DOI:
10.1039/D3SC00957B
(Edge Article)
Chem. Sci., 2023,
14, 4038-4047
Transcriptome-wide identification of single-stranded RNA binding proteins†
Received
20th February 2023
, Accepted 7th March 2023
First published on 24th March 2023
Abstract
RNA–protein interactions are precisely regulated by RNA secondary structures in various biological processes. Large-scale identification of proteins that interact with particular RNA structure is important to the RBPome. Herein, a kethoxal assisted single-stranded RNA interactome capture (KASRIC) strategy was developed to globally identify single-stranded RNA binding proteins (ssRBPs). This approach combines RNA secondary structure probing technology with the conventional method of RNA-binding proteins profiling, realizing the transcriptome-wide identification of ssRBPs. Applying KASRIC, we identified 3180 candidate RBPs and 244 candidate ssRBPs in HeLa cells. Importantly, the 244 candidate ssRBPs contained 55 previously reported ssRBPs and 189 novel ssRBPs. Function analysis of the candidate ssRBPs exhibited enrichment in cellular processes related to RNA splicing and RNA degradation. The KASRIC strategy will facilitate the investigation of RNA–protein interactions.
Introduction
Cellular RNAs exhibit diverse biological functions, which are related to their flexible structures. RNAs usually form complex secondary and tertiary structures, which are governed by Watson–Crick base pairs or interactions with other biomolecules.1 Importantly, RNA binding proteins (RBPs) interact with RNAs throughout their life cycle via the recognition modules in RNAs, such as RNA structures, sequences, and modifications,2,3 which regulate gene expression, splicing, and RNA degradation.4–7 For example, CNBP, a well-known single-stranded RNA binding protein, promotes translation by resolving G4-RNA structures.8 Given the importance of RBPs for RNA structure regulation, the discrimination of protein binding to different RNA structures will benefit the study related to RNA–protein biology.
To date, there have been many progressive methods developed to investigate the binding sites of RNA and its secondary structures in RNA–protein interactions.9–15 Meanwhile, there are also numerous progressive methods that have been developed for transcriptome-wide RBP identification, which combine UV cross-linking with diverse enrichment methods such as poly(A)-dependent capture,16,17 click reaction-based affinity enrichments,18,19 and phase separation-based enrichments.20,21 Aside from experimental methods, a progressive sequence-based approach RBPPred was developed to predict 6657 possible RBPs using computational prediction methods.22 Although there have been various advanced methods developed for RBP identification, experimental methods enabling the transcriptome-wide identification of RBP binding to particular RNA structures are needed. To date, the main methods developed for the identification of ssRBPs include electrophoretic mobility shift assay (EMSA),23 X-ray crystallography,24 nuclear magnetic resonance (NMR),25 and computational predictions,12,26 which provide precise insight into RNA–protein interactions. However, the implementation of these methods primarily relies on prior knowledge of the sequence or structure of RNA and protein, which may be difficult to obtain. To complete the repertoire of ssRBPs, a novel approach enabling the large-scale identification of ssRBPs is necessary.
A number of probes, such as dimethyl sulfate (DMS),27 2-methylnicotinic acid imidazolide-azido (NAI-N3),28 glyoxal,29 and 1-ethyl-3-(3-dimethylaminopropyl)carbodiimide (EDC),30 have been developed for RNA structure mapping. Recently, our group and co-workers found that N3-kethoxal could efficiently label the Watson–Crick interface of unpaired guanines in single-stranded RNAs (ssRNAs) and cause limited protein modifications,31 making it a powerful tool to profile transcriptome-wide RNA secondary structures and intervene in the interaction between ssRNAs and ssRBPs. Herein, combining an N3-kethoxal labeling method with an mRNA interactome capture approach,16,31 we developed a kethoxal assisted single-stranded RNA interactome capture (KASRIC) strategy for the transcriptome-wide identification of ssRBPs. Using KASRIC, we identified 244 candidate ssRBPs, providing a valuable resource for the research of RNA–protein biology.
Results and discussion
Development of a KASRIC strategy for ssRBP identification
Many ssRBPs make contact with RNA bases via hydrogen bonds or stacking interactions that exist at the interface of RNA–protein complexes.32,33 However, double-stranded RNA binding proteins (dsRBPs) recognize target RNAs in a largely sequence independent way by interacting with the RNA backbone and minor groove.34 Herein, an N3-kethoxal was used to specifically label unpaired guanines in ssRNAs. The specific labeling of ssRNAs with the N3-kethoxal disrupts the contact between the bases and amino acid residues, blocking the binding of ssRBPs to ssRNAs. Therefore, when cells were subjected to 254 nm UV irradiation, the level of cross-linking of RNAs and ssRBPs reduced in N3-kethoxal treated cells compared to in untreated cells, whereas the cross-linking of RNAs and dsRBPs remained unaffected. Then, cross-linked RNA–protein complexes were isolated using oligo(dT) magnetic beads, and proteins were quantified by MS after being released by RNase treatment. Proteins with reduced MS intensity in N3-kethoxal and UV treated samples (Block-cCL), compared to UV treated samples (cCL), were identified as ssRBPs (Fig. 1).
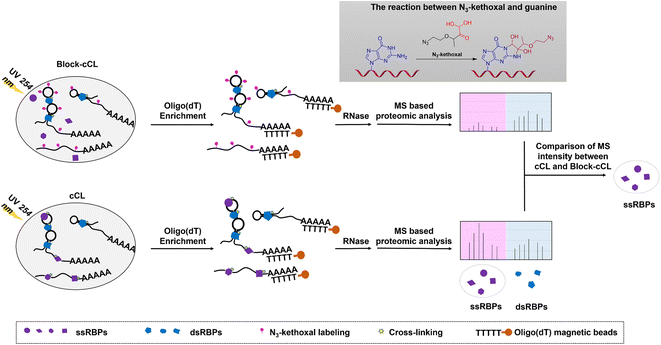 |
| Fig. 1 Schematic representation of the KASRIC procedure. ssRNAs were labeled with N3-kethoxal specifically, which blocked the binding of ssRBPs to ssRNAs, and prevented the cross-linking of ssRBPs and ssRNAs. After 254 nm UV irradiation, fewer ssRBPs were isolated by oligo(dT) magnetic beads in N3-kethoxal treated samples (Block-cCL) compared to untreated samples (cCL). Then, ssRBPs were identified by quantitative proteomic analysis by comparing the MS peak intensity between N3-kethoxal treated and untreated samples. Proteins with reduced MS intensity in Block-cCL compared to cCL were identified as ssRBPs. | |
It needs to be noted that only ssRBPs that dynamically bind to ssRNAs could be identified. In the KASRIC strategy, the identification of ssRBPs heavily relied on the N3-kethoxal labeling of RNA. Proteins that bind RNA stably would prevent N3-kethoxal to label RNA, and thus were failed to be identified. To identify as many ssRBPs as possible, we incubated HeLa cells with N3-kethoxal for a long period of time, so that more ssRBPs could bind to target RNAs dynamically. The concentration and incubation time of N3-kethoxal were set to be 5 mM and 30 min, respectively, which were determined by MTT assay to minimize the disturbance induced by N3-kethoxal treatment (Fig. S1†).
Experimental validation of the KASRIC strategy
We initially tested this strategy by EMSA to explore how the labeling of N3-kethoxal influences the binding affinity of protein to its target RNA. The recombinant proteins, CNBP and TRBP, which are known to bind to ssRNA and double-stranded RNA (dsRNA), respectively,35,36 were expressed and purified (Fig. S2†). The binding affinity assays of the purified proteins verified that CNBP specifically binds to ssRNA with G-rich elements and TRBP preferentially binds to dsRNA (Fig. S3†). Additionally, the selective reaction activity of N3-kethoxal to ssRNA was also verified (Fig. S4†). When RNA was treated with N3-kethoxal before being incubated with the corresponding protein, the binding affinity of CNBP significantly declined in a concentration dependent manner, whereas TRBP showed no obvious change (Fig. 2a and b). These results indicated that N3-kethoxal could successfully block the binding of ssRBP to ssRNA and have a rare influence over the binding of dsRBP to dsRNA. Considering the possible reactivity of N3-kethoxal with lysine, cysteine, and arginine residues in proteins,37 we investigated whether protein modification caused by N3-kethoxal would influence the binding affinity of protein to RNA. Gratifyingly, pretreating proteins (either CNBP or TRBP) with N3-kethoxal had no visible impact on their binding affinity towards the target RNA (Fig. S5†), which was probably due to the lower reaction activity or accessibility of these amino acid residues towards N3-kethoxal.
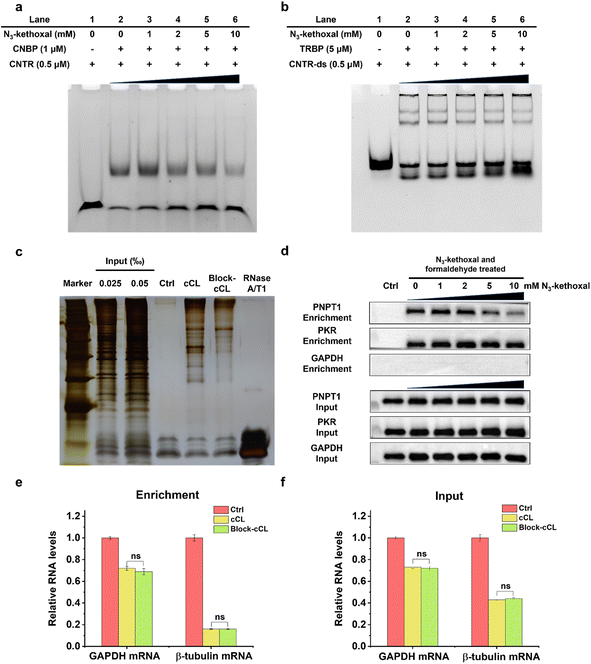 |
| Fig. 2 Characterization of N3-kethoxal labeling. (a) EMSA of CNBP and ssRNA (CNTR). First, ssRNA (0.5 μM) was treated with increasing concentrations of N3-kethoxal, and then incubated in the presence or absence of 1 μM CNBP. (b) EMSA of TRBP and dsRNA (CNTR-ds). dsRNA (0.5 μM) was annealed from CNTR and its complementary RNA, and treated with increasing concentrations of N3-kethoxal before being incubated with 5 μM of TRBP. (c) SDS-PAGE analysis of proteins purified by oligo(dT) magnetic beads. Cells were incubated in the presence of 5 mM of N3-kethoxal for 30 min (Block-cCL) or absence (cCL), and RNA–protein complexes were isolated using oligo(dT) magnetic beads. Isolated proteins were visualized by silver staining. (d) Western blot analysis of PNPT1 and PKR in cell lysates (input) and elution solution (enrichment). Cells were treated with increasing concentrations of N3-kethoxal for 30 min and treated in the presence or absence of formaldehyde. GAPDH was used as a negative control. (e and f) RT-qPCR analysis of GAPDH and β-tubulin mRNA in (e) enrichment and (f) input. Error bars represent the mean ± SD of three biological experiments. Significance was assessed by two-tailed Student's t-test (ns, not significant). | |
Given the satisfying results in vitro, we examined the blocking efficiency of N3-kethoxal in HeLa cells. Firstly, oligo(dT) isolated RBPs were analyzed by sodium dodecyl sulfate polyacrylamide gel electrophoresis (SDS-PAGE) and silver staining (Fig. 2c). Clear protein bands could be observed in both cCL and Block-cCL. However, there was a significant reduction in the intensities and numbers of bands observed in Block-cCL compared to cCL, indicating the successful blocking of proteins in Block-cCL. Furthermore, a known ssRBP PNPT1 and a known dsRBP PKR were selected to examine in vivo N3-kethoxal blocking efficiency by western blot. The enrichment abundance of PNPT1 negatively correlated with the concentration of N3-kethoxal, whereas the enrichment abundance of PKR remained unaffected by N3-kethoxal even at a concentration of 10 mM (Fig. 2d). Meanwhile, the abundance of both PNPT1 and PKR in the input was not influenced by N3-kethoxal, suggesting that N3-kethoxal treatment did not affect native protein levels. RNA analysis revealed that mRNAs were substantially enriched to a similar degree between different samples (Fig. S6†). RT-qPCR exhibited that GAPDH and β-tubulin mRNA were effectively deleted by oligo(dT) capture (Fig. S7†), and both had almost equal RNA abundance in cCL and Block-cCL (Fig. 2e and f), indicating that N3-kethoxal treatment did not influence enriched RNA abundance.
All these results indicated that N3-kethoxal successfully blocked the binding of ssRBP to ssRNA and did not affect the binding of dsRBP to dsRNA. Meanwhile, N3-kethoxal exhibited a limited effect towards native protein levels and RNA levels, showing the feasibility of using the KASRIC strategy to identify ssRBP.
Proteome identification of proteins captured by KASRIC
In the case of proteomic analysis, proteins isolated by oligo(dT) magnetic beads were released from RNA–protein complexes using RNase and digested into peptides with trypsin, which were further analyzed by liquid chromatography-tandem mass spectrometry (LC-MS/MS). Protein abundance was assessed by data-independent acquisition (DIA) mass spectrometry (Dataset S1). DIA is an emerging quantitative technique that shows excellent reproducibility and accuracy in quantification,38 making it valuable for ssRBP identification. In summary, 3824, 3862, and 670 proteins were identified and quantified at least twice in three independent replicates of cCL, Block-cCL, and control, respectively (Fig. S8†). And, the quantitative value showed a strong correlation between three biological replicates (Fig. 3a). 3180 proteins were quantified both in cCL and Block-cCL, and meanwhile enriched in cCL with a fold change of ≥3 and a p value of <0.01 compared to the control (Fig. 3b and c). We considered these enriched 3180 proteins as candidate RBPs identified by KASRIC (Dataset S2).
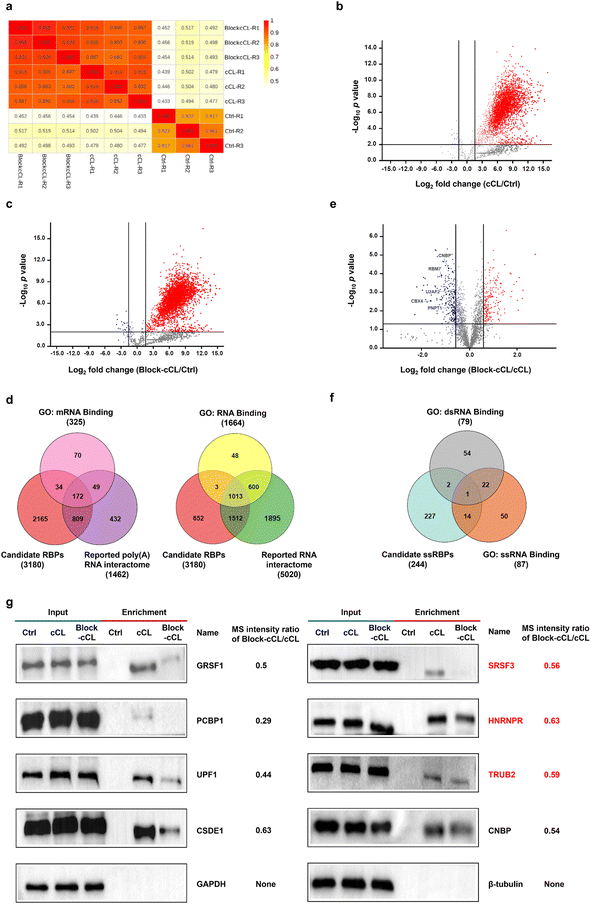 |
| Fig. 3 Proteomic analysis of proteins isolated by KASRIC. (a) Pearson correlation coefficient of different samples. (b and c) Volcano plot displaying the log2 fold change and −log10p value of the identified proteins between (b) cCL and Ctrl and (c) Block-cCL and Ctrl. Proteins with a fold change of ≥3 and a p value <0.01 were considered as being significantly enriched. (d) Venn diagram showing the overlap of 3180 candidate RBPs with known poly(A) RNA interactome or RNA interactome. (e) Volcano plot displaying quantitative proteomic comparison of high-confidence RBPs quantified in Block-cCL and cCL. Proteins with a p value <0.05 and a minimum of 1.5-fold down-regulation in Block-cCL were considered as candidate ssRBPs (blue). (f) Venn diagram showing the overlap of the candidate ssRBPs with GO annotated ssRBPs and GO annotated dsRBPs. (g) Western blot analysis showing the abundance of several candidate ssRBPs in cell lysates (input) and elution solution (enrichment). β-Tubulin and GAPDH were used as a negative control. Novel ssRBPs are marked in red. | |
To further analyze the fidelity of the 3180 candidate RBPs, we integrated human RBPs that had been identified by previous experimental methods, producing a poly(A) RNA interactome containing 1462 proteins (Dataset S3),16–20,39–42 and a RNA interactome containing 5020 proteins (Dataset S4).18–20,43 Among the 3180 candidate RBPs identified by KASRIC, 1015 proteins (32%) overlapped with previously reported poly(A) RNA interactome or mRNA binding proteins (mRBPs) datasets in gene ontology (GO) (Fig. 3d), covering 67% of reported poly(A) RNA interactome and 63% of GO annotated mRBPs, respectively. And, 2528 proteins (79%) overlapped with reported RNA interactome or RBPs datasets in GO (Fig. 3d). Taken together, 2529 candidate RBPs overlapped with datasets reported using previous experimental methods, which we referred to as high-confidence RBPs (Dataset S5). Importantly, 651 novel RBPs were identified by KASRIC (Dataset S5). These data suggested that the majority of proteins captured by KASRIC were valid RBPs, indicating that the KASRIC strategy was credible for identifying RBPs.
In this work, we mainly intended to identify novel ssRBPs. For the reliability of ssRBP identification, only 2529 high-confidence RBPs were taken into account for further screening of the ssRBPs. Under the blocking of N3-kethoxal, the quantification of ssRBPs was less for Block-cCL than for cCL. In this work, RBPs which were down-regulated by 1.5 fold and greater with a p value of <0.05 in Block-cCL compared to cCL, were regarded as candidate ssRBPs (Fig. 3e). In summary, we identified 244 candidate ssRBPs (Dataset S6), which included 15 GO annotated ssRBPs (Fig. 3f), such as CNBP, RBM7, U2AF2, CBX4, and PNPT1 (Fig. 3e). What is more, another 40 ssRBPs that had been reported in previous studies but not annotated in GO datasets were also contained by 244 candidate ssRBPs. Taken together, we identified 55 high-confidence ssRBPs that had been reported in previous studies (Dataset S6). Importantly, 189 novel ssRBPs were identified by KASRIC (Dataset S6). The MS intensity changes between Block-cCL and cCL of several candidate ssRBPs were verified by western blots. All the candidate ssRBPs, including three novel ssRBPs, showed less intensity in Block-cCL compared to cCL (Fig. 3g). Additionally, three GO annotated dsRBPs (MBNL1, SUPV3L1, and EIF4B) were included by the 244 candidate ssRBPs, all of which also exhibited binding activity to ssRNA, which has been reported in a range of studies (Fig. 3f).44–48 These results implied that some RBPs have multiple binding patterns, which is consistent with previous studies.12
To exclude the disturbance caused by changes in native protein levels, we also investigated the influence of N3-kethoxal treatment on native protein levels. Proteins in the HeLa whole-cell lysates treated or untreated with N3-kethoxal were quantified by MS (Dataset S7). 5220 proteins were quantified at least twice in both cCL and Block-cCL. 5104 proteins (98%) showed equal quantification values even at a stringent screening cut-off with a fold change of ≥1.2 (either increase or decrease) and a p value of <0.05 (Fig. S9†). 186 out of 244 candidate ssRBPs were quantified in whole-cell lysates, all of which showed no significant down-regulation in Block-cCL compared to cCL (Dataset S7), excluding the possibility that N3-kethoxal treatment influenced native protein levels.
Functional analysis of proteins captured by KASRIC
We performed GO analysis and Kyoto Encyclopedia of Genes and Genomes (KEGG) pathway analysis of the 2529 high-confidence RBPs and 244 candidate ssRBPs identified by KASRIC using Metascape.49 “RNA binding” related GO terms were significantly overrepresented in both high-confidence RBPs and candidate ssRBPs (Fig. 4a–d). However, both “single-stranded RNA binding” and “double-stranded RNA binding” GO terms were significantly enriched in 2529 high-confidence RBPs (Fig. 4a), suggesting that conventional mRNA interactome capture methods could not enable the discrimination of ssRBPs and dsRBPs. Whereas, only a “single-stranded RNA binding” GO term was significantly overrepresented in candidate ssRBPs (Fig. 4b), verifying that KASRIC was an efficient method to selectively identify ssRBPs.
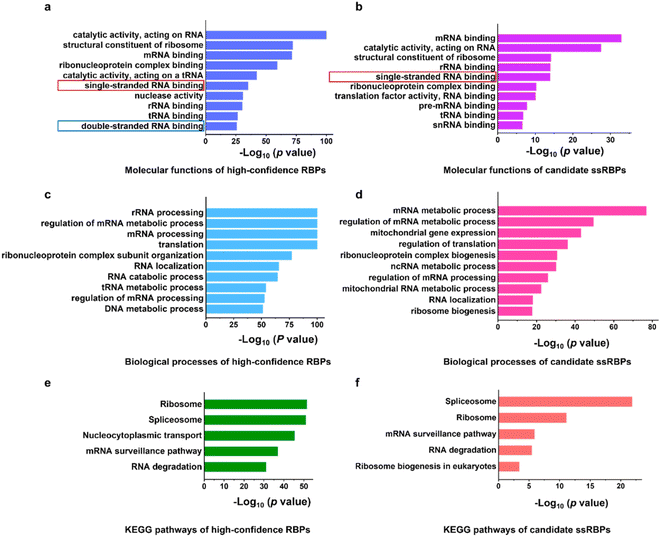 |
| Fig. 4 Functional characterization of proteins captured by KASRIC. (a and b) Molecular function GO terms enriched in (a) high-confidence RBPs and (b) candidate ssRBPs. (c and d) Biological process GO terms enriched in (c) high-confidence RBPs and (d) candidate ssRBPs. (e and f) KEGG pathway analysis of (e) high-confidence RBPs and (f) candidate ssRBPs. | |
With the insight into the function of the candidate ssRBPs, it is intriguing that mitochondrial gene expression and RNA metabolic process related GO terms were obviously enriched in the candidate ssRBPs (Fig. 4d), implying that ssRBPs play essential roles in mitochondrial RNA biology regulation. However, it was unclear whether there were biases caused by cellular metabolism disorder which may be induced by N3-kethoxal. Hence, more comprehensive study was required to reveal the potential function of ssRBPs in mitochondria.
KEGG pathway analysis revealed the specific function of high-confidence RBPs and candidate ssRBPs (Fig. 4e and f). The KEGG pathways of candidate ssRBPs were highly concentrated. Spliceosome were mostly overrepresented in candidate ssRBPs, which included several reported ssRBPs, such as DHX8,50 FUS,51 PCBP1,52 and U2AF2. Other KEGG terms of candidate ssRBPs revealed a prominent correlation with mRNA surveillance and degradation. Three out of the top ten down-regulated proteins in Block-cCL were involved in mRNA decay. Current research has revealed that two general mRNA decay pathways heavily rely on the 5′ → 3′ exonuclease activity of XRN1 and the 3′ → 5′ nuclease activity of exosome.53–55 Both pathways conduct processive single-stranded hydrolysis. Considering the complicated secondary structures of mRNAs in cells, ssRBPs could play important roles in mRNA decay by regulating the single-stranded RNA structure. Indeed, a processive RNA helicase UPF1 involved in the nonsense-mediated mRNA decay (NMD) pathway was significantly identified as ssRBPs in our work (2-fold greater down-regulated in Block-cCL). UPF1 was found to translocate over long ssRNAs, and thus unwind the double-stranded RNA structure and remodel RNA–protein interactions, thus accelerating mRNA decay.56
Profiles of RNA binding domains
Domain analysis of the identified RBPs was performed according to the Pfam database. Most of the high-confidence RBPs and candidate ssRBPs contained classical RNA binding domains (RBDs), non-classical RBDs, and several unknown RBDs (Fig. 5a–c). What is more, several classical RBDs such as RRM, KH, and zf-CCCH, which are widely accepted to bind to ssRNA,32 were significantly overrepresented in the candidate ssRBPs. However, DSRM that binds to dsRNA specifically was only overrepresented in the high-confidence RBPs.
 |
| Fig. 5 RBDs representation of proteins identified by KASRIC. (a, b, and c) Number of identified proteins containing (a) classical RBDs, (b) non-classical RBDs, and (c) unknown RBDs. (d) Average amino acid frequency in RBDs contained by identified proteins. The percentage of every amino acid in the individual RBDs was calculated. The mean and median values of amino acid frequency among the different classified RBDs are represented. | |
We also calculated the average amino acid frequency in the identified RBDs. The results showed no significant difference between the amino acid composition of the high-confidence RBPs and candidate ssRBPs (Fig. 5d). Tyrosine (Y), tryptophan (W), and histidine (H) were underrepresented in the identified RBDs, which is consistent with previous research.57 Gratifyingly, in our work, arginine, lysine, and cysteine showed almost similar amino acid frequency in the RBDs contained in the candidate ssRBPs and high-confidence RBPs, implying that the candidate ssRBPs identified by KASRIC showed no preference to the proteins enriched with arginine, lysine, and cysteine. The results highlighted the previous point that N3-kethoxal caused limited protein modification, which may be disrupted ssRBP identification. Certainly, the amino acid modification in every individual candidate ssRBP required meticulous investigation to greatly eliminate the biases caused by protein modification.
Validating ssRNA binding activity of the candidate ssRBPs
Previously, the RNA secondary structures in RNA binding sites of the 168 RBPs were profiled using the PrismNet method, which integrates RNA structural data with CLIP-seq data to accurately predict dynamic RBP binding.12 Twenty of our candidate ssRBPs were predicted to prefer the ssRNA structure in transcriptome. Additionally, altogether there were 55 high-confidence ssRBPs identified by KASRIC, which contained 15 GO annotated ssRBPs and 40 ssRBPs that had been reported by a range of studies but not annotated in GO datasets (Dataset S6). The data proves that KASRIC enables the identification of 55 known ssRBPs.
To further verify that the candidate ssRBPs we identified were reliable, we performed EMSA experiments to validate the ssRNA binding activities of several candidate ssRBPs. We successfully expressed three proteins: MBNL1, SUPV3L1, and SRP19 (Fig. S10†). MBNL1 and SUPV3L1 were annotated as dsRBP in GO datasets; however, previous studies revealed that they probably also have ssRNA binding activity.45,46 SRP19, a novel ssRBP identified by KASRIC, is known to recognize a particular stem-loop RNA structure of signal recognition particle (SRP) RNA.58 EMSA experiments showed that there were obvious ssRNA bands that mobilized slower under electrophoresis (Fig. 6), revealing that all the proteins possessed ssRNA binding activity. The novel ssRBP SRP19 preferred to bind ssRNA strongly. As reported, MBNL1 and SUPV3L1 apparently possessed both ssRNA and dsRNA binding activities; however, they exhibited stronger binding activity toward ssRNA. The results, consistent with previous study,12 indicated that some RBPs have multiple binding patterns, and that work needs to be done to reveal the mechanism of RNA–protein interactions. The upper results confirm that we have developed an efficient strategy for discovering novel ssRBPs.
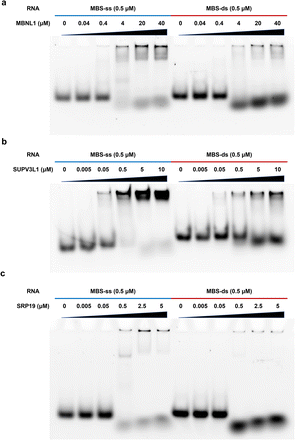 |
| Fig. 6 Validation of candidate ssRBPs by EMSA. (a) EMSA of MBNL1 and ssRNA or dsRNA. (b) EMSA of SUPV3L1 and ssRNA or dsRNA. (c) EMSA of SRP19 and ssRNA or dsRNA. dsRNA was annealed from two complementary RNA. RNAs were incubated with proteins for 30 min and separated using a non-denaturing polyacrylamide gel. | |
Conclusions
In summary, we have demonstrated the use of KASRIC for the transcriptome-wide identification of ssRBPs. N3-kethoxal labels unpaired guanines in ssRNAs with satisfying reaction efficiency, making it a powerful tool to investigate ssRBPs. Our studies verified that N3-kethoxal modification of ssRNAs successfully blocked the binding of ssRBPs to ssRNAs. Applying KASRIC, we identified 244 credible candidate ssRBPs, which contained 55 reported ssRBPs and 189 novel ssRBPs. KASRIC substantially complemented the current list of ssRBPs. The ssRNA binding activity of three candidate ssRBPs was validated by EMSA, including two controversial RBPs (MBNL1 and SUPV3L1) and one novel ssRBP (SRP19). Function analysis of the candidate ssRBPs showed a significant overrepresentation of the “single-stranded RNA binding” GO term, and revealed that candidate ssRBPs could play important roles in biological processes related to RNA splicing and RNA degradation.
The ssRBPs dataset generated by KASRIC could serve as a reference in the investigation of RNA–protein interactions. Our method, together with other advanced techniques, will offer new insights into RNA–protein biology. Further efforts should be made to optimize the blocking efficiency of the probe, which could facilitate the identification of ssRBPs.
Data availability
Raw mass spectrometry data are available via ProteomeXchange using the identifier PXD038757.
Author contributions
X. B., X. W., and X. Z. supervised the project. R. Z., R. X., and X. W. designed the experiments. R. Z., X. F., and X. C. performed all the experiments. R. Z. and Z. M. completed data analysis. J. M. and Y. L. contributed to the design of the experiments. R. Z. and X. F. wrote the manuscript with the help of X. B., X. W., and X. Z.
Conflicts of interest
There are no conflicts to declare.
Acknowledgements
This work was supported by the National Natural Science Foundation of China (92253202, 22177087 to X. W.; 92153303, 21721005 to X. Z.) and the MOST National Key R&D Program of China (2021YFA1100401).
Notes and references
- R. C. Spitale, P. Crisalli, R. A. Flynn, E. A. Torre, E. T. Kool and H. Y. Chang, Nat. Chem. Biol., 2013, 9, 18–20 CrossRef CAS PubMed.
- M. Corley, M. C. Burns and G. W. Yeo, Mol. Cell, 2020, 78, 9–29 CrossRef CAS PubMed.
- X. W. Wang, C. X. Liu, L. L. Chen and Q. C. Zhang, Nat. Chem. Biol., 2021, 17, 755–766 CrossRef CAS PubMed.
- M. Mueller-McNicoll and K. M. Neugebauer, Nat. Rev. Genet., 2013, 14, 275–287 CrossRef CAS PubMed.
- A. Addetia, N. A. P. Lieberman, Q. Phung, T. Y. Hsiang, H. Xie, P. Roychoudhury, L. Shrestha, M. A. Loprieno, M. L. Huang, M. Gale, K. R. Jerome and A. L. Greninger, Mbio, 2021, 12, e00065 CrossRef CAS PubMed.
- L. Liu, C. Luo, Y. Luo, L. Chen, Y. Liu, Y. Wang, J. Han, Y. Zhang, N. Wei, Z. Xie, W. Wu, G. Wu and Y. Feng, Oncogene, 2018, 37, 86–94 CrossRef CAS PubMed.
- X. Y. Wang, X. J. Ge, W. Liao, Y. Cao, R. Li, F. Zhang, B. Zhao and J. Du, Cell Commun. Signaling, 2021, 19, 85 CrossRef CAS PubMed.
- D. Benhalevy, S. K. Gupta, C. H. Danan, S. Ghosal, H. W. Sun, H. G. Kazemier, K. Paeschke, M. Hafner and S. A. Juranek, Cell Rep., 2017, 18, 2979–2990 CrossRef CAS PubMed.
- J. F. Zheng, P. J. Kundrotas, I. A. Vakser and S. Y. Liu, PLoS Comput. Biol., 2016, 12, e1005120 CrossRef PubMed.
- J. Xie, J. F. Zheng, X. Hong, X. X. Tong and S. Y. Liu, Commun. Biol., 2020, 3, 384 CrossRef CAS PubMed.
- J. K. Wei, S. Y. Chen, L. C. Zong, X. Gao and Y. Li, Briefings Bioinf., 2022, 23, bbab540 CrossRef PubMed.
- L. Sun, K. Xu, W. Z. Huang, Y. C. T. Yang, P. Li, L. Tang, T. L. Xiong and Q. F. C. Zhang, Cell Res., 2021, 31, 495–516 CrossRef CAS PubMed.
- M. Corley, R. A. Flynn, B. Lee, S. M. Blue, H. Y. Chang and G. W. Yeo, Mol. Cell, 2020, 80, 903–914 CrossRef CAS PubMed.
- D. Dominguez, P. Freese, M. S. Alexis, A. Su, M. Hochman, T. Palden, C. Bazile, N. J. Lambert, E. L. Van Nostrand, G. A. Pratt, G. W. Yeo, B. R. Graveley and C. B. Burge, Mol. Cell, 2018, 70, 854–867.e9 CrossRef CAS PubMed.
- D. Maticzka, S. J. Lange, F. Costa and R. Backofen, Genome Biol., 2014, 15, R17 CrossRef PubMed.
- A. Castello, B. Fischer, K. Eichelbaum, R. Horos, B. M. Beckmann, C. Strein, N. E. Davey, D. T. Humphreys, T. Preiss, L. M. Steinmetz, J. Krijgsveld and M. W. Hentze, Cell, 2012, 149, 1393–1406 CrossRef CAS PubMed.
- A. G. Baltz, M. Munschauer, B. Schwanhaeusser, A. Vasile, Y. Murakawa, M. Schueler, N. Youngs, D. Penfold-Brown, K. Drew, M. Milek, E. Wyler, R. Bonneau, M. Selbach, C. Dieterich and M. Landthaler, Mol. Cell, 2012, 46, 674–690 CrossRef CAS PubMed.
- X. C. Bao, X. P. Guo, M. H. Yin, M. Tariq, Y. W. Lai, S. Kanwal, J. J. Zhou, N. Li, Y. Lv, C. Pulido-Quetglas, X. W. Wang, L. Ji, M. J. Khan, X. H. Zhu, Z. W. Luo, C. W. Shao, D. H. Lim, X. Liu, N. Li, W. Wang, M. H. He, Y. L. Liu, C. Ward, T. Wang, G. Zhang, D. Y. Wang, J. H. Yang, Y. W. Chen, C. L. Zhang, R. Jauch, Y. G. Yang, Y. M. Wang, B. M. Qin, M. L. Anko, A. P. Hutchins, H. Sun, H. T. Wang, X. D. Fu, B. L. Zhang and M. A. Esteban, Nat. Methods, 2018, 15, 213–220 CrossRef CAS PubMed.
- R. B. Huang, M. T. Han, L. Y. Meng and X. Chen, Proc. Natl. Acad. Sci. U. S. A., 2018, 115, E3879–E3887 CAS.
- J. Trendel, T. Schwarzl, R. Horos, A. Prakash, A. Bateman, M. W. Hentze and J. Krijgsveld, Cell, 2019, 176, 391–403.e319 CrossRef CAS PubMed.
- R. M. L. Queiroz, T. Smith, E. Villanueva, M. Marti-Solano, M. Monti, M. Pizzinga, D.-M. Mirea, M. Ramakrishna, R. F. Harvey, V. Dezi, G. H. Thomas, A. E. Willis and K. S. Lilley, Nat. Biotechnol., 2019, 37, 692 CrossRef CAS PubMed.
- X. L. Zhang and S. Y. Liu, Bioinformatics, 2017, 33, 854–862 CrossRef CAS PubMed.
- H. Hohjoh and M. F. Singer, EMBO J., 1997, 16, 6034–6043 CrossRef CAS PubMed.
- O. Weichenrieder, K. Wild, K. Strub and S. Cusack, Nature, 2000, 408, 167–173 CrossRef CAS PubMed.
- C. Dominguez, J. F. Fisette, B. Chabot and F. H. T. Allain, Nat. Struct. Mol. Biol., 2010, 17, 853 CrossRef CAS PubMed.
- A. Pal and Y. Levy, PLoS Comput. Biol., 2019, 15, e1006768 CrossRef CAS PubMed.
- P. Tijerina, S. Mohr and R. Russell, Nat. Protoc., 2007, 2, 2608–2623 CrossRef CAS PubMed.
- R. C. Spitale, R. A. Flynn, Q. C. Zhang, P. Crisalli, B. Lee, J. W. Jung, H. Y. Kuchelmeister, P. J. Batista, E. A. Torre, E. T. Kool and H. Y. Chang, Nature, 2015, 527, 264 CrossRef CAS PubMed.
- D. Mitchell, L. E. Ritchey, H. Park, P. Babitzke, S. M. Assmann and P. C. Bevilacqua, RNA, 2018, 24, 114–124 CrossRef CAS PubMed.
- D. Mitchell, A. J. Renda, C. A. Douds, P. Babitzke, S. M. Assmann and P. C. Bevilacqua, RNA, 2019, 25, 147–157 CrossRef CAS PubMed.
- X. C. Weng, J. Gong, Y. Chen, T. Wu, F. Wang, S. X. Yang, Y. S. Yuan, G. Z. Luo, K. Chen, L. L. Hu, H. H. Ma, P. L. Wang, Q. F. C. Zhang, X. Zhou and C. He, Nat. Chem. Biol., 2020, 16, 489–492 CrossRef CAS PubMed.
- S. D. Auweter, F. C. Oberstrass and F. H. T. Allain, Nucleic Acids Res., 2006, 34, 4943–4959 CrossRef CAS PubMed.
- A. C. Messias and M. Sattler, Acc. Chem. Res., 2004, 37, 279–287 CrossRef CAS PubMed.
-
S. Hur, in Annual Review of Immunology, ed. W. M. Yokoyama, 2019, vol. 37, pp. 349–375 Search PubMed.
- P. Armas, S. Nasif and N. B. Calcaterra, J. Cell. Biochem., 2008, 103, 1013–1036 CrossRef CAS PubMed.
- X. L. Wang, L. Vukovic, H. R. Koh, K. Schulten and S. Myong, Nucleic Acids Res., 2015, 43, 7566–7576 CrossRef CAS PubMed.
- M. A. Gauthier and H. A. Klok, Biomacromolecules, 2011, 12, 482–493 CrossRef CAS PubMed.
- J. Zhao, Y. Yang, H. Xu, J. Zheng, C. Shen, T. Chen, T. Wang, B. Wang, J. Yi, D. Zhao, E. Wu, Q. Qin, L. Xia and L. Qiao, npj Biofilms Microbiomes, 2023, 9, 4 CrossRef PubMed.
- A. Castello, B. Fischer, C. K. Frese, R. Horos, A. M. Alleaume, S. Foehr, T. Curk, J. Krijgsveld and M. W. Hentze, Mol. Cell, 2016, 63, 696–710 CrossRef CAS PubMed.
- B. M. Beckmann, R. Horos, B. Fischer, A. Castello, K. Eichelbaum, A. M. Alleaume, T. Schwarzl, T. Curk, S. Foehr, W. Huber, J. Krijgsveld and M. W. Hentze, Nat. Commun., 2015, 6, 10127 CrossRef CAS PubMed.
- T. Conrad, A. S. Albrecht, V. R. D. Costa, S. Sauer, D. Meierhofer and U. A. Orom, Nat. Commun., 2016, 7, 11212 CrossRef CAS PubMed.
- M. Milek, K. Imami, N. Mukherjee, F. De Bortoli, U. Zinnall, O. Hazapis, C. Trahan, M. Oeffinger, F. Heyd, U. Ohler, M. Selbach and M. Landthaler, Genome Res., 2017, 27, 1344–1359 CrossRef CAS PubMed.
- Z. Zhang, T. Liu, H. Y. Dong, J. Li, H. F. Sun, X. H. Qian and W. J. Qin, Nucleic Acids Res., 2021, 49, e65 CrossRef CAS PubMed.
- D. Cass, R. Hotchko, P. Barber, K. Jones, D. P. Gates and J. A. Berglund, BMC Mol. Biol., 2011, 12, 20 CrossRef CAS PubMed.
- M. Teplova and D. J. Patel, Nat. Struct. Mol. Biol., 2008, 15, 1343–1351 CrossRef CAS PubMed.
- D. D. H. Wang, X. E. Guo, A. S. Modrek, C. F. Chen, P. L. Chen and W. H. Lee, J. Biol. Chem., 2014, 289, 16727–16735 CrossRef PubMed.
- M. Jain, B. Golzarroshan, C. L. Lin, S. Agrawal, W. H. Tang, C. J. Wu and H. S. Yuan, Protein Sci., 2022, 31, e4312 CrossRef CAS PubMed.
- G. W. Rogers, N. J. Richter, W. F. Lima and W. C. Merrick, J. Biol. Chem., 2001, 276, 30914–30922 CrossRef CAS PubMed.
- Y. Zhou, B. Zhou, L. Pache, M. Chang, A. H. Khodabakhshi, O. Tanaseichuk, C. Benner and S. K. Chanda, Nat. Commun., 2019, 10, 1523 CrossRef PubMed.
- C. Felisberto-Rodrigues, J. C. Thomas, C. McAndrew, Y. V. Le Bihan, R. Burke, P. Workman and R. L. M. van Montfort, Biochem. J., 2019, 476, 2521–2543 CrossRef CAS PubMed.
- X. Y. Wang, J. C. Schwartz and T. R. Cech, Nucleic Acids Res., 2015, 43, 7535–7543 CrossRef CAS PubMed.
- A. V. Makeyev and S. A. Liebhaber, RNA, 2002, 8, 265–278 CrossRef CAS PubMed.
- R. Parker and H. W. Song, Nat. Struct. Mol. Biol., 2004, 11, 121–127 CrossRef CAS PubMed.
- M. Jinek, S. M. Coyle and J. A. Doudna, Mol. Cell, 2011, 41, 600–608 CrossRef CAS PubMed.
- F. Bonneau, J. Basquin, J. Ebert, E. Lorentzen and E. Conti, Cell, 2009, 139, 547–559 CrossRef CAS PubMed.
- F. Fiorini, D. Bagchi, H. Le Hir and V. Croquette, Nat. Commun., 2015, 6, 7581 CrossRef PubMed.
- D. M. Kruger, S. Neubacher and T. N. Grossmann, RNA, 2018, 24, 1457–1465 CrossRef PubMed.
- K. Wild, I. Sinning and S. Cusack, Science, 2001, 294, 598–601 CrossRef CAS PubMed.
|
This journal is © The Royal Society of Chemistry 2023 |
Click here to see how this site uses Cookies. View our privacy policy here.