DOI:
10.1039/D2AN01704K
(Paper)
Analyst, 2023,
148, 146-152
Dual mimic enzyme properties of Fe nanoparticles embedded in two-dimensional carbon nanosheets for colorimetric detection of biomolecules†
Received
18th October 2022
, Accepted 30th November 2022
First published on 1st December 2022
Abstract
The development of novel nanozymes is of great importance for the efficient analysis of biomolecules such as H2O2, glucose, and antioxidants in the diagnosis of some diseases. Herein, novel nanozymes based on Fe nanoparticles (NPs) encapsulated in 2D carbon nanosheets (denoted as Fe@CNs) were constructed and employed in the field of biosensing. Notably, Fe@CNs have intrinsic dual mimic enzyme properties. The colorless colorimetric substrate 3,3′,5,5′-tetramethylbenzidine (TMB) can be oxidized by Fe@CNs as oxidase- and peroxidase-like nanozymes, respectively. The generation of the oxidation state TMB (oxTMB) resulted from the presence of reactive oxygen species (ROS) which were produced by the catalytic decomposition of the dissolved oxygen or H2O2. Thus, a simple colorimetric biosensor was proposed to detect glutathione (GSH), H2O2, and glucose. In addition, the Fe@CN-based nanozymes also have excellent reusability in enzymatic catalysis. After separating from the sensing systems, Fe@CNs can be reused in other catalytic processes. This colorimetric method could be used as a universal sensing platform for the detection of antioxidants and H2O2-related bioanalysis. This work broadens the application of novel nanozymes in biosensing.
Introduction
Nanozymes have attracted increasing attention in many fields due to their superior features of lower cost, easier storage, and higher tolerance to harsh environments in comparison with natural enzymes.1–5 So far, various types of nanomaterials have been developed as nanozymes. For example, zero-dimensional (0D) nanoparticles (NPs)6–10 (e.g. Au NPs, Pt NPs, Fe3O4 NPs, CeO2 NPs, and FeS2 NPs), one-dimensional (1D) nanowires (NWs)11–13 (e.g. Ag NWs, V2O5 NWs, and MnO2 NWs), two-dimensional (2D) nanosheets (NSs)14,15 (e.g. FeS NSs and graphene oxide), and three-dimensional (3D) metal–organic framework materials (MOFs) (e.g. MIL-53(Fe), MIL-47(V), and ZIF-8)16–18 have been utilized as oxidase-like and peroxidase-like nanozymes in the construction of sensing platforms for the assay of H2O2,19,20 glucose,21,22 glutathione (GSH),23,24 cancer cells25,26 and so on. However, the catalytic activity of some nanozymes would be depressed in serum, urine, or living cell samples. Therefore, there is an urgent requirement of novel nanozymes with high enzymatic activity for practical applications.
Previous research shows that the catalytic efficiency of nanozymes is related to the specific surface area of nanomaterials. For instance, the catalytic activity of FeS2 NPs with a larger specific surface area was much higher than that of the bulk FeS2 materials.10 Nevertheless, nanoparticle agglomeration will inevitably result in the reduction of the surface area and thus be detrimental to the subsequent performance of sensing platforms. In order to alleviate the aggregation, the dispersion of NPs on 2D carbon nanosheets (CNs) can be an effective approach.27–30 The enzymatic catalytic activity of NPs on the surface of CNs was effectively enhanced with the increase of the specific surface area. Besides, CNs have porous structures with a high surface area, endowing the 2D nanozymes with a high mass transfer rate.31,32 These factors can effectively enhance the catalytic activity of hybrid 2D CN-based nanomaterials, accelerating the oxidation reaction between the substrates. Moreover, the synthesis methods of hybrid 2D CN-based nanomaterials are simple in operation and usually use low-cost reagents as the raw materials.33–35 Therefore, the fabrication of novel hybrid 2D CN-based nanomaterials pave the way for the development of nanozymes with high catalytic performance.
The majority of 2D nanomaterial-based nanozymes exhibit a single enzyme activity, as they mainly act as oxidase-like or peroxidase-like mimics in biosensors.28–30,36–38 However, 2D nanomaterials with dual mimic enzyme properties, compared with that of nanozymes with a single enzyme catalytic activity, are rare. Based on these findings, the enzymatic properties of Fe NPs encapsulated in 2D CNs (denoted as Fe@CNs) were investigated by the colorimetric method. Fe@CNs can effectively catalyze the oxidation of the colorimetric substrate 3,3′,5,5′-tetramethylbenzidine (TMB) regardless of whether H2O2 is added into the catalytic system or not, demonstrating the oxidase-like and peroxidase-like catalytic activity. Taking advantage of their dual enzymatic activity, Fe@CNs were employed in the construction of a universal sensing platform for the detection of biomolecules such as GSH, H2O2, and glucose. The label-free colorimetric method has the merit of simplicity and could further be expanded for in-field detection by naked-eye observation.
Experimental section
Materials and reagents
All the chemical reagents were of analytical grade and used directly without purification. Polyvinylpyrrolidone (PVP, K30) and Fe(NO3)3·9H2O were purchased from Sigma-Aldrich. Maltose, fructose, lactose, sucrose, galactose, starch, protamine, GSH, cysteine (Cys), alanine (Ala), valine (Val), leucine (Leu), isoleucine (Ile), phenylalanine (Phe), tryptophan (Trp), methionine (Met), proline (Pro), glycine (Gly), serine (Ser), threonine (Thr), tyrosine (Tyr), histidine (His), arginine (Arg), aspartic acid (Asp), asparagine (Asn), lysine (Lys), and glutamic acid (Glu) were obtained from Aladdin (Shanghai, China). 3,3′,5,5′-tetramethylbenzidine (TMB), glucose oxidase (GOx), glucose, and lysozyme were purchased from Sangon Biotech Co., Ltd (Shanghai, China). H2O2 and terephthalic acid (TA) were obtained from Sinopharm Chemical Reagent Co., Ltd (Shanghai, China). 5,5-Dimethyl-1-pyrroline N-oxide (DMPO) and 2,2,6,6-tetramethylpiperidine (TEMP) were purchased from Energy Chemical Reagent Co., Ltd (Shanghai, China). Co(NO3)2, Ba(NO3)2, KNO3, Pb(NO3)2, Zn(NO3)2, Al(NO3)3, Ca(NO3)2, and Mg(NO3)2 were provided by Adamas (Tansoole, Shanghai, China). The human serum sample was purchased from Solarbio Science & Technology Co., Ltd (Beijing, China).
Measurements and characterization
The UV-vis absorption spectra were recorded by employing a TU-1900 double-beam UV-vis spectrophotometer (Persee, Beijing, China). The fluorescence measurement was performed on an F97XP spectrophotometer, where the excitation and emission slit widths were both set to 10 nm. The applicability of the biosensors in the human serum sample was tested in compliance with the protocol approved by the Ethics Committee of Nanjing Tech University (No. 2019-1).
Synthesis of Fe@CNs
Fe@CNs were synthesized on the basis of the previous study.30 The solution of Fe(NO3)3·9H2O (3.0 g) and PVP (1.8 g) in water (60 mL) was continuously stirred at 95 °C until the water was evaporated. The obtained yellow powder was further calcined at 700 °C for 2 h under argon protection to obtain Fe@CNs.
Colorimetric detection of targets such as GSH, H2O2 and glucose
In the catalytic system, the amount of ingredients was the final concentration. 20 mM HAc-NaAc buffer (pH 3.5, 100 μL) containing 0.2 mM TMB, 0.2 g L−1 Fe@CNs and GSH (H2O2, or glucose with 0.2 g L−1 GOx) was incubated at 45 °C for 40 min (or 45 min). Then, the UV-vis absorption spectra of the samples were recorded and the absorbance intensity at 652 nm was used for the assay of the targets.
Results and discussion
Dual enzymatic activities of Fe@CNs
The enzymatic catalytic activity of Fe@CNs was investigated by the colorimetric method. In the catalytic system, TMB was selected as the colorimetric substrate. As TMB itself was colorless (Fig. 1a, lane 1), its UV-vis absorption spectrum exhibited no absorption peak in the wavelength range from 400 nm to 800 nm (Fig. 1a, black curve). However, the sample in which TMB coexisted with Fe@CNs became blue (Fig. 1, lane 3) and exhibited a strong absorption signal at a wavelength of 652 nm (Fig. 1, blue curve). The experimental phenomenon demonstrated that Fe@CNs have oxidase-like catalytic activity. Obviously, a darker blue solution and an enhanced absorbance signal at 652 nm were achieved with the addition of H2O2 into the mixture of TMB and Fe@CNs (Fig. 1, lane 4 and green curve). These results indicated that the efficiency of oxidation of TMB by Fe@CNs could be effectively improved with the help of H2O2. Besides, the presence of Fe@CNs can promote the oxidation reaction between TMB and H2O2 (Fig. 1, lane 2 and red curve). These observations demonstrated that Fe@CNs also have peroxidase-like catalytic activity.
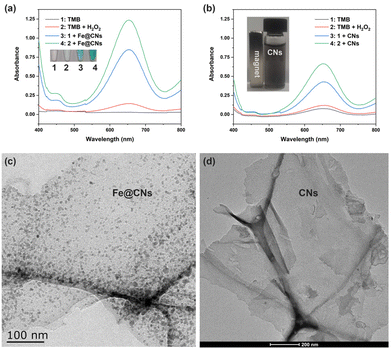 |
| Fig. 1 (a and b) UV-vis absorption spectra of the catalytic systems promoted by Fe@CNs and CNs. Inset: ingredients and photographs of the corresponding samples and the solution of CNs in water. (c and d) The TEM images of Fe@CNs and CNs. | |
In order to get to the root of their catalytic activity, Fe@CNs were cleaned with concentrated hydrochloric acid several times to obtain CNs. As shown in the inset of Fig. 1b, the nonmagnetic products, CNs, were achieved, indicating that the magnetic Fe NPs were removed from Fe@CNs. Moreover, the disappearance of Fe NPs on the surface of CNs (Fig. 1c and d) further confirmed that the CNs were successfully prepared. Similar to Fe@CNs, CNs can promote the oxidase- and peroxidase-based catalytic systems, respectively (Fig. 1b). These results implied that the catalytic activity of Fe@CNs was derived from Fe NPs and CNs. Furthermore, the N2 adsorption–desorption technique was employed to confirm the mesoporous structure of CNs. The obtained Brunauer–Emmett–Teller (BET) surface area and the pore size of Fe@CNs were about 69.26 m2 g−1 (Fig. 2a) and 7.72 nm (Fig. 2b), respectively. These structural characteristics could help Fe@CN-based nanozymes to expose more active sites and accelerate the process of mass transfer. As a result, the reaction substrates can be effectively catalysed by Fe@CNs.
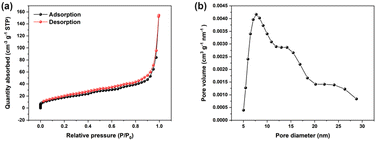 |
| Fig. 2 (a) N2 adsorption/desorption isotherm and (b) pore size distribution of Fe@CNs. | |
Further investigation showed that the catalytic activity of Fe@CNs could be greatly affected by the reaction environment, such as pH, temperature and incubation time. When the pH was increased from 2.0 to 5.0, the oxidase- and peroxidase-like catalytic activities of Fe@CNs were both gradually enhanced first and then rapidly depressed (Fig. S1a and S2a†). The largest enhancement of enzymatic catalytic activity was observed at pH 3.5 (Fig. S1b and S2b†). Subsequently, the incubation temperature of the catalytic systems was optimized as shown in Fig. S1c and S2c.† The optimum reaction temperature for Fe@CNs was 45 °C because the absorbance of the catalytic systems at 652 nm attained the maximum value (Fig. S1d and S2d†). Finally, to achieve the highest enzymatic activities of Fe@CNs, an incubation time of 40 minutes and 45 minutes was selected for the oxidase- and peroxidase-like properties, respectively (Fig. S1e, S1f, S2e and S2f†). Therefore, the Fe@CN-based catalytic systems were used under the abovementioned experimental conditions to achieve the highest catalytic efficiency.
Besides, the reusability of Fe@CNs as oxidase- and peroxidase-like nanozymes was investigated in catalytic systems, respectively. Fig. S3a† shows that there was a small decline in the relative activities of Fe@CN-based nanozymes when the number of cycles was increased. Even after reusing the nanozymes 5 times, the relative activity for oxidase- and peroxidase-like activity can remain 86.4% and 87.9% of their original enzymatic activity, respectively. The results indicated that the Fe@CN-based nanozymes possess good reusability in catalytic systems. As shown in Scheme 1, Fe@CNs possess magnetic properties and can be separated from the solution using a magnet. Thus, the nanozymes of Fe@CNs can be easily recycled. In addition, the catalytic activity of Fe@CNs was investigated in the catalytic system with the presence of 1% serum sample, 1% urine sample, and 1% cell lysate, respectively. As shown in Fig. S3b,† the complex ingredients of these biological samples could affect the oxidase-like and peroxidase-like activity of Fe@CNs. However, 79.20%–90.91% of the original activity can be retained when Fe@CNs were employed in the solution containing 1% biological fluids, demonstrating great potential in the development of biosensors for practical applications.
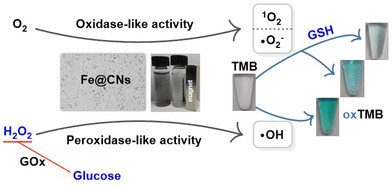 |
| Scheme 1 Schematic for the colorimetric detection of GSH, H2O2, and glucose by employing Fe@CNs as the sensing platforms. | |
Catalytic mechanism of Fe@CN-based nanozymes
In order to fully explore the properties of Fe@CN-based nanozymes, their catalytic mechanisms were investigated in detail. Firstly, the catalytic oxidation of TMB by Fe@CNs as oxidase-like nanozymes was conducted in an environment with different amounts of dissolved oxygen. As shown in Fig. 3a, the oxidase-like activity of Fe@CNs under O2 protection was much higher than that under air and N2 protection. Thus, the dissolved oxygen played an important role in the catalytic process. Subsequently, the reactive oxygen species (ROS) produced in the catalytic system were studied by electron paramagnetic resonance (EPR) spectroscopy. Three resonance lines (Fig. 3b) and six resonance lines (Fig. 3c) were detected in the EPR spectra by using TEMP and DMPO as radical traps, respectively. These results reflected the generation of singlet oxygen (1O2) and the superoxide radical (˙O2−) in the catalytic systems.39,40 It could be concluded that the oxidation of TMB to oxTMB was ascribed to the generation of ROS obtained through the catalytic oxidation of the dissolved oxygen by Fe@CNs as oxidase-like nanozymes. Besides, when the catalytic system of DMPO and H2O2 with Fe@CNs was used in water, four resonance lines with 1
:
2
:
2
:
1 resonance intensity were detected (Fig. 3d), indicating the generation of hydroxyl radicals (˙OH) and the peroxidase-like activity of Fe@CNs.29
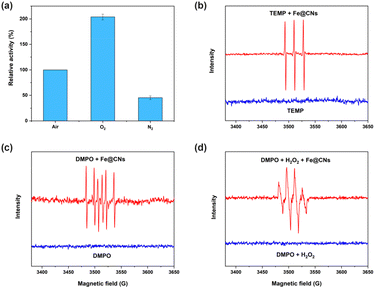 |
| Fig. 3 (a) The effect of O2 and N2 on the oxidase-like activity of Fe@CNs. (b) EPR spectra of TEMP (100 mM) with and without Fe@CNs (50 mg L−1) by using water as the solvent. (c) EPR spectra of DMPO (100 mM) with and without Fe@CNs (50 mg L−1) by using methanol as the solvent. (d) DMPO (100 mM) and H2O2 (100 mM) with and without Fe@CNs (50 mg L−1) by using water as the solvent. | |
In order to study the specific mechanism of Fe@CNs as peroxidase-like nanozymes, further investigation was carried out under the condition without oxygen to eliminate the effect of the oxidase-like activity of Fe@CNs. As shown in Fig. S3c,† there were no characteristic signals in the EPR spectrum of the sample containing Fe@CNs, DMPO and H2O2 by using methanol as the solvent without oxygen. These results suggested that other ROS such as ˙O2− were absent in the catalytic process. Thus, ˙OH played a vital role in the oxidation reaction between TMB and H2O2 promoted by Fe@CNs (Fig. S3d,† lane 1 and lane 2), revealing that the catalytic process could be realized in two steps. As shown in Fig. S3d† (lane 4), Fe@CNs first catalyzed H2O2 to generate ˙OH and TMB was then oxidized by ˙OH, resulting in a strong absorption peak at 652 nm. However, when thiourea as an ˙OH scavenger was added into the product of H2O2 and Fe@CNs before the introduction of TMB into the mixture, a weak adsorption signal was detected (Fig. S3d,† lane 3). The oxidation of TMB was impeded by the consumption of ˙OH by thiourea. These results further confirmed that the chromogenic reaction was triggered by ROS.
Besides, the effect of radical scavengers on the enzymatic activity of Fe@CNs was further explored to confirm the presence of ROS. With the increasing concentration of radical scavengers including SO32−, benzoquinone and thiourea, the enzymatic catalytic activity of Fe@CNs was gradually depressed (Fig. S4 and S5a†). As expected, the addition of thiourea could effectively inhibit the peroxidase-like activity of Fe@CNs. The results were due to the increased consumption of 1O2, ˙O2− and ˙OH,29,39,40 further confirming the production of these ROS in the catalytic process. Because ˙OH could oxidize no-phosphorescence terephthalic acid (TA) to obtain 2-hydroxyterephthalic acid (HTA) with a high phosphorescence signal at 443 nm in the emission spectrum,29 more introduction of Fe@CNs into the mixture of TA and H2O2 led to a higher phosphorescence intensity (Fig. S5b†). The peroxidase-like activity of Fe@CNs and their catalytic mechanism were also confirmed by the photoluminescence method.
The steady-state kinetic analysis of Fe@CN-based nanozymes was carried out via the Michaelis–Menten model and Lineweaver–Burk double-reciprocal model. As shown in Fig. S6 and S7,† the typical Michaelis–Menten curves were obtained with the increase of the substrate amount and their corresponding double reciprocal curves were fitted to the Lineweaver–Burk equation. The calculated Michaelis–Menten constant (Km) values (Tables S1 and S2†) of Fe@CNs to substrates were 0.24 mM (Fe@CNs as oxidase-like nanoenzymes to TMB), 0.45 mM (Fe@CNs as peroxidase-like nanoenzymes to TMB) and 0.50 mM (Fe@CNs as peroxidase-like nanoenzymes to H2O2), which were much lower than those of some Fe-based nanomaterials. These results indicated that Fe@CN-based nanozymes have high affinity to substrates. Compared with horseradish peroxidase (HRP) and other nanomaterial-based peroxidase-like nanozymes, Fe@CNs have a high maximum reaction velocity (Vmax) (Table S2†), indicating their outstanding peroxidase-like catalytic activity.
Fe@CNs as sensing platforms for the detection of biomolecules
Fe@CNs as oxidase-like nanozymes for GSH detection.
It is well known that GSH can affect the catalytic activity of nanozymes. Thus, the presence of GSH in the Fe@CN-based catalytic system can effectively depress the oxidation reaction of TMB by the oxidase-like activity of Fe@CNs, obtaining a colorless mixture and a low absorbance signal at 652 nm (Fig. S8a†). On the basis of these results, Fe@CNs as oxidase-like nanozymes could be employed for the detection of GSH. As shown in Fig. S9,† the absorbance signal at 652 nm in the UV-vis absorption spectrum decreased with the increase of the GSH concentration ranging from 0 to 100 μM and then reached a plateau. The change of the absorbance value at 652 nm has a linear relationship with the GSH concentration in the range of 1–80 μM (R2 = 0.9962, Fig. 4a). The inset of Fig. 4a shows that the color change of the samples corresponded to a good linear relationship. The limit of detection (LOD) for the GSH assay was 0.22 μM (S/N = 3) by using Fe@CNs as the sensing platform, which was better than many previously reported nanomaterial-based oxidase-like nanozymes (Table S3†).
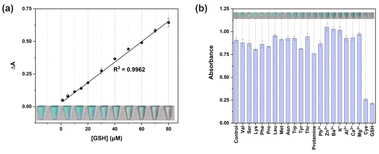 |
| Fig. 4 (a) The linear calibration plot for the GSH assay. Inset: photographs of the samples with different GSH concentrations (1, 5, 10, 15, 20, 30, 40, 50, 60, 70, and 80 μM). (b) The specificity analysis of GSH detection. The concentration of the interfering species and GSH was 70 μM. Inset: photographs of the corresponding samples. | |
Besides, the specificity analysis of GSH detection was performed by adding multiple substances including protamine, amino acids, and metal ions. As shown in Fig. 4b, the absorbance signal at 652 nm of the samples containing GSH and Cys was significantly reduced, obtaining a colorless solution, respectively. However, the presence of other substances has no effect on the oxidation of TMB by Fe@CNs, as indicated by the high absorbance signal and blue product. The decrease of the absorbance induced by GSH and Cys was investigated by conducting the sensing process through different reaction routes (Fig. S8b,† inset). Firstly, TMB was added into Fe@CNs before GSH (Fig. S8b,† lane 2). Compared to the catalytic system without GSH, the presence of GSH just caused a small decline in the absorbance intensity (Fig. S8b,† lane 1). This might be attributed to the reducibility of GSH,41,42 resulting in the reduction of oxTMB. However, when the introduction of GSH was prior to that of TMB into the sensing system, a low absorbance signal was detected (Fig. S8b,† lane 3). As an antioxidant, GSH can react with ROS.43 ROS consumption by GSH was a major impediment to the oxidation of TMB. Thus, the TMB oxidation process was suppressed through the above two factors. As expected, the same phenomenon was exhibited in the catalytic system with Cys which was the main component of GSH. Therefore, this colorimetric method can be used for GSH detection.
In order to study the application of the GSH sensing platform in practical samples, three amounts (20 μM, 40 μM and 60 μM) of GSH were added to 1% human serum sample, respectively. An absorbance intensity at 652 nm was applied to calculate the recoveries. As shown in Table S4,† the recovery rate varied from 98.96% to 105.09%, implying that the GSH sensor designed by employing Fe@CNs with oxidase-like activity could be applied in the actual sample analysis.
Fe@CNs as peroxidase-like nanozymes for the detection of H2O2 and glucose.
Apart from oxidase-like activity, Fe@CNs also have peroxidase-like activity. As the amount of H2O2 has a significant effect on the generation of oxTMB, a catalytic system was employed in the sensing of H2O2. As shown in Fig. 5a and Fig. S10,† the absorbance value at 652 nm first showed a rapid increase with the concentration of H2O2 ranging from 0–300 μM, followed by an upcoming plateau when more H2O2 was added. There was a good linear relationship between the enhancement of absorbance intensity at 652 nm and the H2O2 amount in the range of 5–200 μM (R2 = 0.9993, Fig. 5b). Moreover, the color change of the samples also corresponded to a good linear relationship (Fig. 5b, inset). The calculated LOD for the H2O2 assay was 2.04 μM (S/N = 3), which was lower than that of some previously reported peroxidase-like nanozyme-based sensing platforms (Table S5†). Thus, a simple and feasible colorimetric method for H2O2 detection was established on the basis of the peroxidase-like activity of Fe@CNs.
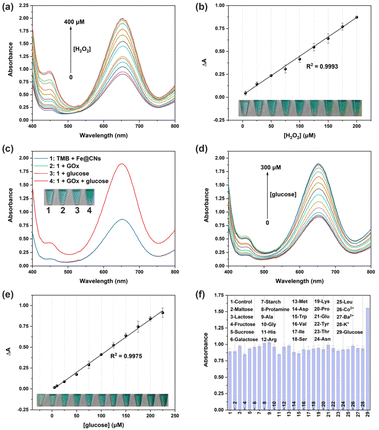 |
| Fig. 5 (a) The UV-vis absorption curves of Fe@CNs and TMB with different concentrations of H2O2. (b) The linear calibration plot for the H2O2 assay. Inset: photographs of the samples with different concentrations of H2O2 (5, 25, 50, 75, 100, 125, 150, 175, and 200 μM). (c) The UV-vis absorption spectra of the catalytic system with different reagents. Inset: ingredients and photographs of the corresponding samples. (d) The UV-vis absorption curves of Fe@CNs with TMB and GOx in the presence of different concentrations of glucose. (e) The linear calibration plot for glucose detection. Inset: photographs of the samples with different concentrations of glucose (5, 10, 25, 50, 75, 100, 125, 150, 175, 200, and 225 μM). (f) The specificity analysis of glucose detection. The concentration of the interfering species and glucose was 150 μM. Inset: the interfering species. | |
In order to expand the application of Fe@CNs as peroxidase-like nanozymes in biosensing, the H2O2 sensing platform was employed for the assay of H2O2-related biomolecules such as glucose. As shown in Fig. 5c, the absorbance signal of the catalytic system (blue curve) could be obviously enhanced with the addition of glucose along with GOx (red curve), showing the great importance of H2O2 generated in the catalytic process. However, neither GOx nor glucose has an effect on the peroxidase-like activity of Fe@CNs (Fig. 5c, green curve and pink curve). Therefore, the Fe@CN-based H2O2 sensing platform can also be used for the detection of glucose.
The sensitivity of this biosensor was investigated by adding different concentrations of glucose into the catalytic system. The absorbance signal enhanced with the increase of the amount of glucose (Fig. 5d and Fig. S11†). When the glucose amount ranged from 5 μM to 225 μM, the change of the absorbance value had a linear relationship with the glucose concentration (R2 = 0.9975, Fig. 5e). Moreover, the color of these corresponding samples deepened gradually in a linear manner (Fig. 5e, inset). Thus, the visualization of the glucose assay can be carried out by using Fe@CNs as the sensing platform. The LOD of glucose can reach 2.28 μM, which was better than that of different nanomaterial-based peroxidase-like nanozymes that act as glucose sensing platforms (Table S6†). Besides, the selectivity of this method is compared as shown in Fig. 5f. Protamine, carbohydrates, amino acids and metal ions failed to enhance the absorbance signal of the catalytic system even though GOx was added into the samples (Fig. S12†). The excellent selectivity of the glucose assay was attributed to the highly specific catalytic ability of GOx to glucose.
To evaluate the feasibility of the sensing method based on the peroxidase-like catalytic activity of Fe@CNs in actual biological systems, known concentrations of H2O2 and glucose were added to 1% human serum samples, respectively. As shown in Table S4,† the recoveries for H2O2 and glucose detection vary from 98.22% to 105.51%, demonstrating great application potential of the Fe@CN-based catalytic system in real samples.
Conclusions
In summary, Fe@CNs have the ability to catalyze the oxidation of the colorimetric substrate TMB with and without the presence of H2O2, possessing oxidase- and peroxidase-like enzymatic activity. Their excellent dual enzyme mimic activity was the result of the generation of ROS in the catalytic process by the decomposition of the dissolved oxygen or H2O2. Based on these properties of Fe@CNs, the colorimetric sensing platforms were established for the assay of bioactive molecules such as GSH, glucose and H2O2. Benefiting from the magnetic feature, Fe@CNs can be easily reused in biosensing. Moreover, the targets could be detected by the naked eye. Therefore, the Fe@CN-based label-free colorimetric method has the distinguishing features of low-cost and simple operation, and exhibits a great application prospect in in-field detection.
Conflicts of interest
There are no conflicts to declare.
Acknowledgements
This work was supported by the National Natural Science Foundation of China (21801132), China Postdoctoral Science Foundation (2021M701505) and Jiangsu Zhongdan Group Co., Ltd.
References
- S. Fedeli, J. Im, S. Gopalakrishnan, J. L. Elia, A. Gupta, D. Kim and V. M. Rotello, Chem. Soc. Rev., 2021, 50, 13467–13480 RSC.
- J. Wu, X. Wang, Q. Wang, Z. Lou, S. Li, Y. Zhu, L. Qin and H. Wei, Chem. Soc. Rev., 2019, 48, 1004–1076 RSC.
- X. Zhang, G. Li, G. Chen, D. Wu, Y. Wu and T. D. James, Adv. Funct. Mater., 2021, 31, 2106139 CrossRef CAS.
- D. Wang, D. Jana and Y. Zhao, Acc. Chem. Res., 2020, 53, 1389–1400 CrossRef CAS PubMed.
- M. Ghorbani, H. Derakhshankhah, S. Jafari, S. Salatin, M. Dehghanian, M. Falahati and A. Ansari, Nano Today, 2019, 29, 100775 CrossRef CAS.
- J. Yun, B. Li and R. Cao, Chem. Commun., 2010, 46, 8017–8019 RSC.
- J. Fan, J.-J. Yin, B. Ning, X. Wu, Y. Hu, M. Ferrari, G. J. Anderson, J. Wei, Y. Zhao and G. Nie, Biomaterials, 2011, 32, 1611–1618 CrossRef CAS PubMed.
- L. Gao, J. Zhuang, L. Nie, J. Zhang, Y. Zhang, N. Gu, T. Wang, J. Feng, D. Yang, S. Perrett and X. Yan, Nat. Nanotechnol., 2007, 2, 577–583 CrossRef CAS PubMed.
- A. Asati, S. Santra, C. Kaittanis, S. Nath and J. M. Perez, Angew. Chem., Int. Ed., 2009, 48, 2308–2312 CrossRef CAS PubMed.
- C. Song, W. Ding, W. Zhao, H. Liu, J. Wang, Y. Yao and C. Yao, Biosens. Bioelectron., 2020, 151, 111983 CrossRef CAS PubMed.
- L. Cao, Y. Fang, Y. Zhang, J. Yang and J. He, Int. J. Nanosci., 2020, 19, 2050004 CrossRef CAS.
- R. Andre, F. Natalio, M. Humanes, J. Leppin, K. Heinze, R. Wever, H. C. Schroeder, W. E. G. Mueller and W. Tremel, Adv. Funct. Mater., 2011, 21, 501–509 CrossRef CAS.
- L. Han, J. Shi and A. Liu, Sens. Actuators, B, 2017, 252, 919–926 CrossRef CAS.
- Z. Dai, S. Liu, J. Bao and H. Ju, Chem. – Eur. J., 2009, 15, 4321–4326 CrossRef CAS PubMed.
- J. Zhang, S. Wu, L. Ma, P. Wu and J. Liu, Nano Res., 2020, 13, 455–460 CrossRef CAS.
- L. Ai, L. Li, C. Zhang, J. Fu and J. Jiang, Chem. – Eur. J., 2013, 19, 15105–15108 CrossRef CAS PubMed.
- X. Niu, X. Li, Z. Lyu, J. Pan, S. Ding, X. Ruan, W. Zhu, D. Du and Y. Lin, Chem. Commun., 2020, 56, 11338–11353 RSC.
- J. Wu, Y. Yu, Y. Cheng, C. Cheng, Y. Zhang, B. Jiang, X. Zhao, L. Miao and H. Wei, Angew. Chem., Int. Ed., 2021, 60, 1227–1234 CrossRef CAS PubMed.
- C. Zhang and Z. Nan, Inorg. Chem., 2022, 61, 13933–13943 CrossRef CAS PubMed.
- Z. Qi, L. Wang, Q. You and Y. Chen, Biosens. Bioelectron., 2017, 96, 227–232 CrossRef CAS PubMed.
- N. Lu, M. Zhang, L. Ding, J. Zheng, C. Zeng, Y. Wen, G. Liu, A. Aldalbahi, J. Shi, S. Song, X. Zuo and L. Wang, Nanoscale, 2017, 9, 4508–4515 RSC.
- J. Zhu, X. Peng, W. Nie, Y. Wang, J. Gao, W. Wen, J. N. Selvaraj, X. Zhang and S. Wang, Biosens. Bioelectron., 2019, 141, 111450 CrossRef CAS PubMed.
- N. K. Dega, A. B. Ganganboina, H. L. Tran, E. P. Kuncoro and R.-A. Doong, Talanta, 2022, 237, 122957 CrossRef CAS PubMed.
- M. Shamsipur, A. Safavi and Z. Mohammadpour, Sens. Actuators, B, 2014, 199, 463–469 CrossRef CAS.
- X. Meng, D. Li, L. Chen, H. He, Q. Wang, C. Hong, J. He, X. Gao, Y. Yang, B. Jiang, G. Nie, X. Yan, L. Gao and K. Fan, ACS Nano, 2021, 15, 5735–5751 CrossRef CAS PubMed.
- L.-N. Zhang, H.-H. Deng, F.-L. Lin, X.-W. Xu, S.-H. Weng, A.-L. Liu, X.-H. Lin, X.-H. Xia and W. Chen, Anal. Chem., 2014, 86, 2711–2718 CrossRef CAS PubMed.
- X. Zhou, M. You, F. Wang, Z. Wang, X. Gao, C. Jing, J. Liu, M. Guo, J. Li, A. Luo, H. Liu, Z. Liu and C. Chen, Adv. Mater., 2021, 33, 2100556 CrossRef CAS PubMed.
- C. Song, H. Liu, L. Zhang, J. Wang, C. Zhao, Q. Xu and C. Yao, Sens. Actuators, B, 2022, 353, 131131 CrossRef CAS.
- W. Ding, H. Liu, W. Zhao, J. Wang, L. Zhang, Y. Yao, C. Yao and C. Song, ACS Appl. Bio Mater., 2020, 3, 5905–5912 CrossRef CAS PubMed.
- C. Song, W. Zhao, H. Liu, W. Ding, L. Zhang, J. Wang, Y. Yao and C. Yao, J. Mater. Chem. B, 2020, 8, 7494–7500 RSC.
- Y. Li, Y. Lu, X. Zhang, H. Cao and Y. Huang, ACS Appl. Nano Mater., 2021, 4, 9547–9556 CrossRef CAS.
- W. Dong, Y. Zhuang, S. Li, X. Zhang, H. Chai and Y. Huang, Sens. Actuators, B, 2018, 255, 2050–2057 CrossRef CAS.
- H. Fan and W. Shen, ChemSusChem, 2015, 8, 2004–2027 CrossRef CAS PubMed.
- Y. Wang and X. Jiang, ACS Appl. Mater. Interfaces, 2013, 5, 11597–11602 CrossRef CAS PubMed.
- Y. He, X. Zhuang, C. Lei, L. Lei, Y. Hou, Y. Mai and X. Feng, Nano Today, 2019, 24, 103–119 CrossRef CAS.
- X. Fan, F. Yang, J. Huang, Y. Yang, C. Nie, W. Zhao, L. Ma, C. Cheng, C. Zhao and R. Haag, Nano Lett., 2019, 19, 5885–5896 CrossRef CAS PubMed.
- J. Mou, X. Xu, F. Zhang, J. Xia and Z. Wang, ACS Appl. Bio Mater., 2020, 3, 664–672 CrossRef CAS PubMed.
- Z. Wang, H. Zhao, K. Chen, F. Zhou, S. Magdassi and M. Lan, Biosens. Bioelectron., 2022, 209, 114229 CrossRef CAS PubMed.
- B. Yuan, H. L. Chou and Y. K. Peng, ACS Appl. Mater. Interfaces, 2022, 14, 22728–22736 CrossRef CAS PubMed.
- S. W. Lv, N. Zhao, J. M. Liu, F. E. Yang, C. Y. Li and S. Wang, ACS Appl. Mater. Interfaces, 2021, 13, 25044–25052 CrossRef CAS PubMed.
- X. Liu, Q. Wang, Y. Zhang, L. Zhang, Y. Su and Y. Lv, New J. Chem., 2013, 37, 2174–2178 RSC.
- P. Ni, Y. Sun, H. Dai, J. Hu, S. Jiang, Y. Wang and Z. Li, Biosens. Bioelectron., 2015, 63, 47–52 CrossRef CAS PubMed.
- M. Y. Jia, L. Y. Niu, Y. Zhang, Q. Z. Yang, C. H. Tung, Y. F. Guan and L. Feng, ACS Appl. Mater. Interfaces, 2015, 7, 5907–5914 CrossRef CAS PubMed.
|
This journal is © The Royal Society of Chemistry 2023 |
Click here to see how this site uses Cookies. View our privacy policy here.