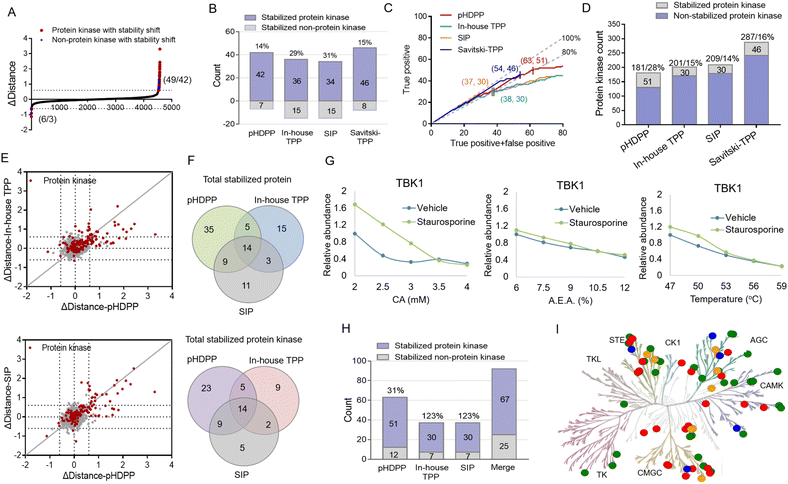 |
| Fig. 4 Comparison of pan-kinase inhibitor staurosporine-stabilized target proteins identified by pHDPP, in-house TPP and SIP approaches in the K562 cell lysate by using the TMT 10-plex strategy. (A) The scatter plot of target proteins with staurosporine-induced stability shift identified by pHDPP in the K562 cell lysate as a Δdistance cutoff of ±0.6. The numbers in parentheses indicate the number of stabilized (or unstabilized) proteins and protein kinases. (B) The number of staurosporine-induced stabilized protein kinases in pHDPP, in-house TPP and SIP datasets using a Δdistance cutoff of 0.6. (C) Number of identified protein kinase targets with the increase in identified overall target proteins by pHDPP (red), in-house TPP (green), SIP (orange) and Savitski-TPP (blue), respectively. The numbers in parentheses indicate the number of stabilized proteins and protein kinases at a specificity of about 80%. We considered the entire protein kinase space as the reference for true positive identification. The gray lines indicated 100% and 80% specificity. (D) Histogram of the number of stabilized protein kinases and totally quantified protein kinases in pHDPP, in-house TPP, SIP and Savitski-TPP approaches. (E) Comparison of the Δdistance value of staurosporine-induced stabilized protein kinases determined by pHDPP with that determined by in-house TPP (left) and SIP (right). (F) Venn diagram of totally stabilized proteins and stabilized protein kinases from pHDPP, in-house TPP and SIP experiments. (G) An example of a stability shift curve where TBK1 was stabilized by staurosporine in pHDPP but not stabilized in TPP and SIP approaches. (H) The number of staurosporine-induced stabilized protein kinases combined by pHDPP, in-house TPP, and SIP datasets was apparently elevated. (I) Kinome map of 67 stabilized protein kinases combined by pHDPP, in-house TPP and SIP approaches in the phylogenetic tree of the human protein kinase family. The green circles represent the overlapped stabilized protein kinase identified by the three approaches; the red, orange and blue circles represent the stabilized protein kinase specifically identified by pHDPP, in-house TPP and SIP approaches, respectively. The kinome tree illustrations were reproduced courtesy of Cell Signaling Technology, Inc. | |
For comparison, the true positive rates (TPRs) were kept at the same level. TPR = (stabilized protein kinase count)/(stabilized non-protein kinase count + stabilized protein kinase count) × 100%. We ranked the protein candidate targets using Δdistance scores and considered the annotated human protein kinases in KinHub (http://www.kinhub.org) as true positives. By using a TPR cutoff of 80%, pHDPP, in-house TPP and SIP yielded 63, 37 and 37 stabilized proteins, of which 51, 30 and 30 were protein kinases (Fig. 4C). Under these conditions, pHDPP yielded much higher target protein identification than in-house TPP and SIP methods. The identification of several top ranked non-protein kinase targets made the TPR in in-house TPP and SIP lower (Fig. 4C and Table S5†). Next, we benchmarked these three datasets against staurosporine targets by Savitski-TPP, where 46 stabilized protein kinases were identified as target hits. Overall, the number of true positive targets found by the pHDPP and Savitski-TPP methods was comparable. However, it was found that the number of identified stabilized protein kinases by pHDPP was still slightly higher even though the total quantified protein kinases were only two third of Savitski-TPP. The percentage of protein kinase hits among all quantified protein kinases for in-house TPP, SIP and Savitski-TPP was comparable (12%, 14% and 16%), while the percentage of protein kinase hits was highest for pHDPP (28%) (Fig. 4D and Table S4†), demonstrating the high sensitivity of pHDPP. The numbers of quantified proteins and identified protein kinase hits in pHDPP, in-house TPP and SIP in this study were smaller than that in Savitski-TPP (Fig. S4†), which is because different workflows were used. In Savitski-TPP, experiments with 10 temperature points were performed to generate melting curves to determine ΔTm, while in this study experiments with only 5 denaturation points were performed to directly determine the distance difference between the two curves.
By direct comparison of the stability shifts of stabilized protein kinases from pHDPP, in-house TPP and SIP experiments (Fig. 4E and S5†), we found that the Δdistance values for most of the stabilized protein kinases in pHDPP were higher than in-house TPP and SIP, which revealed that the acidic agent treatment enabled more differential precipitation of these protein kinases than heating and solvent treatment.
The staurosporine stabilized proteins with the three approaches were further checked to examine the complementarity in the identification of targets. There were 14 overlapped proteins identified by the three approaches, which were all protein kinases, indicating the common specificity of the three approaches. Only a limited number of protein kinases were detected by all three approaches (Fig. 4F), showing that these approaches were highly complementary. By fitting the curves of the protein produced stability shifts in the pHDPP, we found that some protein kinases such as TBK1, VRK1 and RNASEL or non-protein kinases such as CCNB1 and CCNB2 were obviously stabilized only in pHDPP and but not in the other two approaches although these protein kinases were also quantified in these two approaches (Fig. 4G, S6 and Table S5†). Combining the results of the three approaches led to an apparent increase in the total number of identified protein targets. The number of protein kinase targets increased to 67 in total, with an increase of 31%, 123% and 123% of protein kinases in pHDPP, in-house TPP and SIP experiments, respectively (Fig. 4H). As a broad-spectrum kinase inhibitor, these 67 target kinases were distributed across a number of different kinase families (Fig. 4I). It is likely that each approach has its own specifically identified kinase class. Thus, the three approaches may be further tailored to specific compounds or target classes. The above data revealed that these three approaches had the characteristics of good complementarity in target identification. Therefore, some targets that do not respond to a ligand after treating with one of the denaturation conditions may be discovered under the other two denaturation conditions. Clearly, the coverage of identified target proteins can be improved by integrating these three approaches.
The application to identify target proteins of dihydroartemisinin
Dihydroartemisinin (DHA), as the dominant derivative of artemisinin, is widely used for the treatment of malaria.30 Recent studies showed that DHA also has an extraordinary effect on the treatment of various cancers.31–33 However, the anti-tumor mechanisms remain unknown; especially the DHA targeted proteins were little investigated. In this study, we applied pHDPP to screen the potential target proteins of DHA. Five concentration points of CA (2, 2.5, 3, 4 and 5 mM) were employed to induce the protein precipitation in Hela cell lysates treated with DHA and DMSO, and equal volumes of soluble fractions were digested and labeled with the TMT 10-plex reagent. The pooled sample was fractionated into 15 fractions by high pH RPLC prior to LC-MS/MS analysis, which enabled the quantification of more than 4200 proteins after stringent filtering (Fig. 5A). After the data were normalized, we scored the stability shift (Δdistance) by comparing the relative intensity of each protein between DMSO and DHA-treated samples. For the real target protein discovery study of a ligand, it's very difficult to determine the threshold of Δdistance to filter the data because there are no sufficient true positive targets to estimate the TPR. Instead, we consider only a very small number of top stabilized proteins as potential targets. In this study, only about 1% of all quantified proteins with Δdistance > 0.55, i.e., a total of 45 proteins, are considered as potential target proteins of DHA (Fig. 5A and Table S6†).
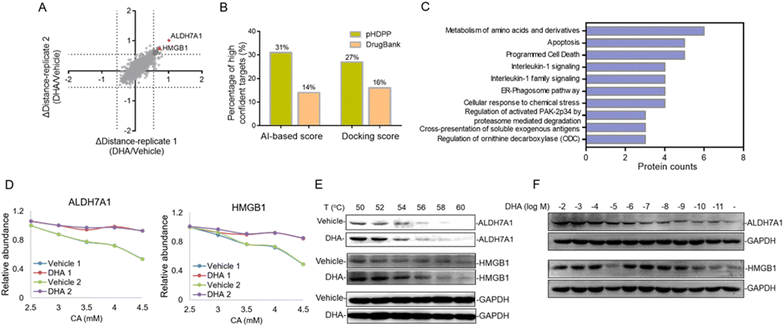 |
| Fig. 5 Identification of the novel target proteins of DHA in Hela cell lysates by pHDPP. (A) Quantitative proteomics readout revealed 45 DHA-induced stabilized potential target proteins. (B) Percentage of high-confidence candidate targets identified by pHDPP and in DrugBank based on AI and docking score rankings. (C) Pathways analysis implied that these candidate target proteins mainly involved the metabolism of amino acids and derivatives and apoptosis signaling pathways. (D) Examples of stability shift curves for ALDH7A1 and HMBG1. (E) Western blotting confirming the stabilization of ALDH7A1 and HMBG1 by DHA in Hela cell lysates by using CETSA. (F) The isothermal dose–response experiment at 56 °C showing the target engagement for ALDH7A1 and HMBG1 in Hela cell lysates. | |
There are 80 proteins annotated as potential targets of DHA in DrugBank. Most of them were derived from a probe-based study.34 Combining the 45 potential targets identified in this study, totally 122 (three targets, ALDH7A1, SF1, and TAGLN, present in both sources) potential targets of DHA in human were obtained. All of these targets were assessed by different simulation methods, including molecular docking and artificial intelligence (AI)-based target prediction by the MolDesigner package (Table S7†). We considered the proteins in the top 20% of AI-based score or docking score to be high-confidence targets. It was found that 31% (AI-based score) or 27% (docking score) of the candidate targets obtained by pHDPP were highly confident, while only 14% (AI-based score) or 16% (docking score) of the candidate targets obtained from DrugBank were highly confident (Fig. 5B and Table S7†). The limited overlapped proteins identified by these two approaches and the low confidence of targets in DrugBank may be because the dihydroartemisinin was biotinylated, which may alter the specificity of small molecules. Moreover, some structural proteins such as ribosomal proteins were identified in the probe-based study, which are likely because of non-specific adsorption. The above results demonstrated that the targets identified by our pHDPP were more confident.
Among the 45 potential targets identified by pHDPP in this work, ALDH7A1 (aldehyde dehydrogenase 7 family member A1) and HMGB1 (high mobility group box 1) proteins ranked within the top 5 according to Δdistance (Fig. 5A and Table S6†). Interestingly, they belonged to the top-ranking functional cluster, which is involved in metabolism and apoptosis pathways according to pathway analysis for all identified target proteins (Fig. 5C). Although ALDH7A1 was also identified by the probe-based approach,34 it was not verified. We fitted the stability curves of these two proteins and found that they had obvious stability shifts in the presence of DHA (Fig. 5D). Furthermore, in order to verify the reliability of the identified candidate targets, we profiled the interaction between ALDH7A1, HMGB1 and DHA by the CETSA approach,16 which is based on ligand-induced changes in protein thermal stability by western blotting readout. It was found that ALDH7A1 and HMGB1 had higher abundance in the DHA treated sample vs. the DMSO treated sample at multiple temperature points (Fig. 5E), indicating that these proteins are resistant to thermal denaturation after exposing to DHA. To determine the affinity of DHA for the candidate targets ALDH7A1 and HMGB1, we performed an isothermal dose–response experiment. Dose-dependent stabilization of ALDH7A1 and HMGB1 was confirmed in the presence of DHA (Fig. 5F). CETSA results for DHA and HMGB1 showed stabilization at low nM concentrations, which revealed that the affinity of DHA and HMGB1 was higher than that between DHA and ALDH7A1 (Fig. 5F). We hence hypothesize that DHA is likely directly binding with ALDH7A1 and HMGB1 proteins.
Additionally, ALDH7A1 and HGMB1 performed well in docking and AI-based target prediction, especially ALDH7A1, which was ranked at the second place in AI prediction results and the sixth place in molecular docking (Table S7†). From the docking result, we found that DHA could bind in the active pocket of ALDH7A1, to block the narrow passage to the active residue Cys330 with the rigid structure of DHA (Fig. 6A). Moreover, the hydrogen bonds between Thr273, Ser275 and DHA would make the interaction much more stable (Fig. 6A). For HMGB1 protein, the AI predicted it as the twentieth in total 72 cancer-related targets and the docking result of HMGB1 showed that the compound would have some interference for the interaction of DNA–protein (Table S7†). The hydrophobic main part of DHA could fit into a small cavity of the HMGB1 DNA binding pocket formed by Phe102, Phe103, Ala126, and Leu129, and also the hydrogen bond between DHA and the Ser107 main chain would be very beneficial for the interaction (Fig. 6B). Collectively, ALDH7A1 and HMGB1 were identified by the low pH denaturing method and confirmed by the thermal denaturing method, molecular docking and artificial intelligence (AI)-based target prediction methods, indicating that they are highly confident target proteins of DHA. Except for these two targets, there are some other targets that could be treated as the potential targets for DHA from the simulation results, such as PDE6D, which had the best score in molecular docking. The natural bending of the DHA three-dimensional structure could overlay with the main part of PDE6D known inhibitors quite well, which would be the basic of the interaction between DHA and PDE6D. Otherwise, the hydrogen bonds between DHA and several key residues, including Trp32, Arg61, and Thr131, could also benefit the interaction (Fig. 6C).
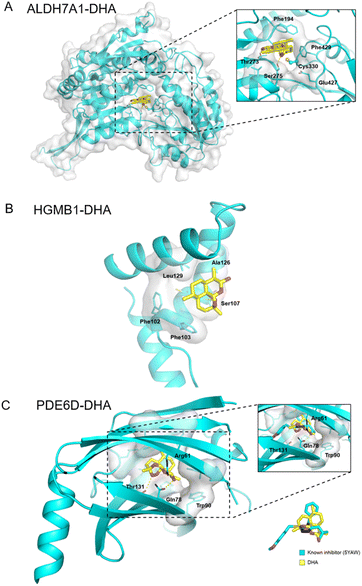 |
| Fig. 6 Docking pose for the DHA within the active site of (A) ALDH7A1, (B) HMGB1 and (C) PDE6D. | |
There is some evidence to show that DHA is effective in cancer therapy.33,35 ALDH7A1 was one of the hits related to the amino acid metabolism pathway. Amino acids in mammalian cells are substrates for new protein synthesis, which is necessary for the cell proliferation within tumor.36,37 The metabolism of amino acids perturbed by DHA may result in the failure of cancer cells to take in enough energy to sustain their proliferative drive. Furthermore, the protein hits including HMGB1, HMGB2, PSMB3, PSMB4 and PSMB7 (Fig. 5D and S7†) are related to the apoptosis pathway, suggesting that DHA's anticancer roles may be partly through inducing programmed cell death in tumor cells. This result was consistent with increasing evidence showing that DHA was able to induce apoptosis towards various cancer types.31,32,38 Collectively, the inhibition or alleviation of tumor development by DHA may be due to the multi-target effects. However, further biological functional verification should be implemented to ultimately unveil the exact mechanism of action by DHA.
Conclusion
In conclusion, we have shown that the pHDPP approach is a powerful tool to probe ligand-induced stability shifts. It enables proteome-wide target identification without chemical modification of drugs. The simplified workflow and data processing can largely reduce the cost and save time. Our approach can be extended to the dose-dependent response assay, which allows the determination of the affinity of a drug to its target proteins directly from cell extracts by quantitative proteomics approaches. pHDPP was also demonstrated to identify targets that cannot be achieved by TPP and SIP. However, the use of an acid may shift the acid–base equilibrium for some small-molecule drugs, which may modulate their interactions with target proteins. Additional experiments are needed to investigate if this method is equally effective in target protein discovery for these drugs. pHDPP was finally applied to reveal the target space of dihydroartemisinin, a dominant derivative of artemisinin, and 45 potential target proteins were identified. Pathway analysis indicated that these target proteins are mainly involved in metabolism and apoptosis pathways. Two cancer-related target proteins, ALDH7A1 and HMGB1, were validated by structural simulation and AI-based target prediction methods. And they were further validated to have strong affinity with DHA by using CETSA.
Experimental section
Reagents and cell culture
Dimethyl sulfoxide (DMSO), Nonidet P-40 (NP-40), protease inhibitor cocktail, formic acid (FA), tris(2-carboxyethyl)phosphine (TCEP), 2-chloroacetamide (CAA) and trypsin (bovine, TPCK-treated) were purchased from Sigma Aldrich (St. Louis, MO, USA). Methotrexate (MTX) was purchased from Sigma Aldrich, AMP-PNP, SNS-032, cyclosporine A (CSA), staurosporine and dihydroartemisinin (DHA) were purchased from Selleck (Houston, TX), and phosphate-buffered saline (PBS, pH 7.4, 1×) was purchased from Gibco (Gaithersburg, MD). Acetonitrile and methanol (HPLC grade) were from Merck (Darmstadt, Germany).
HeLa and 293T cells were grown in a RPMI 1640 medium (Gibco, Rockville, MD), and K562 cells were cultured in an IMDM medium (Gibco, Rockville, MD). Both media contained 10% fetal bovine serum (FBS) (Gibco, NY) and 1% streptomycin (Beyond, Haimen, China) and all cells were cultured under the conditions of 37 °C and 5% CO2.
Cell extract
Cells were harvested and washed with cold PBS three times. Subsequently, the cells were lysed using PBS (pH 7.4, supplemented with 1% EDTA-free cocktail) by three freeze–thaw cycles in liquid nitrogen. The lysis buffer was snap-frozen followed by thawing at 30 °C using a water bath until about 60% of the suspension was thawed, and then they were transferred onto ice until the entire content was thawed. Cell debris was then removed by centrifuging at 20
000g for 10 min at 4 °C and the protein concentration was determined by using a BCA protein assay kit (Thermo Fisher Scientific, San Jose, CA, USA).
Acidic agent-induced denaturation of the K562 proteome
The K562 cell protein extract was divided into 14 aliquots of 50 μL and then treated them with increasing concentrations of different acidic agents (Vc, CA, HCl and FA). Subsequently, the mixtures were equilibrated in parallel at 800 rpm for 20 min at 37 °C by using a heating and cooling shaker (Benchmark, China). Supernatants were collected after the mixtures were centrifuged at 20
000g for 10 min at 4 °C. The protein concentration in the supernatant was measured by SDS–PAGE and visualized with Coomassie staining.
pH measurement for different concentrations of acidic agents
Different concentrations of Vc (1, 3, 6, 9, 12, 15, 17 and 20 mM), CA (1, 2, 3, 4, 5 and 6 mM), FA (1, 3, 6, 9 and 12 mM) and HCl (1, 3, 6, 9 and 12 mM) were dissolved in 10 mM PBS and the corresponding pH was measured by using a pH meter (Mettler-Toledo, Switzerland).
Preparation of cell extracts for stability profiling
Cell lysates of different cell lines were prepared as described in the above cell extract section. The initial concentration of the protein extract was generally in the range of 2–5 μg μL−1. The cell line 293T for MTX, SNS-032 and AMP-PNP experiments; HeLa for CSA and DHA experiments; K562 were used for the staurosporine experiment.
Screening of the acidic agents for pHDPP
Acidic agents including Vc, CA, HCl and FA were used for drug MTX target identification. The supernatant of the 293T cell extract was split into two aliquots, a solution of compounds in buffer (MTX in DMSO) was added to one aliquot and the an equivalent amount of DMSO alone as the vehicle was added to the other aliquot. The final concentration of MTX was 100 μM. After the extract was exposed for 20 min to room temperature using a rotameter at a normal rotating speed, it was divided into 7 aliquots of 50 μL in new 600 μL tubes. Vc, CA, HCl and FA to the indicated concentrations (Vc: 1, 3, 6, 9, 11, 13 and 15 mM; CA: 1, 2, 3, 3.5, 4, 4.5 and 5 mM; HCl: 1, 5, 6, 7, 8, 8.5 and 9 mM; FA: 1, 2, 4, 6, 8, 10 and 12 mM) were added to each of the compound and vehicle-containing samples. Subsequently, the mixtures were equilibrated in parallel at 800 rpm for 20 min at 37 °C. Supernatants were collected after the mixtures were centrifuged at 20
000g for 10 min at 4 °C. The supernatants were subjected to western blotting analysis.
Western blotting
The soluble proteins in supernatants were separated by means of SDS–PAGE and were transferred onto a polyvinylidene difluoride (PVDF) membrane. The membrane was blocked with 5% skim milk. Primary rabbit anti-DHFR, anti-CDK9 (subways, China), rabbit anti-IRSK4, rabbit anti-CDK5, rabbit anti-ALDH7A1, rabbit anti-HMGB1 (Proteintech, Chicago, IL) and rabbit anti-GAPDH (Abcam, Cambridge, UK) antibodies, and secondary goat anti-rabbit HRP-IgG antibodies (Abcam, Cambridge, UK) were used for immunoblotting. The above antibodies were used according to the manufacturer's instructions. The chemiluminescence intensities were visualized and quantified by using an ECL detection kit (Thermo Fisher Scientific, USA) and the images were obtained by using a Fusion FX7 imaging system (Vilber Infinity, France).
Affinity evaluation with dose-dependence response assay
The affinity of drug–target interaction was determined by dose-dependence response assay. The drug solutions were prepared by ten-fold dilution of MTX starting at 10 mM. The 293T cell lysates were incubated with a range of MTX concentrations including a vehicle control (the resulting final concentration started from 100 μM), and then these mixtures were treated with acidic agent of a single concentration (Vc: 12 and 15 mM, CA: 4 and 5 mM). Subsequently, the mixtures were equilibrated in parallel at 800 rpm for 20 min at 37 °C by using a heating and cooling shaker (Benchmark, China). Supernatants were collected after the mixtures were centrifuged at 20
000g for 10 min at 4 °C. The soluble fraction abundance was measured by western blotting and the band intensity was plotted with Graphpad Prism software.
Sample preparation for Vc-induced stability profiling
For acidic agent Vc-treated AMP-PNP experiments, the protein extraction was handled as described in detail above in the preparation of cell extracts for the stability profiling section. Briefly, protein extraction was carried out by three freeze–thaw cycles in liquid nitrogen. After centrifugation, the resulting supernatant was divided into two aliquots, a solution of compound in buffer (AMP-PNP in ddH2O) was added to one aliquot and an equivalent amount of ddH2O alone as the vehicle was added to the other aliquot. The final concentration of AMP-PNP was 2 mM. After the extract was incubated for 20 min at room temperature using a rotameter at a normal rotating speed, it was divided into 7 aliquots of 50 μL in new 600 μL tubes. Indicated Vc concentration gradients (0, 1, 3, 6, 9, 12 and 15 mM) were added to each of the compound and the vehicle-containing samples. Subsequently, the mixtures were equilibrated in parallel at 800 rpm for 20 min at 37 °C by using a heating and cooling shaker. Supernatants were collected after the mixtures were centrifuged at 20
000g for 10 min at 4 °C. The supernatants were subjected to western blotting analysis and sample preparation for MS analysis.
Sample preparation for CA-induced stability profiling
For acidic agent CA-treated SNS-032, CSA, staurosporine and DHA experiments, a solution of compounds in DMSO (the final concentrations of SNS-032, CSA, staurosporine and DHA were 100 μM, 100 μM, 20 μM and 100 μM, respectively) or an equivalent amount of DMSO alone was added to the resulting soluble fraction. After the extract was exposed for 20 min to room temperature using a rotameter at a normal rotating speed, the extract was divided into several aliquots of 50 μL. Subsequently, indicated CA concentration gradients were added to the respective cell extracts (SNS-032: 1, 2, 3, 3.5, 4, 4.5 and 5 mM; CSA: 3.5 and 4 mM; staurosporine: 1.5, 2, 2.5, 3, 3.5, 4 and 4.5 mM in Hela cell lysates; staurosporine: 2, 2.5, 3, 3.5 and 4 mM in K562 cell lysates; DHA: 2.5, 3, 3.5, 4 and 4.5 mM). The mixtures were equilibrated in parallel at 800 rpm for 20 min at 37 °C. Supernatants were collected after the mixtures were centrifuged at 20
000g for 10 min at 4 °C. The supernatants were subjected to western blotting analysis and preparation for MS analysis.
For the CSA experiment, in addition to the supernatant used for the stability profiling, the precipitate was also collected for stability profiling by mass spectrometry. The precipitate was washed twice with PBS at 20
000g for 10 min at 4 °C and prepared for MS analysis.
Sample preparation for thermal proteome profiling (TPP)
The K562 cell lysate was divided into two aliquots, of which one aliquot was treated with 20 μM staurosporine and the other aliquot was treated with an equivalent amount of DMSO at room temperature for 20 min. The respective lysates were divided into 5 aliquots (50 μL each), and then heated individually at different temperatures (47, 50, 53, 56 and 59 °C) for 3 min using a thermal cycler (Biorad, USA), followed by cooling at room temperature for 3 min. The heated lysates were subjected to centrifugation at 20
000g for 20 min at 4 °C to separate soluble proteins from precipitated proteins. The soluble fractions of each aliquot were subjected to isobaric labeling and quantitative proteomics analysis.
Sample preparation for solvent-induced protein precipitation profiling (SIP)
The K562 cell lysate was divided into two aliquots, of which one aliquot was treated with 20 μM staurosporine and the other aliquot was treated with an equivalent amount of DMSO at room temperature for 20 min. The respective lysates were divided into 5 aliquots (50 μL each), and indicated solvent mixture A.E.A (acetone
:
ethanol
:
acetic acid with a ratio of 50
:
50
:
0.1) percentage gradients (6, 7.5, 9, 10.5 and 12%) were added to each of the staurosporine and vehicle-containing samples. Subsequently, the mixtures were equilibrated in parallel at 800 rpm for 20 min at 37 °C. Supernatants were collected after the mixtures were centrifuged at 20
000g for 10 min at 4 °C. The supernatants were subjected to western blotting analysis and sample preparation for MS analysis.
Sample preparation for MS
Firstly, equal volumes of supernatants in the vehicle group and drug treated group were denatured with 8 M guanidine in 50 mM HEPES (pH 8.0) and the precipitates were reconstituted with 6 M guanidine in 50 mM HEPES (pH 8.0). The disulfide bonds of the proteins were then reduced and alkylated by the addition of 10 mM TCEP and 40 mM CAA at 95 °C for 5 min. The solution of samples was replaced by using a 10 kDa ultrafiltration tube (Sartorius AG, Germany) under the conditions of 14
000g at 4 °C. Subsequently, the protein samples were washed two times with 50 mM HEPES (pH 8.0) and then trypsin was added to the samples at a ratio of 1
:
20 (enzyme/protein, w/w) for digestion at 37 °C for 16–20 h.
For Vc-treated MTX and AMP-PNP experiments, as well as CA-treated MTX, SNS-032 and CSA experiments, the resultant digested peptides were subjected to dimethyl labeling. Briefly, the peptides in the vehicle group were labeled with 4% CH2O and 0.6 M CH3BNNa as light labeling, and the peptides in the ligand group were labeled with 4% CD2O and 0.6 M CH3BNNa as heavy labeling. After the reaction was performed for 1 h at 25 °C, ammonia and pure formic acid were added to terminate the reaction. Subsequently, the two differentially labeled digests at the same acidic concentration were mixed and then subjected to desalting with C18 solid-phase extraction (Waters, Milford, MA) according to the manufacturer's protocol. Finally, the desalted samples were lyophilized in a SpeedVac (Thermo Fisher Scientific, San Jose, CA, USA) and stored at −80 °C before use.
For CA-treated staurosporine and DHA experiments, and staurosporine experiments by TPP and SIP, the digested peptides in 5 CA concentration points of vehicle and experiment groups were labeled with 10-plex TMT (Thermo Fisher Scientific, Waltham, MA) reagents. The resultant 10 labeled peptide extracts of vehicle and experiment groups were combined to a single sample per experiment. The labeling process was performed according to the manufacturer's instructions. Additional fractionation was performed by using reversed-phase chromatography at pH 9 [1 mm XSelect CSH C18 column (Waters, Milford, MA)]. The samples of staurosporine experiments prepared by pHPDD, TPP and SIP in the K562 cell lysate were fractioned into 12 fractions by high-pH reversed phase chromatography. The sample of the DHA experiment in the Hela cell lysate was fractioned into 15 fractions.
LC-MS/MS analysis
The samples were dried in vacuo and resuspended in 0.1% formic acid in water. The analysis of tryptic peptides was performed on an Ultimate 3000 RSLCnano system coupled with a Q-Exactive-HF mass spectrometer, controlled by Xcalibur software v2.1.0 (Thermo Fisher Scientific, Waltham, MA, USA). Briefly, 1 μg of the re-suspended peptides was automatically loaded onto a C18 trap column (200 m i.d) at a flow rate of 5 μL min−1. A capillary analytical column (150 μm i.d.) was packed in-house with 1.9 μm C18 ReproSil particles (Dr Maisch GmbH). The mobile phases consisted of 0.1% formic acid (A) and 0.1% formic acid and 80% ACN (B).
For the dimethyl labeled samples, peptides were separated through a gradient of up to 90% buffer B over 120 min at a flow rate of 600 nL min−1. The gradient of the mobile phase started from 9% B to 13% B for 1 min and then was increased linearly to 27% B at 80 min, to 45% at 97 min, and then to 90% B at 98 min and maintained for 10 min and finally equilibrated with mobile phase A for 12 min. The LC-MS/MS system was operated in data-dependent MS/MS acquisition mode. The full mass scan acquired in the Orbitrap mass analyzer was from m/z 350 to 1750 with a resolution of 60
000 (m/z 200). The MS/MS scans were also acquired by using an Orbitrap with a 15
000 resolution (m/z 200), and the AGC target was set to 5 × 104. The spray voltage and the temperature of the ion transfer capillary were set to 2.6 KV and 275 °C, respectively. The normalized collision energy for HCD and dynamic exclusion was set as 27% and 20 s, respectively.
For the TMT-labeled samples, each fraction was run with a 90 minute gradient. The flow rate was set as 600 nL min−1. The gradient of the mobile phase was developed as follows: 12–30% B for 60 min; 30–45% B for 12 min; 45–90% B for 1 min; 90% B was maintained for 8 min; then decreased linearly to 2% B at 82 min and maintained for 8 min for equilibration. Mass spectrometry adopts the full scan/data dependent (full MS/dd-MS2) scan mode. The first-level mass spectrometer parameters were set: the scan range was m/z 350–1750 and the resolution was set to 60
000 (m/z 200). Secondary mass spectrometer parameter settings: the resolution was 30
000 (m/z 200) and the automatic gain control target (AGC) was set to 2 × 105. The normalized collision energy for HCD and dynamic exclusion was set as 35% and 30 s, respectively.
Protein identification and quantification
Raw files were processed with MaxQuant (version 1.6.1.10.). The MS/MS spectra were searched against the Uniprot human database with the Andromeda search engine in MaxQuant. Carbamidomethylated cysteine was searched as a fixed modification, whereas methionine oxidation and N-terminal protein acetylation were searched as variable modifications.
For the dimethyl labeling experiment, multiplicity was set to 2 with dimethLys0 and dimethNter0 lightly labelled, whereas dimethLys4 and dimethNter4 were heavily labelled. Trypsin was set as the proteolytic enzyme and up to two missed cleavages were allowed. Precursor and fragment mass tolerances were set at 10 ppm and 0.02 Da, respectively. The false discovery rate (FDR) for both proteins and peptides were accepted at 0.01. Moreover, the options of re-quantify and match between runs were required. Normalized H/L ratios were used for the subsequent statistical analysis. For the TMT-labeled experiment, quantification by reporter ions (TMT 10-plex) was selected for quantification. Trypsin was specified as proteases. Both peptides and proteins were filtered with a FDR of 0.01.
Data processing
The data exported from MaxQuant were analyzed using excel software. For the dimethyl labeling data, the normalized H/L ratio was presented as log2 fold change (FC) to generate a scatter plot based on LC-MS/MS data from two replicate runs. The proteins that were identified in both replicate runs were used for the subsequent analysis. The maximum average value was kept as the final log2
FC(H/L normalization ratio) if the protein target was identified in different samples. The proteins with average log2
FC(H/L normalization ratio) > 1 in different samples of the same set of experiment were combined and considered as the total potential protein targets. The scatter plots were obtained by using Graphpad Prism 5 software (Graphpad Software, Inc, La Jolla, CA).
For the CSA experiment, the stability shift data statistical analysis was according to our previous work.39 Briefly, the code was written to automatically process the peptide output file from MaxQuant, to perform normalization, to calculate P values and ratios of protein abundance, and to identify proteins with significant stability shifts. The intensity of each peptide was normalized by the median value of the ratio of peptides in vehicle-treated and ligand-treated groups. The normalized intensities were then used for the calculation of peptide ratios between the two groups. Subsequently, the peptides with the median ratio and the two peptides closest to the median ratio, a total of three peptides among all peptides from each protein, were selected and their corresponding reporter intensities in the vehicle and CSA-treated samples were used for protein P value calculation by Student's t-test. Protein ratios were determined using the median of all peptide-feature ratios for each protein. The proteins with P value < 0.05 and abundance fold change > 2 were considered as the direct target of CSA.
For the TMT-labeled quantitative data, the reporter ion intensities acquired from vehicle-treated and drug-treated samples across 5 denatured points were normalized by the ratio of the median value of proteins in vehicle-treated and drug-treated groups. The reporter ion intensities of the 10 datasets were further normalized by the reporter ion intensities of the minimum denaturation point in the vehicle-treated group and then were used to fit stability shift curves. If the normalized abundance of the latter denatured point is higher than that of previous points with increasing acid agent concentration, which will affect the distance calculation, we consider that this protein is false positive. Taking this into account, we used the following data processing way for filtering the candidate targets. Among the 5 denaturation points, the normalized abundance of the latter point was not higher than 0.08 of any previous point whether in the vehicle or in the drug group. The smaller the difference, the stricter the filtering criteria for target identification. The distance of an individual protein at each denatured point was calculated, which was based on the simplification and improvement of the Euclidean distance,29 that is, the normalized reporter ion intensities of the 5 denatured points of the drug group minus that of the vehicle group. Ultimately, the distance at each denatured point was added (Δdistance).
The DAVID online tool was used for functional enrichment analysis of all protein target hits for Gene Ontology (GO) in the AMP-PNP experiment. The Reactome online tool was used for pathway analysis of protein target hits in the DHA experiment.
Simulation methods
Several online databases, including TCMSP, DrugBank, David, and STRING were taken to carry out the network pharmacology study. The three-dimensional structures of total 122 potential targets were obtained from the Protein Data Bank (PDB) or AlphaFold Protein Structure Database (Table S8†), and the coordinates of proteins and essential metal ions only were reserved. All of the structures were obtained by the prepared receptor program from the AutoDockTools suite, and a 60 × 60 × 60 nm3 docking box was set with the center of Top-1 pocket predicted by CavityPlus software. The virtual screening for all targets of DHA was performed by using the high accuracy docking program FIPSDock combined with the iterative anisotropic network model (iterANM)-based docking approach, which would be a significant benefit for increasing the prediction accuracy. In addition, the AI-based target prediction tool MolDesigner package and Daylight-AAC model would verify the potential targets from another perspective. Based on the simulation results from three different angles and also the prediction result from pHDPP, it could identify the potential targets of DHA with higher accuracy.
Validation of novel target proteins of DHA
The HeLa cell lysate was treated with 100 μM DHA or an equivalent volume of DMSO at room temperature for 20 min. The respective lysates were divided into 5 aliquots (50 μL each), and then heated individually at different temperatures (50, 52, 54, 56, 58 and 60 °C) for 3 min using a thermal cycler, followed by cooling at room temperature for 3 min. The heated lysates were subjected to centrifugation at 20
000g for 20 min at 4 °C to separate soluble proteins from precipitated proteins. The soluble fractions of each aliquot were subjected to western blotting.
For the validation of the novel protein target ALDH7A1 and HMGB1 of DHA in dose-dependence response assay, the final concentration of DHA started at 100 μM and gradually diluted by ten-fold (10−2, 10−3, 10−4, 10−5, 10−6, 10−7, 10−8, 10−9, 10−10, and 10−11 M). The Hela cell lysate incubated with a series of DHA concentration gradients was heated at 56 °C, followed by the procedure outlined above for the experiment on dose-dependence response. The abundances of soluble targets were detected by western blotting.
Data availability
The MS raw data have been deposited with the ProteomeXchange Consortium (http://proteomecentral.proteomexchange.org) via the jPOST partner repository (https://jpostdb.org) with the data set identifier PXD036499.
Author contributions
Xiaolei Zhang: conceptualization, data curation, formal analysis, investigation and writing of the original draft. Keyun Wang: investigation and formal analysis. Sijin Wu: validation, formal analysis and writing of the original draft. Chengfei Ruan: formal analysis. Kejia Li: investigation. Yan Wang: investigation. He Zhu: formal analysis. Xiaoyan Liu: investigation. Zhen Liu: resources. Guohui Li: formal analysis and supervision. Lianghai Hu: conceptualization, supervision and writing – review & editing. Mingliang Ye: conceptualization, writing – review & editing, funding acquisition, supervision and project administration.
Conflicts of interest
There are no conflicts to declare.
Acknowledgements
This work was supported, in part, by funds from the China State Key Basic Research Program Grants (2021YFA1302601 and 2020YFE0202200), the National Natural Science Foundation of China (92153302, 22137002 and 22207107) and the Innovation Program (DICP I201935 and DICP I202139) of Science and Research from the DICP, CAS.
Notes and references
- M. J. Keiser, V. Setola, J. J. Irwin, C. Laggner, A. I. Abbas, S. J. Hufeisen, N. H. Jensen, M. B. Kuijer, R. C. Matos, T. B. Tran, R. Whaley, R. A. Glennon, J. Hert, K. L. Thomas, D. D. Edwards, B. K. Shoichet and B. L. Roth, Nature, 2009, 462, 175–181 CrossRef CAS PubMed.
- A. Broer, S. Fairweather and S. Broer, Front. Pharmacol., 2018, 9, 785–795 CrossRef PubMed.
- A. P. M. Guedes, F. Mello-Andrade, W. C. Pires, M. A. M. de Sousa, P. F. F. da Silva, M. S. de Camargo, H. Gemeiner, M. A. Amauri, C. Gomes Cardoso, P. R. de Melo Reis, E. P. Silveira-Lacerda and A. A. Batista, Metallomics, 2020, 12, 547–561 CrossRef CAS PubMed.
- F. B. Reinhard, D. Eberhard, T. Werner, H. Franken, D. Childs, C. Doce, M. F. Savitski, W. Huber, M. Bantscheff, M. M. Savitski and G. Drewes, Nat. Methods, 2015, 12, 1129–1131 CrossRef CAS PubMed.
- A. Mateus, J. Bobonis, N. Kurzawa, F. Stein, D. Helm, J. Hevler, A. Typas and M. M. Savitski, Mol. Syst. Biol., 2018, 14, 8242–8256 CrossRef PubMed.
- H. Park, J. Ha, J. Y. Koo, J. Park and S. B. Park, Chem. Sci., 2017, 8, 1127–1133 RSC.
- J. Kreuzer, N. C. Bach, D. Forler and S. A. Sieber, Chem. Sci., 2014, 6, 237–245 RSC.
- J. W. Lyu, K. Y. Wang and M. L. Ye, TrAC, Trends Anal. Chem., 2020, 124, 115574–115585 CrossRef CAS.
- K. A. Ball, K. J. Webb, S. J. Coleman, K. A. Cozzolino, J. Jacobsen, K. R. Jones, M. H. B. Stowell and W. M. Old, Commun. Biol., 2020, 3, 75–84 CrossRef CAS PubMed.
- I. Piazza, N. Beaton, R. Bruderer, T. Knobloch, C. Barbisan, L. Chandat, A. Sudau, I. Siepe, O. Rinner, N. de Souza, P. Picotti and L. Reiter, Nat. Commun., 2020, 11, 4200–4212 CrossRef PubMed.
- E. C. Strickland, M. A. Geer, D. T. Tran, J. Adhikari, G. M. West, P. D. DeArmond, Y. Xu and M. C. Fitzgerald, Nat. Protoc., 2013, 8, 148–161 CrossRef CAS PubMed.
- X. Zhang, Q. Wang, Y. Li, C. Ruan, S. Wang, L. Hu and M. Ye, Anal. Chem., 2020, 92, 1363–1371 CrossRef CAS PubMed.
- J. Lyu, Y. Wang, C. Ruan, X. Zhang, K. Li and M. Ye, Anal. Chim. Acta, 2021, 1168, 338612–338619 CrossRef CAS PubMed.
- I. Piazza, K. Kochanowski, V. Cappelletti, T. Fuhrer, E. Noor, U. Sauer and P. Picotti, Cell, 2018, 172, 358–372 CrossRef CAS PubMed.
- K. V. Huber, K. M. Olek, A. C. Muller, C. S. Tan, K. L. Bennett, J. Colinge and G. Superti-Furga, Nat. Methods, 2015, 12, 1055–1057 CrossRef CAS PubMed.
- D. Martinez Molina, R. Jafari, M. Ignatushchenko, T. Seki, E. A. Larsson, C. Dan, L. Sreekumar, Y. Cao and P. Nordlund, Science, 2013, 341, 84–87 CrossRef PubMed.
- M. M. Savitski, F. B. M. Reinhard, H. Franken, T. Werner, M. F. Savitski, D. Eberhard, D. M. Molina, R. Jafari, R. B. Dovega, S. Klaeger, B. Kuster, P. Nordlund, M. Bantscheff and G. Drewes, Science, 2014, 346, 55 CrossRef CAS PubMed.
- K. Y. Lu, B. Quan, K. Sylvester, T. Srivastava, M. C. Fitzgerald and E. R. Derbyshire, Proc. Natl. Acad. Sci. U. S. A., 2020, 117, 5810–5817 CrossRef CAS PubMed.
- I. Becher, T. Werner, C. Doce, E. A. Zaal, I. Togel, C. A. Khan, A. Rueger, M. Muelbaier, E. Salzer, C. R. Berkers, P. F. Fitzpatrick, M. Bantscheff and M. M. Savitski, Nat. Chem. Biol., 2016, 12, 908–910 CrossRef CAS PubMed.
- Z. Yu, J. Gao, X. Zhang, Y. Peng, W. Wei, J. Xu, Z. Li, C. Wang, M. Zhou, X. Tian, L. Feng, X. Huo, M. Liu, M. Ye, D. A. Guo and X. Ma, Signal Transduction Targeted Ther., 2022, 7, 1–13 CrossRef PubMed.
- J. G. Van Vranken, J. Li, D. C. Mitchell, J. Navarrete-Perea and S. P. Gygi, Elife, 2021, 10, 70784–70804 CrossRef PubMed.
- H. Almqvist, H. Axelsson, R. Jafari, C. Dan, A. Mateus, M. Haraldsson, A. Larsson, D. Martinez Molina, P. Artursson, T. Lundback and P. Nordlund, Nat. Commun., 2016, 7, 11040–11050 CrossRef CAS PubMed.
- D. Martinez Molina and P. Nordlund, Annu. Rev. Pharmacol. Toxicol., 2016, 56, 141–161 CrossRef CAS PubMed.
- J. R. Grigera and A. N. McCarthy, Biophys. J., 2010, 98, 1626–1631 CrossRef CAS PubMed.
- J. Jiang, J. Chen and Y. L. Xiong, J. Agric. Food Chem., 2009, 57, 7576–7583 CrossRef CAS PubMed.
- P. J. Boersema, R. Raijmakers, S. Lemeer, S. Mohammed and A. J. Heck, Nat. Protoc., 2009, 4, 484–494 CrossRef CAS PubMed.
- S. Sridharan, N. Kurzawa, T. Werner, I. Gunthner, D. Helm, W. Huber, M. Bantscheff and M. M. Savitski, Nat. Commun., 2019, 10, 1155–1167 CrossRef PubMed.
- J. Lyu, C. Ruan, X. Zhang, Y. Wang, K. Li and M. Ye, Anal. Chem., 2020, 92, 13912–13921 CrossRef CAS PubMed.
- C. S. H. Tan, K. D. Go, X. Bisteau, L. Dai, C. H. Yong, N. Prabhu, M. B. Ozturk, Y. T. Lim, L. Sreekumar, J. Lengqvist, V. Tergaonkar, P. Kaldis, R. M. Sobota and P. Nordlund, Science, 2018, 359, 1170–1177 CrossRef CAS PubMed.
- A. Cowell and E. Winzeler, Microbiol. Insights, 2018, 11, 1178636118808529 Search PubMed.
- H. N. Fan, M. Y. Zhu, S. Q. Peng, J. S. Zhu, J. Zhang and G. Q. Qu, Pathol., Res. Pract., 2020, 216, 152795–152801 CrossRef CAS PubMed.
- D. Wang, B. Zhong, Y. Li and X. Liu, Oncol. Lett., 2018, 15, 1949–1954 Search PubMed.
- F. Zhang, Q. Ma, Z. Xu, H. Liang, H. Li, Y. Ye, S. Xiang, Y. Zhang, L. Jiang, Y. Hu, Z. Wang, X. Wang, Y. Zhang, W. Gong and Y. Liu, J. Exp. Clin. Cancer Res., 2017, 36, 68–78 CrossRef PubMed.
- K. C. Ravindra, W. E. Ho, C. Cheng, L. C. Godoy, J. S. Wishnok, C. N. Ong, W. S. Wong, G. N. Wogan and S. R. Tannenbaum, Chem. Res. Toxicol., 2015, 28, 1903–1913 Search PubMed.
- J. D. Paccez, K. Duncan, D. Sekar, R. G. Correa, Y. Wang, X. Gu, M. Bashin, K. Chibale, T. A. Libermann and L. F. Zerbini, Oncogenesis, 2019, 8, 14–27 Search PubMed.
- M. Jain, R. Nilsson, S. Sharma, N. Madhusudhan, T. Kitami, A. L. Souza, R. Kafri, M. W. Kirschner, C. B. Clish and V. K. Mootha, Science, 2012, 336, 1040–1044 CrossRef CAS PubMed.
- L. Vettore, R. L. Westbrook and D. A. Tennant, Br. J. Cancer, 2020, 122, 150–156 CrossRef CAS PubMed.
- H. Chen, B. Sun, S. Wang, S. Pan, Y. Gao, X. Bai and D. Xue, J. Cancer Res. Clin. Oncol., 2010, 136, 897–903 CrossRef CAS PubMed.
- X. Zhang, C. Ruan, H. Zhu, K. Li, W. Zhang, K. Wang, L. Hu and M. Ye, Proteomics, 2020, 20, 1900372–1900380 CrossRef CAS PubMed.
|
This journal is © The Royal Society of Chemistry 2022 |
Click here to see how this site uses Cookies. View our privacy policy here.