DOI:
10.1039/D2SD00109H
(Paper)
Sens. Diagn., 2023,
2, 90-99
Analyzing bronchoalveolar fluid derived small extracellular vesicles using single-vesicle SERS for non-small cell lung cancer detection
Received
23rd June 2022
, Accepted 24th October 2022
First published on 30th October 2022
Abstract
An emerging body of research by biologists and clinicians has demonstrated the clinical application of small extracellular vesicles (sEVs, also commonly referred to as exosomes) as biomarkers for cancer detections. sEVs isolated from various body fluids such as blood, saliva, urine, and cerebrospinal fluid have been used for biomarker discoveries with highly encouraging outcomes. Among the biomarkers discovered are those responsible for multiple cancer types and immune responses. These biomarkers are recapitulated from the tumor microenvironments. Yet, despite numerous discussions of sEVs in scientific literature, sEV-based biomarkers have so far played only a minor role for cancer diagnostics in the clinical setting, notably less so than other techniques such as imaging and biopsy. In this paper, we report the results of a pilot study (n = 10 from each of the patient and the control group) using bronchoalveolar lavage fluid to determine the presence of sEVs related to non-small cell lung cancer in twenty clinical samples examined using surface enhanced Raman spectroscopy (SERS).
Introduction
Clinical and societal burdens of lung cancer
A simple but sensitive liquid biopsy platform has the potential to make cancer screening more accessible to a wider variety of patients.1,2 This is one of the great unmet clinical needs for early detection diagnostics, particularly for lung cancer.3,4 Lung cancer is the single deadliest cancer regardless of gender or ethnicity. However, when caught in early stages, survival rates of lung cancer greatly improve.5 The current 5 year survival rate of lung cancer is about 22%, which is significantly lower than other leading cancers: colorectal cancer-65%; breast cancer-92%; and prostate cancer-98%.6,7 American Cancer Society also lists non-small cell lung cancer (NSCLC) as accounting for almost 85% of all lung cancers. Lung cancer is mostly asymptotic in its early stages, which generally means that when symptoms present and a low-dose CT scan (LDCT) is ordered, the quality adjusted life expectancy (QALE) is 3.6 years.8 Using the surveillance, epidemiology, and end results (SEER)-medicare database and drug costs, the societal burden of treating a stage IV patient is at least twice as much as a stage I lung cancer patient. Private insurance costs are extrapolated to mirror similar proportionality.9,10 From a purely healthcare economics perspective, this results in higher insurance premiums across the risk pool. But, more importantly, from a humanitarian perspective, the treatment options become dismal as the disease progresses, with most late-stage diagnoses resulting in a poor prognosis. sEV detection via liquid biopsy has the potential to transform the diagnostic and treatment paradigms for lung cancer by making lung cancer screening more accessible to unscreened high-risk populations.11,12
The current standard of care for screening lung cancer is the LDCT scan. However, 93% of the U.S. Preventive Services Task Force's recommended 15 million high-risk populations are not being screened, even before the COVID-19 pandemic paused routine screenings.13,14 The National Lung Screening Trial demonstrated a 20% decrease in mortality by using LDCT as a screening modality.14 However, less than 7% of the at-risk population actually receive the LDCT according to the United States Preventive Services Task Force and the American Lung Association. Simpler screening could overcome resource limitations, reduce the need for annual radiation exposure associated with even a LDCT (approximately 20× conventional X-ray), detect lung cancer earlier and improve patient outcomes.
With the well-known limitations of imaging technologies in cancer diagnostics, alternative non-invasive biomarkers are needed.15,16 Clinical partners have remarked that the problem is not the presence of nodules (which can be detected via a static snapshot with LDCT); the problem are the nodules which are increasing in size over time (which require more innovative detection technologies). Longitudinal monitoring via liquid biopsy complements imaging capabilities and can also track minimal residual disease.17,18 The LDCT is a complex protocol requiring extensive equipment capital expenditure, nurses, and trained radiologists to interpret the results. Going global, screening becomes even more impractical and inaccessible in undeveloped regions, with lung cancer still claiming almost 2 million lives every year, according to the World Health Organization. The situation calls for a lung cancer screening technique which increases screening compliance and ensures more accessible screening opportunities, particularly in low-resource settings. This technique has the potential to be deployed for population-screening. LDCT together with tissue biopsy may be ordered as part of traditional confirmation diagnostics when the signals from the tumors are revealed. Among the alternative cancer detection techniques, liquid biopsy, a minimally invasive cancer detection method based on bodily fluids, has the potential to fulfill some of the unmet needs.
A new class of liquid biopsies
Small extracellular vesicles (sEVs), are cell-released vesicles (30–150 nm in diameter) that are present in many bodily fluids. sEVs carry biochemical information representing their parental cells and simultaneously regulating the activities of recipient cells. Cancer cells are known to release significantly upregulated sEVs, making sEVs an ideal biomarker for liquid biopsies.19,20 The lipid membranes of sEVs protect their contents from enzymatic degradation while being trafficked throughout the body, thus preserving diagnostic specificity.21,22 sEVs have attracted attention in recent years specifically because of their potential in disease diagnosis and treatment monitoring, making them promising targets for liquid biopsy-based disease detections, including cancer.
Techniques for sEV isolation
sEV isolation protocols play an important role in vesicular studies to separate and purify the sEVs from other biological substances. Before biochemical characterization of the contents, sEVs first need to be isolated from biofluids in order to obtain purified vesicles. Currently, two types of isolation principles are primarily used for sEV isolation. One method isolates sEVs based on their physical properties such as size and density.23 Differential and density gradient ultracentrifugation are the predominant vesicle isolation techniques based on physical properties.24,25 Other techniques using this principle include size exclusion chromatography, ultrafiltration, and microfluidic isolations. There is a concentrated effort to improve the yield, purity and scalability of physical property-based sEV isolation.26,27 Another approach involves immune-based sEV isolation to separate by specific vesicle population surface markers.28 Immunocapture and immobilization harvest the target sEVs and irrelevant substances are removed by gentle washing.29 Popular sEV protein markers currently include CD9, CD81, and CD63, with new markers actively being developed.30,31
Techniques for sEV analysis
Once the sEV isolation process is complete, the next step is to characterize the sEVs for disease detection/diagnosis purposes. Nanoparticle tracking analysis (NTA) is used to render the size distributions of the isolated vesicle populations.32,33 Transmission electron microscopy (TEM) and/or scanning electron microscopy (SEM) are applied to show the vesicle morphologies, especially the cryo-TEM which clearly renders the sEVs' lipid membrane.34 After characterizing the vesicles' physical properties, biochemical analysis is necessary for examining the contents carried by the sEVs.
Western blot still holds as the gold standard for proteomic characterizations of sEVs. It provides the quantitative difference between different vesicle groups on specific target protein markers. As a bulk analysis technique, the major challenge for western blot comes from the population averaging that could lose distinguishing information among individual vesicles within a particular group.35,36
Genomic analysis is another popular aspect for sEV content studies. Conventional methods include polymerase chain reaction (PCR) and sequencing. PCR is common in genomic analysis of sEVs where primers that are paired to the target DNA are used to initiate the process and over 2 million copies of the target DNA can be achieved in 20 heat cycles.37 This provides genomic characterizations with high specificity. Sequencing is normally used for detecting different genomic targets especially in RNAs. Based on the initial concepts, the next generation sequencing (NGS) was developed to improve the capacity and reduce the time consumption in detections. Progress in genomic technologies is pushing the detection limit to a single cell or even single vesicle level. Collectively genomic techniques provide information about the specific DNA/RNA targets both qualitatively and quantitatively inside sEV populations. Similar to western blot, the requirement to lyse samples during genomic analyses imposes the risk of losing the small, but significant differences among individual vesicles, especially when the existence of sEV subpopulations has been widely recognized.38,39
Flow cytometry is another popular technique for sEV characterization because of two major benefits, single-vesicle sensitivity and relatively short processing time.40 Labeling of the vesicles is normally required before transferring the samples to the flow cytometer.41 Then the process will sort out the vesicle group based on the label/marker with high specificity. There is effort underway to push the detection limit below the particle sizes of 100–300 nm and improve the capability to multiplex.42,43
SERS for sEV analysis
Alternative technologies, including Raman spectroscopy, have demonstrated the potential to characterize sEVs based upon their biochemical composition, at the single vesicle level.44 Raman spectroscopy provides the structural “fingerprints” of the measured molecules through the interaction between the laser and the substances triggering Raman scattering.45 The “fingerprints” are reflected by the spectra collected for the corresponding substances measured. Traditionally, Raman spectroscopy is used in materials engineering to identify molecules and has been used for biological sensing in recent decades. One of the major challenges for Raman spectroscopy is its low probability of achieving the Raman scattering effect, about one in a million during the laser–molecule interactions.46 To overcome this challenge, surface-enhanced Raman spectroscopy (SERS) has been developed, triggering surface plasmon by nanometallic structures.47,48 A body of work has shown the potential of SERS to analyze sEVs for detecting cancers, including but not limited to breast, pancreatic, lung, and gastric with the sensitivity and the specificity both over 80% or even 90%.49–55 Our previously designed SERS substrate has demonstrated single-molecule sensitivity.56,57 The platform was utilized to illustrate the distinguishing p53+/+ and p53 −/− colon cancer cells, indicating its applicability for cancer detections.58 Further, we found that such sensitivity was essential to spectrally measure and separate the cell released sEVs signals sizing at the nanoscale. The platform has been successfully used for analyzing individual sEVs from different sources, demonstrating the distinguishability among different vesicle groups.59 With the quadruple dependence of SERS intensity on the local electromagnetic field intensity, a signal from a single ‘hotspot’ dominates. Our gold nanopyramid correlates the ‘hotspot’ sizes to the sEV sizes (∼80–150 nm), enabling the substrate to collect the SERS signal from individual vesicles one at a time.59,60 Since sEVs are highly complex and decorated by numerous proteins, a single-vesicle analytical technology like SERS is essential for proper characterization and cataloguing.
Scope of the study
In this study, we apply the sEVs collected from bronchoalveolar lavage (BAL) onto our substrate to explore the feasibility of NSCLC detection via SERS measurements of BAL sEVs. Published works have illustrated the feasibility of BAL derived sEVs for cargo/content-based analysis (e.g., protein and RNA/DNA expressions discrepancies) between the NSCLC patients and non-cancer control individuals.61–64 The referenced body of literature indicates that the BAL derived sEVs hold the potential as the source for NSCLC detection but little has been done from the single-vesicle perspective. Although expected to have low sEV concentration due to its nature of procuring,65,66 BAL is directly from the lungs that there is less likelihood of vesicles from other organs based on the current understanding of the sEV biogenesis.67–69 These purer, lung-derived sEVs allowed us to focus on precise sEV populations with less contamination. BAL used in this study is a bridge towards establishing a non-invasive liquid biopsy in that it elucidates the existence of EVs of diagnostic value, which are expected to find their ways to body fluids such as blood. This is despite of the fact that harvesting BAL is not completely noninvasive. Our assay successfully distinguishes NSCLC from controls after a machine learning (ML) algorithm processed the SERS spectral output. To elucidate the distinguishability between the cancer group and the non-cancer control group, both cross-validation and blind tests have been performed. The results further demonstrate the sensitivity of the SERS platform in detecting sEVs in a low vesicle concentration environment. As a pilot study, we show that the SERS spectral features collected from the sEVs in the bronchial washes hold the potential for NSCLC detection. Most importantly, the methods used in this study are feasible for analyzing sEVs in other biofluids (e.g., saliva, urine, or blood) for disease detection.
Materials & methods
Demographics of BAL sample donors
The participant ages ranged from 20 to 80 and included an equal ratio of males to females with 20% identifying as Hispanic. To complete the study in a timely manner, we kept smoking history as a variable and still obtained almost half smokers v. nonsmokers. We ensured the disease samples (n = 10) were representative of diagnoses across all NSCLC stages, from stage I to stage IV. The control set (n = 10) was not NSCLC diagnosed.
sEV isolation
Bronchoalveolar lavage fluid samples were stored in the freezer (−20 °C) upon arrival. The isolation process involved size-exclusion chromatography (SEC) using columns purchased from IZON Science. The samples were thawed at room temperature before isolation. A sterile syringe filter (Millex-GP Filter, 0.22 μm) was used to remove the remaining tissue or precipitates in the samples before loading samples into an IZON column (qEV10/35 nm) for sEV separation. Sample load volume was set up at 10 mL and phosphate-buffered saline (1× PBS) was used as the medium buffer in the equilibration, elution, and flush process. For detailed information, please refer to the vendor's manual. Isolated sEVs were stored in vials and kept in the −20 °C freezer for future use.
SERS substrate fabrication
SERS platforms were fabricated according to the method as we previously reported.56 First, a single layer of self-assembled polystyrene (PS) balls (∅ 500 nm) was generated on a surface of DI water using the Langmuir–Blodgett patterning. The layer was then transferred to a 4′′ (001) silicon dioxide wafer with a top oxide layer thickness of 50 nm. Next, a layer of 50 nm of Cr was deposited using electron beam deposition followed by the removal of PS balls using chloroform. The exposed SiO2 were etched using reactive ion etching to selectively expose Si. Next, the exposed silicon was etched using KOH. Inverted nanopyramids with sidewalls at 57.5-degree angles were created because of different etching rates along the [001] and [111] directions of silicon. The model was finished by removing the residual Cr and SiO2 using 48% HF solution. Then, 200 nm of gold film was deposited onto the pitted surface by electron beam deposition and bonded to a carrier wafer using epoxy before lifting off.
Raman measurement
After sEV isolation, 5 μL of each sEV sample solution was transferred onto the SERS substrate using a micropipette. A Reinshaw inVia Raman spectrometer was used for measurements in this study. All measurements were performed at room temperature. The laser excitation wavelength was 785 nm. The power used was 5 mW. Before usage, the system was first calibrated using the 520 cm−1 peak of silicon. The exposure time was 0.2 s to avoid sample overheating. For collecting SERS spectra from multiple sEVs, a Raman mapping measurement was performed over a 1.2 mm × 1.2 mm square with respect to the center of each sample droplet. The step width was 5 μm to avoid double collecting.
Scanning electron microscopy (SEM)
SEM used in this study was Nova 230. The acceleration voltage was 10 kilovolts. The working distance was ∼5.0 mm. The images were taken at the magnification between 45
000× and 55
000× (see Fig. 2). The electron detector used was TLD (through the lens detector) to obtain the signal from the secondary electrons.
SERS spectral analysis
On average, 30 different sEVs were obtained for each sample to produce spectra with 1129 Raman shifts in the range from 552 to 1681 cm−1 (biological information rich region). Preprocessing steps were applied to alleviate the spectral signature fluctuations caused by sample variations, SERS platform heterogeneity and instrument fluctuation. Particularly, fluorescence background subtraction and noise reduction were performed by batch processing based on asymmetric least square fitting and Savitzky–Golay filtering, followed by min–max normalization that proportionally compresses the original intensity range to [0, 1]. To reveal the spectral differences among the three cell line groups, standard linear discriminant analysis (LDA) was used to reduce the dimensionality for better visualization on a 2-dimensional plot. For machine learning model development, predictive model establishment by supervised learning or classification was the core for the proposed technology. It required appropriate complexity of the classifier to prevent both underfitting and overfitting for the purpose of generalizing the characteristic signature effectively. We used the conventional but powerful algorithm support vector machine (SVM) for classification tasks. Unsupervised learning or clustering analysis was performed by Hierarchical clustering analysis with customized distance metrics. It investigated the intrinsic similarities among the analytes SERS signature and serves as an auxiliary to classification. Repeated leave samples out cross validations were then applied to optimize the model settings, followed by blind tests for evaluating the clinical applicability.
Results and discussion
Experimental flow
The experimental flow of this study is shown in Fig. 1. We performed a twenty-participant UCLA Institutional Review Board (IRB) approved study that leveraged the availability of excess bronchoalveolar lavage (BAL) fluid in samples taken from patients suspected of lung cancer. sEVs were then isolated using size-exclusion chromatography (SEC). The isolated sEV solutions were transferred onto the SERS gold-nanopyramid substrate before measurements. We used 5 microliters per sample droplet per SERS measurement. SERS spectra from individual sEVs were then collected. On average, spectra from 30 individual sEVs were collected from each of the samples. Following confirmed clinical diagnosis, the SERS spectra from the collected sEVs were used to train a customized algorithm to recognize spectral “fingerprints” associated with patients diagnosed as having non-small cell lung cancer/adenocarcinoma (hereafter, NSCLC) or patients identified as not having NSCLC. An intermediate researcher then provided additional samples to the data scientist without revealing the clinical diagnosis for use as a test set.
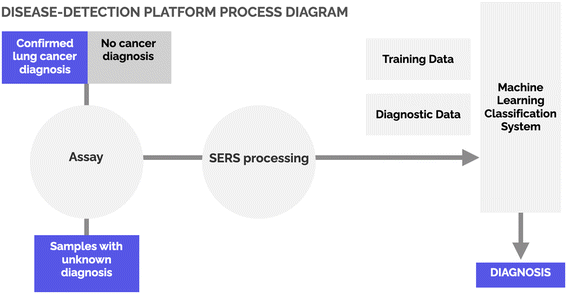 |
| Fig. 1 Experimental flow of single-vesicle SERS analysis of sEVs from BAL. | |
Samples overview
Fig. 2 exhibits an overview of the sEV samples and the SERS gold-nanopyramid substrate used in this study. The transmission electron microscope (TEM) image of the vesicle is shown in Fig. 2a. The image was taken in the cryo-EM mode which clearly resolved the lipid membrane of the vesicle. In addition, the image validated the vesicle sizes fell in the range of sEVs. The scanning electron microscope (SEM) images of the SERS substrate before and after sample introduction have been shown in the Fig. 2b and c respectively. Fig. 2b shows the gold nanopyramids array and Fig. 2c indicates that the vesicles lie in between individual nano-pyramids. The lower quality of resolution in Fig. 2c comparing to Fig. 2b could be attributed to the charging effect caused by the phosphate buffer solution (PBS) crystals lying on top of the substrate.
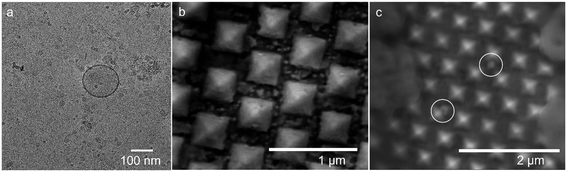 |
| Fig. 2 a) Cryo-EM image of sEVs; b) SEM image of the SERS gold nanopyramid substrate; c) SEM image of the SERS gold nanopyramid substrate after sample introduction. | |
Linear discriminant analysis (LDA) on SERS signatures
LDA was used to reveal the distinguishability between the spectra collected in the NSCLC patient group and the non-NSCLC control group. Such analysis renders the spectral distinguishability before projecting onto a 2D map for better visualization. A 2D rendering is necessary given the complexity of SERS output. Each SERS spectrum was treated as a point in the 1129-dimensional space corresponding to the 1129 wavenumbers of each SERS spectrum (1 sEV = 1129 vectors per spectrum). Next, these “points” were grouped into clusters based on their Euclidian distance from one another. Fig. 3a exhibits the LDA analysis plot on the SERS spectra collected from sEVs between the cancer and the control group. Each plotted dot represents a single SERS spectrum collected from a single sEV. The spectral differences were measured by the Euclidian distances that separated the dots. Cumulatively, the dots self-clustered according to their origin, indicating spectral distinguishability. Zooming in to the cancer cluster, we compared the spectra collected in NSCLC patients with early and late-stage cancer. The LDA plot shown in Fig. 3b also elucidates the spectral distinguishability between the early and late-stage patient groups. Collectively the LDA graphs illustrate the potential of using SERS spectra of individual sEVs derived from BAL for detecting NSCLC. Our results indicate the spectral distinguishability between both the control and cancer groups, and also between the early and late-stage NSCLC groups.
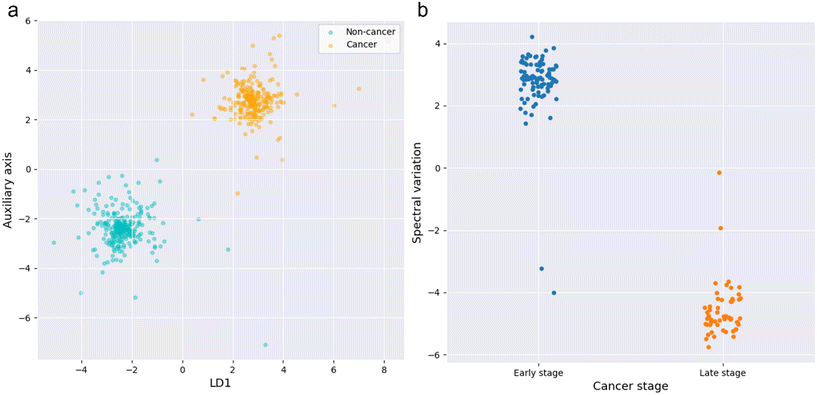 |
| Fig. 3 a) LDA analysis results of SERS spectra collected from sEVs between NSCLC patients and controls; b) between early stage and late stage NSCLC patients. | |
Model training and blind test using support vector machine (SVM)
To explore the clinical applicability of our SERS platform, a blind test was performed. SVM was used to provide the machine learning (ML) model training and testing. SERS spectra collected from 6 donors in each of the patient and the control group (total n = 12) were used as the training set and the SERS spectra collected from the rest of the 8 individuals (4 patients and 4 controls) were left out as the test set. Here the operator for SVM analysis of the SERS spectra was kept “blind” to the diagnosis results until testing concluded. Fig. 4 shows the schematics of model training and testing.
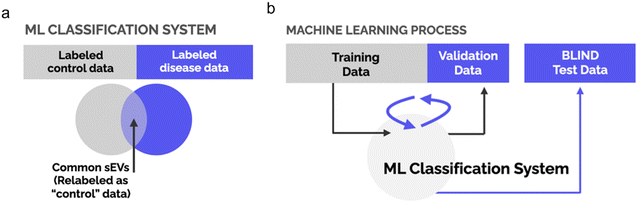 |
| Fig. 4 a) Schematic of SERS spectra relabeling; b) SVM model training and blind testing. | |
That cancer cells secrete cancer-cell specific sEVs leads to a natural conclusion that the anticipated composition of sEV populations isolated from biofluids will include disease specific sEVs. However, the disease population is known to be mixed with normal sEV populations in the biofluids. Given this co-existence of healthy and unhealthy sEV populations, ML training based on SERS spectra collected from individual sEVs would cause a mislabeling issue that impedes model accuracy. To overcome this in the study, we first relabeled the sEVs as shown in Fig. 4a. The relabeling process was through a spectral feature comparison between the spectra from NSCLC patients and the controls. The “common” spectra, indicating the existences of the normal sEVs, in the patient group are relabeled as control. We acknowledge that the limited control data size makes it challenging to parse all the normal spectra in the patient group. However, the principles behind this process help partially correct the mislabeling issue and significantly improve model accuracy.
Fig. 4b shows the ML schematic for model training and blind testing based on relabeled data. The classification model was built on the training set of 12 individuals, 6 from each group. Then, the model was applied to predict every donor in the test set whether the person was likely to be a NSCLC patient or a control. The predicted result was then compared with the actual clinical diagnosis. The SVM model correctly predicted all the diagnosis results of the 8 individuals in the test set based on SERS spectra collected from the BAL derived sEVs. As a proof-of-concept, our results suggest the clinical applicability of the SERS platform to analyze sEVs for NSCLC detection. This ML platform would benefit greatly from samples with a higher sEV count (the recent bronchoalveolar lavage study had an average sEV count of 30 with a median of 22) on the vesicle concentrations of the sample biofluids. Future studies involve improving the throughput on both sample sizes and sEV count per sample. Nonetheless, our study clearly illustrates the potential of using label-free single-vesicle SERS to detect and predict NSCLC based on sEV subpopulations. The methodology involved in this work has the potential for disease detection other than NSCLC with specific validations.
Conclusion
BAL-based liquid biopsy is invasive, but BAL has the distinct advantage of sEV purity with most sEVs likely secreted from lung cells as opposed to sEVs from other organs. The reason we studied BAL is to conclusively establish the biomarker value of lung-derived sEVs. This is the foundation for subsequent studies aimed at noninvasive biofluids such as blood, saliva, and urine. The fact that the platform is capable of detecting sEVs from BAL suggests a strong potential for handling bodily fluids with low vesicular concentrations considering the vesicle counts we obtained. We successfully demonstrated that anomalous sEVs are detectable using the platform's nanotechnology protocols with clinical samples from lung cancer patients, and not detectable in the control group. This BAL pilot study allowed us to intentionally stress-test the platform and still achieve positive results which bodes well for further studies using biofluids with known higher vesicle concentrations. The inherent non-destructive biochemical analysis of single vesicles via SERS makes this biosensing platform powerful when carefully combined with the appropriate ML algorithms.
Live subject
All experiments were performed in accordance with the safety and welfare guidelines of UCLA's Office of the Human Research Protection Program. Institutional Review Board approval was granted under IRB#21-000252. Informed consents were obtained from human participants of this study.
Author contributions
S. T. J., Z. L. & Y. H. X. designed the study. S. T. J., B. V. D., & J. A. S. filed the IRB study. S. O. led the BAL procurements. Z. L. & J. L. isolated sEVs from the BAL samples. Z. L. led the SERS measurements and data analysis. T. L. customized the machine learning algorithm for this study. J. L. helped with SERS substrate fabrications. S. T. J. composed the first draft of the manuscript. Z. L. & Y. H. X. led the manuscript revision with contributions from all other authors.
Conflicts of interest
Y. H. Xie and S. Oh are advisors to NurLabs.
Acknowledgements
This work was supported in part by the National Center for Advancing Translational Sciences at the National Institutes of Health under award numbers 4UH3TR002978-03 & 1U18TR003778-01 to Y. H. X. The authors thank Electron Imaging Center for Nanomachines at California NanoSystems Institute, particularly Dr. Wong Hoi Hui, for providing the cryo-TEM imaging.
References
- K. Pantel and C. Alix-Panabieres, Real-time liquid biopsy in cancer patients: fact or fiction?, Cancer Res., 2013, 73(21), 6384–6388 CrossRef CAS PubMed.
- G. Siravegna, S. Marsoni, S. Siena and A. Bardelli, Integrating liquid biopsies into the management of cancer, Nat. Rev. Clin. Oncol., 2017, 14(9), 531–548 CrossRef CAS.
- C. Rolfo, P. C. Mack, G. V. Scagliotti, P. Baas, F. Barlesi, T. G. Bivona, R. S. Herbst, T. S. Mok, N. Peled, R. Pirker, L. E. Raez, M. Reck, J. W. Riess, L. V. Sequist, F. A. Shepherd, L. M. Sholl, D. S. W. Tan, H. A. Wakelee, I. I. Wistuba, M. W. Wynes, D. P. Carbone, F. R. Hirsch and D. R. Gandara, Liquid Biopsy for Advanced Non-Small Cell Lung Cancer(NSCLC): A Statement Paper from the IASLC, J. Thorac. Oncol., 2018, 13(9), 1248–1268 CrossRef PubMed.
- D. Kwapisz, The first liquid biopsy test approved. Is it a new era of mutation testing for non-small cell lung cancer?, Ann. Transl. Med., 2017, 5(3), 46 CrossRef.
- H. Sung, J. Ferlay, R. L. Siegel, M. Laversanne, I. Soerjomataram, A. Jemal and F. Bray, Global Cancer Statistics 2020: GLOBOCAN Estimates of Incidence and Mortality Worldwide for 36 Cancers in 185 Countries, Ca-Cancer J. Clin., 2021, 71(3), 209–249 CrossRef.
- J. A. Barta, C. A. Powell and J. P. Wisnivesky, Global Epidemiology of Lung Cancer, Ann. Glob. Health, 2019, 85(1), 8 CrossRef PubMed.
- E. C. Ko, D. Raben and S. C. Formenti, The Integration of Radiotherapy with Immunotherapy for the Treatment of Non-Small Cell Lung Cancer, Clin. Cancer Res., 2018, 24(23), 5792–5806 CrossRef CAS PubMed.
- W. C. Black, Computed tomography screening for lung cancer in the National Lung Screening Trial: a cost-effectiveness analysis, J. Thorac. Imaging, 2015, 30(2), 79–87 CrossRef PubMed.
- A. Enstone, M. Greaney, M. Povsic, R. Wyn, J. R. Penrod and Y. Yuan, The Economic Burden of Small Cell Lung Cancer: A Systematic Review of the Literature, Pharmacoecon. Open, 2018, 2(2), 125–139 CrossRef PubMed.
- S. Andreas, C. Chouaid, S. Danson, O. Siakpere, L. Benjamin, R. Ehness, M. H. Dramard-Goasdoue, J. Barth, H. Hoffmann, V. Potter, F. Barlesi, C. Chirila, K. Hollis, C. Sweeney, M. Price, S. Wolowacz, J. A. Kaye and I. Kontoudis, Economic burden of resected(stage IB-IIIA) non-small cell lung cancer in France, Germany and the United Kingdom: A retrospective observational study(LuCaBIS), Lung Cancer, 2018, 124, 298–309 CrossRef PubMed.
- B. Zhou, K. Xu, X. Zheng, T. Chen, J. Wang, Y. Song, Y. Shao and S. Zheng, Application of exosomes as liquid biopsy in clinical diagnosis, Signal Transduction Targeted Ther., 2020, 5(1), 144 CrossRef CAS.
- M. Salehi and M. Sharifi, Exosomal miRNAs as novel cancer biomarkers: Challenges and opportunities, J. Cell. Physiol., 2018, 233(9), 6370–6380 CrossRef CAS.
- D. Ardila, A. P. Kiraly, S. Bharadwaj, B. Choi, J. J. Reicher, L. Peng, D. Tse, M. Etemadi, W. Ye, G. Corrado, D. P. Naidich and S. Shetty, End-to-end lung cancer screening with three-dimensional deep learning on low-dose chest computed tomography, Nat. Med., 2019, 25(6), 954–961 CrossRef CAS PubMed.
- L. T. Tanoue, N. T. Tanner, M. K. Gould and G. A. Silvestri, Lung cancer screening, Am. J. Respir. Crit. Care Med., 2015, 191(1), 19–33 CrossRef.
- L. Fass, Imaging and cancer: a review, Mol. Oncol., 2008, 2(2), 115–152 CrossRef PubMed.
- W. Liang, Y. Zhao, W. Huang, Y. Gao, W. Xu, J. Tao, M. Yang, L. Li, W. Ping, H. Shen, X. Fu, Z. Chen, P. W. Laird, X. Cai, J. B. Fan and J. He, Non-invasive diagnosis of early-stage lung cancer using high-throughput targeted DNA methylation sequencing of circulating tumor DNA(ctDNA), Theranostics, 2019, 9(7), 2056–2070 CrossRef CAS PubMed.
- I. Martins, I. P. Ribeiro, J. Jorge, A. C. Goncalves, A. B. Sarmento-Ribeiro, J. B. Melo and I. M. Carreira, Liquid Biopsies: Applications for Cancer Diagnosis and Monitoring, Genes, 2021, 12(3), 349 CrossRef CAS.
- X. Chen, J. Gole, A. Gore, Q. He, M. Lu, J. Min, Z. Yuan, X. Yang, Y. Jiang, T. Zhang, C. Suo, X. Li, L. Cheng, Z. Zhang, H. Niu, Z. Li, Z. Xie, H. Shi, X. Zhang, M. Fan, X. Wang, Y. Yang, J. Dang, C. McConnell, J. Zhang, J. Wang, S. Yu, W. Ye, Y. Gao, K. Zhang, R. Liu and L. Jin, Non-invasive early detection of cancer four years before conventional diagnosis using a blood test, Nat. Commun., 2020, 11(1), 3475 CrossRef CAS PubMed.
- S. D. Alipoor, E. Mortaz, M. Varahram, M. Movassaghi, A. D. Kraneveld, J. Garssen and I. M. Adcock, The Potential Biomarkers and Immunological Effects of Tumor-Derived Exosomes in Lung Cancer, Front. Immunol., 2018, 9, 819 CrossRef.
- V. S. LeBleu and R. Kalluri, Exosomes as a Multicomponent Biomarker Platform in Cancer, Trends Cancer, 2020, 6(9), 767–774 CrossRef CAS PubMed.
- D. K. Jeppesen, A. M. Fenix, J. L. Franklin, J. N. Higginbotham, Q. Zhang, L. J. Zimmerman, D. C. Liebler, J. Ping, Q. Liu, R. Evans, W. H. Fissell, J. G. Patton, L. H. Rome, D. T. Burnette and R. J. Coffey, Reassessment of Exosome Composition, Cell, 2019, 177(2), 428–445 e18 CrossRef CAS.
- J. Zhang, S. Li, L. Li, M. Li, C. Guo, J. Yao and S. Mi, Exosome and exosomal microRNA: trafficking, sorting, and function, Genomics, Proteomics Bioinf., 2015, 13(1), 17–24 CrossRef CAS.
- R. Xu, D. W. Greening, H. J. Zhu, N. Takahashi and R. J. Simpson, Extracellular vesicle isolation and characterization: toward clinical application, J. Clin. Invest., 2016, 126(4), 1152–1162 CrossRef PubMed.
- P. Duong, A. Chung, L. Bouchareychas and R. L. Raffai, Cushioned-Density Gradient Ultracentrifugation(C-DGUC) improves the isolation efficiency of extracellular vesicles, PLoS One, 2019, 14(4), e0215324 CrossRef CAS PubMed.
- C. N. Davis, H. Phillips, J. J. Tomes, M. T. Swain, T. J. Wilkinson, P. M. Brophy and R. M. Morphew, The importance of extracellular vesicle purification for downstream analysis: A comparison of differential centrifugation and size exclusion chromatography for helminth pathogens, PLoS Neglected Trop. Dis., 2019, 13(2), e0007191 CrossRef CAS.
- E. Willms, C. Cabanas, I. Mager, M. J. A. Wood and P. Vader, Extracellular Vesicle
Heterogeneity: Subpopulations, Isolation Techniques, and Diverse Functions in Cancer Progression, Front. Immunol., 2018, 9, 738 CrossRef.
- K. Sidhom, P. O. Obi and A. Saleem, A Review of Exosomal Isolation Methods: Is Size Exclusion Chromatography the Best Option?, Int. J. Mol. Sci., 2020, 21(18), 6466 CrossRef CAS.
- K. Singh, R. Nalabotala, K. M. Koo, S. Bose, R. Nayak and M. J. A. Shiddiky, Separation of distinct exosome subpopulations: isolation and characterization approaches and their associated challenges, Analyst, 2021, 146(12), 3731–3749 RSC.
- D. Yang, W. Zhang, H. Zhang, F. Zhang, L. Chen, L. Ma, L. M. Larcher, S. Chen, N. Liu, Q. Zhao, P. H. L. Tran, C. Chen, R. N. Veedu and T. Wang, Progress, opportunity, and perspective on exosome isolation - efforts for efficient exosome-based theranostics, Theranostics, 2020, 10(8), 3684–3707 CrossRef CAS.
- G. Li, W. Tang and F. Yang, Cancer Liquid Biopsy Using Integrated Microfluidic Exosome Analysis Platforms, Biotechnol. J., 2020, 15(5), e1900225 CrossRef PubMed.
- S. Lin, Z. Yu, D. Chen, Z. Wang, J. Miao, Q. Li, D. Zhang, J. Song and D. Cui, Progress in Microfluidics-Based Exosome Separation and Detection Technologies for Diagnostic Applications, Small, 2020, 16(9), e1903916 CrossRef PubMed.
- M. Kesimer and R. Gupta, Physical characterization and profiling of airway epithelial derived exosomes using light scattering, Methods, 2015, 87, 59–63 CrossRef CAS.
- V. Sokolova, A. K. Ludwig, S. Hornung, O. Rotan, P. A. Horn, M. Epple and B. Giebel, Characterisation of exosomes derived from human cells by nanoparticle tracking analysis and scanning electron microscopy, Colloids Surf., B, 2011, 87(1), 146–150 CrossRef CAS PubMed.
- E. van der Pol, F. Coumans, Z. Varga, M. Krumrey and R. Nieuwland, Innovation in detection of microparticles and exosomes, J. Thromb. Haemostasis, 2013, 11(Suppl 1), 36–45 CrossRef PubMed.
- T. J. McDonald, M. H. Perry, R. W. Peake, N. J. Pullan, J. O'Connor, B. M. Shields, B. A. Knight and A. T. Hattersley, EDTA improves stability of whole blood C-peptide and insulin to over 24 hours at room temperature, PLoS One, 2012, 7(7), e42084 CrossRef CAS PubMed.
- F. Raimondo, L. Morosi, C. Chinello, F. Magni and M. Pitto, Advances in membranous vesicle and exosome proteomics improving biological understanding and biomarker discovery, Proteomics, 2011, 11(4), 709–720 CrossRef CAS.
- D. Buschmann, B. Kirchner, S. Hermann, M. Marte, C. Wurmser, F. Brandes, S. Kotschote, M. Bonin, O. K. Steinlein, M. W. Pfaffl, G. Schelling and M. Reithmair, Evaluation of serum extracellular vesicle isolation methods for profiling miRNAs by next-generation sequencing, J. Extracell. Vesicles, 2018, 7(1), 1481321 CrossRef PubMed.
- F. Li, J. M. Yoshizawa, K. M. Kim, J. Kanjanapangka, T. R. Grogan, X. Wang, D. E. Elashoff, S. Ishikawa, D. Chia, W. Liao, D. Akin, X. Yan, M. S. Lee, R. Choi, S. M. Kim, S. Y. Kang, J. M. Bae, T. S. Sohn, J. H. Lee, M. G. Choi, B. H. Min, J. H. Lee, J. J. Kim, Y. Kim, S. Kim and D. T. W. Wong, Discovery and Validation of Salivary Extracellular RNA Biomarkers for Noninvasive Detection of Gastric Cancer, Clin. Chem., 2018, 64(10), 1513–1521 CrossRef CAS PubMed.
- J. Ko, E. Carpenter and D. Issadore, Detection and isolation of circulating exosomes and microvesicles for cancer monitoring and diagnostics using micro−/nano-based devices, Analyst, 2016, 141(2), 450–460 RSC.
- L. A. Andronico, Y. Jiang, S. R. Jung, B. S. Fujimoto, L. Vojtech and D. T. Chiu, Sizing Extracellular Vesicles Using Membrane Dyes and a Single Molecule-Sensitive Flow Analyzer, Anal. Chem., 2021, 93(14), 5897–5905 CrossRef CAS PubMed.
- A. Morales-Kastresana, B. Telford, T. A. Musich, K. McKinnon, C. Clayborne, Z. Braig, A. Rosner, T. Demberg, D. C. Watson, T. S. Karpova, G. J. Freeman, R. H. DeKruyff, G. N. Pavlakis, M. Terabe, M. Robert-Guroff, J. A. Berzofsky and J. C. Jones, Labeling Extracellular Vesicles for Nanoscale Flow Cytometry, Sci. Rep., 2017, 7(1), 1878 CrossRef.
- T. K. Kurian, S. Banik, D. Gopal, S. Chakrabarti and N. Mazumder, Elucidating Methods for Isolation and Quantification of Exosomes: A Review, Mol. Biotechnol., 2021, 63(4), 249–266 CrossRef CAS PubMed.
- M. N. Theodoraki, C. S. Hong, V. S. Donnenberg, A. D. Donnenberg and T. L. Whiteside, Evaluation of Exosome Proteins by on-Bead Flow Cytometry, Cytometry, Part A, 2021, 99(4), 372–381 CrossRef CAS PubMed.
- E. V. Efremov, F. Ariese and C. Gooijer, Achievements in resonance Raman spectroscopy review of a technique with a distinct analytical chemistry potential, Anal. Chim. Acta, 2008, 606(2), 119–134 CrossRef CAS PubMed.
- A. Rygula, K. Majzner, K. M. Marzec, A. Kaczor, M. Pilarczyk and M. Baranska, Raman spectroscopy of proteins: a review, J. Raman Spectrosc., 2013, 44(8), 1061–1076 CrossRef CAS.
- X. Wang, S.-C. Huang, S. Hu, S. Yan and B. Ren, Fundamental understanding and applications of plasmon-enhanced Raman spectroscopy, Nat. Rev. Phys., 2020, 2(5), 253–271 CrossRef.
- P. L. Stiles, J. A. Dieringer, N. C. Shah and R. P. Van Duyne, Surface-enhanced Raman spectroscopy, Annu. Rev. Anal. Chem., 2008, 1, 601–626 CrossRef CAS PubMed.
- X. X. Han, R. S. Rodriguez, C. L. Haynes, Y. Ozaki and B. Zhao, Surface-enhanced Raman spectroscopy, Nat. Rev. Methods Primers, 2022, 1(1), 1–17 Search PubMed.
- J. U. Lee, W. H. Kim, H. S. Lee, K. H. Park and S. J. Sim, Quantitative and Specific Detection of Exosomal miRNAs for Accurate Diagnosis of Breast Cancer Using a Surface-Enhanced Raman Scattering Sensor Based on Plasmonic Head-Flocked Gold Nanopillars, Small, 2019, 15(17), e1804968 CrossRef.
- Y. F. Tian, C. F. Ning, F. He, B. C. Yin and B. C. Ye, Highly sensitive detection of exosomes by SERS using gold nanostar@Raman reporter@nanoshell structures modified with a bivalent cholesterol-labeled DNA anchor, Analyst, 2018, 143(20), 4915–4922 RSC.
- Y. Pang, J. Shi, X. Yang, C. Wang, Z. Sun and R. Xiao, Personalized detection of circling exosomal PD-L1 based on Fe3O4@TiO2 isolation and SERS immunoassay, Biosens. Bioelectron., 2020, 148, 111800 CrossRef CAS PubMed.
- T. D. Li, R. Zhang, H. Chen, Z. P. Huang, X. Ye, H. Wang, A. M. Deng and J. L. Kong, An ultrasensitive polydopamine bi-functionalized SERS immunoassay for exosome-based diagnosis and classification of pancreatic cancer, Chem. Sci., 2018, 9(24), 5372–5382 RSC.
- E. A. Kwizera, R. O'Connor, V. Vinduska, M. Williams, E. R. Butch, S. E. Snyder, X. Chen and X. Huang, Molecular Detection and Analysis of Exosomes Using Surface-Enhanced Raman Scattering Gold Nanorods and a Miniaturized Device, Theranostics, 2018, 8(10), 2722–2738 CrossRef CAS.
- Z. Wang, S. Zong, Y. Wang, N. Li, L. Li, J. Lu, Z. Wang, B. Chen and Y. Cui, Screening and multiple detection of cancer exosomes using an SERS-based method, Nanoscale, 2018, 10(19), 9053–9062 RSC.
- S. Zong, L. Wang, C. Chen, J. Lu, D. Zhu, Y. Zhang, Z. Wang and Y. Cui, Facile detection of tumor-derived exosomes using magnetic nanobeads and SERS nanoprobes, Anal. Methods, 2016, 8(25), 5001–5008 RSC.
- P. Wang, O. Liang, W. Zhang, T. Schroeder and Y. H. Xie, Ultra-sensitive graphene-plasmonic hybrid platform for label-free detection, Adv. Mater., 2013, 25(35), 4918–4924 CrossRef CAS PubMed.
- P. Wang, M. Xia, O. Liang, K. Sun, A. F. Cipriano, T. Schroeder, H. Liu and Y. H. Xie, Label-Free SERS Selective Detection of Dopamine and Serotonin Using Graphene-Au Nanopyramid Heterostructure, Anal. Chem., 2015, 87(20), 10255–10261 CrossRef CAS PubMed.
- O. Liang, P. Wang, M. Xia, C. Augello, F. Yang, G. Niu, H. Liu and Y. H. Xie, Label-free distinction between p53+/+ and p53 −/− colon cancer cells using a graphene based SERS platform, Biosens. Bioelectron., 2018, 118, 108–114 CrossRef CAS PubMed.
- Z. Yan, S. Dutta, Z. Liu, X. Yu, N. Mesgarzadeh, F. Ji, G. Bitan and Y. H. Xie, A Label-Free Platform for Identification of Exosomes from Different Sources, ACS Sens., 2019, 4(2), 488–497 CrossRef CAS.
- M. Moskovits, Surface-enhanced spectroscopy, Rev. Mod. Phys., 1985, 57(3), 783–826 CrossRef CAS.
- Y. Zhang, Z. Liu, S. Li, M. Wang, D. Dai, H. Jing and L. Liu, Upregulation of E-cadherin in bronchoalveolar lavage fluid-derived exosomes in patients with lung cancer, Thorac. Cancer, 2020, 11(1), 41–47 CrossRef CAS PubMed.
- Y. Yang, P. Ji, X. Wang, H. Zhou, J. Wu, W. Quan, A. Shang, J. Sun, C. Gu, J. Firrman, W. Xiao, Z. Sun and D. Li, Bronchoalveolar Lavage Fluid-Derived Exosomes: A Novel Role Contributing to Lung Cancer Growth, Front. Oncol., 2019, 9, 197 CrossRef.
- M. Rodriguez, J. Silva, A. Lopez-Alfonso, M. B. Lopez-Muniz, C. Pena, G. Dominguez, J. M. Garcia, A. Lopez-Gonzalez, M. Mendez, M. Provencio, V. Garcia and F. Bonilla, Different exosome cargo from plasma/bronchoalveolar lavage in non-small-cell lung cancer, Genes, Chromosomes Cancer, 2014, 53(9), 713–724 CAS.
- J. E. Kim, J. S. Eom, W. Y. Kim, E. J. Jo, J. Mok, K. Lee, K. U. Kim, H. K. Park, M. K. Lee and M. H. Kim, Diagnostic value of microRNAs derived from exosomes in bronchoalveolar lavage fluid of early-stage lung adenocarcinoma: A pilot study, Thorac. Cancer, 2018, 9(8), 911–915 CrossRef CAS PubMed.
- A. S. Carvalho, M. C. S. Moraes, C. Hyun Na, I. Fierro-Monti, A. Henriques, S. Zahedi, C. Bodo, E. M. Tranfield, A. L. Sousa, A. Farinho, L. V. Rodrigues, P. Pinto, C. Barbara, L. Mota, T. T. Abreu, J. Semedo, S. Seixas, P. Kumar, B. Costa-Silva, A. Pandey and R. Matthiesen, Is the Proteome of Bronchoalveolar Lavage Extracellular Vesicles a Marker of Advanced Lung Cancer?, Cancers, 2020, 12(11), 3450 CrossRef CAS PubMed.
- K. B. Johnsen, J. M. Gudbergsson, T. L. Andresen and J. B. Simonsen, What is the blood concentration of extracellular vesicles? Implications for the use of extracellular vesicles as blood-borne biomarkers of cancer, Biochim. Biophys. Acta, Rev. Cancer, 2019, 1871(1), 109–116 CrossRef CAS PubMed.
- A. A. Farooqi, N. N. Desai, M. Z. Qureshi, D. R. N. Librelotto, M. L. Gasparri, A. Bishayee, S. M. Nabavi, V. Curti and M. Daglia, Exosome biogenesis, bioactivities and functions as new delivery systems of natural compounds, Biotechnol. Adv., 2018, 36(1), 328–334 CrossRef CAS PubMed.
- N. P. Hessvik and A. Llorente, Current knowledge on exosome biogenesis and release, Cell. Mol. Life Sci., 2018, 75(2), 193–208 CrossRef CAS PubMed.
- Y. Zhang, Y. Liu, H. Liu and W. H. Tang, Exosomes: biogenesis, biologic function and clinical potential, Cell Biosci., 2019, 9, 19 CrossRef PubMed.
Footnote |
† Equal contribution. |
|
This journal is © The Royal Society of Chemistry 2023 |
Click here to see how this site uses Cookies. View our privacy policy here.