DOI:
10.1039/D1SD00017A
(Critical Review)
Sens. Diagn., 2022,
1, 87-105
Recent developments towards portable point-of-care diagnostic devices for pathogen detection
Received
4th September 2021
, Accepted 27th October 2021
First published on 29th October 2021
Abstract
The rapid development of accurate and quick diagnostic tools for infectious diseases has made a massive impact in global health. POC devices for pathogen detection have primarily contributed to clinical management in various applications such as pathology, drug discovery and food safety. Diagnostic tests in isolated or remote areas were previously time-consuming, costly, and the methods were extensive and laborious. However, the new generation of miniaturized biosensor technologies aimed at POC testing resulted in a more sensitive, reliable, rapid and cost-effective detection process without needing sophisticated instruments. Among them, a few were developed as ready-to-use devices and are currently commercially available. In this review, we presented the most recent developments in diagnostic methods in pathogen detection and the improvements included in the detection steps in recent POCs for pathogen detection. We also discussed incorporating nanomaterials, microfluidics, lateral flow tests, screen-printed electrodes and smartphones into POC devices used in this area. The challenges and prospects of developing sample-to-results POC devices for pathogen detection were also discussed.
1. Introduction
Diagnosis of infectious diseases due to various pathogen contaminations is of paramount importance in clinical applications, food safety, biodefense, forensic science, and drug discovery. The reduction of infectious disease fatality in resource limited areas is a severe issue worldwide. Analytical techniques with high precision and sensitivity are required for reliable and accurate pathogen detection for constant monitoring. The evolution of pathogen diagnostic assays varied from conventional cell culture-based techniques to modern immunological molecular diagnostics and now to biosensors. An ideal point-of-care (POC) device should be able to work with small volumes of biological, clinical, or chemical samples, to decrease the medical expenses, experimental steps, and analytical time.1 There is a continuous need to develop “sample-to-results” POC devices for on-site monitoring in rural/isolated areas to be operated by semi-skilled personnel for rapid quantification of analytes. This will enable better monitoring towards infection outbreaks and treatment of diseases. Fig. 1 is a schematic overview of POC applications that have been developed by researchers, and among them, few devices are already available in the market to use.
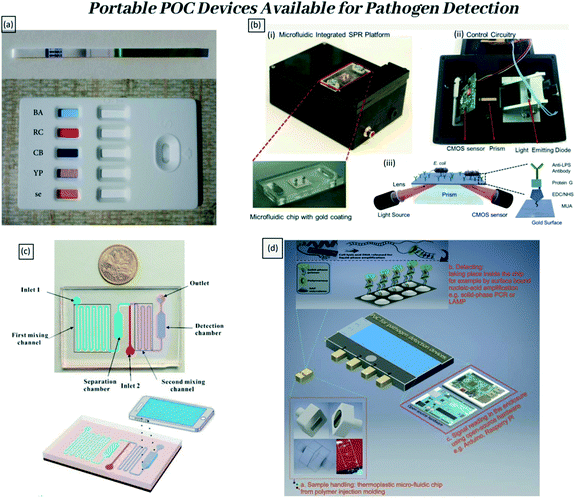 |
| Fig. 1 Examples of portable POC devices available for pathogen detection. (a) Appearance of a commercial LFT device that can detect five biological warfare agents Bacillus anthracis, ricin, Clostridium botulinum/botulinum toxin, Yersinia pestis, and staphylococcal enterotoxin B by Pro Strips (Advnt Biotechnologies, Phoenix, AZ, USA). Adapted with permission from ref. 2, copyright@2021 (Hindawi). (b) Portable plasmonic platform for pathogen detection and quantification. (i) The surface activated disposable microfluidic chips mounted on the device. (ii) The electronic setup of the device is represented from the bottom. (iii) Schematics of the microfluidic integrated SPR platform. The gold surfaces were modified with several activators that can capture E. coli. Adapted with permission from ref. 3, copyright@2015 (Nature). (c) The principle of the proposed colorimetric biosensor for rapid detection of Escherichia coli O157:H7 based on gold nanoparticle aggregation and smart phone imaging. Adapted with permission from ref. 4, copyright@2019 (Elsevier). (d) The three essential components of POC devices for (multiplex) pathogen detection and the proposed solutions. Adapted with permission from ref. 5, copyright@2020 (Elsevier). | |
In the last decade, numerous techniques have been developed for the purpose of pathogen detection (Fig. 1), but only a few made it to the market for real-life applications. This review focused on POC devices that have been commercially developed for pathogen detection, with stronger emphasis on profiling the underlying technologies available for the development of POC devices in this area, as well as their limitations.
2. Methods for the detection and identification of pathogens
2.1. Conventional culture-based method
Microbial culture has been the gold-standard for identification of microbes present in clinical samples for decades. Naturally, the growth medium is composed of essential supplements that will support and promote the growth of microorganisms. For the purpose of further isolation, the growth media are mixed with specific antibiotics, such as ampicillin, kanamycin and tetracycline, to prevent contamination by other microbes on the agar plate.6 This is a basic tool employed worldwide due to its apparent reliability, efficiency and sensitivity. However, this traditional method is very tedious, consisting of many steps, such as sample preparation from food samples, pre-enrichment, enrichment, plating, colony selection and further confirmation via biochemical screening such as morphology observation, staining and biochemical testing.7 Although the method is relatively inexpensive and simple, it is laborious and time-consuming as aseptic techniques were to be followed thoroughly. The identification process could take anywhere between 2 and 10 days and requires trained lab personnel to prepare the samples, perform the tests and interpret the results. Moreover, it is only effective in identifying a fraction of microbes in a given sample, and is incapable of providing accurate microbial virulence factors. In addition, it is not suitable for applications requiring immediate results, in particular, during an outbreak in the food and water industries.
2.2. Nucleic acid-based PCR method
In comparison to the above-mentioned conventional method, the analysis time and cost of running DNA-based microbial identification methods are significantly less, while providing similarly robust and conclusive results. The first-ever DNA-based diagnostic method introduced was the polymerase chain reaction (PCR) technique, developed by Kary Mullis in the 1980s.8 The method isolates, amplifies and quantifies a short DNA sequence on the target bacteria's genetic material from a complex pool of DNA using a specifically designed pair of primers and DNA polymerase as an enzyme. The amplified sequence is later detected using gel electrophoresis. Based on the PCR principle, many advanced nucleic acid amplification techniques were developed such as reverse transcription PCR (RT-PCR)9 and real-time quantitative PCR (qPCR).10 PCR-based detection procedures are rapid, taking anywhere between 5 and 24 hours to obtain results (excluding the enrichment stage), depending on which type of PCR is performed.11 The method is highly specific and sensitive, needing a smaller reagent volume and utilizing instruments that are easily available. This assay has been widely used for pathogen detection from various types of samples, from processed food samples to faecal samples from various living organisms. In the recent COVID-19 pandemic, the causative pathogen SARS-CoV-2 coronavirus has been widely detected using RT-PCR and qRT-PCR worldwide.12–15 The technique is used to quantify the viral load in both symptomatic and asymptomatic patients with very good specificity and sensitivity. Globally, 104 PCR kits are in use to detect this pathogen in hospitals, medical centres, university campuses and airports.15–17 However, usage of a thermal cycler, low sensitivity issues, contamination or carry over issues and false positive issues are some of the disadvantages of this method. Hence, the development of other advanced methods is in demand to detect pathogens.
2.3. Antigen–antibody-based (ELISA) method
After the PCR method, another popular way to detect pathogens is by employing the immunological-based technique called enzyme-linked immunosorbent assay (ELISA). The basic concept of ELISA is based on antibody–antigen interactions, whereby a particular antibody will bind to its specific antigen. The strength of ‘antibody binding’ determines the sensitivity and specificity of the method. In general, an antigen (for example, a bacterial cell or bacterial toxin) is immobilized onto a solid surface to capture the antibody. If there is interaction between the antigen and the antibody, then this is detected by the addition of a substrate that can generate a colour change or fluorescence. Three types of ELISA have been extensively used, which are 1) direct ELISA, 2) indirect ELISA and 3) sandwich ELISA.18 Sandwich ELISA has been previously utilized for the detection of Vibrio parahaemolyticus in seafood,19 with availability of commercial ELISA kits for detection of pathogens in food products, like Salmonella species.20 The ELISA method gives the final readout in a relatively short period of time. However, since it is a single end-point assay, there is a high possibility of obtaining false positive or negative outcomes. Moreover, this technique is considered to be relatively expensive due to the high cost of instruments and reagents needed. The antibody needed in this technique is relatively unstable. Additionally, antibody production is a laborious and costly process. At the same time, it is also challenging to adapt the method for high-throughput screening processes on a larger scale.21,22
2.4. Micro-array-based method
On the other hand, a DNA microarray can perform simultaneous detection of many pathogens at one time that is achievable through miniaturization. In this process, glass slides are coated with hundreds of specific short oligonucleotide probes that are able to target a part of the gene sequence derived from pathogens.23 Due to the small volume size of the reactions, this method is able to achieve a low limit of detection (LOD) and low running cost. Overall, it enables relatively faster detection of pathogens due to reduction in sample preparation time and incorporation of microfluidics technology.24 Pathogen detection using the DNA microarray was previously employed for only viral and fungal infection screening processes.25,26 Recently, a PCR-based microarray assay was reported to achieve good sensitivity by Vora and colleagues in the detection of Escherichia coli (O157:H7) from water samples. The LOD achieved was 100 genomic copies per reaction with a specificity of 97%.27 Thus, this method has big potential in environmental surveillance of pathogen identification.
2.5. Biosensor-based method
A biosensor is a device that contains a bioreceptor and a transducer that can recognize the target analyte derived from pathogens. Essentially, the bioreceptor recognizes the biological event taking place and the transducer converts the biological reaction into a measurable signal.28–30 Biosensors have been increasingly utilized in foodborne and waterborne pathogen detection in order to address one of the main challenges in the area, which is the rapid detection of a small amount of microorganisms, as they help to detect a tiny amount of antigen with high accuracy and rapidity. Some examples of pathogens that could be identified using various biosensors are E. coli, L. monocytogenes and Salmonella.31,32 Additionally, biosensor technologies have been the preferred method for pathogen detection recently as most of the systems are automated with a simple operation procedure, portable, and inexpensive and, most importantly, this area has been increasingly adapted as POC devices. Moreover, the integration of micro- and nano-fabrication technologies into the biosensor platform can enable screening of multiple pathogens in a single device. Increasingly, nanomaterials (NMs), such as nanoparticles, nanosheets, nanotubes, nanowires and nanorods, have been incorporated into biosensor platforms for pathogen detection to increase sensitivity and specificity.33
2.6. Isothermal-based DNA amplification method
As an alternative to PCR-based nucleic acid detection, the molecular biology field has developed various techniques that can amplify DNA isothermally in a single heat block. This means that amplification is performed at a constant temperature, reducing the need for an expensive thermal cycler and skilled personnel as well as shortening the reaction time. Some of the isothermal amplification methods that have been introduced are loop-mediated isothermal amplification (LAMP),34–36 helicase-dependent amplification (HAD),37 isothermal strand displacement amplification (SDA),38 rolling circle amplification (RCA)39 and signal-mediated amplification of RNA technology (SMART).40 Among all these techniques, LAMP appeared to be popular for pathogen detection as it is capable of amplifying medium to long ranges of targeted nucleic acid strands with high efficiency, sensitivity, specificity and stability.41–44 The combination of LAMP in biosensors allowed for a straightforward detection of pathogens using a paper-based colorimetry assay, and this approach could be useful as POC devices.45 One of the recent studies was conducted on the fish pathogen Streptococcus iniae with a colorimetric biosensor using gold nanoparticle-labelled probes and LAMP assay. In this study, the authors were able to detect a LOD of 102 CFU which is more sensitive than normal PCR and the conventional lateral flow strip process. Additionally, the whole process of detection took place less than 2 h.39 Many researchers have combined the LAMP method with an electrochemical sensor (E-Sensor) to achieve better sensitivity and good reproducibility. It has been proven that this E-sensor has the capability of detecting pathogens in a broad detection range like 1 fg μL−1 to 100 pg μL−1.46 However, the LAMP-based sensor also has some drawbacks such as long collection time, deep data analysis, some safety concerns, and the primer design is complicated.47,48
3. Enhancements in current POC devices for pathogen detection
3.1. Sample preparation
Sample processing is a crucial prerequisite step in pathogen detection, and it is required to concentrate raw samples from liters (L) down the microliter (μL) scale, which is a volume that is more suitable for processing by POC detection strategies. For most occasions, the collection of a relatively large raw sample volume which is mixed with other impurities or untargeted elements is required to increase the likelihood of detecting pathogens, which are often present at very low concentrations. Preparation of samples for any sensor-based detection process requires minute observation specially to make the sample pure from unwanted elements that come with the collected raw samples. In a nucleic acid-based sensor, first DNA is extracted with an available extraction kit and then prepared for further analysis using a sensor.49–51
The presence of inhibitors, interference of mix elements, and relatively low microbial cell numbers in a sample to be analyzed can be problematic for minute detection analysis. The presence of these substances in abundance reduces the overall selectivity of the sensor and creates cross contamination issues. Thus, solving the selectivity issue is one of the significant challenges in developing practical biosensors. The basic strategies that allow selective detection using biosensors include having a clear idea of the sample composition, designing the biosensor such that the possible interferences are taken into consideration, and rechecking the method against a standard procedure. More importantly, the sample must be optimized in such a way that they are devoid of any interfering compounds during the detection. This issue can be tackled by having pre-treatments on samples such as separation and concentration of pathogen target analytes from various sample matrices. Sample pre-treatments are generally very difficult and take up the most out of the assay time. Conventional sample preparation for pathogen detection requires tedious procedures. For instance, the procedures involved in this case are inoculation and selective culturing of microorganisms, physical lysis to release the cell contents, sub-cloning or cloning into appropriate hosts, purifications and so on before the presence of target analytes can be tested on desired detection platforms.
3.2. Bio-recognition receptors
To tackle the limitations posed by antibodies, alternative molecules have been studied. Aptamers, artificial binding proteins, molecularly imprinted polymers, and NPs can selectively bind to biomolecules.52 In this review, we described antibodies, bacteriophages, and aptamers in detail. These antibody alternatives do not use animal hosts for antibody production and therefore do not pose any ethical issue for the public and scientific community.53,54
3.2.1. Antibodies.
For decades, antibodies have been primarily used as recognition probes in detecting and diagnosing pathogenic microorganisms. The immune system produces antibodies to defend the body against any foreign antigen exposure. In scientific studies, antibodies are harvested in mammals by immunizing them with specific antigens. Because of their highly precise binding capability, antibodies are employed as recognition probes in detection bioassays. Sandwich-type assays are the best to illustrate the significant roles of antibodies in detection bioassays. In sandwich-type immunoassays, there are at least two antibodies: one plays the role of the recognition probe and the other acts as the signalling probe. The success of the immunoassay relies predominantly on the specificity and sensitivity of the recognition antibody's binding ability with the target of interest. This approach has been applied for the detection of Escherichia coli,55–57Mycobacterium tuberculosis,58 influenza viruses,59 HIV,60,61Staphylococcus aureus,62,63Salmonella species,57,64,65Listeria species,66,67Shigella species,68,69Norovirus strains70,71 and Clostridium species.72,73
Full-length antibodies have two unique polypeptides such as the light and heavy chains. The antigen-restricting site is shaped by six hyper-variable circles, three each from the two chains. Because of the complex engineering involved, the cloning steps for the recombinant expression of a full-length immune response can become complicated. Besides, the fundamental multifaceted nature of full-length antibodies requires a customary articulation framework for the mass generation of full-length antibodies. To overcome this restriction, counteracting agents like substantial chain antibodies have been developed. These neutralizer parts cannot hold the complete antigen and, therefore, restrict a full-length immune response. This makes the process less demanding in terms of development and control.
3.2.2. Bacteriophages.
Bacteriophages infect bacterial cells and are abundantly found in nature. There are about 1032 bacteriophage entities in soil, manure, thermal vents, and water. Apart from their abundance, their ability to survive adverse environmental conditions and reproduce quickly in their specific targeted host makes them ideal regulators of microbial balance on earth and an indicator of dynamic equilibrium amongst bacterial species.74 Phages offer many advantages in biosensing, like the specificity in binding to their target host cells, the ability to lyse and kill their hosts, and the capacity to multiply during an infection process. This makes phages a valuable tool for the detection and identification of bacterial pathogens.
Bacteriophages have been employed in different ELISA formats as recognition molecules to capture analytes. For instance, β-galactosidase, streptavidin, and neutravidin molecules are used in the direct ELISA format. This was seen in the study carried out by Rajkovic and colleagues, where a landscape phage library was used to select target-specific phage particles.75Bacillus anthracis spores were captured by spore binding phages selected from the f8/8 landscape phage library. A phage like M13 was used as a reporter to identify the captured molecules. This event was amplified using anti-M13 horseradish peroxidase (HRP) that binds to each pVIII protein. This approach was successfully used to detect Brucella melitensis. The cells were captured using purified single chain antibodies (scAb) on the HRP conjugated phage that displayed antibody fragments (scFv) in the sandwich ELISA format. This resulted in two orders of magnitude improvement in sensitivity compared to the soluble format.76 In a similar approach, phage-displayed single domain antibodies (sdAb) were used as reporter elements to detect several analytes, including ricin, botulinum-A toxin complex, and Marburg virus in the sandwich ELISA format. The sensitivity of assays was seen to be improved compared to the soluble sdAb.77–79
During the past decade, several bacterial analytes have been detected by SPR biosensors that employ a phage as the bio-recognition probe. Likewise, a lytic phage was used in the SPREETA™ sensor to detect Staphylococcus aureus and showed a LOD of 104 CFU mL−1 of S. aureus cells. In this study, it was observed that the nonspecific Salmonella typhimurium did not create any significant response. At the same time, E. coli detection was performed using a T4 phage probe in the SPR imaging system with a LOD of 104 CFU mL−1.80 A Salmonella-specific M13 phage selected from a commercially available phage display peptide library was employed to detect Salmonella cells with a LOD of 1.3 × 107 CFU mL−1. A similar approach was employed to detect β-galactosidase (β-gal), a well-known coliform marker used by the SPREETA™ sensor. It helped to achieve a linear range of 10−9–10−6 M. SPR sensors successfully detected E. coli bacteria using a T4 phage where the bacterial cells were detected in a concentration range of 7 × 102 to 7 × 108 CFU mL−1.81 In another study carried out by Tawil and co-workers, T4 and BP14 phages were employed in a phase-sensitive SPR to detect E. coli and methicillin-resistant Staphylococcus aureus (MRSA) with a LOD of 103 CFU mL−1 for both species.82 At the same time, another group employed the T4 phage by physical adsorption on long-period fibre gratings (LPGs) to detect E. coli in their studies.83 The LPG sensing principle relies on measuring the changes in resonance wavelength (λR) due to the external refractive index changes. Additionally, a modified, more accurate and extremely sensitive version of the LPG sensing platform was developed by the same group that used telecommunication wavelengths (∼1.56 um). This sensor was shown to have improved the LOD down to 103 CFU mL−1 and achieved a linear range of 103–106 CFU mL−1 for E. coli detection.84 Furthermore, an evanescent mode of a terahertz fibre was recently used by Mazhorova et al. to detect E. coli bacteria using T4 phages.85 The system achieved a LOD of 103 CFU mL−1E. coli bacteria.
Electrochemical biosensors (EB) have received significant attention in the biosensing field due to their low cost, simplicity, portability, high accuracy, and sensitivity.86 Phage particles are utilized as recognition receptors in these devices and can be used as biocatalytic and affinity biosensors.87,88 Phage-based amperometric sensors mostly rely on lytic phages as bacteria-specific recognition receptors and releasing agents of intracellular bacterial enzymes. This approach was initially applied to detect E. coli K12 cells using phage λ as a recognition probe. The activity of released β-D-galactosidase, a highly specific marker of E. coli, was measured using p-aminophenyl-β-D-galactopyranoside (β-PAPG) as a substrate, and the product of the reaction, p-aminophenol (PAP), was detected. The PhiX174 phage was also employed as another recognition probe to detect E. coli contamination in water,89 amperometrically. B. cereus and M. smegmatis cells were also successfully detected by combining phage typing and cell marker enzyme activity, using B1-7064 and D29 phages, respectively. This was done by measuring the activities of alpha-glucosidase and beta-glucosidase enzymes. Laczka and the group used a phagemid system to detect E. coli TG1 cells through alkaline phosphatase activity.90 The phagemid was constructed using a bacteriophage, M13KO7, as a helper phage and a commercial plasmid, pFLAG-ATS-BAP, which contained a gene encoding the reporter enzyme, alkaline phosphatize. M13KO7 is a non-lytic phage that cannot replicate in the cells; therefore, it overproduces enzymes in the cells. This also enhanced the sensitivity of the assay as 1 CFU mL−1 bacterial cell could be detected within 3 h.
Several researchers have also used EIS to detect E. coli cells using biotin displaying T4 phage L91 and wild type T4 phage covalently immobilized on screen-printed carbon electrode arrays for direct impedimetric detection of E. coli K12 cells.92E. coli cells were detected in a concentration range from 104 to 108 CFU mL−1 with a detection limit of 2 × 104 CFU mL−1. Phage-modified magnetic beads have also been used to capture and concentrate bacteria in the samples, which were then integrated with impedance detection. Leung et al. improved the LOD down to 103 CFU mL−1.93 Shabani et al. detected Bacillus anthracis Sterne vegetative cells using gamma phage modified screen-printed carbon electrode microarrays,94 and Bacillus anthracis cells were detected with a LOD of 103 CFU mL−1.95
Simultaneously, few studies have also shown that β-galactosidase can be detected with a response time of ∼100 s over the range of 0.003–210 nM.96S. typhimurium was detected with a LOD of 102 CFU mL−1 and a rapid response time of <180 s. Magnetoelastic (ME) sensors decorated with the E2 phage selected from the landscape f8/8 phage library were used to detect S. typhimurium in milk and fresh products such as spinach leaves, tomato, and cantaloupe.96–98 In a similar approach, methicillin-resistant S. aureus (MRSA) in spinach leaves was successfully detected using lytic phage 12600 as a bio-recognition probe.99 In addition, B. anthracis spores were detected using ME with a LOD of 103 CFU mL−1. In addition, the filamentous E2 phage immobilized on a magnetostrictive millicantilever (MSMC) surface could detect S. typhimurium cells with a LOD of 5 × 108 CFU mL−1.100 In another report, the filamentous JRB7 phage was used to develop an MSMC sensor for detection of B. anthracis spores in water with a LOD of 104 CFU mL−1 and 105 CFU mL−1 by using MSMCs in size of 1.4 mm × 0.8 mm × 35 m and 2.8 mm × 1.0 mm × 35 m, respectively.101
As observed from the above-discussed studies, phage-based sensors show a natural affinity towards their target analytes and therefore are easier to prepare and are available at cheaper rates. The phage display techniques also use wild-type phages that can be genetically modified to display various protein molecules and bind with numerous target molecules. Moreover, owing to the enhanced stability of phages, these sensors have been shown to remain active for prolonged durations even under extreme environmental conditions.102
3.2.3. Aptamers.
Aptamers have been well documented as another fascinating molecular recognition element for pathogen detection.103 They are the most commonly used antibody alternatives. An aptamer is a synthetic short oligonucleotide sequence composed of either DNA or RNA molecules, which can bind to target analytes with high affinity and specificity. These nucleic acid molecular recognition elements were first described in 1990 by the Tuerk and Ellington laboratories.104 Aptamers also have several other advantages over antibodies, like their ease of conjugation with different reporter molecules, lower production cost, high reproducibility without batch-to-batch variations, and no requirements for animal hosts during production.
The recognition ability of aptamers comes from their conformation to three-dimensional structures that generate binding pockets for their intended targets. In general, the aptamer library comprises 1013 to 1015 different nucleic acid molecules. Systematic evolution of ligands by exponential enrichment (SELEX) identifies the newly designed aptamers with the highest affinity and specificity towards user-defined targets.105 Aptamers that bind to the intended target are retained and amplified by PCR amplification. This process is repeated for 10 to 20 rounds to enrich and increase the specificity of the aptamer pool. This approach of using aptamers as bio-recognition receptors has been applied to detect E. coli,106,107M. tuberculosis,108–112 influenza viruses,113 HIV,114–116S. aureus,117–122Salmonella species,123Listeria species,124Shigella species,125Campylobacter species,126Norovirus strains,127,128Vibrio cholera,129 and Clostridium species.130 However, the real-life application of aptamers depends on their susceptibility to nuclease degradation. Hence, sample pre-treatment and enrichment steps are usually required before using an aptamer as a recognition probe.
Various bioreceptors used in different biosensors are summarised in Table 1.
Table 1 Various bioreceptors used in different biosensors
Bio-recognition receptors |
Detection range |
Pathogens detected |
LOD |
Ref. |
Antibodies |
1.0 × 103 to 1.0 × 107 CFU mL−1 |
S. typhimurium
|
5.0 × 102 CFU mL−1 |
131
|
— |
S. enterica
|
102 cells per mL |
64
|
Up to 1 × 108 cells per mL |
E. coli and S. epidermidis |
2.5 × 102 cells per mL |
132
|
— |
E. coli, B. subtilis and S. aureus |
1.5 × 104 cells per mL |
133
|
Bacteriophage |
— |
Clostridium botulinum
|
3.33 × 10−14 mol |
134
|
103–106 CFU mL−1 |
E. coli and S. aureus |
103 CFU mL−1 |
84
|
20 to 2000 CFU 100 mL−1 |
Enterobacteriaceae
|
5 × 104 CFU mL−1 |
90
|
— |
E. coli
|
103 CFU mL−1 |
95
|
0.003 to 210 nM |
E. coli
|
— |
96
|
7 × 102 to 7 × 108 CFU ml−1 |
E. coli
|
104 CFU mL−1 |
83
|
Aptamers |
10–104 CFU mL−1 |
E. coli O157:H7 |
102 CFU mL−1 |
108
|
104–107 CFU ml−1 |
Escherichia Coli O157:H7 |
4.5 × 103 CFU mL−1 |
109
|
1 × 10−7–2 × 10−6 M |
E. coli
|
— |
110
|
0.1 nM to 10 nM |
E. coli
|
— |
111
|
10 to 1 × 106 CFU mL−1 |
S. aureus
|
1.0 CFU mL−1 |
119
|
5 × 102 CFU ml−1 to 5 × 109 CFU ml−1 |
S. aureus
|
105–106 cells per mL |
120
|
0.01–10 μg mL−1 |
S. aureus
|
6 ng mL−1 |
121
|
4.1 × 101–4.1 × 105 CFU mL−1 |
S. aureus
|
41 CFU mL−1 |
122
|
2.4–2.4 × 103 CFU mL−1 |
Salmonella
|
3 CFU mL−1 |
125
|
20 to 2 × 106 CFU mL−1 |
L. monocytogenes
|
20 CFU mL−1 |
126
|
4. Using nanomaterials in POC devices for pathogen detection
New developments in nanoscience have highly influenced medical and analytical sciences. Nanoscience has also affected detection analysis due to its versatile physicochemical properties that depend on particle size, shape and type. The development of electrodes for nanoscale sensors has become an emerging field and has gained widespread attention in diagnostic purposes.135 Nanomaterial-based (NMs) biosensors show enhanced sensitivity, rapid response time and reduced cross-contamination issues. These advantages can potentially facilitate the construction of POC devices28 for quick pathogen detection. Nanoparticles (NPs) also have a large surface-to-volume ratio, enabling various biomolecules to interact with them. The biomolecules are first immobilized to make the reaction sites available to interact with target species like bacteria, toxins, proteins, and nucleic acids. Owing to their biocompatibility and the ability to adsorb particles, NMs used in sensors often modify their electrode surfaces to enhance the overall functioning of the sensors. In addition, NMs strengthen the immobilization of the analytes on the bioreceptor surface by aiding the interactions between the two. Furthermore, NPs act as signalling molecules and allow for signal amplification during the sensing process. Table 2 summarizes the various NM-based biosensors used to detect different pathogens.
Table 2 Nanomaterial-based biosensors used to detect different pathogens
Nanoparticles used during bacterial sensing |
Pathogens detected |
Detection range |
Detection limit |
Ref. |
Graphene oxide |
E. coli
|
10−6 to 10−16 M |
1 × 10−16 M |
136
|
E. coli
|
1 × 10 to 1 × 104 CFU mL−1 |
10 CFU mL−1 |
137
|
S. aureus
|
1 to 40 nmol L−1 |
0.5 nmol L−1 |
138
|
M. tuberculosis
|
1.0 × 10−6 to 1.0 × 10−12 M |
7.96 × 10−13 M |
139
|
P. aeruginosa
|
1.2 × 101–1.2 × 107 CFU mL−1 |
12 CFU mL−1 |
140
|
Quantum dots |
S. aureus and E. coli |
0 to 9 × 107 CFU ml−1 |
— |
141
|
M. tuberculosis
|
1 × 10−11 to 1 × 10−7 M |
8.948 × 10−13 M |
142
|
S. typhimurium
|
1.0 × 102 to 1.0 × 107 CFU mL−1 |
43 CFU mL−1 |
143
|
S. typhimurium
|
103 to 107 CFU ml−1 |
103 CFU mL−1 |
144
|
S. typhimurium
|
1.0 × 104 to 1.0 × 106 CFU mL−1 |
5.4 × 103 CFU mL−1 |
145
|
E. coli O157:H7 |
— |
6.660 CFU mL−1 |
146
|
S. aureus
|
— |
1.070 × 101 CFU mL−1 |
146
|
V. parahaemolyticus
|
— |
2.236 × 101 CFU mL−1 |
146
|
AuNPs |
E. coli O157: H7 |
101–106 CFU ml−1 |
101 CFU ml−1 |
147
|
Escherichia coli O157:H7, Vibrio parahaemolyticus, salmonella, Staphylococcus aureus, listeria monocytogenes, Shigella |
— |
1–9 cells mL−1 |
148
|
V. cholera
|
3.2 × 104 to 28 × 104 cells ml−1 |
— |
149
|
P. aeruginosa and V. cholerae |
— |
∼102 CFU |
150
|
S. enteritidis
|
104 and 108 CFU mL−1 |
103 CFU mL−1 |
151
|
AgNPs |
S. flexneri, E. coli O157:H7, L. mono and S. aureus |
101 to 107 CFU mL−1 |
1.5 CFU mL−1 |
152
|
L. monocytogenes
|
— |
0.015 ng mL−1 |
153
|
S. enterica
|
— |
0.013 ng mL−1 |
153
|
S. typhi
|
103–105 CFU mL−1 |
103 CFU mL−1 |
154
|
H. pylori
|
— |
10 CFU mL−1 |
155
|
PtNPs |
E. coli O157:H7 |
4.0 × 102 to 4.0 × 108 CFU mL−1 |
91 CFU mL−1 |
156
|
S. typhimurium
|
10–15 CFU mL−1 |
2 CFU mL−1 |
157
|
E. coli O157:H7 |
5 × 102 CFU mL−1 to 1 × 107 CFU mL−1 |
1.08 × 102 CFU mL−1 |
158
|
L. innocua
|
— |
100 CFU mL−1 |
159
|
The primary motivation of nanoscale devices is to increase sensitivity, specificity and provide simple detection mechanisms.160,161 NM-based POC devices like biosensors have been rampantly used in recent years to detect various kinds of bacterial and clinical pathogens. NM-based sensors employ many or all of the properties of NPs, including their chemical, optical, magnetic, and electrical features, to detect pathogens.162 The molecular determination forces the enhancement of the sensors to produce POC devices that can perform rapid and inexpensive detection of several pathogens.163 NMs and nanostructures like carbon nanotubes, metal oxide NPs, magnetic NPs, metal nanoclusters, nanogels, and plasmonic nanomaterials have been employed in several studies to achieve this purpose. Recently three widely used NPs have been implemented in biosensing platforms, including gold nanoparticles (AuNPs), silver nanoparticles (AgNPs), and platinum nanoparticles (PtNPs). These NPs are employed as signalling molecules in various electrochemical sensor assays for pathogen detection.164,165 In addition, there are more modified NPs available that are applied for pathogen detection, like quantum dots and graphene oxide. We will discuss biosensors based on these two categories of nanoparticles in the subsequent sections.
4.1. Quantum dots (QDs)
In a study in 2014, Liu and colleagues developed an effective isothermal amplification process using AuNPs. This low-cost paper platform could detect pathogenic bacteria and showed a detection limit of 0.5 pg μL−1 genomic RNA from viable L. monocytogenes. This assay had a high specificity and gave the result within 15 min. This assay was also tested on real samples like milk and cheese; it could be used as a POC-based analysis for foodborne pathogenic bacteria.166 Recently, quantum dots (QDs) have also seen massive potential in the sensor field in NP-based technology owing to their size-dependent optical and electronic properties.167 QDs derive their energy from tiny materials that efficiently work with concentrated samples into a single point, thereby charging them with electric power.
According to quantum theory, QD NPs have distinct energy levels like an individual atom.168 The QD core–shell can detect biomolecules following the florescent process. Xiong et al. observed that core–shell ZnO QDs possessed yellow and green emission and could perform biological labelling. This novel approach involving QD core–shell NPs was stable in aqueous solutions and could easily detect biological samples or liquid analytes. The concentration of live cells was 0.2 mg mL−1 and was protected with this core–shell QD, which could infiltrate into the cytoplasm (Fig. 2).169 The significant advantage of ZnO-based core–shell QDs was their non-toxic and safe nature that could be used in live cells, including human cells. This is an exciting approach in biological samples that do not use complex compounds or reagents.
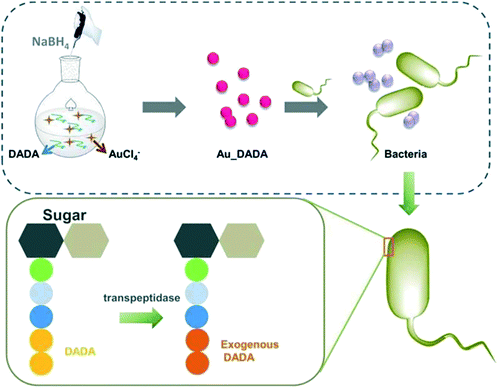 |
| Fig. 2 Schematic representation of modified AuNPs and D-amino acid-modified QDs and the color change of peptidoglycan after incubation with newly modified synthesized AuNPs. Reproduced with permission from ref. 169. | |
Compared to traditional fluorescent dyes such as fluorescein isothiocyanate (FITC), QDs display brighter fluorescence intensities and last longer.170,171 Due to the advantages of QDs over traditional fluorescent dyes, they are widely used as fluorescent labels172 instead of traditional dyes. They are used as biorecognition elements in a wide range of immunoassays to detect various foodborne pathogens.
4.2. Graphene oxide (GO)
NPs have been widely used in sensing platforms for in vitro studies. Such studies use matrix modifications on the electrode surface for signal amplification purposes. The detailed mechanisms correlating to the changes of intrinsic physicochemical and optoelectronic properties play different roles in instantaneously identifying microbes by changes in their color. They act as sensory probes and therapeutic agents and can be used in molecular diagnosis and drug discovery for biological, clinical, and chemical purposes. NMs are vital for pathogen detection and are used in various easy-to-engineer biosensor devices with finely-tuned configurations at the nanoscale level. For example, graphene is a two-dimensional carbon NM with unique properties like high electrical conductivity, large surface area, and mechanical and thermal stability.35 Graphene and its derivatives, graphene oxide (GO), reduced graphene oxide (rGO), and graphene nanoribbons (GN), have also exhibited exciting biosensing applications to detect a variety of pathogens.173 Based on the diverse pathogen sensing processes, we divided our study into the two following sections.
4.2.1. Fluorescence-based GO method and its applications.
Due to the increasing demand for rapid and robust techniques, researchers have reported sensitive sensing of pathogens using GO-based sensors.174 GO was successfully synthesized via the Hummers method in a recent study by employing MnO2O7 to oxidize graphite.175 In another study about rotavirus detection using a GO-based immune-biosensor, the rotavirus was captured by an antibody immobilized on GO sheets using rotavirus-specific antibody. The binding was monitored by observing the fluorescence quenching of GO during fluorescence resonance energy transfer between GO and gold NP-labeled secondary antibodies. This platform utilized antibodies–QDs as capture probes that fluorescence upon excitation with a laser source. Then GO was added to quench the fluorescence in the absence of the target pathogen. However, in the presence of a target, the interaction between the target and immobilized probe hinders the interaction of the probe and GO, thereby causing lower quenching.176 In another similar study, a GO-based fluorescent aptasensor was used to detect Salmonella typhimurium. This study determined a LOD of 100 CFU mL−1 with a linear range from 1 × 103 to 1 × 108 CFU mL−1. Moreover, this process was label-free, sensitive and specific, and could be applied for real sample analysis for pathogen detection.177 Thus, these discoveries show the great potential of GO-based biosensors for pathogen detection, food safety testing, clinical diagnosis and environmental monitoring. Additionally, these newly advanced methodologies are simple to use, have high portability, and do not involve complex steps.
4.2.2. Electrochemical-based GO method and its application.
Recently numerous studies have been carried out based on GO-based electrochemical sensors that can successfully detect various pathogens.178,179 Various sensitive and specific label-free graphene-based electrical biosensors have been reported that can detect different bacteria species. One such study demonstrated the development of a label-free graphene-interfaced capacitor chip for capturing bacterial cells.180 The authors prepared graphene via chemical vapour deposition and functionalized it by noncovalent attachment of 1-pyrenebutanoic acid succinimidyl ester in methanol for 2 h. The anti-E. coli antibodies were then immobilized on the functionalized electrodes. This graphene-based electrical chip could detect E. coli O157:H7 bacteria without the involvement of any chemical mediators. Upon binding with the target E. coli bacteria, a significant increase in the conductance was observed. This sensor gave a LOD of 10 cells per mL with a detection range of 10 to 100 cells per mL.
Another pathogen-detection system was reported in 2012 that was based on the quenching property of GO.181 In this work, graphene was printed onto water-soluble silk, yielding a fully biointerfaced sensing platform. Antimicrobial peptides were then immobilized onto graphene via self-assembly and employed to detect bacteria at a single-cell level. The wireless sensor was fabricated using a resonant coil, which allowed the integration of the sensing device onto the tooth for real-time detection of bacteria in saliva. This approach of interfacing graphene nanosensors and biomaterials showed an extensive detection of biochemical and pathogen targets. In another study, an rGO–gold NP composite was used as the sensing platform to detect Mycobacterium tuberculosis. A DNA electrochemical biosensor was developed using rGO and AuNPs that showed good sensitivity and stability. A nanoparticle–polyaniline nanocomposite was used as a tracer in the experiment. The linear range of detection was noted to be 1.0 × 10−15 to 1.0 × 10−9 M.182
Another electrochemical genosensor based on a multiwalled carbon nanotube–chitosan–bismuth complex and lead sulfide NPs was developed recently to detect pathogenic Aeromonas. The detection limit was recorded as 1.0 × 10−14 M. During real sample analysis, Aeromonas spiked up, like in tap water. In such cases, the LOD was found to be lower than 102 CFU mL−1. This study showed a rapid and sensitive detection process of bacteria samples and could be potentially used for food safety and environmental monitoring applications.183 Another excellent research study involved a chemiresistive immunosensor based on a carbon nanotube that could simultaneously detect Escherichia coli O157:H7 and the bacteriophage T7. This sensor demonstrated good sensitivity (LOD 103 CFU mL−1 for E. coli and 103 PFU mL−1 for bacteriophage, respectively) along with an excellent dynamic range (linear range for E. coli 103 CFU mL−1 to 107 CFU mL−1 and bacteriophage 102 PFU mL−1 to 107 PFU mL−1, respectively) and a fast response time of 5 min in the case of bacteriophage detection and 60 min to detect bacteria.184 The above stated examples are some of the recent advances in the development and application of graphene-based fluorescence and electrochemical biosensors, which can be potentially studied and further developed as POC devices for food safety and in the biomedical field. In Table 3, we have discussed the advantages and disadvantages of these two processes with examples of sensor matrices in detail.
Table 3 The advantages and limitation of fluorescence and electrochemical-based GO methods
Method |
Advantage |
Disadvantage |
Examples |
Ref. |
Fluorescence-based GO method |
GO acts as an excellent fluorescence quencher and thus allows for highly sensitive bio-sensing |
GO can be further purified to remove excess oxygen moieties and functionalized to obtain better fluorescence peaks |
GO-based aptasensor to detect S. typhimurium |
177
|
GO-based immuno biosensor |
176
|
Electrochemical-based GO method |
The large surface area of GO and superior electrical properties like high charge mobility and changeable conductance allow for highly sensitive detection of the analytes |
Bio-anchoring occurs on the sensor's surface without any observable changes in the sensor's electrical properties during detection. Also, such sensors use redox-mediators that affect the signal transduction process |
Label-free graphene-interfaced capacitor chip to detect E. coli cells |
180
|
GO-based fully biointerfaced, wireless sensing platform to detect bacterial cells |
181
|
rGO–gold NP composite-based biosensor to detect Mycobacterium tuberculosis |
182
|
MWCNT–Chi–Bi-based electrochemical genosensor to detect Aeromonas |
183
|
5. Incorporation of microfluidic POC devices for pathogen detection
Several efforts have been made to develop innovative methodologies to overcome the drawbacks posed during POC development. As a result, many alternative pathogen detection and diagnosis techniques are available based on POCs, such as DNA-based biosensors, immunosensors, aptamer sensors, smartphone-assisted sensors, microfluidic platforms, and wearable sensors. Some of these modern pathogen detection approaches and advancements in this field will be discussed in the subsequent sections.
Microfluidic devices are fluid handling devices in which a minute volume of the sample flows into channels that have dimensions ranging from a few to hundreds of microns. Microfluidics has shown growing potential in different applications in recent years, especially in biosensors.185 The use of microfluidics has demonstrated several advantages, such as 1) minimal amount of sample or reagent requirements which reduce the overall cost and the risk of contamination, 2) the target analyte easily gets localized on the sensing area because of the small dimension of the chip, 3) the analysis time is reduced because of the quick mass transport within the microchannels, and 4) the functionalization of the inner surface of the channels that are typically made of plastic, polydimethylsiloxane (PDMS) or glass, thus, enabling the capture of the analyte under continuous flow conditions.
Label-free electrical and electrochemical biosensors have received considerable attention due to their compatibility with microfabrication techniques involving various microfluidic channels. In one of the studies, researchers constructed an on-chip artificial pore to sense bacterial pathogens. The microfluidic chip was fabricated using PDMS. The channels were functionalized with proteins that could specifically bind to the bacterial cell-surface receptors. The capture of cells onto the channels blocks the current flow. Thus, the duration of the recent pulse can differentiate between the specific and nonspecific cells. This method has been used to detect murine erythroleukemia cells. The number of cells can be determined by measuring the change in solution conductivity after cell lysis that occurs with the release of ions from the cells.186 Another study demonstrated the fabrication of a microfluidic silicon chip with thin-film platinum electrodes. The sensing chamber was functionalized with specific antibodies for the target bacteria. This study achieved a LOD of ∼104 CFU mL−1 of E. coli in a tiny chamber of 2 μm height.187
The integration of LAMP amplification onto a lab-on-chip device to detect Salmonella is another thriving area in microfluidics devices (μD) that has been reported.188 In this study, the pathogen was detected by visualizing the SYBR green I dye color change. An inexpensive microfluidic paper device was fabricated via the wax printing technique using a solid ink printer. This study achieved a LOD of 2.6 × 107 CFU mL−1 during Salmonella live-cell detection and showed great sensitivity. The authors reported this method to have great potential for POC testing purposes. Other μD are also available for pathogen detection like microfluidic protein/enzyme-based pathogen sensors, microfluidic antibody/aptamer sensors and microfluidic cell-based pathogen sensors. Moreover, numerous frameworks have been already utilized to detect pathogens in manageable samples under research environment conditions. In one of the recent studies in the year 2020, the authors fabricated a good selectivity, stability and low-cost 3D paper-based microfluidic electrochemical glucose biosensor based on rGP-TEPA/PB sensitive film with a detection limit of 25 μM and with a linear range of 0.1–25 mM. The novelty of their work was the design of sensing metrics which provided a high conductivity and large surface area for good electrocatalytic reduction activity.189 Around the same time, researchers have reported a proof-of-concept using a paper-based microfluidic device for high throughput multiplexed analysis. The main appealing part of this system is the disposable low-cost microfluidic device with a LOD of 3 × 10−5 mol L−1.190 Meanwhile, applications of thread-based microfluidics are also in demand. In a new study, a thread-based microfluidic fuel cell with graphite rod electrodes was developed by the capillary driven reaction of electrolytes.191 Hence, based on these proofs of concept sensing platforms will help to detect various pathogens in the near future.
6. Portable POC devices available for pathogen detection
Many major classes of portable POC diagnostics devices were developed for pathogen detection. The most commonly used POC devices include utilizing lateral flow tests (LFT) and their lateral flow assays (LFA).
6.1. Lateral flow tests
The principle of LFT relies on detecting the pathogen by utilizing a capillary force to flow the sample across the membrane and gather labelling molecules on the embedded capturing antibody. LFT was used primarily for pathogen detection192 in food,193 and it was commercialized for the detection of SARS viruses,194 rotaviruses,195 streptococci, microbes like Bacillus species,196 and meat species.197,198 However, LFT is simple, easy to use, and requires a low cost to manufacture; it cannot perform complex laboratory procedures and requires high accuracy assays such as nucleic acid tests.35 Therefore, significant effort has been focused on integrating signal amplification sensitivity with the rapid and facile sensing modality of LFT. In this regard, a CD-based microfluidic process was utilized to develop separate LFTs that could detect the LAMP amplicon of the H1N1 influenza virus by amplifying H1 and M genes.199
Each microchip consists of an inlet to inject the LAMP reagent into an amplification chamber. A zigzag-shaped micro dispenser channel was allocated to LAMP solution equally in three different compartments. 2 μL of the sample was injected into the inlet. By leveraging centrifuge speeds and amplification times, the LAMP amplicon was detected with a sensitivity of 10 copies per sample (7 μL samples). In one study, Rohrman et al. integrated RPA with LFT to detect 10 copies of HIV DNA samples in 15 minutes (Fig. 3A).200 The amplicon was detected using LFT of single bacteria detection201 (Fig. 3B). In general, the LAMP amplification method has been employed more frequently than other isothermal amplification methods to develop POC devices for pathogen detection.44
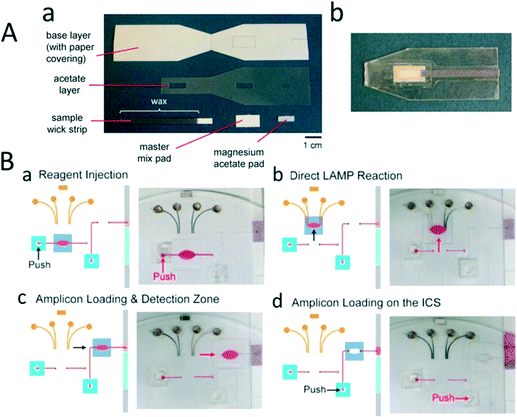 |
| Fig. 3 Lateral flow test for nucleic acid amplification. (A) Lateral flow test strip for detection of the recombinase polymerase amplification product. (a) This consists of a base layer, an acetate layer to isolate different regions, and two pads to apply the master mix and magnesium acetate. The sample was added to the master mix-pad and, after amplification, was detected with a lateral flow strip. (b) Image of the integrated RPA paper chip. (B) Integrated LAMP and LFT in sliding chambers. (a) LAMP reagents were loaded into the reaction chamber by pressing the releasing reservoir with a finger and (b) the chamber slider to perform the LAMP reaction. (c) The chamber was sided into the detection region, and (d) the sample was pushed to load the amplicon on lateral chromatography strips (LCS). Reproduced with permission from ref. 200 and ref. 201. | |
6.2. Incorporation of the screen-printed electrode (SPE) in various biosensor devices
Several platforms have been developed based on screen-printed technology,202 which allows for POC diagnostic application for pathogen detection and quantification. For instance, screen-printed electrode (SPE) technologies are widely used in POC devices to perform electrochemical sensing modalities of DNA amplification products by inter-chelating redox molecules with DNA amplicons35,202,203 (Fig. 4A). In a study by Safavieh et al., a microfluidic chip was developed to detect and quantify E. coli bacteria with a LOD of 38 CFU mL−1 within 35 minutes. In another effort, a portable roll to roll cassette204,205 device was used to detect and quantify pathogens, E. coli and S.aureus,206,207 with a sensitivity of 30 CFU mL−1 and 200 CFU mL−1, respectively. In a closely similar study, Tolba et al. leveraged bacteriophage-encoded peptidoglycan hydrolases to capture and detect Listeria on a gold SPE based on EIS. The LOD was recorded to be 1.1 × 104 and 105 CFU mL−1 in bacteria samples of pure culture and 2% spiked milk, respectively.208 In addition, the SPE has been widely employed to detect toxins and antigens from pathogens.209 Viruses have also been detected using SPE-based electrical sensing techniques. For example, anti-gp-120 was immobilized on the surface of a paper microchip, and 108 copies mL−1 of HIV was detected with an impedance magnitude of 1 kHz frequency210 (Fig. 4B).
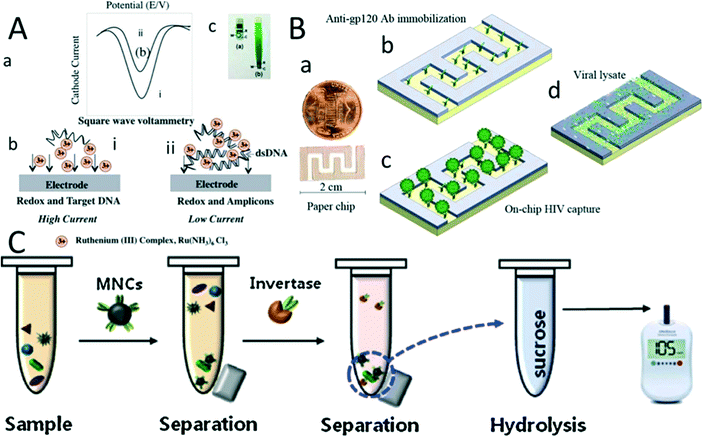 |
| Fig. 4 Pathogen detection on screen-printed electrode chips. (A) Detection of a real-time LAMP-amplicon on a carbon SPE chip using ruthenium hexamine redox. (a) SWV for detection of the LAMP product in (i) the absence and (ii) the presence of a double-stranded DNA amplicon. (b) Schematic of electrochemical sensing modality for LAMP amplicon detection using ruthenium hexamine redox. (c) Image of the carbon screen-printed electrode. (B) (a) Graphene modified silver electrode paper microchip for detection of HIV-1 virus. (b) Anti gp-120 was immobilized on the surface of the paper chip. (c) HIV-1 viruses were captured on the chip. (d) The virus was lysed, and the nano-lysate was measured to detect the virus using impedance spectroscopy. (C) Salmonella bacteria detection using a glucose meter. Antibody conjugated with magnetic beads was used to capture Salmonella in the milk sample. Then it was separated by a magnet. The sample was conjugated with invertase and dispersed in sucrose. The conjugated antibody–bacteria invertase complex hydrolyses sucrose into glucose, which can be monitored by a glucose meter. Reproduced with permission from ref. 210 and ref. 211. | |
A glucose meter is another primary device that can detect microbial pathogens. The low-cost and disposable SPE and rapid electrochemical sensing modality make the glucose meter an appealing technology as a POC diagnostic device. The principle of this device is based on the functionalization of antibodies with invertases. These antibodies are conjugated with the pathogens and then dispersed in a sucrose solution. The hydrolysis of sucrose by invertase releases glucose into the solution. This is found to be proportional to the concentration of the pathogens and can be monitored by a glucose meter212,213 (Fig. 4C). A glucose meter detects various microbial pathogens like Salmonella enterica spiked in milk with a LOD of 10 CFU mL−211. With a similar approach, E. coli in a potable water sample is detected with a lactose substrate, achieving a LOD of 2 × 107 CFU mL−1. E. coli bacteria produce β-galactosidase that transforms lactose into glucose, which is detected by the glucose meter.
6.3. Utilization of smartphones
With the advancements in technology, smartphones can now be integrated with various sensing modalities and can be easily implemented in pathogen detection. Smartphones can record sensing patterns of optical, fluorescence, colorimetric, and electrochemical/electrical techniques to detect bio-analytes214 using their features like high-resolution cameras, facile video documenting systems, and integrated applications with portable electrochemical transducers. Various POC platforms based on smartphones for pathogen detection and their analytical performances are summarised in Table 4.
Table 4 Portable POC diagnostic devices integrated with smartphones for pathogen detection
Sensing modality |
Bio-analyte |
Limit of detection |
Detection range |
Ref. |
Fluorescence microscopy |
HCV |
Single copy virus |
NA |
215
|
Fluorescence microscopy |
E.coli/salmonella |
101 CFU ml−1E. coli102 CFU ml−1Salmonella |
101–108/102–107 CFU mL−1 |
215
|
Fluorescence microscopy with qubit dye |
Salmonella typhimurium
|
103 CFU ml−1 |
NA |
216
|
Mie scattering |
E. coli
|
101 CFU ml−1 |
101–108 CFU ml−1 |
217
|
Bright field microscopy |
P. falciparum/sickle cell |
>1.2 um |
NA |
218
|
Colorimetric lens less |
HCV |
Single copy of virus |
NA |
219
|
Fluorescent optical detection |
E. coli, S. aureus |
10 fg DNA |
NA |
220
|
Fluorescence microscopy |
HSV-1 and HSV-2 |
>99% |
NA |
221
|
Microfluidic LAMP analysis |
E. coli, V. parahaemolyticus, S. typhimurium |
101 copies μL−1 |
NA |
222
|
LAMP assay |
E. canis, H. canis |
10−6 dilution limit |
NA |
223
|
smaRT-LAMP analysis and qPCR |
Salmonella Typhimurium, S. enteritidis, Escherichia coli, Yersinia pseudotuberculosis, Klebsiella pneumoniae, Pseudomonas aeruginosa, S. aureus, S. pneumoniae |
≤10 CFU mL−1 |
5 × 103–1 × 105 CFU ml−1 (2–40 CFU per reaction) |
224
|
Darkfield imaging |
E. coli B strain and T2 bacteriophage |
8 × 104 (CFU) per microdevice |
NA |
225
|
Shafiee et al. detected E. coli bacteria conjugated with AuNPs on a paper chip using a smartphone (Fig. 5A).226 Techniques like Mie scattering have also been used to detect bacteria in real beef samples217 (Fig. 5B). In another study, a valveless microfluidic device was fabricated via the hot embossing method to detect genes of stx2 and eaeA from E. coli and mecA and vicK of S. aureus by using optical fluorescence techniques (Fig. 5C).220 Rodriguez-Manzano et al.219 have developed a single-molecule detection method using LAMP and colorimetric dye, hydroxy naphthol blue (HNB). A sample consisting of HCV nucleic acid was compartmentalized in each reservoir of SlipChip.227 After amplifying each well, the positive results were recorded and monitored using an unmodified cell phone camera (Fig. 5D and E). In another effort, Berg et al.221 developed a 96 well plate ELISA reader using an LED array. The light was then collected using an optical fibre to monitor HIV-1 and HSV-2 viruses with 99.4% and 99.4% accuracy, respectively. Electrochemical sensing modality, thus, has been widely integrated with smartphones. These strategies are highly applied in many clinical diagnostic applications and pave a path towards more modernistic and unique POC devices.
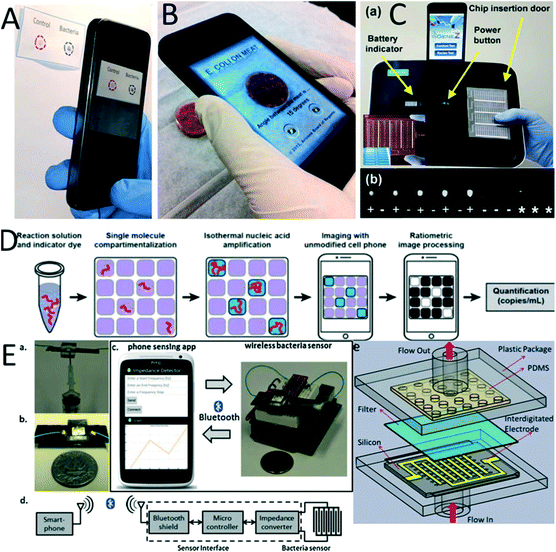 |
| Fig. 5 Smartphone-based POC platforms for pathogen detection. (A) Paper chip for detection of E. coli with a gold nanoparticle conjugated recognition element. (B) Detection of E. coli in a meat sample utilizing Mie scattering with a smartphone. (C) Microfluidic chip for nucleic acid detection using LAMP and optical sensing modality. (D) SlipChip platform integrated with a cell phone to detect single HCV RNA with no additional lenses. (E) Portable impedance meter for the detection of bacteria. (a and b) Image of a microchip where the sample is injected into the chip using a syringe. (c) A communication sensing platform that transfers signals into the smartphone. (d) Schematic of wireless sensing. (e) Schematic of the microfluidic chip configuration. The sample was injected at the bottom, and bacteria filtered on the top of the interdigitated electrode for EIS detection. Reproduced with permission from ref. 217, 219 and 220. | |
7. Conclusions and future trends
In recent years research development in POC diagnostic platforms has shown many advantages in their miniaturization process, nanotechnologies involved, genomics and proteomics studied, and microfluidics used. In addition, POC platforms possess simple test procedures, demonstrate fast analysis time, are readily available for everyone, do not require skilled professionals or high-end instruments, are inexpensive, and require a low sample volume and fewer reagents. POC diagnostics can be performed easily in remote areas without any big investments of laboratory facilities. Moreover, integrating nanotechnology and nanomaterials with biosensors has revolutionized diagnostic and therapeutic applications like microbe detection. The application of novel NPs and NMs in biosensors has resulted in an enhanced signal to noise ratio and reduction in time taken for sensing compared to conventional processes; therefore, it bears excellent potential for POC testing devices.
Despite the booming advantages of POC diagnostic devices, there are still a few challenges that need to be addressed. The image-processing applications on biosensing devices like optical sensors imply them to be facile. However, they do not require an additional integrated appliance, which leads to next-generation applications for affordable POC diagnostic devices that can be monitored online. Under further advanced and developed laboratories, researchers use cloud-based biosensing analysis where data does not need to be stored in an external device. Instead it is directly stored in the cloud for easy accessibility. Therefore, on-spot detection with POC analysis can become user-friendly if the diagnostic results are readily available to the general public online.
Techniques like fluorescent detection that requires light excitation sources can be replaced with colorimetric dyes that do not require any light source for fluorescent excitation. Also, electrochemical and electrical sensing modalities can be integrated with smartphones by designing amperometric, voltammetric, and EIS circuits. However, in all these techniques, wire communication and cloud-based analysis are mandatory and therefore limit mobility. Instead, wireless signal analysis can be used which instantaneously records data and reduces data storage problems. Thus, data communication has opened a new paradigm to develop personalized POC monitoring devices to detect pathogens that cause most infectious diseases.
To further improve such biosensing devices that can detect bacteria, fungi, toxins, and viruses from food, water, air, the following points need to be considered:
• More work needs to be done to improve the modified matrix in biosensors that attach to bio-recognition molecules.
• Various conducting surfaces made of polymers and conductive materials must be further analyzed to obtain better outcome signals in POC devices.
• For advanced detection of toxins, more bacterial species need to be explored so that they can act as biorecognition molecules with better accuracy.
• More biosensors need to be explored and developed such they can be used as on-site detection devices.
• The low LOD and wide range of detection limits make biosensors a very appealing technology to detect various pathogens.
• The biosensing prototypes discussed in previous sections are extremely useful and promising to solve many long-term health complications and environmental problems.
• With the increasing demands of market investment in research and development, researchers will lead to a successful path to make POC devices faster, simpler, and cheaper for the benefit of the people and society.
Funding
Minhaz Uddin Ahmed would like to acknowledge the financial support for the project provided by Brunei Research Council (Grant# BRC-10) from the Economic and Planning Development, Prime Minister's Office of Negara Brunei Darussalam. Mohammadali Safavieh wishes to thank the National Science Engineering Research Council of Canada for the financial support through NSERC Postdoctoral fellow.
Author contributions
Sharmili Roy, Fareeha Arshad, Minhaz Uddin Ahmed, and Shimaa Eissa wrote the parts related to nanomaterials and sensing parts. Mohammadali Safavieh and Minhaz Uddin Ahmed wrote the parts related to microfluidics and LOC. Sanaa G. Alattas, Minhaz Uddin Ahmed, and Mohammed Zourob wrote the parts related to the recognition receptors. Minhaz Uddin Ahmed and Mohammed Zourob proofread the review article.
Conflicts of interest
The authors report no declarations of interest.
References
- M. Zarei, Biosens. Bioelectron., 2018, 106, 193–203 CrossRef CAS PubMed.
- M. Pohanka, Int. J. Anal. Chem., 2021, 2021, 1–9 CrossRef.
- O. Tokel, U. H. Yildiz, F. Inci, N. G. Durmus, O. O. Ekiz, B. Turker, C. Cetin, S. Rao, K. Sridhar, N. Natarajan, H. Shafiee, A. Dana and U. Demirci, Sci. Rep., 2015, 5(1), 9152 CrossRef CAS PubMed.
- L. Zheng, G. Cai, S. Wang, M. Liao, Y. Li and J. Lin, Biosens. Bioelectron., 2019, 124–125, 143–149 CrossRef CAS PubMed.
- T. Nguyen, V. A. Chidambara, S. Z. Andreasen, M. Golabi, V. N. Huynh, Q. T. Linh, D. D. Bang and A. Wolff, TrAC, Trends Anal. Chem., 2020, 131, 116004 CrossRef CAS.
- M. Vouga and G. Greub, Clin. Microbiol. Infect., 2016, 22(1), 12–21 CrossRef PubMed.
- M. Georgieva, A. Leeson-Payne, M. Dumitrascuta, A. Rajnicek, M. Malcangio, W. Huang and J. Neurosci, Methods, 2018, 304, 92–102 Search PubMed.
- K. B. Mullis, Sci. Am., 1990, 262(4), 56–61 CrossRef CAS PubMed , 64-5.
- A. E. Katz, C. A. Olsson, A. J. Raffo, C. Cama, H. Perlman, E. Seaman, K. M. O'Toole, D. McMahon, M. C. Benson and R. Buttyan, Urology, 1994, 43(6), 765–775 CrossRef CAS PubMed.
- J. H. Schefe, K. E. Lehmann, I. R. Buschmann, T. Unger and H. Funke-Kaiser, J. Mol. Med., 2006, 84(11), 901–910 CrossRef CAS PubMed.
- P. G. Lantz, B. Hahn-Hägerdal and P. Rådström, Trends Food Sci. Technol., 1994, 5(12), 384–389 CrossRef CAS.
-
S. Roy and A. Baranwal, Diagnostic Strategies for COVID-19 and Other Coronaviruses, 2020, pp. 135–139 Search PubMed.
-
S. Roy and A. Ramadoss, Environmental and Health Management of Novel Coronavirus Disease (COVID-19), 2021, pp. 3–39 Search PubMed.
-
M. G. Dastidar and S. Roy, Environmental and Health Management of Novel Coronavirus Disease (COVID-19), 2021, pp. 345–378 Search PubMed.
- N. S. Sampath Kumar, A. D. Chintagunta, S. P. Jeevan Kumar, S. Roy and M. Kumar, 3 Biotech, 2020, 10(12), 527 CrossRef CAS PubMed.
- S. L. Nightingale, J. M. Prasher and S. Simonson, Emerging Infect. Dis., 2007, 13(7), 1046–1051 CrossRef PubMed.
- H. Zhu, H. Zhang, S. Ni, M. Korabečná, L. Yobas and P. Neuzil, TrAC, Trends Anal. Chem., 2020, 130, 115984 CrossRef CAS PubMed.
-
J. R. Crowther, ELISA, 2003, vol. 42, pp. 1–218 Search PubMed.
- B. K. Kumar, P. Raghunath, D. Devegowda, V. K. Deekshit, M. N. Venugopal and I. Karunasagar, Int. J. Food Microbiol., 2011, 145(1), 244–249 CrossRef CAS PubMed.
- F. J. Bolton, E. Fritz, S. Poynton and T. Jensen, J. AOAC Int., 2000, 83(2), 299–303 CrossRef CAS PubMed.
-
S. Hosseini, P. Vázquez-Villegas, M. Rito-Palomares and S. O. Martinez-Chapa, SpringerBriefs in Applied Sciences and Technology, 2018, pp. 67–115 Search PubMed.
- L. Wu, G. Li, X. Xu, L. Zhu, R. Huang and X. Chen, TrAC, Trends Anal. Chem., 2019, 113, 140–156 CrossRef CAS.
- M. Severgnini, P. Cremonesi, C. Consolandi, G. De Bellis and B. Castiglioni, Food Bioprocess Technol., 2011, 4, 936–953 CrossRef.
- N. V. Zaytseva, V. N. Goral, R. A. Montagna and A. J. Baeumner, Lab Chip, 2005, 5(8), 805–811 RSC.
- D. Wang, L. Coscoy, M. Zylberberg, P. C. Avila, H. A. Boushey, D. Ganem and J. L. DeRisi, Proc. Natl. Acad. Sci. U. S. A., 2002, 99(24), 15687–15692 CrossRef CAS PubMed.
- B. Spiess, W. Seifarth, M. Hummel, O. Frank, A. Fabarius, C. Zheng, H. Mörz, R. Hehlmann and D. Buchheidt, J. Clin. Microbiol., 2007, 45(11), 3743–3753 CrossRef CAS PubMed.
- G. J. Vora, C. E. Meador, D. A. Stenger and J. D. Andreadis, Appl. Environ. Microbiol., 2004, 70(5), 3047–3054 CrossRef CAS PubMed.
-
S. Roy, S. J. Malode, N. P. Shetti and P. Chandra, Bioelectrochemical Interface Engineering, 2019, pp. 325–342 Search PubMed.
-
A. Kumar, S. Roy, A. Srivastava, M. M. Naikwade, B. Purohit, K. Mahato, V. G. M. Naidu and P. Chandra, Nanotechnology in Modern Animal Biotechnology, 2019, pp. 149–161 Search PubMed.
- C. Ziegler and W. Göpel, Curr. Opin. Chem. Biol., 1998, 2(5), 585–591 CrossRef CAS PubMed.
- V. Velusamy, K. Arshak, O. Korostynska, K. Oliwa and C. Adley, Biotechnol. Adv., 2010, 28(2), 232–254 CrossRef CAS PubMed.
- A. G. Gehring and S. I. Tu, Annu. Rev. Anal. Chem., 2011, 4, 151–172 CrossRef CAS PubMed.
-
N. P. Shetti, S. J. Malode, S. Roy, P. Chandra, K. R. Reddy and S. Chatterjee, Nanomaterials in Diagnostic Tools and Devices, 2020, pp. 293–329 Search PubMed.
- Y. Shang, J. Sun, Y. Ye, J. Zhang, Y. Zhang and X. Sun, Crit. Rev. Food Sci. Nutr., 2020, 60(2), 201–224 CrossRef CAS PubMed.
- S. Roy, I. A. Rahman, J. H. Santos and M. U. Ahmed, Food Control, 2016, 61, 70–78 CrossRef CAS.
-
S. Roy, M. M. Hossain, M. Safavieh, H. N. Lubis, M. Zourob and M. U. Ahmed, Food Chemistry, Function and Analysis, 2017, pp. 367–392 Search PubMed.
- M. Vincent, Y. Xu and H. Kong, EMBO Rep., 2004, 5(8), 795–800 CrossRef CAS PubMed.
- C. Shi, Q. Liu, C. Ma and W. Zhong, Anal. Chem., 2014, 86(1), 336–339 CrossRef CAS PubMed.
- Y. Zhou, J. Xiao, X. Ma, Q. Wang and Y. Zhang, Appl. Microbiol. Biotechnol., 2018, 102(12), 5299–5308 CrossRef CAS PubMed.
- M. J. Hall, S. D. Wharam, A. Weston, D. L. N. Cardy and W. H. Wilson, BioTechniques, 2002, 32(3), 604–606 CrossRef CAS PubMed , 608–611.
- N. F. N. Azam, S. Roy, S. A. Lim and M. Uddin Ahmed, Food Chem., 2018, 248, 29–36 CrossRef CAS PubMed.
- H. Munirah, S. Roy, J. L. Z. Ying, I. A. Rahman and M. U. Ahmed, Sci. Bruneiana, 2016, 15 Search PubMed.
- S. Roy, S. X. Wei, I. A. Rahman and M. U. Ahmed, Malays. J. Microbiol., 2016, 5, 332–338 Search PubMed.
- M. Safavieh, M. K. Kanakasabapathy, F. Tarlan, M. U. Ahmed, M. Zourob, W. Asghar and H. Shafiee, ACS Biomater. Sci. Eng., 2016, 2(3), 278–294 CrossRef CAS PubMed.
- S. Roy, N. F. Mohd-Naim, M. Safavieh and M. U. Ahmed, ACS Sens., 2017, 2(11), 1713–1720 CrossRef CAS PubMed.
- Y. Fu, X. Zhou, X. Duan, C. Liu, J. Huang, T. Zhang, S. Ding and X. Min, Sens. Actuators, B, 2020, 321, 128502 CrossRef CAS.
- J. W. F. Law, N. S. A. Mutalib, K. G. Chan and L. H. Lee, Front. Microbiol., 2015, 5, 770 Search PubMed.
- M. Di Fabrizio, S. Lupi and A. D'Arco, JPhys Photonics, 2021, 3(3), 032001 CrossRef.
- I. Banerjee, T. Salih, H. Ramachandraiah, J. Erlandsson, T. Pettersson, A. C. Araújo, M. Karlsson and A. Russom, RSC Adv., 2017, 7(56), 35048–35054 RSC.
- K. Kant, M. A. Shahbazi, V. P. Dave, T. A. Ngo, V. A. Chidambara, L. Q. Than, D. D. Bang and A. Wolff, Biotechnol. Adv., 2018, 36(4), 1003–1024 CrossRef CAS PubMed.
- J. Hwang, S. Lee and J. Choo, Nanoscale, 2016, 8(22), 11418–11425 RSC.
-
B. Purohit, A. Kumar, K. Mahato, S. Roy and P. Chandra, Nanotechnology in Modern Animal Biotechnology: Concepts and Applications, 2019, pp. 133–147 Search PubMed.
- E. H. Chowdhury and T. Akaike, Biotechnol. Bioeng., 2005, 90(4), 414–421 CrossRef CAS PubMed.
- Y. Alhamoud, D. Yang, S. S. Fiati Kenston, G. Liu, L. Liu, H. Zhou, F. Ahmed and J. Zhao, Biosens. Bioelectron., 2019, 141, 111418 CrossRef CAS PubMed.
- S. Schlafer and R. L. Meyer, J. Microbiol. Methods, 2017, 138, 50–59 CrossRef PubMed.
- J. Wang and S. T. Yau, Anal. Methods, 2014, 4(14), 5387–5391 RSC.
- M. Xu, R. Wang and Y. Li, Talanta, 2016, 148, 200–208 CrossRef CAS PubMed.
- N. Singh, V. Sreenivas, A. Sheoran, S. Sharma, K. B. Gupta, G. K. Khuller and P. K. Mehta, J. Microbiol. Methods, 2016, 120, 56–64 CrossRef CAS PubMed.
- J. Lum, R. Wang, K. Lassiter, B. Srinivasan, D. Abi-Ghanem, L. Berghman, B. Hargis, S. Tung, H. Lu and Y. Li, Biosens. Bioelectron., 2012, 38(1), 67–73 CrossRef CAS PubMed.
- A. Bhimji, A. A. Zaragoza, L. S. Live and S. O. Kelley, Anal. Chem., 2013, 85(14), 6813–6819 CrossRef CAS PubMed.
- M. F. M. Fathil, M. K. Md Arshad, S. C. B. Gopinath, U. Hashim, R. Adzhri, R. M. Ayub, A. R. Ruslinda, M. Nuzaihan, A. H. Azman, M. Zaki and T. H. Tang, Biosens. Bioelectron., 2015, 70, 209–220 CrossRef CAS PubMed.
- K. H. Kwon, S. Y. Hwang, Y. K. Park, J. W. Yoon, S. Kim and J. Hong, J. Food Saf., 2014, 34(3), 249–256 CrossRef CAS.
- X. He, L. Zhang, P. Liu, L. Liu, H. Deng and J. Huang, Immunol. Res., 2015, 61(3), 281–293 CrossRef CAS PubMed.
- S. K. Pandey, C. R. Suri, M. Chaudhry, R. P. Tiwari and P. Rishi, Mol. BioSyst., 2012, 8(7), 1853–1860 RSC.
- B. Tian, R. S. Bejhed, P. Svedlindh and M. Strömberg, Biosens. Bioelectron., 2016, 77, 32–39 CrossRef CAS PubMed.
- I. H. Cho and J. Irudayaraj, Anal. Bioanal. Chem., 2013, 405(10), 3313–3319 CrossRef CAS PubMed.
- M. Anese, F. Bot, A. Panozzo, G. Mirolo and G. Lippe, Food Chem., 2015, 172, 685–691 CrossRef CAS PubMed.
- P. Bouvrette and J. H. T. Luong, Int. J. Food Microbiol., 1995, 27(2–3), 129–137 CrossRef CAS PubMed.
- G. Zhao, X. Zhan and W. Dou, Anal. Biochem., 2011, 408(1), 53–58 CrossRef CAS PubMed.
- T. Matsushita, N. Shirasaki, Y. Tatsuki and Y. Matsui, Water Res., 2013, 47(15), 5819–5827 CrossRef CAS PubMed.
- A. Thongprachum, P. Khamrin, N. Chaimongkol, R. Malasao, S. Okitsu, M. Mizuguchi, N. Maneekarn and H. Ushijima, J. Med. Virol., 2010, 82(12), 2106–2109 CrossRef PubMed.
- Y. S. Fang, S. Y. Chen, X. J. Huang, L. S. Wang, H. Y. Wang and J. F. Wang, Biosens. Bioelectron., 2014, 53, 238–244 CrossRef CAS PubMed.
- C. L. Morgan, D. J. Newman and C. P. Price, Clin. Chem., 1996, 42(2), 193–209 CrossRef CAS.
- S. Jain and R. Gulati, Natl. J. Maxillofac. Surg., 2011, 2(1), 33–37 CrossRef PubMed.
- A. Rajkovic, B. El Moualij, Y. Fikri, K. Dierick, W. Zorzi, E. Heinen, A. Uner and M. Uyttendaele, Food Anal. Methods, 2012, 5(3), 319–326 CrossRef.
- V. A. Petrenko and G. P. Smith, Protein Eng., 2000, 13(8), 589–592 CrossRef CAS PubMed.
- J. Brigati, D. D. Williams, I. B. Sorokulova, V. Nanduri, I. H. Chen, C. L. Turnbough and V. A. Petrenko, Clin. Chem., 2004, 50(10), 1899–1906 CrossRef CAS PubMed.
- A. Hayhurst, S. Happe, R. Mabry, Z. Koch, B. L. Iverson and G. Georgiou, J. Immunol. Methods, 2003, 276(1–2), 185–196 CrossRef CAS PubMed.
- L. J. Sherwood, L. E. Osborn, R. Carrion, J. L. Patterson and A. Hayhurst, J. Infect. Dis., 2007, 196(Suppl 2), S213–S219 CrossRef CAS PubMed.
- E. Goldman and S. Walper, Methods Mol. Biol., 2014, 1108, 201–210 CrossRef CAS PubMed.
- E. R. Goldman, J. L. Liu, R. D. Bernstein, M. D. Swain, S. Q. Mitchell and G. P. Anderson, Sensors, 2009, 9(1), 542–555 CrossRef CAS PubMed.
- T. Ktari, H. Baccar, M. B. Mejri and A. Abdelghani, Int. J. Electrochem., 2012, 2012(8), 421692 Search PubMed.
- S. K. Arya, A. Singh, R. Naidoo, P. Wu, M. T. McDermott and S. Evoy, Analyst, 2011, 136(3), 486–492 RSC.
- N. Tawil, E. Sacher, R. Mandeville and M. Meunier, Biosens. Bioelectron., 2012, 37(1), 24–29 CrossRef CAS PubMed.
- M. Smietana, W. J. Bock, P. Mikulic, A. Ng, R. Chinnappan and M. Zourob, Opt. Express, 2011, 19(9), 7971–7978 CrossRef CAS PubMed.
- S. M. Tripathi, W. J. Bock, P. Mikulic, R. Chinnappan, A. Ng, M. Tolba and M. Zourob, Biosens. Bioelectron., 2012, 35(1), 308–312 CrossRef CAS PubMed.
- A. Mazhorova, A. Markov, A. Ng, R. Chinnappan, O. Skorobogata, M. Zourob and M. Skorobogatiy, Opt. Express, 2012, 20(5), 5344–5355 CrossRef PubMed.
- J. Wang, Biosens. Bioelectron., 2006, 21(10), 1887–1892 CrossRef CAS PubMed.
- T. Neufeld, A. Schwartz-Mittelmann, D. Biran, E. Z. Ron and J. Rishpon, Anal. Chem., 2003, 75(3), 580–585 CrossRef CAS PubMed.
- O. Laczka, C. García-Aljaro, F. J. del Campo, F. X. M. Pascual, J. Mas-Gordi and E. Baldrich, Anal. Chim. Acta, 2010, 677(2), 156–161 CrossRef CAS PubMed.
- M. Yemini, Y. Levi, E. Yagil and J. Rishpon, Bioelectrochemistry, 2007, 70(1), 180–184 CrossRef CAS PubMed.
- A. S. Mittelmann, E. Z. Ron and J. Rishpon, Anal. Chem., 2002, 74(4), 903–907 CrossRef CAS PubMed.
- A. Leung, P. M. Shankar and R. Mutharasan, Sens. Actuators, B, 2007, 125(2), 688–703 CrossRef CAS.
- A. Shabani, M. Zourob, B. Allain, C. A. Marquette, M. F. Lawrence and R. Mandeville, Anal. Chem., 2008, 80(24), 9475–9482 CrossRef CAS PubMed.
- A. Shabani, C. A. Marquette, R. Mandeville and M. F. Lawrence, Talanta, 2013, 116, 1047–1053 CrossRef CAS PubMed.
- V. Nanduri, I. B. Sorokulova, A. M. Samoylov, A. L. Simonian, V. A. Petrenko and V. Vodyanoy, Biosens. Bioelectron., 2007, 22(6), 986–992 CrossRef CAS PubMed.
- E. V. Olsen, I. B. Sorokulova, V. A. Petrenko, I. H. Chen, J. M. Barbaree and V. J. Vodyanoy, Biosens. Bioelectron., 2006, 21(8), 1434–1442 CrossRef CAS PubMed.
- R. S. Lakshmanan, R. Guntupalli, J. Hu, V. A. Petrenko, J. M. Barbaree and B. A. Chin, Sens. Actuators, B, 2007, 126(2), 544–550 CrossRef CAS.
- M. K. Park, J. W. Park, H. C. Wikle and B. A. Chin, Sens. Actuators, B, 2013, 176, 1134–1140 CrossRef CAS.
- S. Li, Y. Li, H. Chen, S. Horikawa, W. Shen, A. Simonian and B. A. Chin, Biosens. Bioelectron., 2010, 26(4), 1313–1319 CrossRef CAS PubMed.
- S. M. Usman Ali, O. Nur, M. Willander and B. Danielsson, Sens. Actuators, B, 2010, 145(2), 869–874 CrossRef CAS.
- N. Bhardwaj, S. K. Bhardwaj, J. Mehta, G. C. Mohanta and A. Deep, Anal. Biochem., 2016, 505, 18–25 CrossRef CAS PubMed.
- J. Wan, M. L. Johnson, R. Guntupalli, V. A. Petrenko and B. A. Chin, Sens. Actuators, B, 2007, 127(2), 559–566 CrossRef CAS.
- L. Fu, S. Li, K. Zhang, I. H. Chen, J. M. Barbaree, A. Zhang and Z. Cheng, IEEE Sens. J., 2011, 11(8), 1684–1691 Search PubMed.
- S. S. Sekhon, S. G. Kim, S. H. Lee, A. Jang, J. Min, J. Y. Ahn and Y. H. Kim, Mol. Cell. Toxicol., 2013, 9(4), 311–317 CrossRef CAS.
- C. Tuerk and L. Gold, Science, 1990, 249(4968), 505–510 CrossRef CAS PubMed.
- R. Green, A. D. Ellington and J. W. Szostak, Nature, 1990, 347(6291), 406–408 CrossRef CAS PubMed.
- D. O. Demirkol and S. Timur, Int. J. Polym. Mater. Polym. Biomater., 2016, 65(2), 85–90 CrossRef CAS.
- J. Khang, D. Kim, K. W. Chung and J. H. Lee, Talanta, 2016, 147, 177–183 CrossRef CAS PubMed.
- R. B. Queirós, N. De-Los-Santos-Álvarez, J. P. Noronha and M. G. F. Sales, Sens. Actuators, B, 2013, 181, 766–772 CrossRef.
- R. B. Queirós, C. Gouveia, J. R. A. Fernandes and P. A. S. Jorge, Biosens. Bioelectron., 2014, 62, 227–233 CrossRef PubMed.
- R. Aimaiti, L. Qin, T. Cao, H. Yang, J. Wang, J. Lu, X. Huang and Z. Hu, Appl. Microbiol. Biotechnol., 2015, 99(21), 9073–9083 CrossRef CAS PubMed.
- F. He, Y. Xiong, J. Liu, F. Tong and D. Yan, Biosens. Bioelectron., 2016, 77, 799–804 CrossRef CAS PubMed.
- X. L. Tang, Y. X. Zhou, S. M. Wu, Q. Pan, B. Xia and X. L. Zhang, J. Infect., 2014, 69(6), 569–580 CrossRef PubMed.
- I. Shiratori, J. Akitomi, D. A. Boltz, K. Horii, M. Furuichi and I. Waga, Biochem. Biophys. Res. Commun., 2014, 443(1), 37–41 CrossRef CAS PubMed.
-
M. F. Fatin, A. R. Ruslinda, M. K. Md Arshad, S. Norhafizah, M. A. Farehanim, R. M. Ayub and U. Hashim, IECBES 2014, Conference Proceedings - 2014 IEEE Conference on Biomedical Engineering and Sciences: ‘Miri, Where Engineering in Medicine and Biology and Humanity Meet’, 2014 Search PubMed.
- A. Rahim Ruslinda, K. Tanabe, S. Ibori, X. Wang and H. Kawarada, Biosens. Bioelectron., 2013, 40(1), 277–282 CrossRef CAS PubMed.
- E. Herrera-Carrillo and B. Berkhout, Adv. Exp. Med. Biol., 2015, 848, 71–95 CrossRef CAS PubMed.
- A. Abbaspour, F. Norouz-Sarvestani, A. Noori and N. Soltani, Biosens. Bioelectron., 2015, 68, 149–155 CrossRef CAS PubMed.
- A. Baumstummler, D. Lehmann, N. Janjic and U. A. Ochsner, Lett. Appl. Microbiol., 2014, 59(4), 422–431 CrossRef CAS PubMed.
- Y. Huang, X. Chen, N. Duan, S. Wu, Z. Wang, X. Wei and Y. Wang, Food Chem., 2015, 166, 623–629 CrossRef CAS PubMed.
- Y. Lian, F. He, H. Wang and F. Tong, Biosens. Bioelectron., 2015, 65, 314–319 CrossRef CAS PubMed.
- J. Shangguan, Y. Li, D. He, X. He, K. Wang, Z. Zou and H. Shi, Analyst, 2015, 140(13), 4489–4497 RSC.
- J. J. Clarkson, S. Dodsworth and M. W. Chase, Plant Syst. Evol., 2017, 303(8), 1001–1012 CrossRef.
- X. Ma, Y. Jiang, F. Jia, Y. Yu, J. Chen and Z. Wang, J. Microbiol. Methods, 2014, 98, 94–98 CrossRef CAS PubMed.
- S. H. Lee, J. Y. Ahn, K. A. Lee, H. J. Um, S. S. Sekhon, T. Sun Park, J. Min and Y. H. Kim, Biosens. Bioelectron., 2015, 68, 272–280 CrossRef CAS PubMed.
- D. S. Ediriweera, A. Kasturiratne, A. Pathmeswaran, N. K. Gunawardena, B. A. Wijayawickrama, S. F. Jayamanne, G. K. Isbister, A. Dawson, E. Giorgi, P. J. Diggle, D. G. Lalloo and H. J. de Silva, PLoS Neglected Trop. Dis., 2016, 10(7), e0004813 CrossRef PubMed.
- N. Duan, X. Ding, S. Wu, Y. Xia, X. Ma, Z. Wang and J. Chen, J. Microbiol. Methods, 2013, 94(3), 170–174 CrossRef CAS PubMed.
- R. Sharma, V. V. Agrawal, P. Sharma, R. Varshney, R. K. Sinha and B. D. Malhotra, J. Phys.: Conf. Ser., 2012, 358(1), 012001 CrossRef.
- K. J. Huang, Y. J. Liu, G. W. Shi, X. R. Yang and Y. M. Liu, Sens. Actuators, B, 2014, 201, 579–585 CrossRef CAS.
- J. Dong, H. Zhao, M. Xu, Q. Maa and S. Ai, Food Chem., 2013, 141(3), 1980–1986 CrossRef CAS PubMed.
- H. Zhou, D. Yang, N. P. Ivleva, N. E. Mircescu, R. Niessner and C. Haisch, Anal. Chem., 2014, 86(3), 1525–1533 CrossRef CAS PubMed.
- V. K. Gasparyan and I. L. Bazukyan, Anal. Chim. Acta, 2013, 766, 83–87 CrossRef CAS PubMed.
- H. C. Wu, Y. L. Huang, S. C. Lai, Y. Y. Huang and M. F. Shaio, Lett. Appl. Microbiol., 2001, 32(5), 321–325 CrossRef CAS PubMed.
- A. Mokhtarzadeh, R. Eivazzadeh-Keihan, P. Pashazadeh, M. Hejazi, N. Gharaatifar, M. Hasanzadeh, B. Baradaran and M. de la Guardia, TrAC, Trends Anal. Chem., 2017, 97, 445–457 CrossRef CAS PubMed.
- I. Tiwari, M. Singh, C. M. Pandey and G. Sumana, RSC Adv., 2015, 5(82), 67115–67124 RSC.
- R. Jijie, K. Kahlouche, A. Barras, N. Yamakawa, J. Bouckaert, T. Gharbi, S. Szunerits and R. Boukherroub, Sens. Actuators, B, 2018, 260, 255–263 CrossRef CAS.
- Y. Ning, Q. Gao, X. Zhang, K. Wei and L. Chen, J. Biomol. Screening, 2016, 21(8), 851–857 CrossRef CAS PubMed.
- M. H. M. Zaid, C. E. N. Che-Engku-Chik, N. A. Yusof, J. Abdullah, S. S. Othman, R. Issa, M. F. Md Noh and H. Wasoh, Molecules, 2020, 25(15), 3373 CrossRef CAS PubMed.
- S. Shahrokhian and S. Ranjbar, ACS Sustainable Chem. Eng., 2019, 7(15), 12760–12769 CrossRef CAS.
- H. Safardoust-Hojaghan, M. Salavati-Niasari, O. Amiri and M. Hassanpour, J. Mol. Liq., 2017, 241, 1114–1119 CrossRef CAS.
- M. H. Mat Zaid, J. Abdullah, N. A. Yusof, Y. Sulaiman, H. Wasoh, M. F. Md Noh and R. Issa, Sens. Actuators, B, 2017, 241, 1024–1034 CrossRef CAS.
- L. Hao, L. Xue, F. Huang, G. Cai, W. Qi, M. Zhang, Q. Han, Z. Wang and J. Lin, Micromachines, 2020, 11(3), 281 CrossRef PubMed.
- L. Yang and Y. Li, J. Food Prot., 2005, 68(6), 1241–1245 CrossRef PubMed.
- P. L. Guo, M. Tang, S. L. Hong, X. Yu, D. W. Pang and Z. L. Zhang, Biosens. Bioelectron., 2015, 74, 628–636 CrossRef CAS PubMed.
- D. Wang, F. Lian, S. Yao, Y. Liu, J. Wang, X. Song, L. Ge, Y. Wang, Y. Zhao, J. Zhang, C. Zhao and K. Xu, ACS Omega, 2020, 5(36), 23070–23080 CrossRef CAS PubMed.
- Y. Wang and E. C. Alocilja, J. Biol. Eng., 2015, 9, 16 CrossRef PubMed.
- F. Zheng, P. Wang, Q. Du, Y. Chen and N. Liu, Front. Chem., 2019, 7, 232 CrossRef CAS PubMed.
- N. Mahheidari, J. Rashidiani, H. Kooshki and K. Eskandari, Curr. Nanosci., 2020, 16(5), 793–804 CrossRef CAS.
- H. Peng and I. A. Chen, ACS Nano, 2019, 13(2), 1244–1252 CAS.
- T. Bu, P. Jia, J. Liu, Y. Liu, X. Sun, M. Zhang, Y. Tian, D. Zhang, J. Wang and L. Wang, Food Chem.: X, 2019, 3, 100052 CAS.
- W. Gao, B. Li, R. Yao, Z. Li, X. Wang, X. Dong, H. Qu, Q. Li, N. Li, H. Chi, B. Zhou and Z. Xia, Anal. Chem., 2017, 89(18), 9836–9842 CrossRef CAS PubMed.
- Z. Fu, X. Zhou and D. Xing, Sens. Actuators, B, 2013, 182, 633–641 CrossRef CAS.
- H. J. Qi, S. H. Chen, R. Z. Hao, H. Shi, M. L. Zhang and S. Q. Wang, Anal. Methods, 2012, 4, 1178–1181 RSC.
- P. Gill, A. H. Alvandi, H. Abdul-Tehrani and M. Sadeghizadeh, Diagn. Microbiol. Infect. Dis., 2008, 62(2), 119–124 CrossRef CAS PubMed.
- F. Zhu, G. Zhao and W. Dou, Microchim. Acta, 2018, 185(10), 455 CrossRef PubMed.
- Z. Dehghani, T. Nguyen, M. Golabi, M. Hosseini, A. H. Rezayan, J. Mohammadnejad, A. Wolff and A. C. Vinayaka, Food Control, 2021, 121, 107664 CrossRef CAS.
- Q. Guo, J. J. Han, S. Shan, D. F. Liu, S. S. Wu, Y. H. Xiong and W. H. Lai, Biosens. Bioelectron., 2016, 86, 990–995 CrossRef CAS PubMed.
-
D. C. Vanegas, Y. Rong, N. Schwalb, K. D. Hills, C. Gomes and E. S. McLamore, Smart Biomedical and Physiological Sensor Technology XII, 2015, p. 948708 Search PubMed.
- M. Auffan, J. Rose, J. Y. Bottero, G. V. Lowry, J. P. Jolivet and M. R. Wiesner, Nat. Nanotechnol., 2009, 4(10), 634–641 CrossRef CAS PubMed.
- L. M. Bellan, D. Wu and R. S. Langer, Wiley Interdiscip. Rev.: Nanomed. Nanobiotechnol., 2011, 3(3), 229–246 CAS.
- Z. Jiang, B. Feng, J. Xu, T. Qing, P. Zhang and Z. Qing, Biosens. Bioelectron., 2020, 166, 112471 CrossRef CAS PubMed.
- K. Mahato, P. K. Maurya and P. Chandra, 3 Biotech, 2018, 8(3), 149 CrossRef PubMed.
- A. Merkoçi, Biosens. Bioelectron., 2010, 26(4), 1164–1177 CrossRef PubMed.
- D. Ghosh and N. Chattopadhyay, J. Lumin., 2015, 160, 223–232 CrossRef CAS.
- H. Liu, F. Zhan, F. Liu, M. Zhu, X. Zhou and D. Xing, Biosens. Bioelectron., 2014, 62, 38–46 CrossRef CAS PubMed.
- X. He and N. Ma, Colloids Surf., B, 2014, 124, 118–131 CrossRef CAS PubMed.
- H. M. Xiong, Y. Xu, Q. G. Ren and Y. Y. Xia, J. Am. Chem. Soc., 2008, 130(24), 7522–7523 CrossRef CAS PubMed.
- X. Yang, Y. Dang, J. Lou, H. Shao and X. Jiang, Theranostics, 2018, 8(5), 1449–1457 CrossRef CAS PubMed.
- Y. Ruan, W. Yu, F. Cheng, X. Zhang, T. Rao, Y. Xia and S. Larré, IET Nanobiotechnol., 2011, 5(2), 47 CrossRef CAS PubMed.
- J. D. Smith, G. W. Fisher, A. S. Waggoner and P. G. Campbell, Microvasc. Res., 2007, 73(2), 75–83 CrossRef CAS PubMed.
- R. Zhang, T. Belwal, L. Li, X. Lin, Y. Xu and Z. Luo, Compr. Rev. Food Sci. Food Saf., 2020, 19(3), 1465–1487 CrossRef PubMed.
- Y. Ma, J. Han, M. Wang, X. Chen and S. Jia, J. Materiomics, 2018, 4(2), 108–120 CrossRef.
- F. Tian, J. Lyu, J. Shi and M. Yang, Biosens. Bioelectron., 2017, 89(Pt 1), 123–135 CrossRef CAS PubMed.
- J. H. Kang, T. Kim, J. Choi, J. Park, Y. S. Kim, M. S. Chang, H. Jung, K. T. Park, S. J. Yang and C. R. Park, Chem. Mater., 2016, 28(3), 756–764 CrossRef CAS.
- J. H. Jung, D. S. Cheon, F. Liu, K. B. Lee and T. S. Seo, Angew. Chem., Int. Ed., 2010, 49(33), 5708–5711 CrossRef CAS PubMed.
- Y. F. Duan, Y. Ning, Y. Song and L. Deng, Microchim. Acta, 2014, 181, 647–653 CrossRef CAS.
- G. Vinodha, L. Cindrella and P. D. Shima, Mater. Res. Express, 2019, 6(8), 085548 CrossRef CAS.
- P. R. Barthasarathy and W. W. A. Wan Salim, Proceedings, 2020, 60(1), 13 Search PubMed.
- A. Pandey, Y. Gurbuz, V. Ozguz, J. H. Niazi and A. Qureshi, Biosens. Bioelectron., 2017, 91, 225–231 CrossRef CAS PubMed.
- M. S. Mannoor, H. Tao, J. D. Clayton, A. Sengupta, D. L. Kaplan, R. R. Naik, N. Verma, F. G. Omenetto and M. C. McAlpine, Nat. Commun., 2012, 3, 763 CrossRef PubMed.
- C. Liu, D. Jiang, G. Xiang, L. Liu, F. Liu and X. Pu, Analyst, 2014, 139(21), 5460–5465 RSC.
- A. M. Fernandes, M. H. Abdalhai, J. Ji, B. W. Xi, J. Xie, J. Sun, R. Noeline, B. H. Lee and X. Sun, Biosens. Bioelectron., 2015, 63, 399–406 CrossRef CAS PubMed.
- C. García-Aljaro, L. N. Cella, D. J. Shirale, M. Park, F. J. Muñoz, M. V. Yates and A. Mulchandani, Biosens. Bioelectron., 2010, 26(4), 1437–1441 CrossRef PubMed.
- S. Solanki, C. M. Pandey, R. K. Gupta and B. D. Malhotra, Biotechnol. J., 2020, 15(5), e1900279 CrossRef PubMed.
- A. Carbonaro, S. K. Mohanty, H. Huang, L. A. Godley and L. L. Sohn, Lab Chip, 2008, 8(9), 1478–1485 RSC.
- D. A. Boehm, P. A. Gottlieb and S. Z. Hua, Sens. Actuators, B, 2007, 126(2), 508–514 CrossRef CAS.
- S. Q. Jin, S. M. Guo, P. Zuo and B. C. Ye, Biosens. Bioelectron., 2015, 63, 379–383 CrossRef CAS PubMed.
- L. Cao, G. C. Han, H. Xiao, Z. Chen and C. Fang, Anal. Chim. Acta, 2020, 1096, 34–43 CrossRef CAS PubMed.
- E. L. Fava, T. A. Silva, T. M. do Prado, F. C. de Moraes, R. C. Faria and O. Fatibello-Filho, Talanta, 2019, 203, 280–286 CrossRef CAS PubMed.
- Z. Liu, D. Ye, R. Chen, B. Zhang, X. Zhu, J. Li and Q. Liao, Int. J. Hydrogen Energy, 2018, 43(49), 22467–22473 CrossRef CAS.
- G. A. Posthuma-Trumpie, J. Korf and A. Van Amerongen, Anal. Bioanal. Chem., 2009, 393(2), 569–582 CrossRef CAS PubMed.
- L. Wang, X. Shen, T. Wang, P. Chen, N. Qi, B. C. Yin and B. C. Ye, Biosens. Bioelectron., 2020, 165, 112364 CrossRef CAS PubMed.
- W. E. Doering, M. E. Piotti, M. J. Natan and R. G. Freeman, Adv. Mater., 2007, 19(20), 3100–3108 CrossRef CAS.
- Y. Al-Yousif, J. Anderson, C. Chard-Bergstrom and S. Kapil, Clin. Diagn. Lab. Immunol., 2002, 9(3), 723–725 Search PubMed.
- S. Roy, S. X. Wei, J. L. Z. Ying, M. Safavieh and M. U. Ahmed, Biosens. Bioelectron., 2016, 86, 346–352 CrossRef CAS PubMed.
- Z. Yu, M. Yu, Z. Zhang, G. Hong and Q. Xiong, Nanoscale Res. Lett., 2014, 9(1), 343 CrossRef PubMed.
- S. Roy, I. A. Rahman and M.
U. Ahmed, Anal. Methods, 2016, 8(11), 2391–2399 RSC.
- J. H. Jung, B. H. Park, S. J. Oh, G. Choi and T. S. Seo, Lab Chip, 2015, 15(3), 718–725 RSC.
- B. A. Rohrman and R. R. Richards-Kortum, Lab Chip, 2012, 12(17), 3082–3088 RSC.
- D. Lee, Y. T. Kim, J. W. Lee, D. H. Kim and T. S. Seo, Biosens. Bioelectron., 2016, 79, 273–279 CrossRef CAS PubMed.
- M. U. Ahmed, M. M. Hossain, M. Safavieh, Y. L. Wong, I. A. Rahman, M. Zourob and E. Tamiya, Crit. Rev. Biotechnol., 2016, 36(3), 495–505 Search PubMed.
- M. U. Ahmed, S. Nahar, M. Safavieh and M. Zourob, Analyst, 2013, 138(3), 907–915 RSC.
- M. Safavieh, M. U. Ahmed, E. Sokullu, A. Ng and M. Zourob, Analyst, 2013, 139(2), 482–487 RSC.
- M. Safavieh, M. U. Ahmed, A. Ng and M. Zourob, Biosens. Bioelectron., 2014, 58, 101–106 CrossRef CAS PubMed.
-
M. Safavieh, M. U. Ahmed and M. Zourob, Proceedings of IEEE Sensors, 2013 Search PubMed.
- R. Chavali, N. S. Kumar Gunda, S. Naicker and S. K. Mitra, Anal. Methods, 2014, 64(1), 6223–6227 RSC.
- M. Tolba, M. U. Ahmed, C. Tlili, F. Eichenseher, M. J. Loessner and M. Zourob, Analyst, 2012, 137(24), 5749–5756 RSC.
- S. A. Lim and M. U. Ahmed, RSC Adv., 2016, 6(3), 24995–25014 RSC.
-
M. Safavieh, S. Khetani, V. Kaul, D. R. Kuritzkes and H. Shafiee, Advances in Global Health through Sensing Technologies 2015, 2015, p. 9490 Search PubMed.
- J. Joo, D. Kwon, H. H. Shin, K. H. Park, H. J. Cha and S. Jeon, Sens. Actuators, B, 2013, 188, 1250–1254 CrossRef CAS.
- J. Su, J. Xu, Y. Chen, Y. Xiang, R. Yuan and Y. Chai, Chem. Commun., 2012, 9(5), 1852–1861 Search PubMed.
- Y. Xiang and Y. Lu, Anal. Chem., 2012, 84(9), 4174–4178 CrossRef CAS PubMed.
- H. Zhang, X. Ma, Y. Liu, N. Duan, S. Wu, Z. Wang and B. Xu, Biosens. Bioelectron., 2015, 74, 872–877 CrossRef CAS PubMed.
- Q. Wei, H. Qi, W. Luo, D. Tseng, S. J. Ki, Z. Wan, Z. Göröcs, L. A. Bentolila, T. T. Wu, R. Sun and A. Ozcan, ACS Nano, 2013,(10), 9147–9155 CrossRef CAS PubMed.
- A. M. Nicolini, C. F. Fronczek and J. Y. Yoon, Biosens. Bioelectron., 2015, 67, 560–569 CrossRef CAS PubMed.
- P. S. Liang, T. S. Park and J. Y. Yoon, Sci. Rep., 2014, 4, 5953 CrossRef CAS PubMed.
- D. N. Breslauer, R. N. Maamari, N. A. Switz, W. A. Lam and D. A. Fletcher, PLoS One, 2009, 4(7), e6320 CrossRef PubMed.
- J. Rodriguez-Manzano, M. A. Karymov, S. Begolo, D. A. Selck, D. V. Zhukov, E. Jue and R. F. Ismagilov, ACS Nano, 2016, 10(3), 3102–3113 CrossRef CAS PubMed.
- R. D. Stedtfeld, D. M. Tourlousse, G. Seyrig, T. M. Stedtfeld, M. Kronlein, S. Price, F. Ahmad, E. Gulari, J. M. Tiedje and S. A. Hashsham, Lab Chip, 2012, 12(8), 1454–1462 RSC.
- B. Berg, B. Cortazar, D. Tseng, H. Ozkan, S. Feng, Q. Wei, R. Y. L. Chan, J. Burbano, Q. Farooqui, M. Lewinski, D. Di Carlo, O. B. Garner and A. Ozcan, ACS Nano, 2015, 9(8), 7857–7866 CrossRef CAS PubMed.
- H. Van Nguyen, V. D. Nguyen, F. Liu and T. S. Seo, ACS Omega, 2020, 5(35), 22208–22214 CrossRef PubMed.
- A. Upadhyay, R. M. Waleed, J. Wang, J. Zhao, Q. Guan, C. Liao and Q. Han, Parasitol. Res., 2021, 120(5), 1–11 CrossRef PubMed.
- L. Barnes, D. M. Heithoff, S. P. Mahan, G. N. Fox, A. Zambrano, J. Choe, L. N. Fitzgibbons, J. D. Marth, J. C. Fried, H. T. Soh and M. J. Mahan, EBioMedicine, 2018, 36, 73–82 CrossRef PubMed.
- S. İ. Dönmez, S. H. Needs, H. M. I. Osborn and A. D. Edwards, Sens. Actuators, B, 2020, 323, 128645 CrossRef.
- H. Shafiee, W. Asghar, F. Inci, M. Yuksekkaya, M. Jahangir, M. H. Zhang, N. G. Durmus, U. A. Gurkan, D. R. Kuritzkes and U. Demirci, Sci. Rep., 2015, 5, 8719 CrossRef PubMed.
- W. Du, L. Li, K. P. Nichols and R. F. Ismagilov, Lab Chip, 2009, 9(16), 2286–2292 RSC.
Footnote |
† Sharmili Roy and Fareeha Arshad contributed equally to this manuscript. |
|
This journal is © The Royal Society of Chemistry 2022 |
Click here to see how this site uses Cookies. View our privacy policy here.