DOI:
10.1039/D2CS00761D
(Review Article)
Chem. Soc. Rev., 2024,
53, 2530-2577
Porous materials as effective chemiresistive gas sensors
Received
19th September 2023
First published on 1st February 2024
Abstract
Chemiresistive gas sensors (CGSs) have revolutionized the field of gas sensing by providing a low-power, low-cost, and highly sensitive means of detecting harmful gases. This technology works by measuring changes in the conductivity of materials when they interact with a testing gas. While semiconducting metal oxides and two-dimensional (2D) materials have been used for CGSs, they suffer from poor selectivity to specific analytes in the presence of interfering gases and require high operating temperatures, resulting in high signal-to-noise ratios. However, nanoporous materials have emerged as a promising alternative for CGSs due to their high specific surface area, unsaturated metal actives, and density of three-dimensional inter-connected conductive and pendant functional groups. Porous materials have demonstrated excellent response and recovery times, remarkable selectivity, and the ability to detect gases at extremely low concentrations. Herein, our central emphasis is on all aspects of CGSs, with a primary focus on the use of porous materials. Further, we discuss the basic sensing mechanisms and parameters, different types of popular sensing materials, and the critical explanations of various mechanisms involved throughout the sensing process. We have provided examples of remarkable performance demonstrated by sensors using these materials. In addition to this, we compare the performance of porous materials with traditional metal-oxide semiconductors (MOSs) and 2D materials. Finally, we discussed future aspects, shortcomings, and scope for improvement in sensing performance, including the use of metal–organic frameworks (MOFs), covalent-organic frameworks (COFs), and porous organic polymers (POPs), as well as their hybrid counterparts. Overall, CGSs using porous materials have the potential to address a wide range of applications, including monitoring water quality, detecting harmful chemicals, improving surveillance, preventing natural disasters, and improving healthcare.
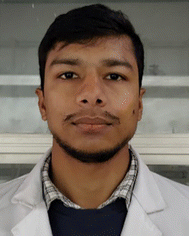
Akashdeep Sharma
| Akashdeep Sharma, a doctoral candidate in the Hybrid Porous Materials Laboratory at the Department of Chemistry of the Indian Institute of Technology, Jammu (India) under Prof. Kolleboyina Jayaramulu. He obtained his master's degree from the University of Jammu specialized in organic chemistry. His current research focuses on developing organic porous materials for sensor based applications. |
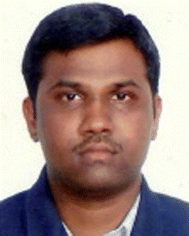
Sunil Babu Eadi
| Dr Sunil Babu Eadi holds a BS degree in Chemistry from Andhra University, India, and MS degree in Chemistry from the University of Hyderabad, India. He earned his PhD degree in Advanced Materials Engineering from Chungnam National University in 2015. Following his doctoral studies, Dr Eadi served as a postdoctoral researcher at the Kumoh National Institute of Technology in Gumi, Korea, from 2015 to 2018. Subsequently, from 2018 to 2023, he held the position of Research Professor at Chungnam National University, Republic of Korea. Currently, a Scientific Officer at the Indian Institute of Technology Jodhpur, his research focuses on chemical sensor development, Contact Resistance Reduction Technology and Silicide Technology. |
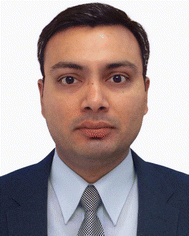
Hemanth Noothalapati
| Dr Hemanth Noothalapati, an Assistant Professor at Faculty of Life and Environmental Sciences, Shimane University, Japan, earned his PhD in Applied Chemistry from National Chiao Tung University (NCTU), Taiwan, through a prestigious Taiwan Scholarship in 2013. Following a postdoctoral fellowship at NCTU's Ultimate Spectroscopy and Imaging laboratory, he started his independent research at Shimane University. Specializing in label-free molecular spectroscopy and imaging, Dr Noothalapati employs chemometrics, machine learning and artificial intelligence to explore diverse applications in biology, medicine, materials, and the environment. His innovative approach contributes to advancing scientific understanding and technological applications in these fields. |
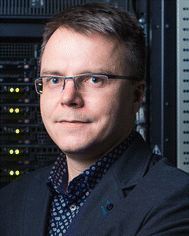
Michal Otyepka
| Michal Otyepka is Head of CATRIN-RCPTM, a research division at Palacký University in Olomouc. He is a member of the Scientific Board of the Czech Grant Agency and the LUMI Supercomputer (Finland). His research interests cover physical–chemical properties and reactivity of graphene derivatives and 2D materials, noncovalent interactions to 2D materials, and photoluminescence properties of carbon dots (CDs). He has been developing the chemistry of fluorographene (2D chemistry) toward graphene derivatives, which can be applied in (bio)sensing, catalysis, and energy storage. He specializes also in modeling of biomolecules, nanomaterials, and complex molecular systems and the development of force fields, multiscale methods, and their applications. |
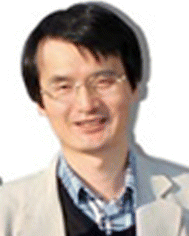
Hi-Deok Lee
| Prof. Hi-Deok LEE, with BS, MS, and PhD degrees in electrical engineering from the Korea Advanced Institute of Science and Technology (1990, 1992, 1996), joined LG Semicon Company Ltd. in 1993, contributing to CMOS technology development. Since 2001, he is a Professor at Chungnam National University, specializing in nanoscale CMOS technology, reliability physics, and sensor enhancement. A recipient of the Excellent Professor Award (2001, 2003, 2014), Prof. LEE was a Visiting Scholar at the University of Texas at Austin (2006–2008). A member of the Institute of Electronics, he currently heads the Intelligent ICT Education & Research Program for Future Defense Technology. His research focuses on nanoscale CMOS technology, reliability physics, silicide technology, test element group design, and sensor development. |
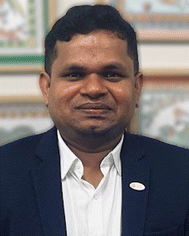
Kolleboyina Jayaramulu
| Kolleboyina Jayaramulu (Ram) is an Assistant Professor in the Department of Chemistry Indian Institute of Technology Jammu, India. He earned a PhD in Materials Chemistry at Jawaharlal Nehru Centre for Advanced Scientific Research, Bangalore, India. His scholarly pursuits have been further enriched by international experiences, having been honored with an Alexander von Humboldt Postdoctoral Fellowship in Germany, an ICMS Postdoctoral Fellowship and Sakura Science Exchange Program Japan. Ram is indeed a distinguished member of the prestigious Indian National Young Academy of Sciences (INYAS) (2023–2027). His research expertise is in the design and development of the structure–property relationship of hybrid (2D) porous materials for industrially relevant conditions. |
1. Introduction
The detrimental impact of growing air pollution on our planet and human well-being is undeniable. The rapid surge in industrialization and globalization has led to the widespread release of harmful gases and volatile compounds into our environment and homes. These emissions have far-reaching consequences, profoundly affecting both human health and the delicate ecological balance of Earth.1 According to the United Nations Environmental Agency, approximately 7 million premature deaths every year are due to air pollution. The deadliest illnesses linked to PM 2.5 air pollution are stroke, heart disease, lung disease, lower respiratory diseases (such as pneumonia), and cancer. High levels of fine particles also contribute to other illnesses, like diabetes, can hinder cognitive development in children and also cause mental health problems.2 Furthermore, as emphasized by the United Nations, air pollution has exacted a devastating toll on the biodiversity of our planet's plant and animal ispecies. The presence of sulfur and nitrogen oxides in our atmosphere has given rise to acid rain and smog, causing extensive harm to plant life and marine ecosystems. To combat these pressing problems of air pollution, innovative solutions are urgently needed. One promising approach involves the use of chemical sensors capable of detecting and alarming us to the presence of harmful pollutants. These sensors can serve as early warning systems, allowing us to take proactive measures to reduce pollution and protect our health and the environment. Chemiresistive gas sensors (CGSs) have emerged as a promising technology for the accurate and simple detection of harmful gases and volatile organic compounds (VOCs) in various applications. These sensors are based on the principle that certain gases and VOCs can induce changes in the electrical resistance of a sensing material.3–5 This property allows CGSs to detect and quantify the presence of specific substances, providing valuable information for assessing air quality, ensuring safety, and monitoring environmental conditions.
CGSs have found applications in diverse fields. Advances in nanotechnology, materials science, and fabrication techniques continue to expand the range of sensing materials and improve the overall functionality of these sensors. CGSs play a vital role in environmental monitoring, particularly in detecting and quantifying pollutants. For example, in urban areas with heavy traffic, these sensors are deployed in air quality monitoring stations to measure concentrations of gases like carbon monoxide (CO), nitrogen dioxide (NO2), and volatile organic compounds (VOCs). Such sensors provide real-time data, allowing authorities to assess air quality and implement measures to mitigate the adverse effects of pollution, such as the reduction of CO emissions from vehicles.4,6,7 In healthcare, they offer the potential for non-invasive disease diagnosis by detecting specific biomarkers in exhaled breath. They enable the detection of biomarkers associated with various diseases. For instance, in diabetes management, sensors can detect glucose levels in blood or interstitial fluid, providing crucial information for insulin dosing. Additionally, breath analysis using CGSs has shown promise for non-invasive disease diagnosis and monitoring. A prime example is the detection of acetone in the breath of individuals with diabetes as an indicator of their blood glucose levels.8–10 They also play a crucial role in industrial safety, where the detection of hazardous gases is vital to protect workers and prevent accidents. CGSs are indispensable in industrial settings for process control and safety. They monitor and regulate parameters like gas concentrations, humidity, and solvent levels, ensuring optimal operating conditions. These sensors are vital for leak detection as well, preventing potentially hazardous situations in industries dealing with volatile substances. For example, in the chemical industry, these sensors can detect leaks of toxic or flammable gases, allowing for immediate response and containment.11,12 The food industry relies on CGSs to assess food quality and safety. They play a crucial role in monitoring food freshness and preventing waste. For example, these sensors can detect spoilage gases, such as ammonia and ethylene, emitted by fruits, and vegetables, enabling timely interventions to maintain food quality. Additionally, CGSs are used in food packaging to ensure integrity, prevent contamination and ensure product safety.12–14 CGSs are a crucial component of chemical and biological warfare agent detection systems. They can identify toxic substances and provide early warning in defence and security applications. For instance, in military applications, these sensors are used to detect chemical agents and protect personnel from exposure to harmful substances. Additionally, they are employed in explosives detection, enhancing security measures at airports and public spaces by detecting trace amounts of explosive materials.15–17
Recent advancements have expanded the utility of CGSs to emerging applications. These include the detection of volatile organic compounds (VOCs) in indoor air quality monitoring. For example, in smart buildings, these sensors can detect VOCs released from building materials or cleaning products, ensuring healthy indoor air quality. Wearable devices incorporating CGSs have been developed for personal health monitoring, measuring parameters like sweat electrolyte levels. In the food and perfume industries, electronic noses equipped with these sensors are used for flavour and fragrance analysis, ensuring product consistency and quality. Efforts are ongoing to enhance the performance of CGSs by improving their selectivity, sensitivity, and response time. In the medical field, CGSs are extensively used for diagnostic purposes. Fig. 1 shows the different types of gas detection techniques in general use.
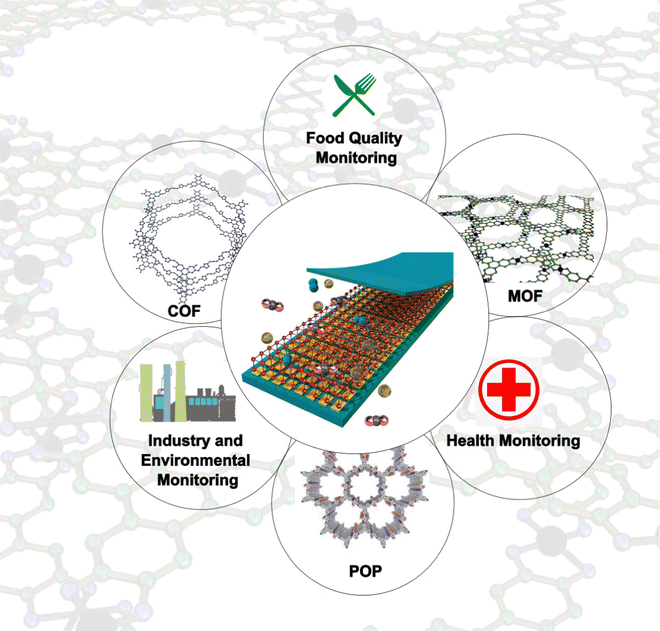 |
| Fig. 1 The schematic illustration portrays a range of advanced porous materials such as MOFs, COFs, and POPs, designed for applications in chemiresistive gas sensors across the industrial, environmental, healthcare, and food quality monitoring sectors. | |
The significance of CGSs lies in their potential to address the drawbacks associated with traditional detection technologies, While electrochemical, colorimetric, luminescent, sol–gel, infrared (IR), and paramagnetic IR optical sensors are effective, they often suffer from complexities such as high costs, limited scalability, and energy consumption issues. In contrast, CGSs offer advantages such as simplicity, cost-effectiveness, compactness, and low power requirements, making them attractive candidates for widespread adoption. These sensors can be designed using a variety of sensing materials, including metal oxides, polymers, nanomaterials, and carbon-based materials like graphene and carbon nanotubes. The sensing material's surface interacts with the target gases or VOCs, causing changes in its electrical conductivity or resistance. These changes are then translated into measurable signals that can be analyzed to identify and quantify the concentration of the target substances. Traditional chemiresistive materials and porous materials for gas sensing have distinct characteristics and advantages, and comparing them can help us understand the differences and potential benefits of using porous materials in gas sensing applications.
Traditional chemiresistive gas sensors are often based on metal oxides e.g., SnO2, ZnO, WO3etc. These materials change their electrical resistance in the presence of specific gases due to chemical reactions on their surface. The rapid expansion of metal-oxide-based sensors can be traced back to the pioneering work of Seyama et al., employing a ZnO thin film as the sensing layer, successfully showcasing the feasibility of gas sensing through uncomplicated electrical devices.18 Since then, there have been tremendous reports on the applications of semiconducting metal oxides as gas sensors such as TiO2, SnO2, WO3, V2O5, Fe2O3, NiO, CeO2, CuO, In2O3, Nb2O5, etc. Tin dioxide (SnO2) sensors are widely used for detecting gases like methane and carbon monoxide.19,20 SMO gas sensor devices have several unique advantages such as low cost, small size, measurement simplicity, durability, ease of fabrication, and low detection limits (<ppm levels). In addition, most SMO-based sensors tend to be long-lived and somewhat resistant to poisoning. For these reasons, they have rapidly grown in popularity, becoming the most widely used gas sensors available these days using various conducting polymers, carbonaceous materials and various metal/metal oxide nanoparticles. However, the selectivity of specific analytes such as acetone, methanol, ethanol, isoprene etc., by various metal oxide materials is still a big problem.
However, porous materials possess certain requisite features that make them potential materials for gas sensing. In order to get detected by the sensing device, adsorption and desorption of gas molecules is the primary requirement.21–30 Porous materials being custodians of exceptional surface area and bearers of appropriate interactive functional sites fulfil the need of host–analyte interaction. CGSs feature the transduction of chemical interactions into electrical outputs such as conductance or resistance in low-cost, high performance, less-energy consumption and portable devices. In order to bring chemiresistivity in the sensing materials, conduction of charges in the material is the foremost requirement. Porous materials provide vast options for selecting such appropriate materials owing to their extended frameworks that work as conduction highways for the movement of charges.
Porous materials are an interesting class of materials that have developed a great career in terms of storage, molecular level seiving, catalysis, water treatment, sensing and so on. The use of modern crystalline porous materials such as MOFs, COFs and POPs was well-established as a sensing platform primarily due to their porous nature.21,23,31–49 An unavoidable feature for an efficient chemiresistive gas sensing material is the electrical response towards the analyte gas which not all porous materials usually offer. Thus, this section will deal with only those materials meticulously which are usually conductive in nature or which show an electrical response towards the target gases based on their band-gap energies.
Metal organic frameworks (MOFs) provide exceptionally high surface area, rigid and ordered framework, and functional group versatility that provide optimal host–analyte interaction and high selectivity. MOFs can be 2D or 3D based on the geometry of the organic linker being used.29,50–57 Although 3D MOFs provide facile host–guest interactions, their non-conductive nature limits their use in chemiresistive sensing. Conversely, 2D MOFs serve as highly conductive materials owing to the planar and conjugated organic monomeric units such as porphyrin, triphenylene, phthalocyanine, etc. and thus offer an excellent option as a chemiresistive gas sensing material.58 COFs can provide highly tunable structures and vast functionalization opportunities which remain beneficial for efficient guest–host interactions. Although their electrical conductivities are not much higher, their crystalline nature enables them to be excellent chemiresistive sensing materials even with their minute conductivities, though metal and conductive carbon doping based conductivity increments were reported previously. Graphene-based materials are conductive in nature and thus their functionalized counterparts are realized to show chemiresistive nature for gas sensing purposes.
Graphene has extensively gone through various modifications and hybridizations to produce materials such as graphene oxide (GO), reduced graphene oxide (rGO), metal/metal oxide decorated graphene nanocomposites, Graphene–polymer composites and so on.59 These modifications have been used for facilitating host–analyte interactions that are beneficial for sensing various analytes including gases. In this section, MOFs and COFs will be focused extensively as porous materials for chemiresistive gas sensing, while other prominent porous materials that have created milestones in this field will also be discussed. The remarkable expansion of chemiresistive gas sensing using advanced porous materials is clearly evident, as reflected in the steadily rising number of publications from 2014 to 2023 (Fig. 2a and b). However, a diverse array of porous materials have found application in chemiresistive gas sensors, with key milestones highlighted in Fig. 2c. In summary, the choice between traditional chemiresistive materials and porous materials for gas sensing depends on the specific requirements of the application. Traditional materials are often preferred for their rapid response, robustness, and cost-effectiveness, while porous materials are gaining attention for their exceptional sensitivity and selectivity, especially in applications where precise gas detection is critical. Researchers continue to explore ways to harness the advantages of both types of materials for enhanced gas sensing capabilities.
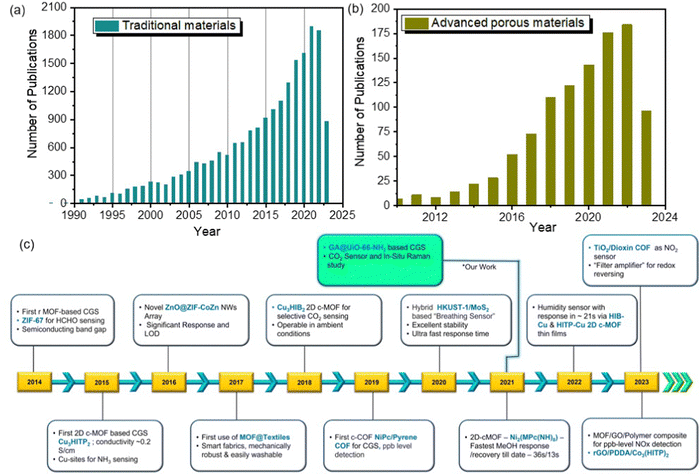 |
| Fig. 2 Publication history of chemiresistive gas sensing (a) traditional material (b) advanced porous MOFs, COFs and POPs over the years. Data are obtained from the web of science by searching the keywords “chemiresistive gas sensing” and “porous material” (up to September 15, 2023). The number of publications on chemiresistive gas sensing materials using traditional materials has almost doubled every year since 2000, and a similar trend has been followed by advanced porous materials since 2014. Data are obtained (c) timeline chart showing the major developments of and progress in advanced porous materials towards chemiresistive gas sensing in terms of synthesis, design pristine, hybrids and derivatives of MOFs, COFs and POPs for various gas sensing applications. | |
2. Definition and operating principle of chemiresistive sensors
CGSs are devices that detect and quantify the presence of gases and volatile organic compounds (VOCs) based on changes in electrical resistance. The operating principle of CGSs relies on the interaction between a sensing material and the target analyte. In general, a gas sensor comprises two main elements: a receptor and a transducer, as depicted in Fig. 3 The sensing material (receptor) used in CGSs is carefully chosen to exhibit a change in its electrical conductivity or resistance (transducer) when exposed to specific gases or VOCs (analytes).
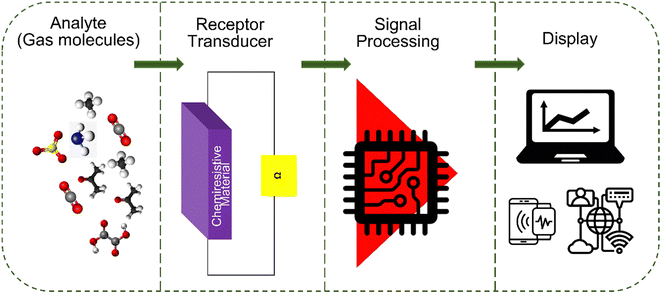 |
| Fig. 3 Schematic illustration depicts gas sensor parts and typical measurement characteristics of chemiresistive sensors. | |
This material can be a metal oxide, a conducting polymer, a nanomaterial, or a combination of these. The surface of the sensing material is designed to have a high surface-to-volume ratio to maximize the interaction with the target analyte. When the target gas or VOC molecules come into contact with the sensing material's surface, they adsorb onto it, causing a change in the electrical conductivity or resistance of the material. This change in resistance is proportional to the concentration of the target analyte in the surrounding environment. The electrical resistance of the sensing material is typically measured using a setup that includes electrodes connected to a measurement circuit. The resistance measurement can be performed using various techniques, including four-terminal measurements, two-terminal measurements, or impedance spectroscopy.
The measured resistance value is then correlated with the concentration of the target gas or VOCs using calibration curves or mathematical models. The selectivity and sensitivity of CGSs can be enhanced by functionalizing the sensing material's surface. This involves modifying the surface with specific coatings, catalysts, or receptors that selectively interact with the target analyte, increasing the sensor's response to the desired gas while minimizing interference from other substances. Overall, the operating principle of CGSs relies on the change in electrical resistance of a sensing material when exposed to target gases or VOCs, allowing for the detection and quantification of these substances as shown in Fig. 4. The simplicity, sensitivity, and selectivity of CGS make them attractive for a wide range of applications, including environmental monitoring, industrial safety, healthcare, and more.
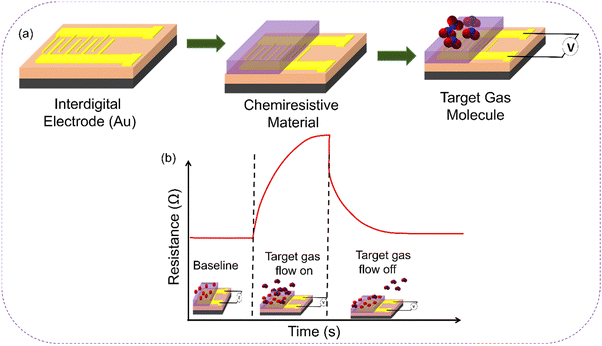 |
| Fig. 4 A schematic illustration provides the potential chemiresistive sensing mechanism, including: (a) the step-by-step synthesis of a chemiresistive gas sensor setup, (b) the interaction of the effect of gas molecules on inducing a positive change in resistance. Notably, upon the removal of gas molecules, the system returns to a steady-state baseline, indicating the reversibility of gas molecules interaction. | |
2.1. Device structure
Device structure, in the context of gas sensors, refers to the physical architecture and composition of the sensor's core components. It encompasses the arrangement of materials, electrodes, and other integral elements within the sensor framework. This seemingly technical aspect carries immense significance as it fundamentally shapes the sensor's performance characteristics. One critical facet influenced by the device structure is sensitivity. The meticulous selection of materials and their spatial organization can determine how responsive the sensor is to the presence of specific gases. For example, semiconductor gas sensors employ carefully designed sensing layers, where the arrangement of semiconductor materials directly impacts the sensor's ability to detect and respond to particular gases. The design and configuration of these layers are tailored to optimize sensitivity. Selectivity is another vital aspect influenced by the device structure. It determines the sensor's capacity to distinguish between different gases in complex environments. By engineering the sensor's structure to interact selectively with specific gas molecules, interference from unrelated gases can be minimized. This selectivity is crucial in applications where accurate identification of target gases is paramount, such as environmental monitoring or safety systems.
Fig. 5 shows the different device structures used for gas detection. Response time, a critical metric for gas sensors, is intimately tied to the device structure. The arrangement of sensor components can affect the time it takes for the sensor to detect and register changes in gas concentration. An optimized structure ensures rapid response, enabling timely actions in critical situations like gas leak detection or air quality monitoring. Furthermore, the overall performance and reliability of a gas sensor are heavily influenced by its structural design. A robust structure can withstand environmental variations, ensuring stable and consistent operation over extended periods. This durability is crucial, particularly in industrial settings where sensors may be exposed to harsh conditions. In essence, the device structure in gas sensors is not merely a technical detail but a foundational element that determines the sensor's ability to fulfil its intended purpose. It is through careful consideration and engineering of this structure that gas sensors can exhibit enhanced sensitivity, selectivity, response time, and reliability, making them invaluable tools across a spectrum of applications, from ensuring workplace safety to safeguarding the environment and advancing healthcare diagnostics. Looking forward to the future of gas sensor technology, the existing guidelines for device fabrication are poised to serve as a cornerstone for further advancements. These principles are expected to guide the development of next-generation gas sensors with enhanced capabilities and broader applications. Anticipated advancements include the exploration of nanostructured oxide semiconductors with even smaller crystallite sizes, potentially revolutionizing gas sensing by significantly boosting sensitivity. Future technologies may also focus on advanced methods for the precise dispersion of sensitizers within semiconductor materials, leading to sensors with remarkable selectivity and responsiveness. The optimization of sensing layer parameters, enabled by advanced materials engineering and computational modelling, promises to produce sensors that are both highly selective and exceptionally durable. Additionally, thin film-type gas sensors, often overlooked but showing promise, may gain renewed attention with the maturation of advanced fabrication techniques like Sputtering, PECVD, ALD etc. In this vision of the future, gas sensors are poised to play a pivotal role in addressing multifaceted challenges, from environmental monitoring to healthcare diagnostics, setting new standards in sensor performance and utility.
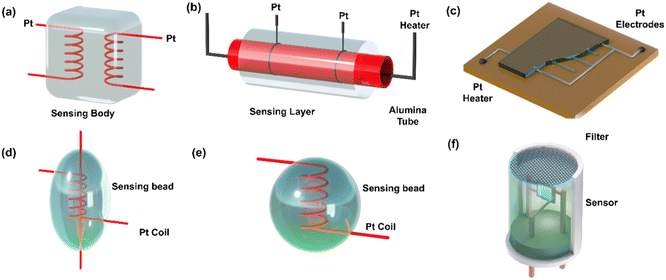 |
| Fig. 5 Chemiresistive gas sensors are commonly built using various structures, including (a) sintered blocks, (b) thin alumina tube coatings, (c) screen-printed thick films, (d) small beads with coil and needle electrodes, (e) small beads with a single coil (heater and electrode), and (f) practical sensor elements assembled with metal caps and filters. Redraw the picture with permission from ref. 60 Copyright 2023 Elsevier. | |
2.2. Electrical and gas sensing characterization measurements
The electrical and gas sensing properties of fabricated gas sensors with a comprehensive analysis of their performance conducted through a systematic measurement approach. The evaluation can be carried out using a multi-meter (Keithley 2400) with two conductive electrodes, as illustrated in the schematic diagram and digital photograph presented in Fig. 6. The experimental setup involves placing the sensors within a sealed chamber on a manually controlled heater equipped with one inlet and one outlet. Initial evacuation of the chamber should be performed using a high vacuum pump, achieving a pressure range from 760 torr to 10−3 torr. Subsequently, the carrier gas, either dry air or N2 gas, can be introduced into the sensing chamber, with precise control over the gas amount facilitated by external mass flow controllers (MFCs). Ensuring the formation of an Ohmic contact between the active layer and electrodes is a crucial step before each measurement. This confirmation can be achieved through current–voltage (I–V) measurements, wherein the applied voltage varies from −5 V to +5 V in increments in applied bias V. To enhance stability, the sensor is preheated before the actual sensing measurements. The gas dynamics of the sensor are assessed by passing the sensing gas (Target), along with the carrier gas, and measuring the resulting current and resistance. Furthermore, the sensing response can be examined at different temperatures by adjusting the heater temperature. The carrier gas flow rate is maintained in the chamber, while the sensing gas concentration is varied by controlling the flow rate. This systematic and controlled approach allows for a comprehensive examination of the gas sensors' performance, shedding light on their electrical and gas sensing capabilities under varying conditions.
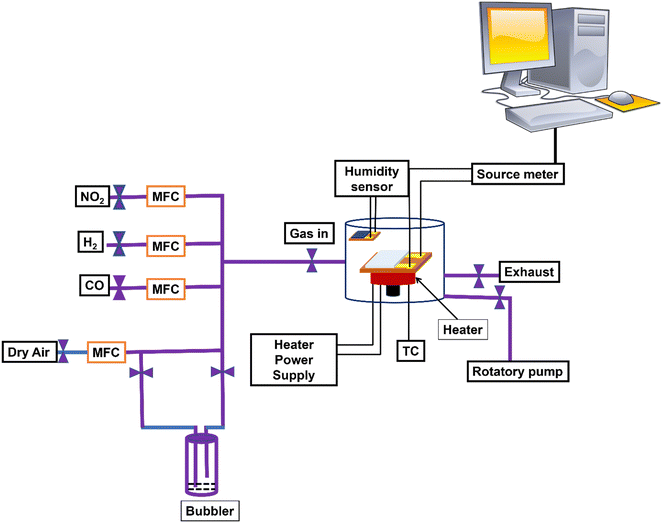 |
| Fig. 6 Schematic illustration of electrical and gas sensing measurements. | |
2.3. Fabrication techniques
Various methods have been employed to fabricate nanostructured metal oxide materials for gas sensing applications, each imparting a wide range of sensor characteristics. The properties of these materials heavily rely on their composition and structure, which, in turn, are dictated by the building blocks of nanoparticles. Key factors governing the overall gas sensor performance include particle size, morphology, and crystal structure.61 Synthesis techniques for nanomaterials can be broadly categorized as either “top-down” or “bottom-up” approaches.62–77 Top-down approaches typically initiate with a bulk material, from which nanoscale structures are created by subsequent material removal processes. Common top-down methods encompass e-beam lithography, photolithography, milling, and dry or ion/plasma etching. While top-down processes generally offer high manufacturing throughput, they have limited control over surface morphology. Additionally, these methods often involve complex fabrication techniques that are less suitable for cost-effective and large-scale industrial production, particularly in applications like gas sensing.
2.3.1. Top-down approaches.
Top-down approaches involve starting with a bulk material and then creating nanoscale structures by removing or etching away material. Common top-down methods include:
E-beam lithography.
This technique uses a focused electron beam to pattern a surface, allowing for the precise creation of nanostructures. However, it is limited in its scalability and is more suited for research and small-scale fabrication.
Photolithography.
Photolithography uses light to transfer a pattern onto a substrate coated with a photosensitive material. It is widely used in the semiconductor industry but may not be suitable for gas sensor materials that require specific morphologies.
Milling.
Mechanical milling involves grinding and reducing bulk material into fine nanoparticles. It is a versatile technique but can lead to agglomeration and limited control over particle size and shape.
Dry or ion/plasma etching.
These methods involve removing material from a substrate using chemical reactions or ion bombardment. While they offer high throughput, they may not provide precise control over surface morphology and often require sophisticated equipment.
Top-down processes are known for their potential for high manufacturing throughput, making them attractive for certain industries. However, they may not offer the level of control over surface morphology and composition needed for gas-sensing materials. Additionally, the complex fabrication techniques involved in some of these methods can be cost-prohibitive for large-scale production.78,79
2.3.2. Bottom-up approaches.
On the other hand, bottom-up approaches involve the assembly of nanomaterials atom by atom or block by block. Nanoparticle building blocks are generated on surfaces by depositing vapor molecules (in the gas phase) or ions (in the liquid phase). These atoms/ions are then assembled to form crystal planes or atomic clusters, which can subsequently grow into larger particles and material structures. These crystal planes and clusters ultimately give rise to the nanostructure of the sensing material. Alternatively, one can utilize nanoparticles synthesized in either the gas phase (aerosol) or the liquid phase (colloid) and then further manipulate these building blocks for desired sensor material properties.
Vapor phase deposition.
In this method, vapor molecules are deposited onto a substrate, where they assemble atom by atom or block by block to form nanostructures. Techniques like chemical vapor deposition (CVD) and metal organic chemical vapor deposition (MOCVD) atomic layer deposition, and Sputtering fall under this category. Solution-based methods: these methods involve creating nanoparticle building blocks in the gas phase (aerosol) or the liquid phase (colloid). These building blocks can then be assembled into desired structures. Solution-based techniques include sol–gel synthesis and hydrothermal/solvothermal growth. Bottom-up approaches offer precise control over crystal structure, particle size, and morphology. This level of control is essential for optimizing gas sensing materials to enhance sensitivity and selectivity. These methods are more suitable for research and development geared toward tailoring materials for specific gas sensing applications.80 In summary, the choice of synthesis method significantly impacts the characteristics of metal oxide nanomaterials for gas sensing applications. While top-down approaches offer high throughput, they often lack surface morphology control and cost-effectiveness. Bottom-up methods, on the other hand, enable precise control over material structure and are more suitable for tailoring properties to specific gas sensing requirements.
2.4. Gas sensing characteristics
2.4.1. Response and response transients.
The “response” of a gas sensor is a fundamental parameter that describes how the sensor reacts when exposed to a particular gas or a change in gas concentration. It essentially quantifies the sensor's ability to detect and respond to the presence of a specific gas. This response is typically expressed as a change in an electrical property (e.g., resistance, capacitance, voltage) or another measurable output of the sensor when exposed to the target gas. The response is often characterized by metrics such as sensitivity, which quantifies how much the sensor's output changes in response to a given change in gas concentration. For instance, in a semiconductor gas sensor, an increase in the concentration of a specific gas like carbon monoxide (CO) may lead to a decrease in the sensor's electrical resistance. The magnitude of this resistance change constitutes the sensor's response to CO. “Response transients” refer to the dynamic behaviour of a gas sensor during the transition from one gas environment to another. Gas sensors do not instantaneously reach a steady-state response when exposed to a new gas. Instead, they exhibit a time-dependent response, which includes an initial transient phase before stabilizing into a steady response. These transients encompass the sensor's adjustment to the new gas environment and are crucial to understanding sensor performance. The response transients provide insights into several aspects of sensor behaviour, including response time (the time taken to reach a stable response), recovery time (the time taken to return to the baseline response after gas removal), and the sensor's ability to discriminate between different gases during dynamic gas concentration changes. Monitoring and characterizing response transients are essential for assessing a sensor's suitability for specific applications. For instance, in safety-critical scenarios like gas leak detection, a rapid response time is crucial to ensure timely warnings, while a prolonged recovery time might be acceptable. In contrast, in applications where gas discrimination is vital, understanding how a sensor responds during transitions between different gases is essential for accurate detection and identification.
2.4.2. Sensitivity (S).
Sensitivity measures the responsiveness of a gas sensor to changes in gas concentration. It quantifies how much the sensor's output (e.g., resistance, voltage, or current) changes for a given change in gas concentration. High sensitivity means even small changes in gas concentration produce significant changes in the sensor's output. The formula for sensitivity is
where S is the sensitivity, ΔR is the change in sensor response (e.g., resistance), and ΔC is the change in gas concentration.
High sensitivity is desirable in applications where precise measurement of gas concentration is crucial, such as in medical diagnostics or air quality monitoring.
2.4.3. Selectivity (Se).
Selectivity evaluates a gas sensor's ability to distinguish between different gases or to respond predominantly to a specific target gas while minimizing interference from other gases. It is often expressed as a selectivity coefficient (K), calculated as
where Se is the selectivity, St is the sensitivity to the target gas, and Si is the sensitivity to an interfering gas.
Selectivity is vital in applications where multiple gases are present, and accurate detection of the target gas is essential. Gas sensors with high selectivity minimize false alarms and improve accuracy.
2.4.4. Response time (T90).
Response time measures the time it takes for a gas sensor to reach 90% of its final, steady-state response after exposure to a change in gas concentration. A fast response time is crucial in applications where rapid detection is critical, such as in safety systems. The formula for response time is
where T90 is the response time, t1 is the time when the gas concentration change is initiated, and t2 is the time when the sensor's response reaches 90% of its final value.
Short response times are desirable in scenarios like gas leak detection and industrial safety.
2.4.5. Recovery time (T90).
Recovery time measures the time it takes for a gas sensor's response to return to 10% of its final baseline value after the gas concentration has been reduced or removed. It is essential to understand how quickly a sensor returns to normal conditions after exposure to the gas. The formula for recovery time is the same as for response time:
But, in this case, it measures the time for the sensor's response to drop to 10% of the baseline. Short recovery times are important in applications where it is critical to confirm that a hazardous gas has dissipated.
2.4.6. Linearity.
Linearity assesses how well a gas sensor's response follows a linear relationship with gas concentration. A sensor is considered linear if its output varies proportionally with changes in gas concentration. Linearity is often evaluated through regression analysis, where the sensor's response is compared to known reference values over a range of gas concentrations.
In practical terms, a linear sensor provides accurate and consistent readings across a broad range of gas concentrations, simplifying calibration and data interpretation.
2.4.7. Accuracy.
Accuracy measures the closeness of a gas sensor's readings to the true gas concentration. It is typically expressed as a percentage or an absolute error. Accuracy is determined by comparing the sensor's measurements to known reference values under controlled conditions. High accuracy is essential in applications like medical diagnostics or environmental monitoring, where precise gas concentration measurements are critical for decision-making.
2.4.8. Range.
The range of a gas sensor defines the minimum and maximum gas concentrations within which it can provide reliable measurements. Sensors are often designed with specific concentration ranges in mind, and their performance may vary outside these limits. Choosing a sensor with an appropriate range is crucial to ensure accurate measurements for a given application.
2.4.9. Stability.
Sensor stability refers to the ability to provide consistent and reliable measurements over time. Stable sensors maintain their performance characteristics, including sensitivity and selectivity, over extended periods of use. Stability testing involves continuous monitoring of the sensor's response under specified conditions. Stable sensors are desirable in long-term applications, such as industrial process control and environmental monitoring, where consistent measurements are essential.
2.4.10. Cross-sensitivity.
Cross-sensitivity measures how sensitive a sensor is to gases other than the target gas. It quantifies the extent to which interfering gases can affect the sensor's response. Cross-sensitivity is often expressed as a percentage or ratio, indicating the sensor's response to an interfering gas compared to its response to the target gas.
3. Traditional sensing materials and fabrication techniques
Sensing materials play a crucial role in converting various physical, chemical, or biological signals into measurable signals, enabling the development of highly sensitive, selective, and reliable sensors. Fig. 7 shows a timeline of all reported materials in their development for successful chemiresistive gas sensors.
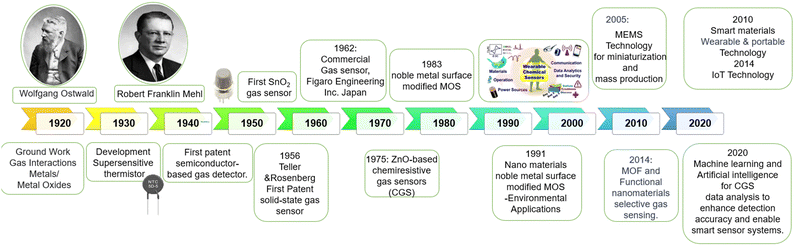 |
| Fig. 7 Timeline illustrating the developmental stages of reported materials for chemiresistive gas sensors.81–85 | |
3.1. Metal oxides
Metal oxide nanomaterials have garnered significant attention in the field of gas sensors due to their unique properties, high sensitivity, and low-cost fabrication. In the 1970s, Taguchi obtained a patent for using tin oxide chemiresistors as efficient gas sensors.86 Since then, extensive research has been focused on improving sensor performance in terms of sensitivity, selectivity, stability, and response time, while also reducing fabrication costs. MOSs such as ZnO, TiO2, WO3, Fe2O3, SnO2, CuO, NiO and Cr2O3 or Co3O4 have been extensively studied.61,87–93
Presently, metal oxide semiconductor (MOS) chemiresistors are widely adopted in industrial settings, particularly where target gas concentrations are relatively high (ppm or % levels). Renesas Corporation in Japan produces industrial MOS H2 sensors with an operational range from <10 to 1000 ppm, while Figaro Inc. manufactures MOS gas sensors for measuring H2S, NH3, CO, and other gases within the 10–1000 ppm range. MOS gas sensors show great promise for air quality monitoring due to their cost-effectiveness and portability, leading to significant efforts in optimizing their capabilities for detecting key gaseous pollutants. Although most MOSs exhibit changes in resistance upon the adsorption of gaseous species on their surface, specific materials demonstrate better suitability for different target gases. For instance, ZnO, SnO2, and TiO2 are commonly used for measuring CO, while WO3 is traditionally employed for NO2 sensors.94–96 Researchers have employed techniques such as doping, nano-structuring, and creating composites with other materials to enhance sensor specifications, including sensitivity, selectivity, and response/recovery times. Extensive research endeavours have been dedicated to the practice of doping or surface functionalization of metal oxides with noble metals such as Au, Ag, Pd, and Pt, among others. For instance, the deliberate incorporation of dopants like Pt and Pd, carefully dispersed across the surfaces of SnO2 grains, has yielded remarkable improvements in the gas sensing capabilities of SnO2, particularly concerning gases like CO, CH4, and NO2.97 Similarly, the introduction of Pd doping into SnO2 nanorod thin films, a process achieved by chemical vapor deposition and enhanced with plasma has significantly elevated their gas sensing performance, notably in the presence of H2 at 300 °C.98 Crucial factors affecting the sensitivity of Pd-doped SnO2 hollow nanofibers, obtained via electrospinning, include the concentration of Pd doping and the operating temperature. These factors played pivotal roles in determining their responses to gases such as H2, CO, CH4, and C2H5OH.99 Furthermore, an increase in the Pd dopant concentration in Pd-doped SnO2 nanowires led to a reduction in the operating temperature and an enhancement in the sensor's responsiveness to H2.100 This concerted effort in metal oxide doping and surface functionalization has opened up new avenues for advancing gas sensing technologies, offering the potential for highly sensitive and selective detection across a wide range of gases. Nanoscience has transformed material processing, allowing precise manipulation at the molecular level. Nanostructures, being smaller, offer increased surface areas for gas interactions, leading to compact, lightweight, energy-efficient, and highly sensitive sensors. ZnO, known for its versatile morphologies, such as nanowires, nanosheets, nanorods, nanobelts, nanocombs, nanotubes, nanohelices, and nanorings, has gained significant attention in gas sensing research.101–111 In particular, 1D nanowires and nanofibers have extremely large surface areas and narrow diameters, which is desirable for their sensing performance.112 1D nanotubes are the most representative structure in 1D materials. Compared with nanowire sensors, the surface area of the hollow 1D nanomaterials can be maximized to gain improved sensing performance.113 Extensive research has been conducted on one-dimensional (1D) composite metal-oxide materials, driven by their superior physical and chemical properties compared to individual components. Interactions within these metal oxide systems can alter the electronic properties of nanocrystals, influencing their reactivity and potential applications. Notably, sensor properties can be significantly enhanced by modifying composition, structure, and work functions. For instance, composite sensors combining SnO2 and ZnO exhibited greater sensitivity to butanol vapours than their individual components.114 Similarly, using WO3–SnO2 nanocomposites, with the 20 mol% WO3–SnO2 nanocomposite sensor displaying a remarkable response to NO2.115 Additionally, sensors based on the Fe2O3–In2O3 heterostructure exhibited the highest response to C2H5OH.116 Despite extensive research efforts, there remain several notable challenges when it comes to employing MOS (Metal Oxide Semiconductor) sensors for chemical monitoring. First, these sensors ideally demand high selectivity, meaning that they should be able to distinguish and accurately measure the target gas while remaining unaffected by other gases present in the environment. Additionally, sensitivity is a critical factor, and it must stay consistent regardless of fluctuations in temperature and humidity. Lastly, repeatability is paramount; MOS sensors must consistently provide reliable and consistent measurements over time. Recent advancements in nanomaterial synthesis, however, have offered solutions to some of these challenges. These breakthroughs have led to the development of MOS gas sensors that demonstrate remarkable limits of detection. These developments bring promising prospects for significantly enhancing the effectiveness of MOS gas sensors in a wide range of air quality monitoring applications.
3.2. Conducting polymers such as polyaniline, polypyrrole, and polythiophene
In recent years, there has been a remarkable surge in interest surrounding polymer-based chemiresistive gas sensors, primarily attributed to the unique properties inherent in organic conducting polymers. This category of polymers includes materials such as polypyrrole (PPy), polyaniline (PANI), polythiophene (PTh), poly(3,4-ethylenedioxythiophene) (PEDOT), and polyacetylene (PA), all of which have emerged as exceptionally promising candidates for advancing gas sensor technology.117–121 One of their standout advantages is their capability to operate effectively at room temperature. Moreover, these polymers are easily synthesized through chemical or electrochemical processes and possess commendable mechanical properties, making them highly desirable for gas sensing applications.122–124 The outcomes of research into conducting polymer-based gas sensors have been quite promising. For instance, sensors constructed with intertwined PANI nanowires, synthesized via electrochemical polymerization, have exhibited exceptional responsiveness to 0.5 ppm NH3.125 The sensing performance of 2D PANI films is particularly noteworthy, with the lowest detection limit recorded at 30 ppb for NH3 within a concentration range of 15 to 120 ppb at room temperature, surpassing the performance of most previously reported PANI film-based gas sensors.126 Furthermore, the versatility of organic conducting polymers is evident in their ease of synthesis and adaptability into various forms, including thin films, nanofibers, and nanoparticles.127–131 This adaptability empowers designers to create sensors tailored to specific requirements. Moreover, their chemical properties and sensitivity to particular gases can be fine-tuned through techniques like chemical doping, nano structuring, and composite formation with other materials, thereby amplifying the sensors’ performance and selectivity. For instance, adding Au nanoparticles (70–120 nm) to PANI nanowires improved H2S sensing. This enhancement includes better detection limits, superior selectivity, and reproducibility. The improved performance results from interactions between H2S and Au nanoparticles, as well as heightened PANI conductivity via electron transfer from PANI to Au.132 Nevertheless, despite their potential, polymer-based gas sensors have exhibited drawbacks in terms of low sensitivity, slow response and recovery times, poor thermal stability, and limited selectivity, which have constrained their broader utility. Fortunately, scientists have demonstrated that polymer/metal oxide nanocomposites hold promise in mitigating these shortcomings. These nanocomposites not only address the deficiencies of polymers and metal oxides but also significantly enhance their sensitivity, thermal stability, and response speed. Extensive scientific investigation has yielded a deeper understanding of the mechanisms responsible for these improvements in gas sensing performance. It is now established that alterations in microstructure and the creation of P–N junctions play pivotal roles in enhancing the sensing capabilities of these materials. Various nanostructured metal oxides like SnO2, TiO2, Fe2O3, GeO2, ZnO, WO3, Nb2O5, and MoO3 have been combined with PANI for gas detection.133–139 For example, PANI/TiO2 nanofiber sensors were developed for detecting low levels of NH3. P–N heterojunction monohybrids were formed through a polymerized reaction, enhancing NH3 sensitivity to 50 ppt.140 Notably, Li et al. introduced gas sensors consisting of hybrid PANI/WO3 nanocomposites on flexible PET substrates. They designed flower-like and hollow sphere WO3@PANI nanocomposites for detecting NH3 at room temperature. These sensors achieved impressive response values, with flower-like WO3@PANI reaching 20.1 and hollow sphere WO3@PANI reaching 25 in response to 100 ppm NH3 at room temperature.141,142 Jun et al. prepared sensors based on tube-in-tube SnO2@PPy construction for detecting DMMP at extremely low concentrations (0.05 ppb).143 In conclusion, polymer-based chemiresistive gas sensors, featuring materials such as polypyrrole, polyaniline, polythiophene, PEDOT, and polyacetylene, offer a compelling alternative to conventional metal oxide sensors. Their ability to operate efficiently at room temperature, coupled with their sensitivity and potential for low-power consumption, positions them as attractive candidates for the development of advanced gas sensing technologies with enhanced efficiency and reliability. Continued research and development in this domain are poised to see polymer-based gas sensors play a pivotal role across various applications, encompassing air quality monitoring, industrial safety, and environmental preservation.
3.3. Carbonaceous materials
Nanomaterials have ushered in a new era in the realm of gas sensors, representing a paradigm shift in sensor technology. These materials, which include carbon nanotubes, graphene, and metal nanoparticles, have redefined the boundaries of sensitivity, selectivity, and response times in gas sensing applications. Their unique properties and remarkable potential have sparked extensive research and development efforts, revolutionizing the field of gas sensors. Let's explore their individual contributions to gas sensor reports:
3.3.1. Carbon nanotubes (CNTs).
Carbon nanotubes (CNTs), with their extraordinary electrical, mechanical, and thermal properties, stand out as one of the most prominent nanomaterials in gas sensor innovation. The CNTs discovery in 1991 by Ijima has generated great interest among researchers to explore their unique properties.144 These cylindrical carbon structures exhibit an immense surface area-to-volume ratio, enabling efficient gas adsorption and interaction. CNT-based sensors have demonstrated exceptional sensitivity to various gases, including volatile organic compounds (VOCs), ammonia (NH3), Nitrogen dioxide (NO2), and carbon dioxide (CO2).145–147 The tunability of CNT properties, such as diameter, functionalization, and defect density, offers a versatile platform for tailoring sensor characteristics to specific gas targets. For instance, CNT films grown using PECVD exhibited strong sensitivity to NO2 at room temperature, striking a balance between higher resistance variations and fast, reproducible baseline recovery.148 Also, building upon the improved gas-sensing capabilities of one-dimensional hierarchical structures, the researchers developed hierarchical CNT composite nanomaterials. These nanomaterials came in two types: p-type (p-PANI/MWCNT) and n-type (n-PANI/MWCNT). They exhibited heightened sensitivity to NO2 and NH3, respectively, with rapid response times 5.2 seconds for NO2 and 1.8 seconds for NH3 and remarkably low detection limits (16.7 ppb for NO2 and 6.4 ppb for NH3).149 Functionalizing carbon nanotubes (CNTs) with various groups, metals, oxides, and polymers alters their electronic properties, enhancing selectivity and response to specific gases. Notably, the interaction between target molecules and these modifications varies significantly.150,151 For instance, carboxylated monolayer CNT-based sensors exhibited sensitivity to CO with a detection limit of 1 ppm, whereas pure monolayer CNTs did not respond to this gas.152 Additionally, the amino group (–NH2) functionalization of monolayer CNTs was studied for its NO2 gas sensitivity. The amino group acted as a charge transfer agent, increasing the number of electrons transferred from the nanotube to the NO2 molecule.153 Experimental and theoretical studies point to a promising future for CNTs in this field. Their unique structure and properties are poised to become integral components in sensors designed for detecting various materials, including gases and organic compounds. Modifying CNTs with functional groups, metal nanoparticles, polymers, and metal oxides is anticipated to significantly enhance sensor selectivity. Their impressive electric catalytic activity, rapid electron transfer capabilities, and the robust stability of nanotube compounds with redox polymers will position them as valuable assets in the realm of electrochemical biosensors. Ongoing research will focus on discovering novel modifying agents to further elevate the performance of CNT-based sensors.
3.3.2. Graphene.
Graphene is a single layer of carbon atoms arranged in a two-dimensional honeycomb lattice. Its outstanding electrical conductivity, large surface area, and high mechanical strength have made it a prominent candidate for gas sensing applications.154,155 Shi et al. reported the high-performance NO2 sensor based on chemically modified graphene, realizing an ultrahigh response and excellent selectivity, but the recovery time is too long (>30 min) to promote in practical gas sensing application. Theoretical studies highlight that introducing defects and dopants onto graphene significantly enhances sensitivity in graphene-based gas sensors by promoting stronger gas molecule adsorption.156 Zhang et al. conducted DFT calculations, revealing robust interactions between small gas molecules like NO2, CO, NO, NH3, and modified graphene (boron, nitrogen, and defective graphene) compared to pristine graphene (PG). Modified graphene displays higher gas molecule adsorption energies and more substantial charge transfer, distinguishing it from PG.157 Schedin et al. pioneered a micrometre-sized sensor using a few-layer PG, detecting single molecules of NO2 in high vacuum. They differentiated electron acceptors like NO2 and H2O from donors like CO and NH3 through their distinct effects on resistivity. This shows graphene's significant potential in gas detection.158 Functionalizing graphene sheets with organic molecules, metal-oxides, and metal particles through covalent bonding or supramolecular assembly is a widely adopted approach. Organic molecules with hydrophilic groups (e.g., –SO3−, –COOH, –OH) enhance rGO sheet dispersibility. Molecules with extra electric charges increase the interlaminar electrostatic repulsion, preventing aggregation among rGO sheets. Specific functional groups on organic molecules can significantly improve gas sensing performance. For example, Shi et al. modified graphene with sulfophenyl groups to create a sulfonated rGO (S–G) gas sensor, resulting in increased hole concentration and improved NO2 sensing. Donor groups like amidogen (–NH2) on organic molecules enhance electron transfer between gas molecules and graphene.159,160 Wan et al. created a flexible, transparent graphene-polyaniline (PANI) nanocomposite film for highly sensitive NH3 detection. The wearable sensor detected NH3 from 100 ppb to 100 ppm with a rapid response and recovery times of 36s and 18s, respectively. It exhibited specific NH3 response and reliable repeatability.161 Numerous metal oxides such as ZnO, SnO2, WO3, etc. were reported to form hybrid gas sensing materials with graphene for achieving sensitive gas detectivity.162,163 Liu et al. developed room-temperature H2S gas sensors using SnO2 quantum wire/rGO nanocomposites through a simple colloidal synthesis method. The sensors exhibited high sensitivity, selectivity, and rapid response-recovery for H2S concentrations between 10 and 100 ppm. Notably, they achieved a response time of 2s and full recovery at 22 °C. The enhanced H2S sensing performance resulted from the combination of SnO2 quantum wires and rGO nanosheets, which improved H2S adsorption and electron transfer, making these sensors effective.164 Noble metals boost graphene-based gas sensors, enabling effective room-temperature gas sensing through their catalytic properties and rapid electron transfer.165,166 Wang et al. achieved a 2.8 times response to 10 ppm NO2 using an Ag–NA–rGO sensor compare to SnO2-rGO, highlighting Ag nanoparticles’ role in enhancing NO2 adsorption and synergy with graphene sheets.167 Kim et al. developed a high-performance hydrogen sensor with graphene decorated with Pd–Ag nanoparticles using microelectromechanical systems (MEMS) techniques. The graphene-Pd/Ag nanocomposite sensors showed a low detection limit of 100 ppm, a response of 16.2% to 5000 ppm H2, and excellent gas sensing linearity. This enhancement stems from the coupling of graphene and Pd–Ag nanoparticles, significantly improving H2 sensing.168 Graphene-based composites, formed by combining graphene with metals, metal oxide nanoparticles, polymers, quantum dots, and nanowires, show immense potential for gas detection. Research has demonstrated their high sensitivity, selectivity, and enhanced sensing response across various gases and temperatures. Future prospects include exploring new material combinations to develop even higher-performing gas sensors. Research on graphene-based ternary and quaternary composites is ongoing, aiming to improve selectivity issues.
Yoon et al. presented a highly sensitive gas sensor, 10C-PPy@SLG, that detects NO2 and NH3. Fabricated via electrochemical polymerization on single-layer graphene, the sensor exhibits outstanding selectivity, sensitivity, and mechanical durability. It attains ultralow detection limits (0.03 ppb for NO2, 0.04 ppb for NH3) with swift response and recovery times. Remarkably, the sensor can be reset without heat or light treatment.169 Furthermore, Kwon et al. reported that ultrasensitive n-channel graphene gas sensors were developed using n-doping with ethylene amines, achieving selective detection of oxidizing gases. Graphene doped with diethylenetriamine (DETA) exhibited the highest sensitivity to NO2, with a detection limit of 0.83 ppq. The interaction between electron-rich graphene and NO2, supported by first-principles calculations, contributed to superior performance. The gate-free graphene sensors, enabled by non-destructive molecular n-doping, were successfully fabricated on flexible plastic, showcasing outstanding NO2 detection capabilities and potential for selective detection in gas mixtures.170 However, challenges remain in achieving large-scale production of high-quality graphene and ensuring environmental stability for industrial applications. Quantum detection of gases with graphene is advancing rapidly, although practical implementation requires overcoming certain limitations. Theoretical studies will continue to play a crucial role in understanding gas-graphene interactions and guiding the design of enhanced sensing systems. Future research will likely focus on hybridizing graphene with various functional materials to further improve sensor performance, considering sensitivity and selectivity as key factors. Comparisons with other gas sensing materials will also drive advancements in graphene-based gas sensor technology. In summary, traditional materials such as metal oxides, polymers, and carbon nanomaterials have significantly advanced gas sensor technology. Their exceptional properties, such as high surface area, electrical conductivity, and chemical reactivity, enable the development of highly sensitive, selective, and reliable gas sensors. Reports on gas sensors incorporating these nanomaterials continue to demonstrate their potential for various applications, ranging from environmental monitoring to industrial safety and healthcare diagnostics. Kim and her colleagues designed organometallic fluorescent probes to detect carbon monoxide (CO) and accurately measure carboxyhaemoglobin (HbCO) levels in animal blood. These sensors can work effectively even when exposed to a low CO dose of 100 parts per million for 10 minutes. Such CGSs can be designed for use in health sector gas detection applications.171
With ongoing research and innovation, nanomaterial-based gas sensors are likely to continue playing a crucial role in addressing real-world challenges related to gas detection and monitoring. While these sensing materials offer many advantages for gas sensors, they also have some disadvantages that should be considered in specific applications. Here are some of the key disadvantages:
Selectivity issues.
Metal oxide gas sensors can suffer from cross-sensitivity, where they respond to multiple gases simultaneously. This lack of selectivity can lead to false positive readings and make it challenging to accurately identify the specific gas of interest in complex gas mixtures.
Temperature dependence.
The sensitivity and response of metal oxide gas sensors are highly temperature-dependent. Operating the sensor at a specific temperature is crucial for optimal performance. However, this temperature dependence can make the sensor more susceptible to fluctuations in ambient temperature, leading to variations in the sensor's response.
Baseline drift.
Over time, metal oxide gas sensors may exhibit baseline drift, where their electrical resistance gradually changes even in the absence of the target gas. Baseline drift can affect the sensor's accuracy and reliability and may require frequent calibration.
Humidity sensitivity.
Metal oxide gas sensors can be sensitive to changes in humidity levels, which can interfere with gas sensing accuracy. High humidity can alter the sensor's response and introduce additional noise in the signal.
Long-term stability.
Some metal oxide gas sensors may experience degradation or loss of sensitivity over time due to environmental exposure or contamination. Ensuring long-term stability and maintaining consistent performance can be a challenge in real-world applications.
High power consumption.
Some metal oxide gas sensors require elevated operating temperatures for improved sensitivity. This can result in high power consumption, limiting their use in low-power and portable applications.
Response and recovery time.
Metal oxide gas sensors typically exhibit relatively slow response and recovery times compared to other gas sensing technologies. Rapid changes in gas concentration may not be accurately captured by these sensors.
Toxicity and environmental concerns.
Some metal oxide materials used in gas sensors may contain toxic elements, raising environmental and safety concerns during manufacturing, use, and disposal.
Sensor aging.
Over time, metal oxide gas sensors may experience degradation in their sensing properties, leading to reduced sensitivity and overall performance. Regular sensor replacement or maintenance may be necessary for long-term applications.
4. Overview of the key challenges in gas detection and the role of CGSs in addressing them
Gas detection poses several challenges that need to be addressed for effective monitoring and safety. CGSs play a significant role in overcoming these challenges. Here is an overview of key challenges in gas detection and how CGS addresses them: Sensitivity and selectivity. Detecting low concentrations of target gases and VOCs while minimizing false alarms from interfering substances is a challenge in gas detection. CGSs offer high sensitivity and selectivity by leveraging the specific interactions between the sensing material and the target analytes. Functionalization techniques further enhance selectivity, allowing for accurate detection and discrimination of specific gases or VOCs.
Real-time monitoring
Timely detection and response to gas leaks or hazardous substances are critical for safety and environmental monitoring. CGSs provide real-time monitoring capabilities, enabling rapid detection and immediate response. They offer continuous monitoring and can trigger alarms or activate safety measures when gas concentrations exceed pre-set thresholds, facilitating prompt actions to mitigate risks.
Portability and field applications
Some gas detection scenarios require portable and field-deployable solutions, such as industrial safety or environmental monitoring in remote areas. CGSs can be designed in compact formats, suitable for handheld devices or integration into wireless sensor networks. Their low power requirements and robustness make them ideal for on-site measurements and remote monitoring, ensuring effective gas detection in various environments.
Cost-effectiveness
Cost is a significant factor for widespread deployment of gas detection systems. CGSs offer cost advantages compared to certain alternatives. The fabrication processes for CGSs can be relatively simple and scalable, reducing production costs. Additionally, the sensing materials used in CGS are often affordable, contributing to overall cost-effectiveness.
Integration with IoT and data management
Managing and analyzing data from multiple gas detection sensors can be challenging. CGSs can be seamlessly integrated into IoT networks, enabling real-time data transmission, remote monitoring, and centralized data management. This integration facilitates data analysis, pattern recognition, and the development of predictive models, enhancing the effectiveness of gas detection systems.
Long-term stability and reliability
Long-term stability and reliability of gas detection systems are crucial for continuous monitoring. CGSs are designed to be robust and resistant to environmental factors. Ongoing research focuses on improving the stability of sensing materials, reducing drift, and enhancing the longevity of chemiresistive sensors, ensuring reliable and accurate performance over extended periods.
CGSs address these challenges by providing high sensitivity, selectivity, real-time monitoring, portability, cost-effectiveness, and integration capabilities. As research and development in this field continue, further advancements in chemiresistive sensor technology will enhance gas detection systems’ performance, making them more efficient, reliable, and accessible for a wide range of applications in safety, environmental monitoring, and industrial processes.
4.1. Performance optimization and signal transduction
Performance optimization and signal transduction are critical aspects of CGSs to ensure accurate and reliable gas detection. Here is an overview of the key considerations for optimizing the performance of CGSs and the techniques employed for effective signal transduction:
Sensing material selection.
The choice of sensing material is crucial for achieving the desired performance characteristics. Different materials exhibit varying affinities and responses to target gases or VOCs. Selecting a suitable sensing material with high sensitivity and selectivity towards the target analyte is essential. Additionally, the stability and repeatability of the sensing material's response should be considered for long-term performance.
Surface functionalization.
Functionalization techniques can enhance the interaction between the sensing material and target analytes, improving sensitivity and selectivity. Surface functionalization can involve the deposition of specific coatings, catalysts, or receptors that selectively bind to the target analyte. This process enhances the adsorption and detection of the desired gas, while minimizing interference from other substances, thus optimizing the sensor's performance.
Transduction techniques.
CGSs employ various transduction techniques to convert the changes in electrical resistance into measurable signals. The choice of transduction technique depends on the specific application requirements and the desired sensitivity. Common transduction methods include direct resistance measurement, impedance spectroscopy, and frequency-dependent measurements. These techniques allow for the accurate quantification of the target gas or VOC concentration.
Signal processing and analysis.
Signal processing and analysis techniques play a vital role in extracting meaningful information from the sensor's response. This involves filtering and amplifying the sensor's electrical signal, followed by data analysis and interpretation. Signal processing techniques can enhance the sensor's signal-to-noise ratio and improve the detection limit. Advanced data analysis methods, such as pattern recognition algorithms and machine learning techniques, can be employed to identify and classify different gases or VOCs based on their unique sensor responses.
Calibration and validation.
Calibration is essential for establishing a relationship between the sensor's electrical response and the concentration of the target analyte. Calibration curves or mathematical models are developed using known concentrations of the target gas or VOC. Regular calibration and validation ensure the sensor's accuracy and reliability over time.
Stability and drift compensation.
Long-term stability of CGSs is crucial for continuous and reliable gas detection. Sensor drift, caused by environmental factors or aging effects, can lead to false readings and reduced accuracy. Drift compensation techniques, such as baseline correction, temperature compensation, and periodic recalibration, help maintain the sensor's stability and compensate for any drifts, ensuring consistent and accurate measurements.
Overall, optimizing the performance of CGSs involves careful selection of sensing materials, surface functionalization, appropriate transduction techniques, signal processing, calibration, and addressing stability and drift issues. By considering these factors and employing suitable techniques, CGSs can achieve high sensitivity, selectivity, and accuracy, enabling reliable gas detection for various applications in healthcare, safety, environmental monitoring, and industrial processes.
4.2. Strategies to improve selectivity and sensitivity
Functionalization techniques are used for enhancing the interaction between sensing materials and target analytes. Transduction methods include resistance measurement, impedance spectroscopy, and other signal transduction approaches. This comprehensive review provides valuable insights into the advancements made in chemiresistive sensor technology, highlighting its potential to revolutionize gas detection across various sectors. The knowledge and understanding presented in this review will aid researchers, engineers, and stakeholders in harnessing the full potential of CGS and driving further advancements in this field.
5. Chemiresistive gas sensing of porous materials
To highlight the importance and advancement of this field, some very good reviews have already been published on porous materials for sensing purposes.172 We have also tried to summarise all of the prominent porous materials such as MOFs, COFs, POPs and their hybrid examples which are also tabulated as given in the respective sections based on pristine and hybrid sensing materials. Metal–organic frameworks (MOFs) have emerged as an interesting class of porous materials that possess highly crystalline structures, robust frameworks, first-rate high surface area, high conductivities and tunable pore sizes. These properties of MOFs make them practically useful and highly functional in many arenas such as energy storage,173–175 gas storage176–178 and separation,179–181 sensing,182–184 biomedical field185–187 and other scientific areas. Looking into a world where sensing is one of the inevitably required things, MOFs are one of those materials that have proved themselves to work excellently in this field owing to the requisite properties that are required for the purpose of sensing.188–190
5.1. CGS sensing metal–organic framework based materials
5.1.1. Pristine MOFs.
The very first work of using a pristine MOF for chemiresistive gas sensing was reported in 2014, when Zhang et al.191 studied the chemiresistive nature of the cobalt-based zeolitic imidazole framework (Co-ZIF-67); although gas sensing with ZIFs has already been studied before,192 the ‘chemiresistive’ sensing technique for gas sensing by using a MOF was never been used before. In this work, the group studied and demonstrated the sensing of various gases such as acetone, formaldehyde, methanol, and triethylamine (Fig. 8a–d). The band Gap of a material is a crucial property of a material that plays a driving role in its chemiresistive nature since the optimum band gap is directly related to the conductive properties of a material. MOFs generally have a high band gap of ∼4–8 eV which is not suitable for their application involving electrical properties. In this work, Co-ZIF-67 was used which was found to have a low band gap of 1.98 eV which is adequate for the electrical conductivity required for chemiresistive operation. The porous sodalite-like structure of Co-ZIF-67 possesses high specific surface area (1832.2 m2 g−1), providing a highly accessible surface for the interaction of gases (Fig. 8a). Among the targeted gases, formaldehyde was found to give the highest sensing response (Fig. 8b). Response and recovery performances (Fig. 8c) of this material were found to be fast but not faster than typical metal-oxide-based sensing materials. This can be due to the high surface area of MOFs that take more time to reach the highest adsorption–desorption equilibrium extent and thus shows the highest resistance value. The selectivity of a gas by a particular sensing material is due to various factors (discussed in Section 4). The same group reported the use of a cobalt imidazolate framework [Co(Im)2]n as a sensor for trimethylamine (TMA) gas (Fig. 8d). The resulting imidazole framework exhibits excellent selectivity, high gas response, and a low detection limit of 2 ppm, primarily due to the weak interaction between the TMA molecules and the framework.
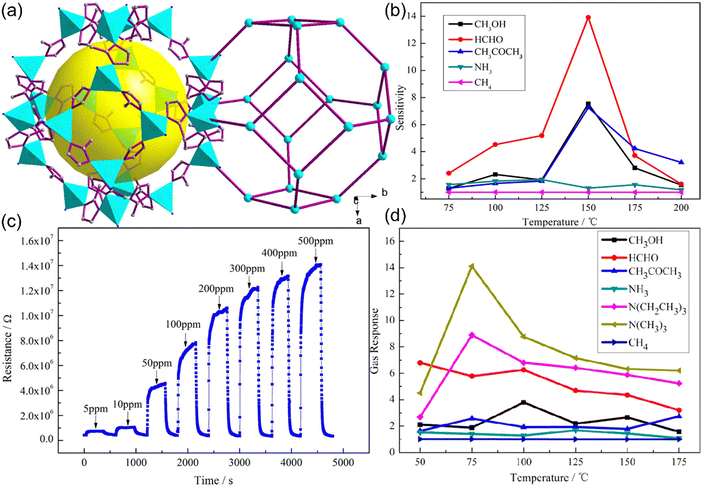 |
| Fig. 8 (a) Sodalite topology of Co-ZIF-67. (b) Sensitivity of the Co-ZIF-67 sensor to various gases from 75–200 °C. (c) Chemiresistive sensing performance of ZIF-67 at different gas concentrations. Reproduced with permission from ref. 191 Copyright 2014, American Chemical Society. (d) Sensitivity of the [Co(im)2]n sensor to various gases from 50–175 °C. Reproduced with permission from ref. 193 Copyright 2014, American Chemical Society. | |
The ZIF-based materials were able to sense specific gases with high selectivity and good detection limits, but when it comes to sensing diversity for gases, efficient sensing was shown only for a few analyte gases. Also, the band gap is low for the ZIF-based MOFs but still, higher conductivities are needed for fast chemiresistive sensing operations. Conductivity in chemiresistive gas sensing materials is of utmost importance in order to bring the best performance out of them. Although high conductivities in MOFs were reported previously,194–201 their applications remain limited and had never been used specifically for chemiresistive gas sensing before the revolutionary work by Campbell et al.202 (Fig. 9a). High electrical conductivities of two-dimensional structures inspired the fabrication of 2D MOFs using such specific metals that can form 2D geometries as well as 2D organic linkers such as triphenylene, porphyrin and phthalocyanine-based monomers. In this current work, authors synthesized various novel 2D conducting Cu and Ni-MOFs (2D-cMOFs).202,203 Response-recovery performance of the Cu3(HITP)2 based chemiresistive gas sensor (Fig. 9b) revealed a fast chemiresistive response of the sensing material towards various NH3 vapour concentrations. The swift response could be due to the 2D morphologically provided highly accessible interaction sites. Since Ni and Cu are in different electronic configurations (d8vs. d9, respectively), the theoretical studies suggest that the charge transfer between the target gas and the sensing 2D MOF material directly impacts the electronic response because metals with different electron counts result into different Fermi levels and thus tune the band gap values.204 For example, it is predicted that the use of higher electron count elements in place of Ni, such as Cu, in M3HITP2 type MOFs raises the Fermi level of this conducting material (Fig. 9d and e).205 This was also found to be legitimate in experimental evidence as polar analytes such as methanol, ethanol, and acetone show high variations in sensing responses whereas not much difference could be seen for non-polar analytes, such as cyclohexane and pentane, which lack free electrons (Fig. 9c). These results provide us with the opportunity to tune the sensing performance of chemiresistive gas sensors by examining the effect of versatile metals in 2D MOFs. To further study the effect of different metals on the sensing of gases in 2D MOFs, Dinca et al. worked out three triphenylene-based 2D MOFs with copper and nickel for metallic nodes. The high intrinsic conductivities of these MOFs come through the planar extended structure that is stacked on one another to form 1D pores. The electrons are free to move more swiftly through the rigid and planar triphenylene cores consisting of conjugated double bonds and electron-rich nitrogen and oxygen atoms, in the case of HITP and HHTP, respectively. Carefully looking at the sensing performances of these 2D MOFs with various analytes, the Cu3(HITP)2, Co3(HHTP)2 and Ni3(HITP)2 show high differences in sensing responses. The main difference is suggested to come majorly from different metal centres (Cu vs. Ni), although other factors such as different heteroatoms (NH and O) in HATP and HHTP linkers, respectively, and sensing device nature should also have significant effects.
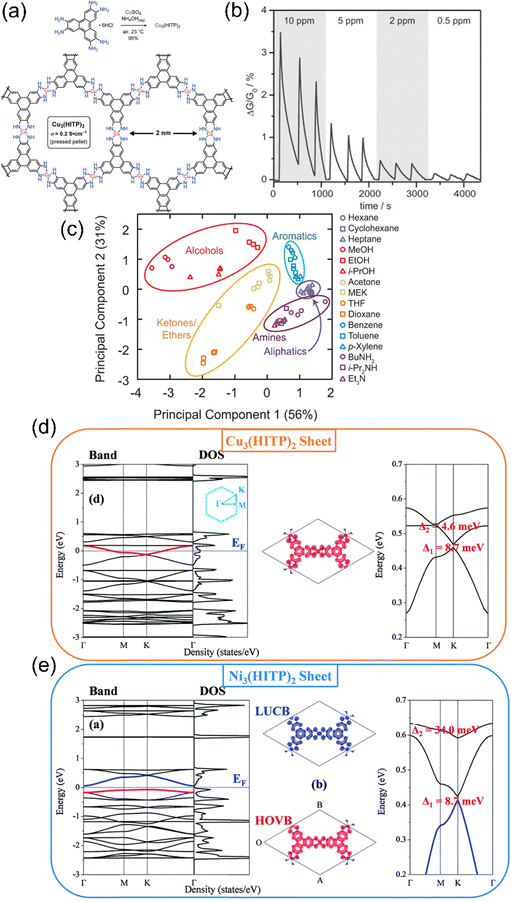 |
| Fig. 9 (a) First report on 2D conductive MOFs (with conductivity and pore size) (b) relative responses of a Cu3(HITP)2 sensor to 0.5, 2, 5, and 10 ppm of ammonia. Reproduced with permission from ref. 202 Copyright 2015, Wiley-VCH. (c) PCA analysis for groupings of various VOCs for sensing purposes. Reproduced with permission from ref. 203 Copyright 2015, American Chemical Society. (d) and (e) Theoretical band, DOS, charge density isosurface and Kagome bands and SOC gaps in 2D M3(HITP)2 MOFs (d) Ni3(HITP)2 and (e) Cu3(HITP)2 respectively. Reproduced with permission from ref. 204 Copyright 2015, Royal Society of Chemistry. | |
Another essential parameter that cannot be overlooked while studying the sensing of an analyte is the limit of detection or LOD values. The LOD of a sensing material can be described by the ability of the material to sense the lowest gas concentration and produce a readable intensity of the chemiresistive signal. This parameter is directly related to the interaction between the analyte and the sensing material. The sensing material would be able to sense the target analyte when the analyte molecules get free access to the materials’ interaction sites and the interaction causes a change in the electronic parameters of the material.
MOFs are known to provide record-breaking surface area from a materials perspective172,206 and accessible functional sites207,208 for analytes owing to their highly ordered rigid porosity due to excellent crystallinity. Two-dimensional MOFs possess ordered long 1D channels with accessible metal and linker functional sites that induce sub-ppm level detection of analyte gases. For instance, 2D conductive MOFs were able to sense lower gas concentrations. Conductive MOFs provide reliable high cross-reactive detection of a particular analyte gas among various VOCs and high electrical conductivities favour the ease of sensing response for gas analytes. However 2D MOFs with powder or bulk morphology are not much favourable for fast charge transfer and also fast gas diffusion. In this regard, Yao et al. came up with a layer-by-layer (LBL) fabrication technique for synthesizing conductive MOFs, here Cu3(HHTP)2-xC, which can excellently control the 2D conductive MOF's thin-film thickness down to an accuracy of 2 nm (Fig. 10a–c).209 The LBL thickness controlled synthesis of 2D-cMOFs was effected by simultaneously spraying metal salt and organic ligand on the –OH functionalized substrate such as quartz, sapphire etc (Fig. 10a). This improved the sensitivity of already existing 2D conductive MOFs that were used in powder or bulk forms, as depicted by the sensing of the ammonia gas (ΔRavg = 129%) which is many folds as compared to the previous studies (Fig. 10b and c).210 This thin layer morphology of the MOFs also helped in a fast response time due to the fast accessibility of the available surface area to the target gases. In terms of the layered growth of 2D conductive MOFs, Smith et al.,183 have been working extensively on textile-based materials, came up with a 2D conductive MOF fabrication methodology on textiles for flexible MOFs using Ni3(HITP)2 and Ni3(HHTP)2 MOFs via a bottom-up modular growth approach (Fig. 10d). This methodology led to the expected sub-ppm level detection of gases (NO and H2S), high gas responses (49–98%) and room temperature sensing. MOFs can also be equipped with various functionalities that can play as interaction sites for various gases and VOCs to produce chemiresistive modulation in the sensing MOF material. MOFs can be tuned very easily in terms of functionality which comes from the organic linkers. Different gases interact with different functional groups with some particular force of interaction and this feature can be easily induced into MOFs to improve the MOF–gas interaction. One of the such initial studies was demonstrated by Wang et al.211 where they exploited the free carboxyl functionalities of MOF linkers for their strong H-bonding ability with volatile amine protons. MD Mello et al. played with the interaction sites in the organic linker fragments of UiO-66 MOF where they used BDC, BDC–NH2 and BDC–OH as organic linkers for the detection of acidic gases such as NO2, SO2etc.212 As expected from common acid–base interactions, in these MOFs, basic functionalities such as NH2, OH etc interacted in a similar way with the acidic gases such as NO2, SO2etc. Since there is some electronic charge transfer from basic to acidic moieties in acid–base interactions, the resulting change in the electron density of the basic moiety can be seen reflected as a chemiresistive signal when employing the MOF material in chemiresistive gas sensing.
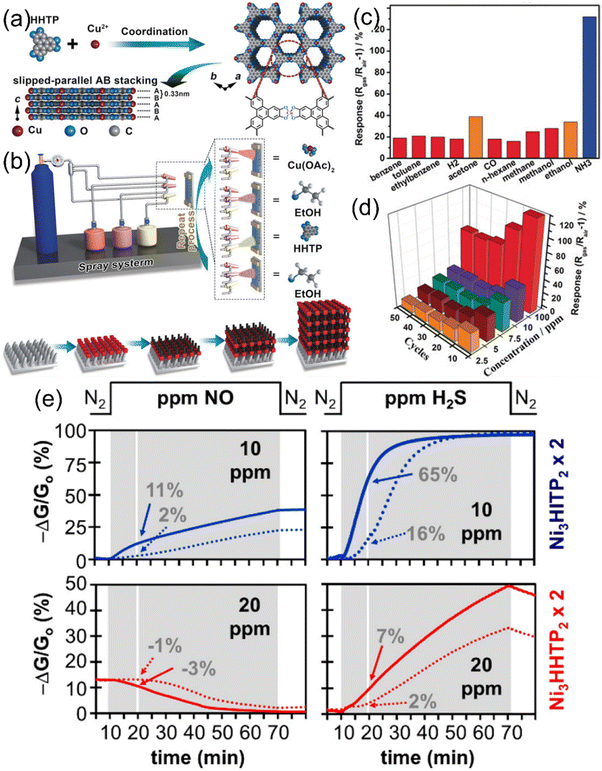 |
| Fig. 10 (a) Illustration of the Cu3(HHTP)2 crystal structure (b) the preparation of Cu3(HHTP)2 thin-film gas sensors. (c) Response of Cu3(HHTP)2 towards different reducing gases. (d) Response of Cu3(HHTP)2 towards NH3 analytes with different concentrations. Reproduced with permission from ref. 209 Copyright 2017, Wiley-VCHGmbH. (e) Customized Teflon container with SOFT-textile-MOF based sequentially stacked sensing material and gas response curves for the I-material (solid line) and II-material (dashed line) for Ni3(HHTP)2 and Ni3(HITP)2. Reproduced with permission from ref. 183 Copyright 2017, American Chemical Society. | |
The detection of the gases was carried out at a temperature of 150 °C, but for an ideal gas sensor, it should be operable at ambient temperature to widen the operation window of gas sensing in various fields. At elevated temperatures, the multiple heat cycles can lead to abnormal crystal growth which can break the electronic interconnections213 and can also reduce the fabricated sensors' lifetime. Thus, chemiresistive gas sensing is always sought and preferred at room temperature over high-temperature gas sensing. But, high temperature is required for higher sensitivity and reversibility in the case of most of the traditional metal-oxide gas sensors. Since porous materials provide a high degree of sensing material–gas analyte interaction owing to their high surface area and approachable interactive sites due to their highly crystalline nature (especially in the case of MOFs and COFs), thus high-temperature requirements for gas sensing are unneeded. Extensive work on 2D MOFs and the study of their electronic properties to apply for chemiresistive gas sensing has been done. The gas sensing performance gives excellent results at room temperature revealing the structural and functional superiority of 2D porous materials such as 2D MOFs over conventional non-porous materials. Stassen et al. worked up on copper hexaiminobenzene(HIB)-based 2D electronically conductive MOFs for CO2 sensing.214 Cu3(HIB)2 consisting of imino-semiquinonate (–NH–) groups which are electron-rich and thus interact with acidic gases such as CO2. –NH– moieties are found to be of significant relevance since CO2 sensing with the hexaoxytriphenylene (HOTP)-based MOF, Cu3(HOTP)2 provided unmeasurable signals indicating the efficient electron donating influence of imino moieties in contrast to oxo moieties. Working in the direction of 2D conductive MOFs, Meng et al. fabricated phthalocyanine-based 2D MOFs containing Ni and Cu as metal centres and as phthalocyanine-cavity metal – thus producing bimetallic 2D MOFs.215 These 2D MOFs provided three major advances in chemiresistive gas sensing. First, the presence of two-dimensional structures of the MOFs due to planar, conjugated phthalocyanine linkers, they provide a high surface area, excellent conductivity, and a highly ordered distribution of active sites in the sensing material. Intrinsic conductivity of metal-phthalocyanine moieties provides ultra sensitivity to the sensing material towards the target gases (H2S, NO, NH3). Second, by varying the organic linker (metallophthalocyanine and metallonaphthalocyanine), sensitivity and selectivity can be tuned via isoreticular modulation of the sensing MOF material. Third, due to high intrinsic conductivities (∼10−2 S cm−1), excellent sensing performance can be seen even at lower sensing voltages (∼0.01 V to 1.0 V).
Thus, the sensor requires a lesser amount of energy to operate. 2D c-MOFs provide highly electroactive chemiresistive sensing materials but faster sensing performance and fair gas selectivity are challenging task. Improvising this condition, Wang et al. improved these two features in a single 2D c-MOF by tuning its surface-polarity.216 Usually, 2D c-MOFs are hydrophilic in nature due to the presence of metal nodes and polar atoms such as N, O etc (Fig. 11a). Grafting with long alkyl chains, hydrophobicity can be introduced into the same material. With the infusion of hydrophobicity, the adsorption/desorption of water vapours becomes more frequent, thus decreasing response/recovery times by a much greater extent. For instance, surface modification studies of 2D c-MOF Ni2[MPc(NH)8] with organosilanes such as phenyltrichlorosilane (PTCS), (3-aminopropyl)trimethoxysilane (APTMS), and octadecyltrimethoxysilane (OTMS) (Fig. 11b). Out of these, Ni2[MPc(NH)8]-OTMS produced the highest levels of hydrophobicity (water contact angle = 138°) which leads to faster recovery from humidity (Fig. 11c) and thus was used as a VOC sensing material. Since the polarity trend of lower alcohols follows methanol > ethanol > isopropanol trend, methanol with the highest polarity showed the fastest diffusion and response towards the surface modified sensing material (Fig. 11d–f). Thus, surface modification of 2D c-MOFs established opportunities to develop these materials in the field of electronics. Discussing the advantages of 2D c-MOFs, they provide a 2D surface to the analytes for the interaction for sensing purposes. The surface underlying area thus remains unapproached by the analyte particles which was highlighted by Lin et al. while proposing an improvement in this scenario by replacing 2D c-MOFs with LBL grown 3D c-MOFs.217 Langmuir–Blodgett deposition of one kind of material on the relevant substrate provides a thickness-controlled and highly oriented material designing approach. This approach was used to fabricate a Cu-HHTP based 3D sensing material which otherwise previously used to be synthesized as 2D c-MOF materials. LBL over flat surfaces yields only surface-exposed materials whereas over 3D substrates such as a nanowire array, provides higher surface area of layered material with higher exposure to active sites. This resulted in an ultralow detection of ammonia gas (5 ppb) which is about a thousand times lower than reported for 2D Cu-HHTP MOFs. Since the 3D Cu-HHTP material is grown on TiO2-NWAs, which doesn’t show an observable response towards the ammonia gas, the sensing performance of this material can be considered solely due to the 3D-grown Cu-HHTP MOF.
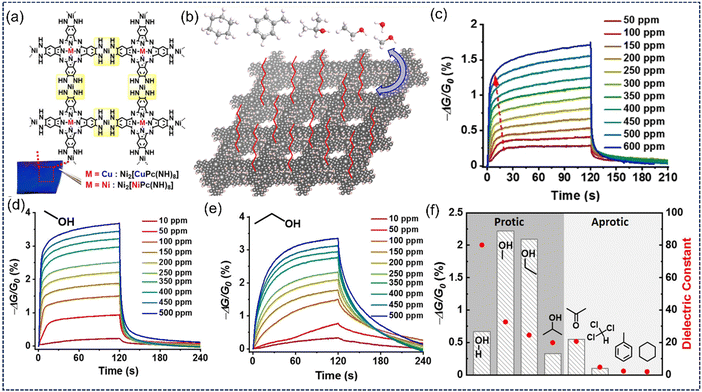 |
| Fig. 11 (a) Chemical structure and Ni2[MPc(NH)8] (M = Cu & Ni). (b) Surface modification of Ni2[MPc(NH)8] MOF by APTMS, PTCS and OTMS and (c) response curves (−ΔG/Go values vs time) for different H2O vapors concentration fed to the sensing material. (d) and (e) Response curves (−ΔG/Go values vs time) for different (d) methanol and (e) ethanol concentrations fed to the sensing material. (f) Response curves (−ΔG/Go values vs. time) of various protic and aprotic analytes interact with Ni2[MPc(NH)8]. Reproduced with permission from ref. 216 Copyright 2021, Wiley-VCH. | |
Carrying the excellent potential of 2D-based c-MOFs, Meng et al. (Fig. 12a and b) and Aykanat et al. synthesized (Fig. 12c–e) bimetallic phthalocyanine and napthalophthalocyanine based 2D conducting MOFs in two different studies but by the same group to enlighten the sensing and differentiating various gases such as H2S, NH3 and NO in the former studies by Meng et al. and CO gas sensing tunability and intensifying the sensing performance in the latter studies by Aykanat et al.218 In the former experiments, isoreticular synthesis of robust bimetallic MOFs and their chemiresistive response towards various gases reveals the effective interaction between analyte and sensing material due to the presence of uniformly distributed metallic and linker sites, which are able to effectively differentiate between various types of gases. During the latter experiments, Co and Ni-based MPc-O8-Cu MOFs were generated, structured as Co or Ni inserted hydroxy-functionalized phthalocyanine linkers interconnected via Cu nodes. The 2D nature and presence of bi-metals in the structure resulted into the high conductivity, thus providing the advantage of using a low voltage (0.1 V) to power the sensing device and receive optimal results. The bimetallic phthalocyanine pattern of the sensing material helped for two particular reasons – first, the need for active CO host sites and the sites for electronic transduction of the electronic perturbation due to CO interaction with the metal-phthalocyanine MOF.
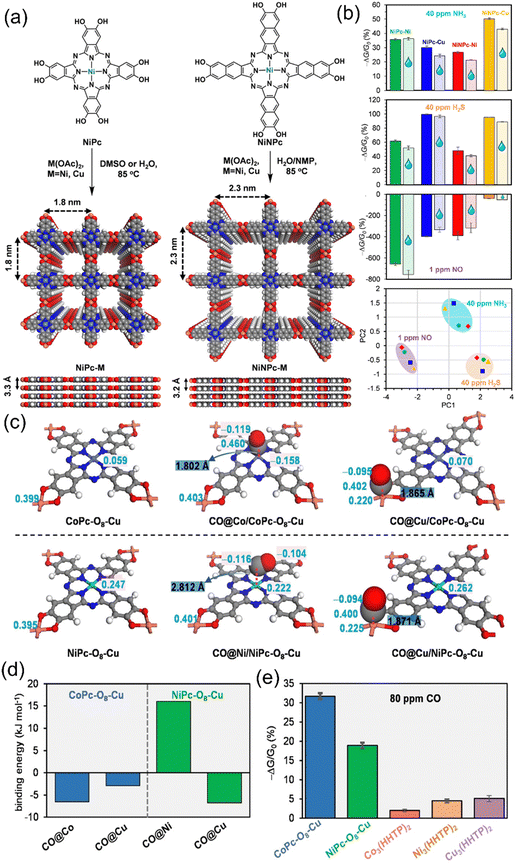 |
| Fig. 12 (a) Illustration of and naphthalocyanine- and phthalocyanine-based MOFs – NiNPc-M and NiPc-M respectively. (b) Chemiresistive gas sensing response for NiPc-M and NiNPc-M MOFs upon exposure to 40 ppm of NH3 and H2S, and 1 ppm of NO in dry nitrogen (solid bar) and drenched in 5000 ppm H2O (bar with droplet symbols). PCA analysis for NiPc-Cu (blue), NiPc-Ni (green), NiNPc-Cu (yellow) and NiNPc-Ni (red) sensor arrays. Reproduced with permission from ref. 183 Copyright 2017, American Chemical Society. (c) Bonding optimization of Co- and Ni-based Pc-MOFs. (d) Binding energy calculations for CO interaction with Co and Ni-Pc MOFs. (e) Highest sensing performance for Co- and Ni-based Pc-MOFs as compared with other triphenylene linker-based 2D c-MOFs. Reproduced with permission from ref. 218 Copyright 2015, Wiley-VCHGmbH. | |
Second, the presence of Cu induces redox active charge hopping through the 2D-cMOF network by acting as a node for metallophthalocyanine network extension. The presence of these features in these MOFs helped in the ultralow sub-ppm level detection of CO. Further insight into the CO sensing performance was achieved by computational studies using DFT calculations. The interaction of CO with Co-based MOFs (Fig. 12c) was optimized and some constant CO–Cu and CO–Co bonding distances were realized for the best sensing results. The linearity of these bonds was realized by the metal–CO back bonding. Binding energy optimization studies revealed the incompatibility of the Ni based MOF to bind CO efficiently because of the positive free energy for the Ni–CO binding whereas the negative Co–CO free energy suggested the positive results in the case of the Co-based MOF (Fig. 12d). Also, the high sensitivity of the Co-based MOF than the Ni-based MOF can be explained by the same theoretical reasons. The superior response from phthalocyanine based sensing materials as compared with the triphenylene based 2D sensing materials could be because of the presence of doped Co- and Ni- metallic entities. Thus, the synthesis of pristine MOFs, their incorporation into the fabricated chemiresistive gas sensing device and tuning of their features such as porosity, functionalization, and morphology results in the tuning of sensing performances of the pristine MOF-based gas sensors (Table 1).
Table 1 MOF and its hybrids/derivatives for chemiresistive gas sensors
S. no. |
Material |
VOCs |
Sensing mechanism |
Response (%) |
LOD (ppm) |
t
res–trec (s) |
Ref. |
DA = Donor–acceptor; CT = Charge transfer;Charge carrier transport = CCT;Ethylenediamine = EDA;Formaldehyde = FA;Trimethylamine = TMA;Electron transfer = ET;Intrasheet conductivity = IC;Proton transfer = PT;Hydrogen bonding = HB. |
1 |
ZIF-67 |
TMA |
|
14.1 |
2 |
|
193
|
2 |
ZIF-67 |
FA |
|
13.9 |
5 |
|
191
|
3 |
Cu3(HITP)2 |
NH3 |
|
|
0.5 |
|
202
|
4 |
M3(HHTP)2 (M = Cu, Ni) |
Various VOCs |
CT |
|
|
|
203
|
5 |
Ni3(HHTP)2@textile |
NO |
CT |
49 ± 10 |
1.4 |
|
183
|
6 |
H2S |
98 ± 9 |
0.23 |
|
7 |
Ni3(HITP)2@textile |
NO |
CCT |
81 ± 6 |
0.16 |
|
8 |
H2S |
97 ± 2 |
0.52 |
|
9 |
Cu3(HHTP)2 |
NH3 |
DA |
129 |
0.5 |
81.6–46.6 |
209
|
10 |
Cd(H2L)2 |
EDA |
HD-DA |
|
|
<3–10 |
211
|
11 |
[Cd(TMA)(DPP)0.5 H2O]n |
Humidity |
Proton hopping |
|
|
11–56 |
282
|
12 |
UiO-66-NH2 |
SO2 |
DA |
21.6–2.7 |
1 |
26.8 ± 5.4 |
212
|
NO2 |
7.6–0.4 |
10 |
|
CO2 |
11.4–2.2 |
500 |
35 ± 2 |
13 |
Cu3HIB2 |
CO2 |
DA |
−0.62 |
|
420–660 |
214
|
14 |
Cu-TCPP@ Cu-HHTP |
NH3 |
CCT |
94 |
|
91.8–643.2 |
283
|
C6H6 |
|
153 |
|
15 |
NiNPc |
NH3 |
CT/Redox action |
43–45 |
0.31 |
|
215
|
H2S |
64–98 |
0.019 |
NO |
657–397 |
0.001 |
16 |
Cu-BHT |
NH3 |
ET |
−7.88 |
0.23 |
58–102 |
284
|
17 |
Ni2[MPc(NH)8] |
CH3OH |
Proton transfer |
4.7 |
10 |
36–13 |
216
|
18 |
Cu-HHTP |
NH3 |
Charge/Mass transport |
42 |
0.005 |
35–900 |
217
|
19 |
Cu-HITP |
NH3 |
CCT |
7.1 |
0.5 |
|
285
|
20 |
NiPc@CoTAA |
NO2 |
ET |
37.6 |
|
300–3600 |
286
|
21 |
HITP@Cu-HHTP |
NH3 |
CCT |
80 |
0.024 |
<60–600 |
287
|
Benzene |
75 |
0.096 |
22 |
HIB-Cu |
Humidity |
CT |
|
200 |
21–40 |
288
|
23 |
Zn-BDC-NH2 |
H2 |
|
2.93 |
|
|
289
|
24 |
CoPc-O8-Cu |
CO |
|
27.4 ± 0.8![[thin space (1/6-em)]](https://www.rsc.org/images/entities/char_2009.gif) |
0.5-3 |
|
218
|
NiPc-O8-Cu |
|
18.9 ± 0.8![[thin space (1/6-em)]](https://www.rsc.org/images/entities/char_2009.gif) |
|
|
25 |
Co−pyNDI |
NH3 |
CT |
46.7 |
0.00015 ppb |
168–198 |
290
|
Ni−pyNDI |
NH3 |
426 |
Zn−pyNDI |
|
32.5 |
26 |
Cu2O/CuO |
Ethanol |
DA |
|
|
|
291
|
27 |
ZIF-CoZn/ZnO |
Acetone |
DA |
27 |
0.0019 |
43.2–61.2 |
292
|
28 |
ZnO@ZIF-8 N |
H2 |
DA |
1.44 |
|
|
113
|
29 |
ZnO@ZIF-8 |
FA |
DA |
— |
5.6 |
16–9 |
223
|
30 |
Pd@ZnO (ZIF-8)-WO3 NFs |
Toluene |
DA |
S = 4.37 |
0.1 |
|
293
|
31 |
PEDOT@MIL−101(Cr) |
NO2 |
DA |
0.9 |
0.06 |
30–150 |
259
|
32 |
PdO@ZnO(ZIF-8)-SnO2 |
Acetone |
DA |
5.6 |
0.010 |
<20–64 |
294
|
33 |
PdO@Co3O4(ZIF-67) |
Acetone |
DA |
2.51 |
0.1 |
|
295
|
34 |
Pd NWs@ZIF-8 |
H2 |
DA |
3. |
0.6 |
7–10 |
225
|
35 |
ZnFe2O4@MOF-5 |
Acetone |
DA |
64.4 |
|
|
296
|
36 |
PdO@Co3O4-nSnO2 |
Acetone |
DA |
22.8 |
1 |
90.8–108.4 |
297
|
37 |
ZnO@ZIF-8 |
Acetone ethanol |
DA |
|
|
|
298
|
|
ZnO@ZIF-67 |
|
|
|
|
38 |
Pd-ZnO@ZnCo2O4 |
Acetone |
DA |
69 |
|
|
299
|
39 |
ZIF8/Pd/ZnO NWs |
H2 |
DA |
8.5 ± 0.5 |
|
|
227
|
40 |
ZIF- Co3O4 rods@ZnO |
Acetone |
DA |
25 |
0.005 |
|
300
|
41 |
ZIF-67@Co3O4 |
p-Xylene |
DA |
78.6 |
|
63–86 |
301
|
Toluene |
43.8 |
|
42 |
ZIF-67@WS2 |
NO2 |
DA |
48.2 |
0.1 |
|
302
|
43 |
ZIF-67@SnO2 |
CO2 |
DA |
48.2 |
|
18–25 |
229
|
44 |
POM@ZIF-8@ZnO |
FA |
DA |
4.4 |
0.387 |
15.1–16.2 |
230
|
45 |
TiO2/Co3O4 NFs by TBT@ZIF-67 |
Ethanol |
DA |
16.7 |
5 |
|
303
|
46 |
ZnO NSs@ZIF-L |
CO |
DA |
3.2 |
0.134 |
30–1 |
304
|
VOCs |
1.4 |
0.02 |
47 |
Pt@Cu3(HHTP)2 |
NO2 |
DA |
62.11–57.38 |
|
828–840 |
233
|
48 |
Ag@ZnO@ZIF-71 |
H2 |
DA |
|
|
|
226
|
49 |
Au-ZnO@ZIFs |
Acetone |
DA |
231 |
0.0001 |
|
305
|
50 |
In2O3/MoS2 MIL-68(In) |
NO2 |
DA |
371.9 |
|
|
306
|
51 |
MIL-53(Al)/CNT |
CO2 |
Pore transition of MOF |
15 |
|
< 30 |
249
|
52 |
ZIF-8/ZnO NRs |
H2S |
DA |
52.1 |
0.05 |
420 |
307
|
53 |
HKUST-1/MoS2 |
H2O |
PT |
8–14 |
|
0.38 |
182
|
54 |
Cu(BTC)@PDMS |
CO |
DA |
−0.46 |
|
|
264
|
MIL-160@PDMS |
Humidity |
|
|
55 |
Ln(acac)3@ZIF-8 |
NO2 |
DA |
187.9 |
0.0002 |
1050–1230 |
308
|
56 |
CoSnO3@MOF |
H2S |
DA |
12.1 ± 10 |
0.00018 |
|
234
|
57 |
G@Cu-BTC |
CHCl3 |
DA |
∼0.15 |
>1 |
|
309
|
|
G@ZIF-8 |
∼0.10 |
|
|
G@UiO-66 |
∼0.04 |
|
58 |
ZnO/CuO from Zn/Cu-BTC |
H2S |
DA |
393.35 |
0.0003 |
173–3000 |
310
|
59 |
GA@UiO-66-NH2 |
CO2 |
DA |
8.6 |
|
18 |
221
|
60 |
GO@PDDA@Co3(HITP)2 |
NO2 |
IC |
9.05 |
0.0068 |
24–41 |
311
|
61 |
t-ZnO@ZIF-8 |
H2 |
DA |
546 |
|
1–2 |
228
|
5.1.2. MOF hybrids/derivatives.
MOFs can be broadly classified into two types of materials – one type that includes MOFs as templates or precursors for hybrids/composites that contain MOF as a constituent and other types that includes materials that themselves don’t contain MOF entities but are derived from MOFs. Metal-oxide semiconductor (MOS)-based chemiresistive gas sensors have already been developed significantly by improving their sensing performance by hybridizing with heteronanostructures,219 doping or loading of metal oxides/mixed metals220 and making composites with carbon materials such as graphene.221 MOFs provide an exceptional surface area and a robust framework for granting chemical stability to the materials they get linked with. MOF–MOS composites have been developed with critical insight into the conductivity, stability, selectivity and sensing time of the resultant material in chemiresistive gas sensing. These composites have mostly served as an upgrade to the pristine materials. MOFs can have fixed-size pores that can be used for molecular separation.222
Drobek et al recognized this trait of MOFs and used it for creating a metal-oxide/MOF composite viz. ZIF-8 cloaked ZnO nanowires (NWs) (ZnO/ZIF-8 NWs) (Fig. 13a) for size-based response selectively to H2 while being aloof for larger C6H6 and C7H8 molecules (credit to ZIF-8 porosity) while giving excellent sensitivity to H2 at 300 °C (credit to ZnO NWs) (Fig. 13b).113 Since MOF synthesis requires a metal source, Tian et al. followed an interesting strategy where they used ZnO nanorods (NRs) as the source for the zinc ions for the synthesis of ZIF-8 MOF shell around themselves.223 This synthetic technique not only provided the MOF–MOS core–shell heterostructure, the selectivity ratio for formaldehyde sensing was also improved (Fig. 13c) with respect to other interfering VOCs such as ethanol, acetone, ammonia etc. in contrast to the bare ZnO nanorod sensors because of the molecular sieving via ZIF-8 windows.224 Koo et al using a similar protocol, fabricated H2 gas sensors by developing a ZIF-8 nanofiltration network on Pd NWs.225 This work improved the hydrogen gas sensing speeds and selectivity by fastening the adsorption/desorption of H2 molecules on/from Pd-NWs. Molecular sieving was exploited to restrict the entry of larger molecules of O2 (0.345 nm) and N2 (0.364 nm) than H2 molecules (0.289 nm) with a ZIF-8 micropore size of 0.34 nm. Molecular sieving properties of pristine MOFs can be modulated with the help of metal nanoparticles. As metal NPs have the tendency to be engulfed inside the MOF pores, their spacious occupation further restricts the larger molecules and thus smaller gas molecules can be separated more specifically.
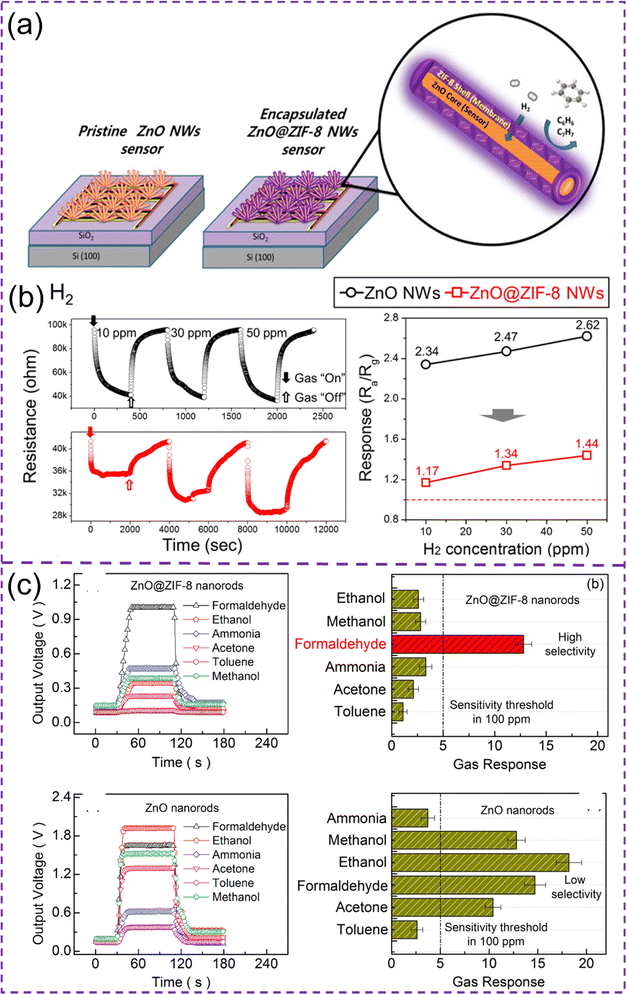 |
| Fig. 13 (a) Structural illustration of ZnO/ZIF-8 NWs. (b) H2 sensing performance of ZnO/ZIF-8 NWs (left). Responses of ZnO (black) and ZnO/ZIF-8 NWs (red) towards H2 (right). Reproduced with permission from ref. 113 Copyright 2016, American Chemical Society. (c) Various VOCs sensing response curves and selectivity for ZnO/ZIF-8 NRs and pristine ZnO NRs. Reproduced with permission from ref. 223 Copyright 2016, American Chemical Society. | |
Zhou et al., while considering this idea, performed H2 sensing by using Ag NPs encapsulated ZnO/ZIF-71 which showed higher selectivity towards H2 and decreased response for acetone owing to their size preferences (Fig. 14a).226 This work thus supported the enhancement of metal oxide sensing performance which otherwise would have been poor as the normal case. The variation in the sieving process changes from pore to pore due to the different levels of Ag nanoparticles filling inside the pores of ZIF-71. Temperature programmed desorption (TPD) studies revealed the obstruction of acetone desorption while supporting the hydrogen desorption by increasing the silver nanoparticle concentration inside the ZIF-71 pores (Fig. 14b). This revealed the effect of pore size tuning on the molecular sieving by embedding metal nanoparticles inside the cavities of the MOF and thus the subsequent sensing of singled out gases. The sensing response of the ZnO@ZIF-71⊃Ag NRA towards acetone and hydrogen with different Ag-loading concentrations also support the metal nanoparticle based sieving tuning where with an increase in the Ag-load, H2 response increases and acetone response decreases (Fig. 14c).
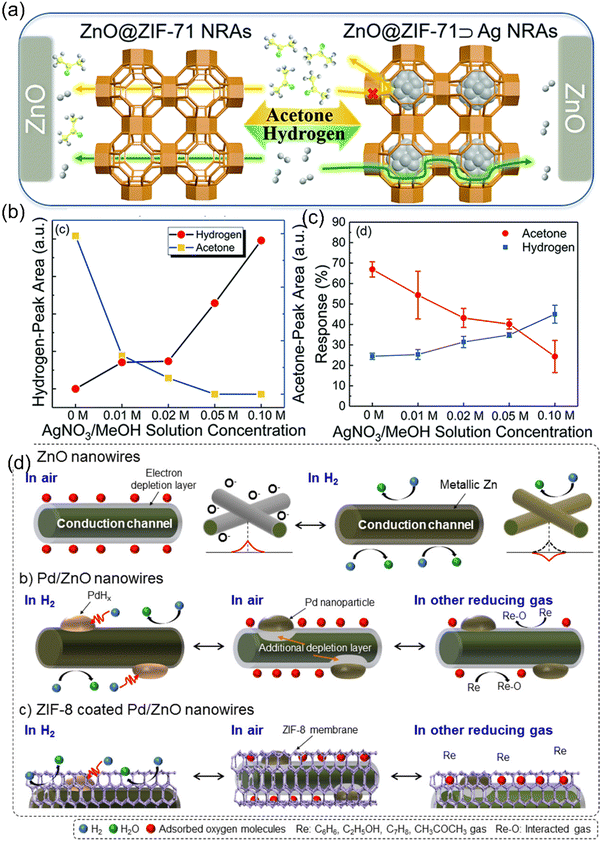 |
| Fig. 14 (a) Schematic illustration of H2 sensing by tuning the molecular sieving properties of ZIF-71 by incorporating Ag NPs. (b) The total desorption peak area control of acetone-TPD spectra and H2-TPD spectra. (c) The sensing response of ZnO@ZIF-71⊃Ag NRAs for various Ag NPs concentration loading to 20 ppm H2 and acetone. Reproduced with permission from ref. 226 Copyright 2021, Royal Society of Chemistry. (d) Schematic illustration of the sensing mechanism for ZnO NWs, Pd/ZnO NWs and ZIF-8/Pd/ZnO NWs. Reproduced with permission from ref. 227 Copyright 2018, American Chemical Society. | |
Similarly, recently, the molecular sieving property of ZIF-8 was exploited for the H2 sensing at lower temperatures of 100 °C as compared to the previously studied ZnO-NPs/ZIF-8 composites. In this work, Poschmann et al. pointed out the use of ZnO nanoparticles in polycrystalline powder form and the conductivity hindrance effect of the ZnO–MOF–ZnO sequenced sensing material morphology.228 Thus, they used the tetrapodal single-crystalline ZnO microparticles to avoid this undesired conductivity reduction. The ZIF-8 layer over the single-crystalline ZnO microparticles provides excellent selectivity towards the H2 molecules, even in the presence of optimum methane concentration. This is observed due to the pinhole-free morphology of ZnO microparticles plus ZIF-8's microporosity. Another compounding reason is the increased rigidity of the pore windows due to the composite formation with single unit ZnO particles. Here, due to the single-crystalline nature of the ZnO particles which are surrounded by MOF layers, the change in conductivity cannot possibly occur due to the lack of adsorbed atmospheric oxygen molecules. This might be because of the presence of oxygen defects on the crystal surface which provide the interacting surface for the hydrogen molecules and result into the change in the electrical parameters. One important thing to note here is the optimum thickness of the MOF layers grown over the metal/metal-oxide nanowires. Thin MOF layers are being used here to provide molecular sieving and faster diffusion, whereas thicker MOF layers decrease the traveling speed of gas molecules and thus increase the response and recovery times. SnO2 NPs/ZIF-67, due to the tuneable porosity of the MOF counterpart, was explored as a novel gas sensing material by DMello et al.229 The exclusive and stable sensing response to CO2 was delivered because of the suggested synergistic effect between SnO2 and ZIF-67. Synergistic involves the transfer of electrons at the interface of ZIF-67 and SnO2 from the organic linker (Imidazole) to the MOS metal (Sn) and parallelly from the oxide to the MOF metal (Co), which stabilizes the carbonate formation from CO2 after interaction with oxides. Synergistic effects were further explored by Wang et al. as they studied formaldehyde detection via higher photocurrent emergence values for the POM@ZIF-8@ZnO (POM = Polyoxometalate) as compared to ZIF-8/ZnO nanorods.230 ZIF-8 pores served as an analyte concentrating medium so that formaldehyde molecules can gather densely and produce an equivalently higher photocurrent. These observations further corroborated the advantageous role of synergistic effects of metal-oxide-MOF combinations. Getting some mechanistic insight into these excellent outcomes of metal-oxide NWs/MOFs hybrids, incorporating metal nanoparticles greatly advances the sensing performance of the MO NWs/MOFs materials. Metal atoms can exist as agglomerated nanoparticles which are found to show a spill-over effect. Gaseous molecules while interacting with the metallic nanoparticles dissociate into smaller fragments and thus can interact more intensely with the surrounding host material.
Hydrogen spill-over effect (HSPE), first discovered in 1964, is a peculiar surface phenomenon in which H2 molecules dissociate into H-atoms after being “cut” by tiny metal nanoparticles and subsequently “spill” over the supported material.231 Weber et al. fabricated a ZnO NWs/ZIF-8 hybrid embedded with Pd nanoparticles (NPs) for chemiresistive H2 sensing and increased selectivity excellently towards the H2 sensing compared to bare ZnO NWs observed because of the molecular filtering out VOCs other than H2 on the basis of size.227 The improved results were seen because of the spill-over effect due to Pd-NPs combined with the nanofiltration due to ZIF-8 pores. Although Pd@ZnO NWs/ZIF-8 exhibits a lower response towards H2 sensing, the porous ZIF-8 cladding helped this material to outperform both Pd@ZnO NWs and ZnO NWs in H2 selectivity. The comparison of H2 sensing mechanisms for ZnO NWs, Pd/ZnO NWs and ZIF-8@Pd/ZnO NWs as gas sensing materials is schematically shown in (Fig. 14d). H2 being a reducing gas leads to reduction of ZnO to Zn metal in ZnO NWs and additional Pd NPs to PdHx in Pd/ZnO NWs and ZIF-8@Pd/ZnO NWs, each of which causes conductivity increments. Due to the absence of a spill-over effect for the reducing gases other than H2 results in no significant enhancement in the sensing performance for these VOCs, thus producing a better comparative study of H2 sensing. Metal nanoparticles are remarkable catalysts and due to their nano-sized nature, they can be infused into nano, micro and macroporous materials. Smaller size of metal NPs provides them with larger surface area which thus improves the catalytic performance of their hybrids.232
Based on these properties of metal NPs, their encapsulation in MOFs has also shown an enhancement in their catalytic properties. 2D conductive MOFs incorporated into metal NPs such as Pd, Pt and Au NPs, have been shown to improve both their catalytic as and conducting properties. Koo et al. demonstrated the revamping of Cu-HHTP based c-MOFs by fusing them with Pd and Pt NPs (Fig. 15a and b).233 The catalytic effect of metal NPs was observed for Pt@Cu3(HHTP)2 and can be supported by the activation energy depletion of NO2 adsorption by the sensing material which resulted in the higher decrease in the p-type c-MOFs resistance while interacting with strong electron acceptor NO2 gas (Fig. 15e). In the case of Pd@Cu3(HHTP)2, the activation energy was only raised up but it still showed a decrease in the resistance of the sensing material. This provided an insight further into the electronic structure change upon NO2 adsorption on the sensing material. While interacting with the Pd NPs, NO2 molecules interacted via the formation of a nitrito-complex with the Pd NPs, absorbing electron density from them, and thus reducing the Schottky junction barrier that persists between Pd NPs and c-MOF. This increased the conductivity of the sensing material and thus enhanced its sensing performance via electronic sensitization of NO2 by Pd NPs (Fig. 15e). This provides the sensing material with a more enhanced response towards the NO2 gas molecules (Fig. 15c) and thus acts as a state-of-the-art NO2 gas sensing device (Fig. 15d). The study of reaction kinetics of these eventual reactions was beneficial for understanding the effect of metal nanoparticles’ catalytic properties on the NO2 gas interaction reactions with the sensing material surface. Adsorption/desorption rates of NO2 on/from M@Cu3(HHTP)2 were enhanced more prominently during Pt NP doping as compared to Pd NP doping. This trend was observed because Pt NPs decrease the activation energy of NO2 interaction with the metal nanoparticles while Pd NPs increase the activation energy for the same as compared with pristine Cu3(HHTP)2.
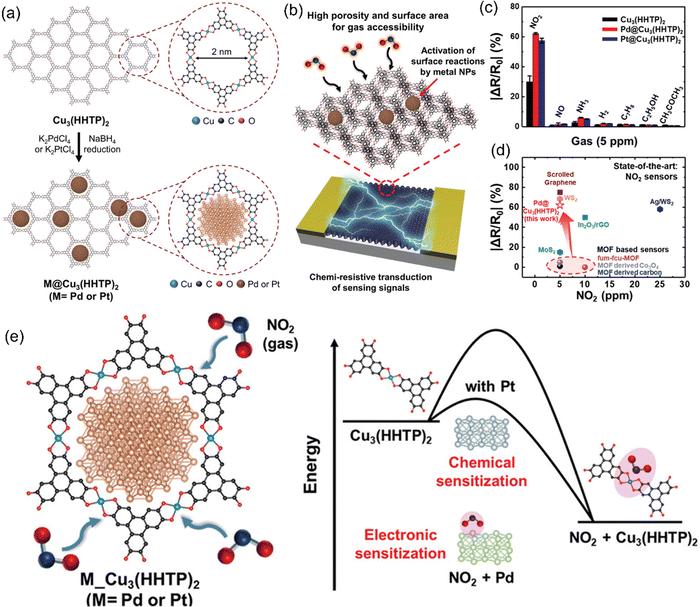 |
| Fig. 15 (a) Schematic illustration of the structure of Cu3(HHTP)2 2D MOF synthesis of M@Cu3(HHTP)2 (M = Pd or Pt). (b) Chemiresistive gas sensing conceptual illustration for NO2 interaction with M@Cu3(HHTP)2. (c) calculated response toward NO, NH3, H2, C7H8, C2H5OH, and CH3COCH3 analytes(d) Responses of interaction of various VOCs with Cu3(HHTP)2 and M@Cu3(HHTP)2. (e) Schematic illustration of the interaction of NO2 on M@Cu3(HHTP)2 and Cu3(HHTP)2. Reproduced with permission from ref. 233 Copyright 2019, Wiley-VCHGmbH. | |
Chemiresistive gas sensing by metal oxides usually provides exceptional sensitivity and response as compared to pristine porous materials because of their direct surface accessibility to the target gases. However their poor selectivity makes them less efficient in generating the response data more accurately and the presence of humidity makes them even more vulnerable. The solution to this inevitable situation was demonstrated in an intriguing work by Qu et al. where they improved the sensing performance of a bimetallic oxide CoSnO3 by enclaving them inside MOF/PDMS (Fig. 16a) combination that provides corroborated advantages of molecular sieving by MOFs and hydrophobicity by PDMS.234 The effect of humidity on the sensor performance can be seen while performing the H2S gas sensing by pristine CoSnO3, CoSnO3/MOF and CoSnO3/MOF/PDMS. Since the hydrophobic nature of PDMS provided a high shield from humidity, it can be seen that there is barely an effect on the baseline resistance due to the stability of the encapsulated CoSnO3/MOF against humidity (Fig. 16b–d). Thus, growth of hydrophobic materials such as PDMS by chemical vapour deposition (CVD) on the humidity sensitive gas sensing materials can provide an assuring technique for synthesizing highly stable gas sensing materials. This work makes use of the advantages of CVD such as uniform coating of the materials and thickness control of the coating material. Universal application of this technique was demonstrated by implementing the technique on other metal-oxide/MOF composites such as SnO2/MOF@PDMS and ZnO/MOF@PDMS showing similarly improved results in contrast with their pristine counterparts (Fig. 16e and f).
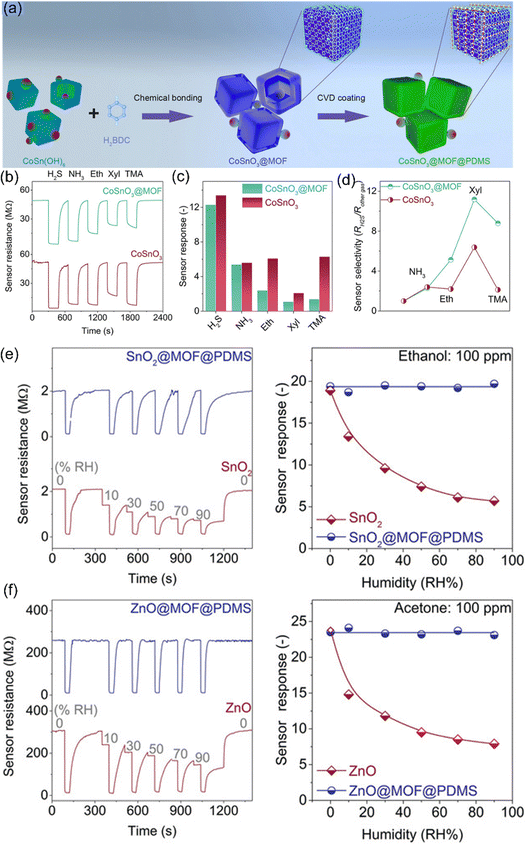 |
| Fig. 16 (a) Schematic illustration of tandem MOF/PDMS encapsulation on CoSnO3 nanocubes. (b) and (c) Dynamic response curve for various gases and sensor response in CoSnO3, CoSnO3@MOF and CoSnO3@MOF@PDMS nanocubes for H2S interaction under dry and humid conditions. (d) Selectivity enhancement of CoSnO3@MOF over pristine CoSnO3. (e) Dynamic gas sensing transients and sensor response in SnO2 and SnO2@MOF@PDMS nanocubes for ethanol interaction (e) and in ZnO and ZnO@MOF@PDMS for acetone interaction (f) under dry and humid conditions. Reproduced with permission from ref. 234 Copyright 2020, Wiley-VCHGmbH. | |
Carbon exists in various allotropic forms such as diamond, graphite, fullerenes, graphene, nanotubes, lonsdaleite, quantum dots and so on. These allotropes of carbon have been serving as chemiresistive gas sensing materials, in pristine235,236 as well as composite forms.237–240 MOF–Carbon composites have been used for gas adsorption,241,242 molecular separation,243 energy storage,244 chemical sensing245 and so on. Chemical sensing by pristine MOFs is undoubtedly possible but due to their poor conductivities and lower quantum yields, their hybrids with carbon materials provide a passage to improved sensors which have proved to give enhanced sensing performance.246,247 Many such chemical sensors have been employed in electrochemical and luminescence-based sensors, while chemiresistive gas sensing events are rare due to a limited number of reported conductive MOFs. Some MOFs are reported to show a stepwise change in the crystallites’ structure (a property known as switchability) upon interaction with analytes248 but entities which further lead to transduction into measurable signals are very few. Careful insight into these unique properties of MOFs by Freund et al., a switchable MOF composite with carbon to form conductive films was evolved into a CO2 gas sensor. For this, MIL-53(Al) was blended with carbon nanotubes (CNTs) and carbon black (CB) since the MOF is non-conductive and CNTs and CB are highly conductive – thus forming a conductive composite with chemiresistive nature. The mechanism of chemiresistive analyte sensing in switchable MOF crystals/carbon composites is demonstrated in Fig. 17a. The change in resistivity of this composite happens because carbon additives, when added to MOFs, form a percolating network that forms an electronic flow pathway when the addition reaches a particular threshold value. A structural change occurs in the percolation network which then causes a subsequential change in the electrical parameters of the conductive composite. The change in the electrical parameters with respect to the change in the volume of the material can be interpreted as the discontinuity produced in the framework connection which causes an increase in the resistance in the sensing material, thus serving as a chemiresistive device material. This can be investigated for CO2 and CH4 absorption, where the former gas physisorption causes a significant stepwise resistance increment but a very minor increment can be seen in the case of the latter one (Fig. 17b and c). Thus, this strategy of inducing a conductive carbon material inside a non-conductive but highly porous and ‘‘breathable’’ MOF can serve as assuring material for the purpose of enhancement in the gas sensing properties.
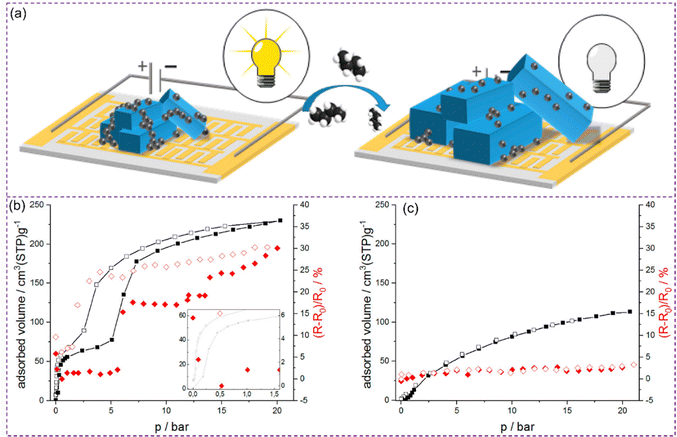 |
| Fig. 17 (a) Schematic illustration of chemiresistive sensing of a usual MOF/conductive carbon hybrid. (b) and (c) CO2 and CH4 interaction induced resistance variations in CB/film hybrid. (Red diamonds = relative resistance change in the material and black squares = physisorption data; Closed symbols = adsorption; open symbols = desorption). Reproduced with permission from ref. 249 Copyright 2019, American Chemical Society. | |
The availability of pores and cavity sizes of MOFs provides a way for the facile tunability of these pores by exploiting the dimensional scope of organic linkers or via incorporation of flexible polymers due to their vast size variations.250 When it comes to induce conductivity in non-conducting MOFs, conduction polymers (CPs) are a promising option. Conduction polymers such as Poly[3,4-(ethylenedioxy)thiophene] (PEDOT), Polyaniline (PANi), Polypyrrole (Ppy) and many more can be polymerized into the free spaces of porous materials such as 1D nanochannels,251,252 2D nanoslits253 and 3D porosity.254 CPs provide tunable conductivities, flexible and free standing material morphology255 whereas MOFs with high porosity and a well-defined geometrical framework can be used as host moieties for the incorporation of foreign materials, ranging from nanoparticles256 to macromolecules.257 Coalescence of MOFs with CPs has proven to be of great importance in the fields of supercapacitors,255 photoconduction251 and so on. For chemiresistive gas sensing, the use of conduction polymers has been there since 1980s when conductive polymers such as polythiophene films were used for the sensing of both oxidizing and reducing gases by analysing changes in the resistance of the polymer.258 So their hybrid assemblies with MOFs can act as symbiotic materials that can provide high-end individual properties-based performances. Keeping this in mind, exploiting the ultrahigh surface area of non-conducting 3D MOFs and the conducting nature of low surface area CPs, (pristine SBET = 2 m2 g−1) into the pores of the 3D MOF MIL-101(Cr) (pristine SBET ∼3100 m2 g−1), resulted into the formation of a highly porous and conductive composite (Fig. 18a).259 PEDOT, as a p-type semiconductor, is already known to identify the presence of NO2, which is an oxidizing gas, by means of chemiresistive analysis through intrinsic Fermi level modification.260 But the agglomeration of the polymeric structures of PEDOT impedes the accessible surface area to the interacting gases. Thus, the composite was found to give excellent performance for the same purpose compared with the pristine PEDOT polymer as the highly ordered MIL framework provides impartially distributed growth of the PEDOT polymer inside its pores. This uniform growth of polymer by nano structuring approach inside MOF's pores helps the resulted MOF/CP composite to interact with the target gas with high accessibility. Thus, the role of MOF's well-defined framework working as a template for the sensing CP material to improve its sensitivity can be seen as an advancement in developing MOFs as gas sensing porous materials. Flexibility and stretchability in polymers can provide high surface area as a template material for coating various foreign materials for applications in oil/water separation,261 wearable pressure sensors262 and so on. PDMS as a microporous template provides high surface area and microchannels for facile gas/air flow through them.263 Using this property of the microporous PDMS elastomer, Hwang et al. coated two high performance MOFs on PDMS – Cu-BTC as an excellent CO selective adsorbing material264 and MIL-160 for high uptake of humidity.265 These two separate MOF–PDMS composites act as high-performance gas filters and provide a highly selective and stable H2 gas sensor. Sensing of H2 gas provided by semiconducting metal oxides (SMOs) based chemiresistive gas sensors usually get affected by external factors such as the interaction of competing gases in addition to the target gas and humidity. These MOF–PDMS composites provide excellent filtration of these disturbances and thus greatly enhance the sensing performance of pristine SMOs. While covering the SMO with MOF–PDMS composite, Cu-atoms in Cu-BTC provides eminent CO adsorption which improves the H2/CO response selectivity in addition to the humidity adsorption by metal centres in MIL-160. This corroborated filtration performance of MOFs provides a promising stage for improving the sensing performance of a gas sensor by blocking the unwanted analytes and suppressing the effect of relative humidity.
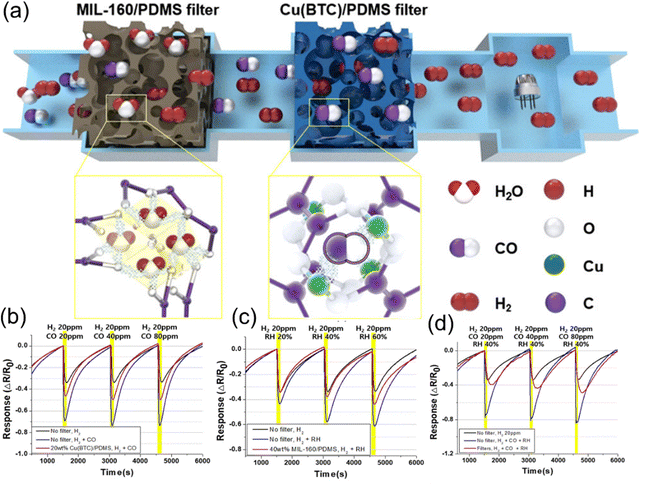 |
| Fig. 18 (a) Schematic illustrations of H2 gas adsorption with improved selectivity via structural hybridization of microporous MIL-160/PDMS and Cu-(BTC)/PDMS filters with SnO2 thin film. (b) Sensor response towards H2 gas in a mixture of H2 and 20/40/80 ppm CO gas with 20 wt%/without microporous Cu-(BTC)/PDMS filter. (c) Sensor response towards H2 gas and a mixture of H2 and 20/40/60 RH% with 40 wt%/without microporous MIL-160/PDMS filter. (d) Sensor response towards H2 gas RH 40% air, and 20/40/60 ppm CO gas with/without microporous Cu(BTC)/PDMS and MIL-160/PDMS filters. Reproduced with permission from ref. 264 Copyright 2020, American Chemical Society. | |
The hybrid's enhanced sensing performances can be seen in Fig. 18b–d. Dynamic response curves and sensing responses of H2 sensing in solely as well as in various combinations with CO (Fig. 17b) with and without the PDMS–Cu-BTC filter, with various RH% (Fig. 17d) with and without the MIL-160/PDMS filter and finally sensing H2 from a mixture of H2 + CO + RH and combining both the filters reveal the high selectivity towards the H2 gas as well as an improved sensing response as compared with the pristine SMO sensors.
Graphene being one of the most highly explored 2D conducting materials can be merged with insulating MOFs to form composites with improved conductivities.266–269 Additionally, the merging of functionalized graphene with MOFs also invokes optimal interacting properties in the thus formed MOF/Graphene composite.270–281 These inculcations are suitable enough for improving chemiresistive gas sensing properties owing to the improved conductivity and the presence of active interacting sites. Consequently, our group worked in this direction by fabricating a functionalized graphene-MOF composite, and we reported a covalently assembled hybrid constructed from graphene acid (GA) and amine functionalized UiO-66-NH2 for chemiresistive CO2 sensing (Fig. 19a).221 Having hierarchical porosity, and the micropores are located at the octahedral UiO-66-NH2 nanocrystals and the mesopores are built up through the stacking of GA through the MOF nanocrystals. The hierarchical architecture facilitates gas diffusion through the material, hence providing faster access to the interaction sites for the analyte and the interconnected conductive network ensures quick readout of the signal. The faster access to the amine interaction sites ensures faster response and recovery times for CO2 sensing (tres = 18.5 s, trec = 18.7 s). Consequently, the introduction of amine functional groups inside the porous pathway of GA@UiO-66-NH2 provides a high response of 8.6% (Fig. 19b–d) compared to the pristine GA which shows a negligible response to CO2 under the same sensing conditions. In comparison, graphene has a low electrical resistivity (∼10−6 ohms) and high carrier electron mobility. Still, the lack of specific and strong interaction sites for the adsorption of gaseous analytes, renders it unusable for chemiresistive sensing in its pristine form. These results are clarified by temperature-dependent in situ Raman spectroscopy during the exposure of the material to CO2. While up to 125 °C, the softening takes place which can be observed further up to 200 °C, suggesting the gradual uptake of CO2 into the porous GA-UiO-66-NH2 network while strongly interacting with the amide bonds. A further increase in the temperature causes an increase in the frequencies of both –CO–NH linkages and Fermi modes of CO2 suggesting a very weak interaction of CO2 with the sensing material.
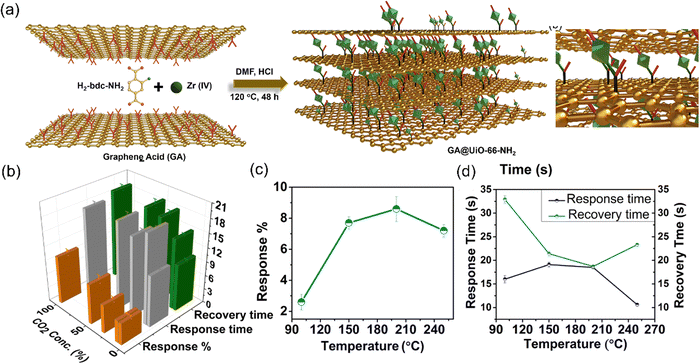 |
| Fig. 19 (a) Schematic illustration of the synthesis of GA@UiO-66-NH2 and GA@UiO-66-NH2 porous network with amide linkage. (b) Gas sensing performance of GA@UiO-66-NH2 (from 100% to 5% CO2) at an operating temperature of 200 °C. Gas sensing characteristics (% response, response time and recovery time) of the hybrid at different concentrations of CO2; Temperature effect on the sensing characteristics of GA@UiO-66-NH2 (c) on the response % and (d) response and recovery times. Reproduced with permission from ref. 221 Copyright 2021 Royal Society of Chemistry. | |
5.2. Covalent-organic frameworks as chemiresistive gas sensors
Covalent-organic frameworks (COFs) have been employed for sensing purposes due to their highly ordered structure, functional group versatility, and high thermal and chemical stability. They have been primarily utilized for explosive sensing, metal ion sensing, humidity sensing, biosensing, gas sensing, and more.312 However, covalent-organic frameworks (COFs) are in their nascent stage regarding the application of chemiresistive gas sensing.313 One major reason for this is the challenging task of bringing intrinsic electronic conductivity in them in addition to the robust framework that is the fundamental necessity for a successful gas sensing material with requisite results that can outshine or at least compete with the already available sensing materials’ performance (Table 2).314–321
Table 2 COF and its hybrids for chemiresistive gas sensors
S. no. |
Material |
VOCs |
Sensing mechanism |
Response (%) |
LOD (ppm) |
t
res/trec (s) |
Ref. |
1 |
COF-TXDBA |
Humidity |
Lewis acid–base interaction |
2.3 |
|
37 |
322
|
42 |
2 |
COF-DC-8 |
NH3 |
CT-DA |
39 |
0.07 |
|
323
|
H2S |
62 |
0.204 |
NO |
3939 |
0.005 |
NO2 |
6338 |
0.016 |
3 |
M-TPCOF |
NO2 |
DA |
2713 (Co) |
0.0068 |
318 (Co) |
329
|
2056 (Cu) |
510 (Cu) |
690 (Cu) |
4 |
COF-5 |
Humidity |
|
90 |
|
26/16 |
325
|
5 |
COF@PANI |
NH3 |
|
7 × 105 |
|
|
5
|
COF@Ppy |
6 |
TiO2@COF-316 |
NO2 |
DA |
572 (NA) |
1.41 |
8–1.1 |
330
|
7 |
Pd@TpPa-SO3H COF |
H2 |
DA |
60.1 ± 10 |
0.2% |
5.3–3.1 |
332
|
5.2.1. Pristine COFs.
Despite their poor conductivity, some researchers have actively pursued using COFs as chemiresistive gas sensors, aiming to compare them directly with other sensing materials due to their promising porous and versatile functionality. Singh et al. reported a Truxene-based COF for humidity sensing.322 The high surface area and ordered structural porosity along with the boronate ester groups in the COF resulted into an excellent interactive material for humidity. The stability of this COF in a high humid environment is might be due to its structural robustness. The conductivity required for the chemiresistiveness comes from the planar COF sheets and the multilayer formation of water vapours at higher RH% that acts as a proton conduction medium throughout the sensing COF material. Thus, the sensing can be attributed much to the humid medium rather than the intrinsic COF conductivity. COFs most often are very low (∼10−3 to 10−10 S cm−1) or non-conductive materials. To tackle this problem of conductivity requirements, many researchers have worked to produce conductive COFs by modulating their spatial stacking, electronic band gap and tuning framework backbone functionalities. Meng et al. have reported a pristine COF, named COF-DC-8, with an intrinsic bulk electronic conductivity of 2.51 × 10−3 S m−1 that was the highest reported for a COF at that time323 (presently, Huang et al. achieved 12.7 S m−1 for a piperazine-linked COF).324 The high conductivity can be attributed to full π–π conjugation spread over the entire intrinsic 2D framework and the DFT-suggested out-of-plane charge transferring due to the anisotropic band structure (Fig. 20a and b) (synthesized by condensation of two planar, rigid and themselves fully conjugated metal-phthalocyanine and pyrene core monomers). This COF showed chemiresistive nature towards various gases with excellent results for NH3, NO2, H2S and NO gases (Fig. 20c–f). The metal-analyte interaction was exploited in this case for chemiresistiveness since metal doped phthalocyanine rings have improved electronic conductivities in contrast with the pristine phthalocyanine rings. The charge transfer interaction of oxidizing (NO and NO2) and reducing (H2S and NH3) gases with the Ni-centre corresponds to the mechanistic performance of the sensing COF material. Ni containing free valence electrons has the potential to interact weakly via non-covalent interactions as well as stronger covalent interactions too with the analyte gases. This results into a significant change in the electronic parameters supporting the highly sensitive chemiresistive nature of the COF towards the targeted gases which might also be a reason for the low and ultralow ppb level detection limits for this material. COFs are usually formed as a powdered material by traditional solvothermal processes but for convenient practical applications, robust materials play the required role. For an efficient, practical gas sensor, the sensing COF material should have such properties so that the sensor can work for longevity with the same performance. Fathoming this need for the COF-based chemiresistive gas sensors, Mei et al. fabricated a flexible and robust COF-5 film on a polyimide (PI) substrate and employed it for humidity sensing.325
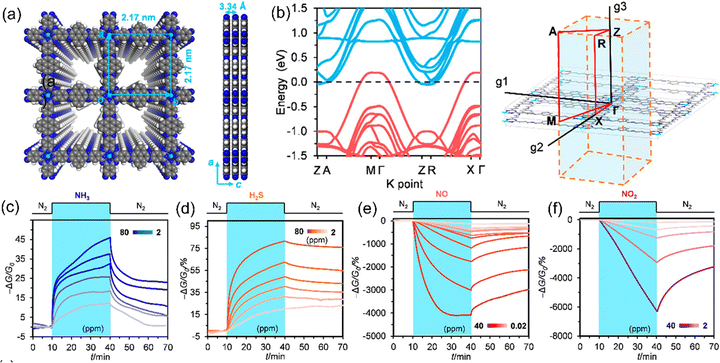 |
| Fig. 20 (a) Schematic representation of a phthalocyanine-based 2D conducting COF (COF-DC-8). (b) DFT calculated anisotropic electronic band structure and the corresponding first Brillouin zone. Response curves of COF-DC-8 when interacting with (c) NH3, (d) H2S, (e) NO, (f) NO2 gases. Reproduced with permission from ref. 323 Copyright 2019, American Chemical Society. | |
The synthesis of COF films was approached via a vapour-assisted method. This method provides a sophisticated way to control the film thickness of the COF material by changing the monomer concentration in the solution. After achieving the required thickness of the COF-5 film and sensor fabrication, the device was tested from 11–98% RH range which showed a remarkable linear response between log of resistance and relative humidity. The COF-5 film showed better performance in gas sensing than the COF-5 powder. The uniform morphology and uninterrupted conductive contact due to the 2D film nature proved to be superior to the discrete powder in terms of electrical sensing requirements.
5.2.2. COF hybrids.
Synthesizing intrinsic COFs with high electrical conductivities is one tremendous challenge that limits their application as a practically usable chemiresistive material. Polymers, on the other hand, such as polyaniline326 and polypyrrole,327 have high intrinsic conductivities – about 600 S cm−1 and 380 S cm−1, respectively. Conducting polymers are known for their composition into various materials such as MOFs, COFs, Metal oxides, Carbon materials etc. to either induce or increase their electrical conductivities of the latter. Their infusion with these materials has been explored extensively in the field of chemiresistive gas sensing. This strategy for COF-Conductive polymer composites for chemiresistive gas sensing was effected insightfully by Sahiner and group in 2019, by using a mesoporous COF developed from condensation between melamine and dibromoalkane monomeric units, to create in situ formation conditions for conducting polymers such as polypyrrole (PPy) and polyaniline (PANi) which resulted into a semi-interpenetrated network of COF and conductive polymers.5 This intrinsic growth of conducting polymers inside the COF led to an increase in the electrical conductivity by 3-million folds for COF-PANi and 0.5-million folds for COF-PPy networks, respectively. This high conductivity was utilized for gas sensing (NH3 & HCl vapours) as well as dye sensing (Methyl Orange and Methyl Blue) purpose in a chemiconductive manner. Both pristine COF and COF-Polymer composites were tested for gas and dye sensing. Towards NH3 and HCl vapours, pristine COF showed an increase in conductance while both COF-Polymer composites showed an opposite effect. This could primarily be due to the protonation of amine groups which resulted into the formation of positively charged ammonium ions, thus causing an increase in the ionic conductivity of the pristine COF material. The opposite effect in the case of composites could be explained by the fact that the reducing gases such as ammonia cause de-doping of PANi328 by deprotonating the bridging amino groups and over-oxidation of chlorine ions by PPy. Materials in their bulk phase cannot able to offer the entire surface area for interaction with the analyte particles. In contrast, 2D layered materials or bulk materials after exfoliation reveal their maximum surface area and thus can interact with the lower concentrations of the analyte particles. In this regard, Liu et al. developed porphyrin-based 2D COF nanosheets (M-TPCOF, M = Co, Cu) where porphyrin moieties can be used to coordinate metal active sites as a result of their post-metallization (Fig. 21a).329
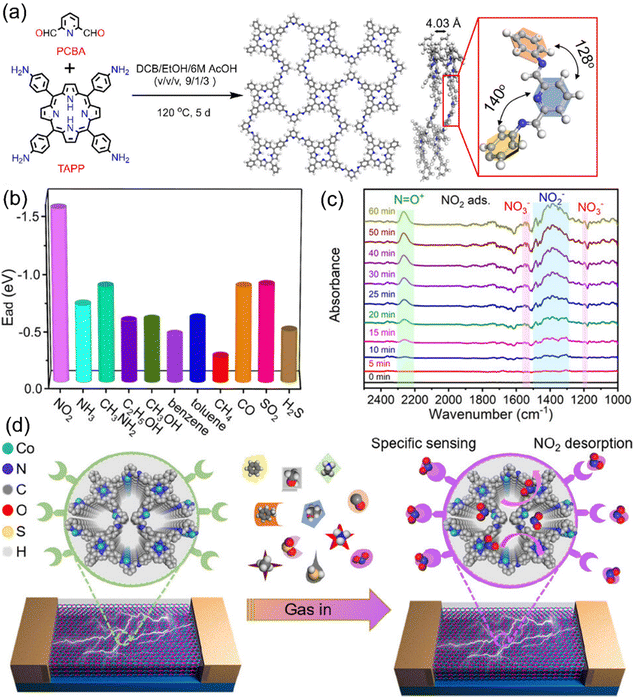 |
| Fig. 21 (a)–(d) Synthesis, structural characterization and sensing performance of M-TPCOF (M = Co & Cu) towards NO2 gas. (a) Synthesis and structural features of H2-TPCOF before metalation. (b) Adsorption energy DFT based comparison of interaction of various gases with Co-TPCOF. (c) In situ DRIFT spectra of Co-TPCOF during NO2 adsorption. (d) Schematic demonstration of specific chemiresistive sensing of NO2 by M-TPCOF. Reproduced with permission from ref. 329 Copyright 2022, Wiley-VCHGmbH. | |
The easily formed stable nanosheets after exfoliation are a result of the non-linear linker (2,6-pyridinedicarboxaldehyde) used in synthesizing this COF which hinders the π–π stacking of extended porphyrin layers due to the strong deviation from the planar COF layers. Thus, it produced high surface area nanosheets with exposed metal active sites which increased the extent of NO2 gas interaction with the metal active sites and thus resulted in the excellent sensitivity. The highly specific affinity towards the NO2 gas is proposed to the presence of electron-rich metal centres (Co & Cu) due to the highly electron withdrawing nature of acidic NO2 gas, which eventually produces excellent selectivity performance as can be figured by the adsorption studies of various analytes (Fig. 21b). DRIFT IR spectroscopy has proven to be a useful technique for analysing gas-host interactions by carefully observing the changes in the intensity of interaction signals (Fig. 21c). The broad peak at 1500–1290 cm−1 corresponds to the N
O stretching of the monodentate nitrite or the asymmetric stretching NO2 vibration of the nitro group. The peak at 1398–1353 cm−1 implies to Co–NO2 species, indicating Co as the active site for NO2. A significantly wider peak observed at 2310–2230 cm−1 might be due to the N
O stretching in the NO+ ions. With an ultra-low LOD of 6.8 ppb, the device showed a fast response and strong binding of NO2 with the metal centre of M-TPCOF nanosheets. This level of sensing performance is a result of few-layered structures of the materials, which provide fast diffusion access pathways to the gases. Thus, distortion-based exfoliation of metalloporphyrin bulk COFs to COF nanosheets provides scope for the exfoliated material-based sensing performance improvements. COFs can attune surface properties effectively due to their versatile nature towards functional modifications. They can be used for the easy tuning of redox properties of the materials based on their electronic interactions with them.
This nature of COFs was beautifully explored by Chen et al. while working with the MOS-based chemiresistive gas sensors. The TiO2 nanowire array was coated with COF-316 (a dioxin-linked COF), which resulted into the reversing of the reductive to oxidative nature of TiO2 (Fig. 22a).330 This work represents the benefits of 2D porous materials for enhancing the sensing properties of a pre-existing metal-oxide gas sensor. The high porosity, facile redox interchange and abundant active sites offered by the COF-sheath over TiO2 nanowires for NO2 gas sensing can be possible due to these intrinsic properties of porous COFs. Redox reversing being the striking advantage of this COF/TiO2 heterojunction can be explained by the Z-scheme of charge separation.331 Since the band gaps in COF-316 and TiO2 overlap, the electrons excited from the valence band to the conduction band in TiO2 relax by occupying the valesnce band in COF-316 under the influence of an intrinsically evolved electric field between COF/TiO2 heterojunction. This event results into the concealment of oxidative sites on TiO2 and revelation of the reductive sites on the outer COF-sheath for the sensing reversal from the reductive gas sensing such as EtOH towards the oxidizing gas sensing such as NO2 (Fig. 22b). Due to the intrinsic hydrophobic nature of the TiO2/COF-316 hybrid (contact angle = 108.3°) in contrast with the hydrophilic nature of the bare TiO2 (contact angle = 7.1°), the sensing device integrated with this hybrid leads to undisturbed sensing performance of the device with consistent results of the NO2 sensing (Fig. 22c).
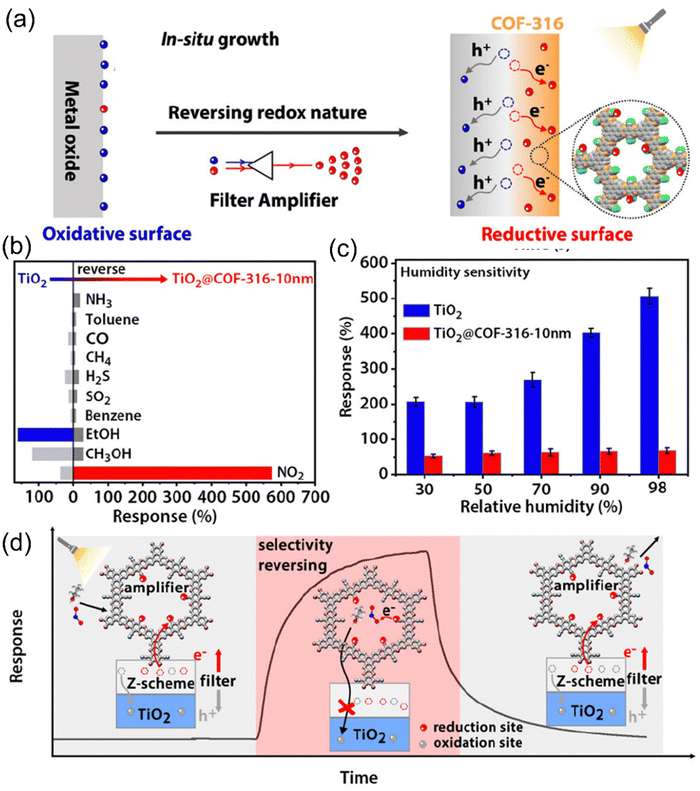 |
| Fig. 22 (a) Schematic representation of COF-316 structure and ‘filter amplifier’ and ‘redox reversal’ nature of TiO2/COF-316 hybrid. (b) Sensing response of TiO2/COF-316 hybrid towards NO2 compared with other gases. (c) Response analysis of bare TiO2 and TiO2/COF-316 hybrid towards RH%. (d) Chemiresistive response of NO2 interaction with TiO2/COF-316 hybrid in the presence of light and corresponding illustrated sensing mechanism. Reproduced with permission from ref. 330 Copyright 2023, American Chemical Society. | |
The NO2 sensing mechanism of the TiO2/COF-316 hybrid reveals the reversal of the sensing of oxidizing NO2 gas (Fig. 22d). This hybrid promotes the reductive sensing mechanism for NO2 gas which otherwise produces an oxidative sensing mechanism for pristine TiO2-based sensors. Hydrogen gas detection is of crucial concern since it is a highly flammable gas and it provides one of the cleanest fuels, making it a precious entity. Various hydrogen chemiresistors are already in function including metal based (Pd, Pt) materials, transition metal dichalcogenides (TMDs) and graphene-based materials. Although graphene-based materials are good in terms of conductivity and stability, but they have poor interaction with gases due to the lack of any functionality. Thus, inducing functionality or adding other materials such as metal nanoparticles (Pd/Pt) improves interaction of graphene materials with hydrogen gas molecules. Eventually, Krishanveni et al. synthesized Pd-doped imine-COF which offers a stable hybrid structure and highly efficient hydrogen sensing performance.332 The presence of sulphonic functional groups in the constituent COF monomer effectively stabilizes the Pd-nanoparticles. The interaction of H2 molecules with the Pd-decorated COF particles was excellently improved as compared to that of bulk COF by acid exfoliating the bulk COF material to COF-nanosheets since individual Pd-doped COF nanosheets provide maximum surface area for hydrogen molecules to get interacted with the exfoliated nanosheets. This provides highly approachable Pd nanoparticles for hydrogen spillover, i.e., dissociation of H2 particles onto the Pd nanoparticles and the resulting formation of PdHx species. This causes electron enrichment of the material and a subsequent decrease in the resistance which can be termed as chemiresistive change in the material.
5.3. Porous conjugated polymers as chemiresistive gas sensors
Porous conjugated polymers or conjugated porous polymers (CPPs) are a class of porous materials that are realized by the polymerization of monomeric units via a strong bonding between them.333–335 They are different from other porous materials such as MOFs and COFs as they do not rely on the crystallinity of the materials. Thus, they can be prepared with robust conditions keeping in mind only the stability of the product and no special conditions for achieving crystallinity. This makes CPPs a promising choice for chemiresistive gas sensing materials. Although they are very stable materials and can be potentially used in harsh environments, not much work in the direction of chemiresistive gas sensing is done (Table 3). In this regard, Wisser et al. performed a polymerisation of 4,4-diacetyl-2,2-diamino-biphenyl and 1,4-diacetylbenzene resulting into microporous polymers (DUT-92(NH2) and DUT-92(NO2)).336 These polymeric materials were mixed with PTFE binder and carbon black to form flexible sensor films. The porous structure of the polymer causes a huge uptake of the target VOCs which causes expansion of the material, resulting into an increase in the tunnelling distance between the embedded particles of conductive carbon black and thus a simultaneous increase in the resistivity of the material. This mechanism is in contrast with the change in the resistivity in non-porous polymeric materials. A high surface area of about 680 m2 g−1 provides effective interaction with VOCs which leads to the LOD values of <1 ppm. The swelling behaviour is observed to be effective for effective sensing performances of DUT-family polymers towards various VOCs.
Table 3 Porous organic polymers based chemiresistive gas sensors
S. no. |
Material |
VOCs |
Sensing mechanism |
Response (%) |
LOD (ppm) |
t
res–trec (s) |
Ref. |
1 |
T-2DP |
NO2 |
CT |
452.6 |
0.0002 |
35–47, 56–140 |
337
|
2 |
Heptazine-based COP |
NH3 |
DA |
70 |
1 |
65–9 |
340
|
3 |
Hydrazide-based COP |
H2S |
Proton conduction |
51 |
|
9–12 |
338
|
4 |
CONs |
NO2 |
CT |
73.7 |
0.00024 |
|
339
|
5 |
P2O5-CTF |
NH3 |
CT |
−8 |
|
54–200 |
341
|
5.3.1. Pristine porous organic polymers as chemiresistive gas sensors.
Porous organic polymers represent the polymeric porous materials consisting of only organic constituting parts. The class consists of – conjugated microporous polymers (CMPs), porous aromatic frameworks (PAFs), hyper cross-linked polymers (HCPs), polymers of intrinsic-microporosity (PIMs), covalent organic polymers (COPs) and covalent triazine frameworks (CTFs). Covalent organic frameworks (COFs) are also a kind of POP material but their high superiority over the other POPs in terms of crystallinity and potential conductive nature makes them to be discussed critically as a separate section. Owing to their porous nature, we will be interested in POPs to check their potential for applications which involve the interaction of analyte molecules while passing through the porous passage, like gas sensing. Not all POPs have been explored for the chemiresistive gas sensing application because of the need for conductivity in the material, whereas most POPs, except COFs and CTFs, are either non-conductive or become conductive in hybrid forms.
Thus, we would be highlighting here some important landmarks that prove the potential of these materials in chemiresistivity based sensing applications. Since CTFs are conductive in nature, they remain as the protagonist in the current scenario. Yang et al. synthesized a 2D organic polymer derived from a covalent triazine framework (CTFs), shortened as T-2DP (Fig. 23a).337 The presence of a porous 2D pathway provides the facile gas transport and the presence of the high-density imine functional groups provides optimum adsorption/desorption which leads to highly improved sensing performances compared with the CTF-based sensor. This T-2DP-based sensor thus produces superfast response and recovery times for NO2 sensing. Response/recovery times (35–47 s) are fast due to the presence of uniformly distributed interacting sites (–C
N– sites in this material) (Fig. 23b). The distribution of interacting sites is usually out-of-plane in the case of 2D inorganic materials such as MXenes, Graphene, rGO and so on. In the case of the present material, the interacting sites are in the 2D plane, which contributes to the conduction of electrons in this material. Thus, the interaction of target gas molecules (NO2) with these in-plane interacting sites produces highly sensitive output signals (Fig. 23c) with an excellent sensitivity of 452.6 ppm−1. The performance comparison with the corresponding bulk CTF based sensing material gives similar sensing behaviour with diminished sensitivity because of the slow diffusion of the analyte gas into the bulky CTF assembly. Due to the excellent recovery property of T-2DP, it produces highly reproducible response results with a mere 4% perturbation in response values over a series of 15 cycles (Fig. 23d). Comparative chemiresistive studies for CTF, RGO and MXene indicate that T-2DP is superior to all of them for faster response/recovery values by several magnitudes (Fig. 23e–h).
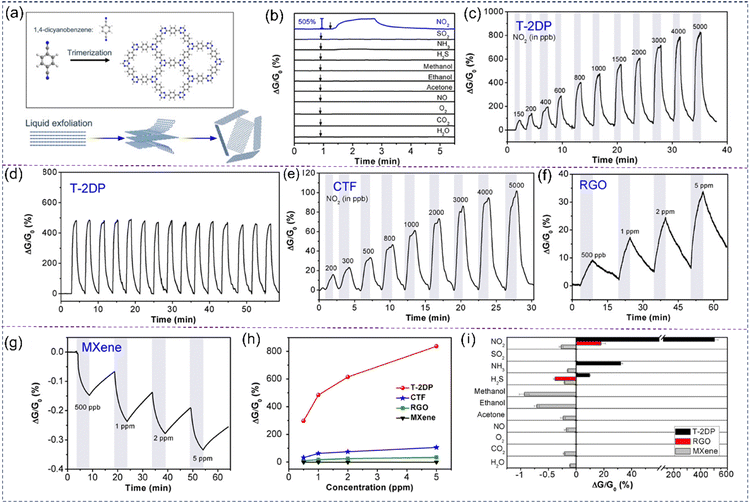 |
| Fig. 23 (a) Diagrammatic illustration of a triazine-based 2D polymer. (b) Exclusive chemiresistive response towards NO2 gas while comparing with other inorganic and organic analytes. (c) Response curve of the T-2DP sensor towards NO2 sensing at ppb-level. (d) Chemiresistive response of T-2DP towards NO2 (1 ppm) feed. Dynamic sensing response of (e) pristine CTF to various NO2 concentrations (5 ppm to 200 ppb), (f) RGO to different NO2 levels (5 ppm to 500 ppb). (g) MXene to different NO2 levels (5 ppm to 500 ppb). (h) Response vs. concentration curves for T-2DP compared to CTF, RGO, and MXene. (i) Response of MXene, RGO and T-2DP to various target analytes. Reproduced with permission from ref. 337 Copyright 2020, American Chemical Society. | |
Non-crystalline POPs usually show poor electronic conductivity due to the absence of an electronic conduction channel in contrast to what is usually found in, say, 2D conductive COFs. Maiti et al. synthesized a trialdehydebenzene and phenyl-dihydrazine combined hydrazide linkage based COP for the room temperature sensing of H2S gas.338 Amine based macromolecules show conductivity through proton-conduction mechanism. This sensing material also showed fast sensing behaviour due to its high sensitivity towards acidic gases. H2S being an acidic gas, when interacts with this sensing material, produces an abrupt decrease in the proton conductivity of the material which is seen as fast response time (9 s) for 200 ppm of gas input. Thus, non-electronic conductive materials can be used for gas sensing by exploiting their proton conductivity, similar to what exists in amine-based covalent organic polymers. Metal-oxide semiconductors give excellent sensitive performance in the case of gas sensing but their selectivity and sensitivity are demolished to a great extent when they are kept under humid conditions because of the active site's deactivation by the humidity. Organic-based materials provide a superior alternative for chemiresistive gas sensing jobs when they are stable under humid conditions, with accurate and reliable values. Taking forward the concept, Ko et al. fabricated a NO2 sensing device, powered by covalent organic nanosheets which are two-dimensional semiconductive in nature, abbreviated CON-10.339 The 2D nanosheet morphology of this material endows it with a hydrophobic nature with a water contact angle of 135.4° along with the thermal stability of this material up to 320 °C. The exfoliated nature of CON-10 nanosheets provides a highly exposed surface area for the analyte gas molecules to interact with the π-electrons moving on these nanosheets surface analogous to the graphene electronic conduction behaviour. This can be the reason for the lower concentration detection limit of 2.242 ppb. The hydrophobic behaviour of CON-10 benefits the material to detect NO2 gas in the ambient atmosphere and weather. Thus, the material is proposed to be suitable for trace-level detection of NO2. The inherent hydrophobicity in this material is supposed to be the result of uniformly distributed covalent bonding and the presence of N–S bonds.
The hydrophobic nature of the sensing material is an important requirement for the real-time application of a chemiresistive gas sensor since it protects the material from the baseline resistance change when repeated cycles of the gas sensing are performed as is being observed in the present case. Comparing with the SnO2 NW-based gas sensor, the damping effect of relative humidity on the baseline resistance value of the sensing material can be observed as highly impactful. High selectivity towards the NO2 gas sensing can be observed in this material because of the hydrophobic nature of the CON nanosheets as they prevent the interaction of other gases with the sensing material, thus causing a negligible change in the conductivity values of the sensing material. CONs display excellent sensing performance at the real-life application value and thus prove the extensive morphological dependence of gas sensing performance.
Similar to other porous materials, hydrogen-bonded organic frameworks (HOFs) consist of the polymerization of functional organic moieties through hydrogen bonding. They exhibit high permanent porosity, facile synthesis, and show promising applications such as molecular/gas separation, optical and biomedical applications.342 Wang et al. synthesized a porphyrin-based hydrogen-bonded organic framework (HOF) for NO2 sensing, leveraging abundant amine groups that contribute to excellent selectivity and an ultrafast response at room temperature.343–345 The one-dimensional channels of HOF, the sensor demonstrated response and recovery times of 17.6 s and 15.4 s, respectively. The abundance of amine groups in the sensor results in n-type semiconductor behavior, leading to an ultrafast response to acidic NO2 gas molecules. Similarly, Lee et al. demonstrated the significance of HOFs in the separation of noble gases, such as isolating xenon (Xe) from an Xe/Kr mixture.346 Xenon (Xe) gas-based devices find diverse applications, ranging from lighting, laser technology, space exploration to medical devices. Nevertheless, monitoring selective Xe gas and achieving sensitive separation using chemiresistive gas sensing will be an interesting study in the field of sensors.
6. Conclusion and future perspective
The field of chemiresistive gas sensing has rapidly gained prominence due to its remarkable responsiveness to various gases, driven by swift electronic changes in the sensing material. Achieving sensitivity in gas sensing demands an ideal interplay between the gas sensing material and target gases, a substantial surface area providing accessible interaction sites, and rapid responses to analytes. Porous materials have emerged as highly promising candidates in the realm of chemiresistive gas sensing, as they effectively meet these essential requirements for modern and efficient gas sensing materials.
This review offers an up-to-date overview of advancements in chemiresistive gas sensing, covering fundamental principles, sensing parameters, and the various materials employed. It places particular emphasis on porous materials, including metal–organic frameworks (MOFs) and their hybrids, covalent organic frameworks (COFs) and their hybrids, graphene-based materials, and porous conjugated polymers. The future of chemiresistive gas sensors holds exciting prospects driven by advancements in materials, fabrication techniques, and sensor designs. Advanced materials, such as 2D wonders like graphene and transition metal dichalcogenides, hybrid nanomaterials, and functionalized polymers are at the forefront.146,347–354 These materials offer exceptional sensitivity and selectivity, promising the detection of a broader spectrum of gases, including elusive volatile organic compounds and industrial hazards. The world of nanotechnology continues to unfold, with nanowires, quantum dots, and hybrid nanomaterials driving the development of highly efficient sensors capable of swift responses and astonishingly low detection thresholds. Additionally, the integration of machine learning and data analytics promises to make gas sensors smarter and more responsive. These sensors will not only detect gases but also interpret data in real-time, recognizing patterns and identifying multiple gases simultaneously. Such intelligent sensors are set to revolutionize environmental monitoring, healthcare, and safety systems across various domains (Fig. 24). Furthermore, we are on the brink of witnessing gas sensors embedded in wearable devices and seamlessly integrated into the Internet of Things (IoT).355–373
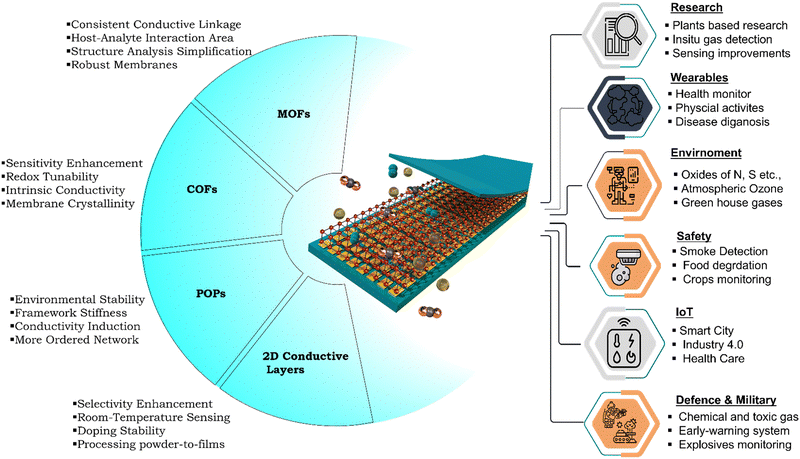 |
| Fig. 24 Schematic illustration of current summary, future perspectives of advanced porous materials based CGS including critical challenges. | |
This transformation enables continuous monitoring of air quality, personal health, and safety in diverse environments, from urban landscapes to remote industrial sites. Flexible and printable sensors are also emerging, offering a cost-effective, disposable solution for various applications. These sensors are used in food packaging, healthcare, and beyond. Environmental applications remain a key focus, with gas sensors leading the charge in monitoring pollution, greenhouse gases, and indoor air quality. Healthcare is another area where gas sensors are poised to make significant contributions. From disease diagnosis through breath analysis to non-invasive health assessments via skin emissions, these sensors are poised to enable early disease detection and personalized healthcare. Industrial safety, particularly in the energy sector, is set to benefit as gas sensors play pivotal roles in detecting hazardous gases. Their deployment ensures safer oil and gas exploration and production. As the world turns its gaze towards environmental sustainability, gas sensors will embrace eco-friendly materials and sustainable manufacturing processes. This shift aligns with the growing commitment to environmental responsibility. Finally, global collaborations are catalysing these advancements. Scientists, engineers, and industries worldwide are uniting, pooling knowledge, and resources to accelerate the development and commercialization of cutting-edge sensor technologies. In conclusion, the future of gas sensors is radiant, holding promise for improving air quality, safety, healthcare, and environmental stewardship on a global scale. In this perspective, porous materials provide almost all the basic requirements as an effective chemiresistive gas sensing material. They provide high surface area, functional group versatility, an extended structural framework with electronic conjugation and many more properties for optimal host–analyte interaction and the aftermath results consisting of reliable and accurate sensing performance and data. Since there is always a scope for improvement in any work, this field can also be taken further towards a more powerful gas sensing level by considering some key future perspective points.
1. MOFs have already proven valuable in chemiresistive gas sensing due to their outstanding surface area and tuneable functionality, facilitating close interaction with target gases. Additionally, certain 2D conductive MOFs like M-HHTP, M-HITP, and M-TCPP exhibit conductivity, offering electronic conduction for chemiresistive behaviour. To enhance their performance, there is room for improving MOFs by transitioning toward a more crystalline nature and developing high-surface-morphology frameworks. Controlling the shape of MOF-based nanoparticles can also be optimized to maximize the accessible surface area for analyte gases. MOFs are primarily used in powder form, posing processing challenges. Considering the actual material for gas sensing purposes, thin-films and membranes like material are of considerable importance. Therefore, it is essential to focus on developing facile synthetic methods for MOF membranes or easily processable solutions. Exploration into MOF combinations with materials like CNTs, metal oxides, and conducting polymers has shown promise. Further investigations into highly conductive materials like MXenes hold potential, although stability improvements, especially under ambient conditions, are necessary.
2. COFs face challenges due to their instability in humid or ambient conditions and limited conductivity. To make them viable for gas sensing, more robust and conductive COFs are needed. COFs offer adaptable frameworks and versatile functionality, crucial for ideal target gas sensing. They are particularly suitable for gas sensing in living organisms, avoiding concerns associated with metal-based materials like MOS and MOFs. While examples using other materials like MOS and MOFs already exist, particularly for VOCs sensing in humans and plants, research involving COFs in this context remains unexplored. Also, the requirement of flexible and robust COF materials is a compelling need for a device level development of this work. Some COF membranes and thin-films are already being explored,374–376 but no reports on their pristine membranes or films were found to the best of our knowledge. Further, investigation in this direction can be done by developing new universal methods for processing COFs to thin-films and membranes.
3. Graphene, a 2D conductive material, has been successfully employed in chemiresistive gas sensing applications. Graphene, primarily in its 2D monolayer form, serves as an excellent chemiresistive material owing to its superior electronic properties compared to multilayer graphene. Consequently, graphene and its derivatives, as well as hybrids, have emerged as top-notch materials for room-temperature chemiresistive gas sensing. However, despite their physical and chemical robustness, graphene-based materials face challenges such as limited selectivity and sensitivity due to their chemical inertness, which hampers host-gas analyte interactions. To address this issue, efforts have been made to introduce metal/metal oxide sites and related functionalities into pristine graphene, resulting in improved gas sensing performance. Further enhancements can be achieved by developing these hybrids with more intriguing morphologies and additional functionalities, building upon the success of materials like GO, rGO, and G-COOH in gas sensing. Incorporating dopants like B, N, or -SH becomes crucial for fine-tuning the redox properties and electronic structure, including band gaps and Schottky barriers, to enable precise sensing of interacting gases. Synthesis of graphene-based devices is still restricted to monolayers or thin-layer graphene derivatives which are difficult to achieve as it require critical precision and sophisticated tools. Chemical exfoliation methods to develop large monolayers need to be explored further.
4. Porous organic polymers including amorphous porous materials like porous carbon black, ionic liquids, hyper-cross-linked polymers (HCPs), and PIMs offer notable advantages in terms of stability, ease of synthesis, and functionality. Despite these merits, their complex microstructure and lack of long-range order pose challenges, particularly regarding electronic conductivity. To overcome this hurdle, inducing conductivity through space becomes a viable strategy, addressing the need for extended conjugation and through-bond or through-space conduction. This can be accomplished by meticulously controlling the orientation of individual moieties, allowing their empty orbitals or charges to traverse the entire amorphous framework and enhancing the utility of these materials in chemiresistive gas sensing. Powders are usually not processable if they are robust in nature. Thus, solution processable POPs or membranes are the need of the hour. To synthesize these membranes or film methods like doctor blade, Langmuir–Blodgett nanostructured film formation kind of methods are required to be more in use for producing effective chemiresistive films.
Conflicts of interest
There are no conflicts of interest to declare.
Acknowledgements
A. S. and S. E. equally contributed to this work. K. J. R. acknowledges support from the Indian Institute of Technology Jammu for providing UGC-DAE CSR project CRS/2022-23/01/708, SERB CRG/2023/004685,CSIR-HRDG 01/3101/23/EMR-II and DST/INT/DAAD/P-06/2023. We acknowledge the support of the project TECHSCALE (no. CZ.02.01.01/00/22_008/0004587) financed from the ERDF and ESF. This work was supported by the Ministry of Education, Youth and Sports of the Czech Republic through the e-INFRA CZ (ID:90254). This work was supported by the Materials and Components Technology Development Program of Ministry of Trade, Industry and Energy (MOTIE)/Korea Institute for Industrial Economics and Trade (KIET) (10080527). This work was also supported by the Ministry of Education of the Republic of Korea and the National Research Foundation of Korea (NRF-2022S1A5C2A03093218). The financial support of the European Union under the REFRESH – Research Excellence For Region Sustainability and High-tech Industries project number CZ.10.03.01/00/22_003/0000048 via the Operational Programme Just Transition is also acknowledged
References
- H. François, R. Samacoïts, D. N. Bird, J. Köberl, F. Prettenthaler and S. Morin, Nat. Clim. Change, 2023, 13, 935–942 CrossRef
.
- I. Manisalidis, E. Stavropoulou, A. Stavropoulos and E. Bezirtzoglou, Front. Public Health, 2020, 8 Search PubMed
.
- H. Zhang, Z. Zhang, Z. Li, H. Han, W. Song and J. Yi, Nat. Commun., 2023, 14, 3495 CrossRef CAS PubMed
.
- S. Dhall, B. R. Mehta, A. K. Tyagi and K. Sood, Sens. Int., 2021, 2, 100116 CrossRef
.
- N. Sahiner and S. Demirci, J. Porous Mater., 2019, 26, 481–492 CrossRef CAS
.
- D. J. Buckley, N. C. Black, E. G. Castanon, C. Melios, M. Hardman and O. J. D. M. Kazakova, 2D Mater., 2020, 7, 032002 CrossRef CAS
.
- H. Tai, S. Wang, Z. Duan and Y. Jiang, Sens. Actuators, B, 2020, 318, 128104 CrossRef CAS
.
- M. Righettoni, A. Amann and S. E. Pratsinis, Mater. Today, 2015, 18, 163–171 CrossRef CAS
.
- A. T. Güntner, S. Abegg, K. Königstein, P. A. Gerber, A. Schmidt-Trucksäss and S. E. Pratsinis, ACS Sens., 2019, 4, 268–280 CrossRef PubMed
.
- Q. Li, W. Zeng and Y. Li, Sens. Actuators, B, 2022, 359, 131579 CrossRef CAS
.
- H. Yu, C. Guo, X. Zhang, Y. Xu, X. Cheng, S. Gao and L. Huo, Adv. Sustainable Syst., 2022, 6, 2100370 CrossRef CAS
.
- R. S. Andre, L. A. Mercante, M. H. M. Facure, R. C. Sanfelice, L. Fugikawa-Santos, T. M. Swager and D. S. Correa, ACS Sens., 2022, 7, 2104–2131 CrossRef CAS PubMed
.
- M. Roy and B. K. Yadav, J. Food Sci. Technol., 2022, 59, 846–858 CrossRef PubMed
.
- L. Lu, Z. Hu, X. Hu, D. Li and S. Tian, Food Res. Int., 2022, 162, 112214 CrossRef CAS PubMed
.
- D. Barreca, C. Maccato and A. Gasparotto, Adv. Mater. Interfaces., 2022, 9, 2102525 CrossRef CAS
.
- B. C. Hauck, B. S. Ince and P. C. Riley, ACS Sens., 2023, 8, 2945–2951 CrossRef CAS PubMed
.
- M. Grabka, Z. Witkiewicz, K. Jasek and K. Piwowarski, Sensors, 2022, 22(15), 5607 CrossRef CAS PubMed
.
- G. Neri, Chemosensors, 2015, 3, 1–20 CrossRef CAS
.
- E. P. Nascimento, H. C. T. Firmino, G. A. Neves and R. R. Menezes, Ceram. Int., 2022, 48, 7405–7440 CrossRef CAS
.
- Y. Masuda, Sens. Actuators, B, 2022, 364, 131876 CrossRef CAS
.
- A. P. Cote, A. I. Benin, N. W. Ockwig, M. O'Keeffe, A. J. Matzger and O. M. Yaghi, Science, 2005, 310, 1166–1170 CrossRef CAS PubMed
.
- M. E. Davis, Nature, 2002, 417, 813–821 CrossRef CAS PubMed
.
- S.-Y. Ding and W. Wang, Chem. Soc. Rev., 2013, 42, 548–568 RSC
.
- S. J. Hollister, Nat. Mater., 2005, 4, 518–524 CrossRef CAS PubMed
.
- P. Horcajada, T. Chalati, C. Serre, B. Gillet, C. Sebrie, T. Baati, J. F. Eubank, D. Heurtaux, P. Clayette, C. Kreuz, J.-S. Chang, Y. K. Hwang, V. Marsaud, P.-N. Bories, L. Cynober, S. Gil, G. Ferey, P. Couvreur and R. Gref, Nat. Mater., 2010, 9, 172–178 CrossRef CAS PubMed
.
- P. Horcajada, R. Gref, T. Baati, P. K. Allan, G. Maurin, P. Couvreur, G. Ferey, R. E. Morris and C. Serre, Chem. Rev., 2012, 112, 1232–1268 CrossRef CAS PubMed
.
- L. Ma, C. Abney and W. Lin, Chem. Soc. Rev., 2009, 38, 1248–1256 RSC
.
- J. S. Seo, D. Whang, H. Lee, S. I. Jun, J. Oh, Y. J. Jeon and K. Kim, Nature, 2000, 404, 982–986 CrossRef CAS PubMed
.
- N. Stock and S. Biswas, Chem. Rev., 2012, 112, 933–969 CrossRef CAS PubMed
.
- T.-S. Wong, S. H. Kang, S. K. Y. Tang, E. J. Smythe, B. D. Hatton, A. Grinthal and J. Aizenberg, Nature, 2011, 477, 443–447 CrossRef CAS PubMed
.
- R. Dawson, A. I. Cooper and D. J. Adams, Prog. Polym. Sci., 2012, 37, 530–563 CrossRef CAS
.
- C. S. Diercks and O. M. Yaghi, Science, 2017, 355, 923 CrossRef CAS PubMed
.
- S.-Y. Ding, M. Dong, Y.-W. Wang, Y.-T. Chen, H.-Z. Wang, C.-Y. Su and W. Wang, J. Am. Chem. Soc., 2016, 138, 3031–3037 CrossRef CAS PubMed
.
- S.-Y. Ding, J. Gao, Q. Wang, Y. Zhang, W.-G. Song, C.-Y. Su and W. Wang, J. Am. Chem. Soc., 2011, 133, 19816–19822 CrossRef CAS PubMed
.
- H. M. El-Kaderi, J. R. Hunt, J. L. Mendoza-Cortes, A. P. Cote, R. E. Taylor, M. O'Keeffe and O. M. Yaghi, Science, 2007, 316, 268–272 CrossRef CAS PubMed
.
- Q. Fang, Z. Zhuang, S. Gu, R. B. Kaspar, J. Zheng, J. Wang, S. Qiu and Y. Yan, Nat. Commun., 2014, 5 Search PubMed
.
- X. Feng, X. Ding and D. Jiang, Chem. Soc. Rev., 2012, 41, 6010–6022 RSC
.
- H. Furukawa and O. M. Yaghi, J. Am. Chem. Soc., 2009, 131, 8875–8883 CrossRef CAS PubMed
.
- K. Geng, T. He, R. Liu, K. T. Tan, Z. Li, S. Tao, Y. Gong, Q. Jiang and D. Jiang, Chem. Rev., 2020, 120, 8814–8933 CrossRef CAS PubMed
.
- N. Huang, P. Wang and D. Jiang, Nat. Rev. Mater., 2016, 1, 16068 CrossRef CAS
.
- S. Kandambeth, A. Mallick, B. Lukose, M. V. Mane, T. Heine and R. Banerjee, J. Am. Chem. Soc., 2012, 134, 19524–19527 CrossRef CAS PubMed
.
- S. Lin, C. S. Diercks, Y.-B. Zhang, N. Kornienko, E. M. Nichols, Y. Zhao, A. R. Paris, D. Kim, P. Yang, O. M. Yaghi and C. J. Chang, Science, 2015, 349, 1208–1213 CrossRef CAS PubMed
.
- P. Miro, M. Audiffred and T. Heine, Chem. Soc. Rev., 2014, 43, 6537–6554 RSC
.
- A. G. Slater and A. I. Cooper, Science, 2015, 348, 988 CrossRef CAS PubMed
.
- F. J. Uribe-Romo, J. R. Hunt, H. Furukawa, C. Kloeck, M. O'Keeffe and O. M. Yaghi, J. Am. Chem. Soc., 2009, 131, 4570–4571 CrossRef CAS PubMed
.
- P. J. Waller, F. Gandara and O. M. Yaghi, Acc. Chem. Res., 2015, 48, 3053–3063 CrossRef CAS PubMed
.
- F. Wang, X. Wu, X. Yuan, Z. Liu, Y. Zhang, L. Fu, Y. Zhu, Q. Zhou, Y. Wu and W. Huang, Chem. Soc. Rev., 2017, 46, 6816–6854 RSC
.
- H. Xu, J. Gao and D. Jiang, Nat. Chem., 2015, 7, 905–912 CrossRef CAS PubMed
.
- T. Zhang, G. Xing, W. Chen and L. Chen, Mater. Chem. Front., 2020, 4, 332–353 RSC
.
- H. Furukawa, K. E. Cordova, M. O'Keeffe and O. M. Yaghi, Science, 2013, 341, 974 CrossRef CAS PubMed
.
- S. L. James, Chem. Soc. Rev., 2003, 32, 276–288 RSC
.
- C. Janiak, Dalton Trans., 2003, 2781–2804 RSC
.
- L. E. Kreno, K. Leong, O. K. Farha, M. Allendorf, R. P. Van Duyne and J. T. Hupp, Chem. Rev., 2012, 112, 1105–1125 CrossRef CAS PubMed
.
- J. Y. Lee, O. K. Farha, J. Roberts, K. A. Scheidt, S. B. T. Nguyen and J. T. Hupp, Chem. Soc. Rev., 2009, 38, 1450–1459 RSC
.
- H. Li, M. Eddaoudi, M. O'Keeffe and M. Yaghi, Nature, 1999, 402, 276–279 CrossRef CAS
.
- J.-R. Li, J. Sculley and H.-C. Zhou, Chem. Rev., 2012, 112, 869–932 CrossRef CAS PubMed
.
- N. L. Rosi, J. Eckert, M. Eddaoudi, D. T. Vodak, J. Kim, M. O'Keeffe and O. M. Yaghi, Science, 2003, 300, 1127–1130 CrossRef CAS PubMed
.
- M. Majumder, M. S. Santosh, R. Viswanatha, A. K. Thakur, D. P. Dubal and K. Jayaramulu, Energy Storage Mater., 2021, 37, 396–416 CrossRef
.
- K. Jayaramulu, S. Mukherjee, D. M. Morales, D. P. Dubal, A. K. Nanjundan, A. Schneemann, J. Masa, S. Kment, W. Schuhmann, M. Otyepka, R. Zbořil and R. A. Fischer, Chem. Rev., 2022, 122, 17241–17338 CrossRef CAS PubMed
.
-
N. Yamazoe and K. Shimanoe, in Semicon. Gas Sens., ed. R. Jaaniso and O. K. Tan, Woodhead Publishing, 2013, pp. 3–34 Search PubMed
.
- K. G. Krishna, S. Parne, N. Pothukanuri, V. Kathirvelu, S. Gandi and D. Joshi, Sens. Actuators, A, 2022, 341, 113578 CrossRef CAS
.
- B. Ates, S. Koytepe, A. Ulu, C. Gurses and V. K. Thakur, Chem. Rev., 2020, 120, 9304–9362 CrossRef CAS PubMed
.
- E. Bakker and M. Telting-Diaz, Anal. Chem., 2002, 74, 2781–2800 CrossRef CAS PubMed
.
- M. L. Hu, S. A. A. Razavi, M. Piroozzadeh and A. Morsali, Inorg. Chem. Front., 2020, 7, 1598–1632 RSC
.
- S. M. Kanan, O. M. El-Kadri, I. A. Abu-Yousef and M. C. Kanan, Sensors, 2009, 9, 8158–8196 CrossRef CAS PubMed
.
- S. Kumar, R. Rani, N. Dilbaghi, K. Tankeshwar and K. H. Kim, Chem. Soc. Rev., 2017, 46, 158–196 RSC
.
- X. Liu, T. Ma, N. Pinna and J. Zhang, Adv. Funct. Mater., 2017, 27 Search PubMed
.
- S. Mao, J. Chang, H. Pu, G. Lu, Q. He, H. Zhang and J. Chen, Chem. Soc. Rev., 2017, 46, 6872–6904 RSC
.
- J. M. Moon, N. Thapliyal, K. K. Hussain, R. N. Goyal and Y. B. Shim, Biosens. Bioelectron., 2018, 102, 540–552 CrossRef CAS PubMed
.
- S. K. Pandey, K. H. Kim and K. T. Tang, Trends Anal. Chem., 2012, 32, 87–99 CrossRef CAS
.
- N. G. Patel, P. D. Patel and V. S. Vaishnav, Sens. Actuators, B, 2003, 96, 180–189 CrossRef CAS
.
- S. S. Shendage, V. L. Patil, S. A. Vanalakar, S. P. Patil, N. S. Harale, J. L. Bhosale, J. H. Kim and P. S. Patil, Sens. Actuators, B, 2017, 240, 426–433 CrossRef CAS
.
- N. P. Shetti, S. D. Bukkitgar, K. R. Reddy, C. V. Reddy and T. M. Aminabhavi, Biosens. Bioelectron., 2019, 141 Search PubMed
.
- E. Singh, M. Meyyappan and H. S. Nalwa, ACS Appl. Mater. Interfaces, 2017, 9, 34544–34586 CrossRef CAS PubMed
.
- P. L. Wang, L. H. Xie, E. A. Joseph, J. R. Li, X. O. Su and H. C. Zhou, Chem. Rev., 2019, 119, 10638–10690 CrossRef CAS PubMed
.
- H. Yuan, S. A. A. A. Aljneibi, J. Yuan, Y. Wang, H. Liu, J. Fang, C. Tang, X. Yan, H. Cai, Y. Gu, S. J. Pennycook, J. Tao and D. Zhao, Adv. Mater., 2019, 31 Search PubMed
.
- X. Zhou, S. Lee, Z. Xu and J. Yoon, Chem. Rev., 2015, 115, 7944–8000 CrossRef CAS PubMed
.
-
S. Barnola, N. Posseme, S. Landis and M. Darnon, in Plasma Etching Processes for CMOS Devices Realization, ed. N. Posseme, Elsevier, 2017, pp. 59–94 Search PubMed
.
- Y. Chen, Microelectron. Eng., 2015, 135, 57–72 CrossRef CAS
.
- S. Vallejos, F. Di Maggio, T. Shujah and C. Blackman, Chemosensors, 2016, 4(1), 4 CrossRef
.
- T. Seiyama, A. Kato, K. Fujiishi and M. Nagatani, Anal. Chem., 1962, 34, 1502–1503 CrossRef CAS
.
- A. J. Bandodkar, I. Jeerapan and J. Wang, ACS Sens., 2016, 1, 464–482 CrossRef CAS
.
- H.-Y. Li, S.-N. Zhao, S.-Q. Zang and J. Li, Chem. Soc. Rev., 2020, 49, 6364–6401 RSC
.
- N. S. S. Capman, X. V. Zhen, J. T. Nelson, V. R. S. K. Chaganti, R. C. Finc, M. J. Lyden, T. L. Williams, M. Freking, G. J. Sherwood, P. Bühlmann, C. J. Hogan and S. J. Koester, ACS Nano, 2022, 16, 19567–19583 CrossRef CAS PubMed
.
- Z. Ballard, C. Brown, A. M. Madni and A. Ozcan, Nat. Mach. Intell., 2021, 3, 556–565 CrossRef
.
-
N. Taguchi, US Pat., 3631436, 1971 Search PubMed
.
- A. Dey, Mater. Sci.Eng. B, 2018, 229, 206–217 CrossRef CAS
.
- I. Simon, N. Bârsan, M. Bauer and U. Weimar, Sens. Actuators, B, 2001, 73, 1–26 CrossRef CAS
.
- T. P. Mokoena, H. C. Swart and D. E. Motaung, J. Alloys Compd., 2019, 805, 267–294 CrossRef CAS
.
- N. Rahman, J. Yang Zulfiqar, M. Sohail, R. Khan, A. Iqbal, C. Maouche, A. A. Khan, M. Husain, S. A. Khattak, S. N. Khan and A. Khan, Sens. Actuators, A, 2021, 332, 113128 CrossRef CAS
.
- J. M. Xu and J. P. Cheng, J. Alloys Compd., 2016, 686, 753–768 CrossRef CAS
.
- X. Tian, X. Cui, T. Lai, J. Ren, Z. Yang, M. Xiao, B. Wang, X. Xiao and Y. Wang, Nano Mater. Sci., 2021, 3, 390–403 CrossRef CAS
.
- H.-J. Kim and J.-H. Lee, Sens. Actuators, B, 2014, 192, 607–627 CrossRef CAS
.
- N. Barsan and U. J. Weimar, J. Phys.: Condens. Matter, 2003, 15, R813 CrossRef CAS
.
- A. M. Pineda-Reyes, M. R. Herrera-Rivera, H. Rojas-Chávez, H. Cruz-Martínez and D. I. Medina, Sensors, 2021, 21(13), 4425 CrossRef CAS PubMed
.
- C. Dong, R. Zhao, L. Yao, Y. Ran, X. Zhang and Y. Wang, J. Alloys Compd., 2020, 820, 153194 CrossRef CAS
.
- J. Kappler, N. Bârsan, U. Weimar, A. Dièguez, J. L. Alay, A. Romano-Rodriguez, J. R. Morante and W. Göpel, Fresenius, J. Anal. Chem., 1998, 361, 110–114 CrossRef CAS
.
- Y. C. Lee, H. Huang, O. K. Tan and M. S. Tse, Sens. Actuators, B, 2008, 132, 239–242 CrossRef CAS
.
- J.-K. Choi, I.-S. Hwang, S.-J. Kim, J.-S. Park, S.-S. Park, U. Jeong, Y. C. Kang and J.-H. Lee, Sens. Actuators, B, 2010, 150, 191–199 CrossRef CAS
.
- Y. Shen, T. Yamazaki, Z. Liu, D. Meng, T. Kikuta, N. Nakatani, M. Saito and M. Mori, Sens. Actuators, B, 2009, 135, 524–529 CrossRef CAS
.
- B. Zhang, M. Li, Z. Song, H. Kan, H. Yu, Q. Liu, G. Zhang and H. Liu, Sens. Actuators, B, 2017, 249, 558–563 CrossRef CAS
.
- S. Agarwal, P. Rai, E. N. Gatell, E. Llobet, F. Güell, M. Kumar and K. Awasthi, Sens. Actuators, B, 2019, 292, 24–31 CrossRef CAS
.
- Y. Zhang, J. Xu, Q. Xiang, H. Li, Q. Pan and P. Xu, J. Phys. Chem. C, 2009, 113, 3430–3435 CrossRef CAS
.
- A. Mortezaali and R. Moradi, Sens. Actuators, A, 2014, 206, 30–34 CrossRef CAS
.
- M. Thepnurat, T. Chairuangsri, N. Hongsith, P. Ruankham and S. Choopun, ACS Appl. Mater. Interfaces, 2015, 7, 24177–24184 CrossRef CAS PubMed
.
- A. Wei, L. Pan and W. Huang, Mater. Sci. Eng. B, 2011, 176, 1409–1421 CrossRef CAS
.
- V. S. Bhati, M. Hojamberdiev and M. Kumar, Energy Rep., 2020, 6, 46–62 CrossRef
.
- R. Kumar, O. Al-Dossary, G. Kumar and A. Umar, Nanomicro Lett., 2015, 7, 97–120 Search PubMed
.
- S. G. Leonardi, Chemosensors, 2017, 5(2), 17 CrossRef
.
- R. Ahmad, S. M. Majhi, X. Zhang, T. M. Swager and K. N. Salama, Adv. Colloid Interface Sci., 2019, 270, 1–27 CrossRef CAS PubMed
.
- L. Schmidt-Mende and J. L. MacManus-Driscoll, Mater. Today, 2007, 10, 40–48 CrossRef CAS
.
- K.-S. Choi and S.-P. Chang, Mater. Lett., 2018, 230, 48–52 CrossRef CAS
.
- M. Drobek, J.-H. Kim, M. Bechelany, C. Vallicari, A. Julbe and S. S. Kim, ACS Appl. Mater. Interfaces, 2016, 8, 8323–8328 CrossRef CAS PubMed
.
- M. Velumani, S. R. Meher and Z. C. Alex, J. Mater. Sci.: Mater. Electron., 2018, 29, 3999–4010 CrossRef CAS
.
- J. Sukunta, A. Wisitsoraat, A. Tuantranont, S. Phanichphant and C. Liewhiran, Appl. Surf. Sci., 2018, 458, 319–332 CrossRef CAS
.
- M. Ivanovskaya, D. Kotsikau, G. Faglia, P. Nelli and S. Irkaev, Sens. Actuators, B, 2003, 93, 422–430 CrossRef CAS
.
- H. Bai and G. Shi, Sensors, 2007, 7(3), 267–307 CrossRef CAS
.
- Q. Fang, D. G. Chetwynd, J. A. Covington, C. S. Toh and J. W. Gardner, Sens. Actuators, B, 2002, 84, 66–71 CrossRef CAS
.
- N. Gao, J. Yu, Q. Tian, J. Shi, M. Zhang, S. Chen and L. Zang, Chemosensors, 2021, 9(12), 335 CrossRef
.
- D. Kumar and R. C. Sharma, Eur. Polym. J., 1998, 34, 1053–1060 CrossRef CAS
.
- S. J. Park, C. S. Park and H. Yoon, Polymers, 2017, 9(5), 155 CrossRef PubMed
.
- J. Jang and J. H. Oh, Chem. Commun., 2002, 2200–2201 RSC
.
- M. R. Simmons, P. A. Chaloner and S. P. Armes, Langmuir, 1995, 11, 4222–4224 CrossRef CAS
.
- M. G. Han, S. K. Cho, S. G. Oh and S. S. Im, Synth. Met., 2002, 126, 53–60 CrossRef CAS
.
- J. Wang, S. Chan, R. R. Carlson, Y. Luo, G. Ge, R. S. Ries, J. R. Heath and H.-R. Tseng, Nano Lett., 2004, 4, 1693–1697 CrossRef CAS
.
- H. Sahabudeen, H. Qi, B. A. Glatz, D. Tranca, R. Dong, Y. Hou, T. Zhang, C. Kuttner, T. Lehnert, G. Seifert, U. Kaiser, A. Fery, Z. Zheng and X. Feng, Nat. Commun., 2016, 7, 13461 CrossRef CAS PubMed
.
- C. L. Hansen, M. O. A. Sommer and S. R. Quake, Proc. Natl. Acad. Sci. U. S. A., 2004, 101, 14431–14436 CrossRef CAS PubMed
.
- L. Veeramuthu, M. Venkatesan, J.-S. Benas, C.-J. Cho, C.-C. Lee, F.-K. Lieu, J.-H. Lin, R.-H. Lee and C.-C. Kuo, Polymers, 2021, 13(24), 4281 CrossRef CAS PubMed
.
- N. K. Guimard, N. Gomez and C. E. Schmidt, Prog. Polym. Sci., 2007, 32, 876–921 CrossRef CAS
.
- X. Liu, W. Zheng, R. Kumar, M. Kumar and J. Zhang, Coord. Chem. Rev., 2022, 462, 214517 CrossRef CAS
.
- S. Virji, J. Huang, R. B. Kaner and B. H. Weiller, Nano Lett., 2004, 4, 491–496 CrossRef CAS
.
- L. M. G. Padua, J.-M. Yeh and K. S. Santiago, Polymers, 2019, 11(12), 1918 CrossRef CAS PubMed
.
- C. Liu, H. Tai, P. Zhang, Z. Yuan, X. Du, G. Xie and Y. Jiang, Sens. Actuators, B, 2018, 261, 587–597 CrossRef CAS
.
- S. Kotresh, Y. T. Ravikiran and K. M. Batoo, Sens. Actuators, A, 2017, 263, 687–692 CrossRef CAS
.
- S. Bai, Y. Tian, M. Cui, J. Sun, Y. Tian, R. Luo, A. Chen and D. Li, Sens. Actuators, B, 2016, 226, 540–547 CrossRef CAS
.
- S. B. Kulkarni, Y. H. Navale, S. T. Navale, F. J. Stadler, N. S. Ramgir and V. B. Patil, Sens. Actuators, B, 2019, 288, 279–288 CrossRef CAS
.
- V. Talwar, O. Singh and R. C. Singh, Sens. Actuators, B, 2014, 191, 276–282 CrossRef CAS
.
- T. Sen, N. G. Shimpi, S. Mishra and R. Sharma, Sens. Actuators, B, 2014, 190, 120–126 CrossRef CAS
.
- H. Tai, Y. Jiang, G. Xie, J. Yu and X. Chen, Sens. Actuators, B, 2007, 125, 644–650 CrossRef CAS
.
- J. Gong, Y. Li, Z. Hu, Z. Zhou and Y. Deng, J. Phys. Chem. C, 2010, 114, 9970–9974 CrossRef CAS
.
- S. Li, A. Liu, Z. Yang, L. Zhao, J. Wang, F. Liu, R. You, J. He, C. Wang, X. Yan, P. Sun, X. Liang and G. Lu, Sens. Actuators, B, 2019, 289, 252–259 CrossRef CAS
.
- S. Li, P. Lin, L. Zhao, C. Wang, D. Liu, F. Liu, P. Sun, X. Liang, F. Liu, X. Yan, Y. Gao and G. Lu, Sens. Actuators, B, 2018, 259, 505–513 CrossRef CAS
.
- J. Jun, J. S. Lee, D. H. Shin, J. Oh, W. Kim, W. Na and J. Jang, J. Mater. Chem. A, 2017, 5, 17335–17340 RSC
.
- S. Iijima, Nature, 1991, 354, 56–58 CrossRef CAS
.
- P. Dariyal, S. Sharma, G. S. Chauhan, B. P. Singh and S. R. Dhakate, Nanoscale Adv., 2021, 3, 6514–6544 RSC
.
-
M. Penza, P. J. Martin and J. T. W. Yeow, Gas Sens. Fundam., ed. C.-D. Kohl and T. Wagner, Springer, Berlin Heidelberg, 2014, pp. 109–174 Search PubMed
.
- T. Han, A. Nag, S. Chandra Mukhopadhyay and Y. Xu, Sens. Actuators, A, 2019, 291, 107–143 CrossRef CAS
.
- C. Cantalini, L. Valentini, I. Armentano, L. Lozzi, J. M. Kenny and S. Santucci, Sens. Actuators, B, 2003, 95, 195–202 CrossRef CAS
.
- W. Zhang, S. Cao, Z. Wu, M. Zhang, Y. Cao, J. Guo, F. Zhong, H. Duan and D. Jia, Sensors, 2020, 20, 149 CrossRef CAS PubMed
.
-
N. Jamil, F. Jameel, S. Z. Bajwa, A. Rehman, R. F. Hussain Khan, A. Mahmood and W. S. Khan, in Metal Oxide Carbon Hybd. Mater., ed. M. A. Chaudhry, R. Hussain and F. K. Butt, Elsevier, 2022, pp. 459–474 Search PubMed
.
- M. N. Norizan, M. H. Moklis, S. Z. Ngah Demon, N. A. Halim, A. Samsuri, I. S. Mohamad, V. F. Knight and N. Abdullah, RSC Adv., 2020, 10, 43704–43732 RSC
.
- D. Fu, H. Lim, Y. Shi, X. Dong, S. G. Mhaisalkar, Y. Chen, S. Moochhala and L.-J. Li, J. Phys. Chem. C, 2008, 112, 650–653 CrossRef CAS
.
- T. H. Tran, J.-W. Lee, K. Lee, Y. D. Lee and B.-K. Ju, Sens. Actuators, B, 2008, 129, 67–71 CrossRef CAS
.
- T. Wang, D. Huang, Z. Yang, S. Xu, G. He, X. Li, N. Hu, G. Yin, D. He and L. Zhang, NanoMicro Lett., 2016, 8, 95–119 CrossRef PubMed
.
- W. Yuan and G. Shi, J. Mater. Chem. A, 2013, 1, 10078–10091 RSC
.
- Y.-H. Zhang, L.-F. Han, Y.-H. Xiao, D.-Z. Jia, Z.-H. Guo and F. Li, Comput. Mater. Sci., 2013, 69, 222–228 CrossRef CAS
.
- Y.-H. Zhang, Y.-B. Chen, K.-G. Zhou, C.-H. Liu, J. Zeng, H.-L. Zhang and Y. J. N. Peng, Nanotechnology, 2009, 20, 185504 CrossRef PubMed
.
- F. Schedin, A. K. Geim, S. V. Morozov, E. W. Hill, P. Blake, M. I. Katsnelson and K. S. Novoselov, Nat. Mater., 2007, 6, 652–655 CrossRef CAS PubMed
.
- W. Yuan, A. Liu, L. Huang, C. Li and G. Shi, Adv. Mater., 2013, 25, 766–771 CrossRef CAS PubMed
.
- B. Zou, Y. Guo, N. Shen, A. Xiao, M. Li, L. Zhu, P. Wan and X. Sun, Sensors, 2017, 17(12), 2954 CrossRef PubMed
.
- G. Wu, H. Du, Y. L. Cha, D. Lee, W. Kim, F. Feyzbar-Khalkhali-Nejad, T.-S. Oh, X. Zhang and D.-J. Kim, Sens. Actuators, B, 2023, 375, 132858 CrossRef CAS
.
- F.-L. Meng, Z. Guo and X.-J. Huang, Trends Anal. Chem., 2015, 68, 37–47 CrossRef CAS
.
- U. Latif and F. L. Dickert, Sensors, 2015, 15(12), 30504–30524 CrossRef CAS PubMed
.
- Z. Song, Z. Wei, B. Wang, Z. Luo, S. Xu, W. Zhang, H. Yu, M. Li, Z. Huang, J. Zang, F. Yi and H. Liu, Chem. Mater., 2016, 28, 1205–1212 CrossRef CAS
.
- A. V. Singhal, H. Charaya and I. Lahiri, Crit. Rev. Solid State Mater. Sci., 2017, 42, 499–526 CrossRef CAS
.
- S. Basu and S. K. Hazra, Journal, 2017, 3 Search PubMed
.
- Z. Wang, Y. Zhang, S. Liu and T. Zhang, Sens. Actuators, B, 2016, 222, 893–903 CrossRef CAS
.
- B. Sharma and J.-S. Kim, Int. J. Hydrog. Energy, 2018, 43, 11397–11402 CrossRef CAS
.
- T. Yoon, J. Jun, D. Y. Kim, S. Pourasad, T. J. Shin, S. U. Yu, W. Na, J. Jang and K. S. Kim, J. Mater. Chem. A, 2018, 6, 2257–2263 RSC
.
- B. Kwon, H. Bae, H. Lee, S. Kim, J. Hwang, H. Lim, J. H. Lee, K. Cho, J. Ye, S. Lee and W. H. Lee, ACS Nano, 2022, 16, 2176–2187 CrossRef CAS PubMed
.
- G. Shi, T. Yoon, S. Cha, S. Kim, M. Yousuf, N. Ahmed, D. Kim, H.-W. Kang and K. S. Kim, ACS Sens., 2018, 3, 1102–1108 CrossRef CAS PubMed
.
- Y.-M. Jo, Y. K. Jo, J.-H. Lee, H. W. Jang, I.-S. Hwang and D. J. Yoo, Adv. Mater., 2023, 35, 2206842 CrossRef CAS PubMed
.
- A. Mahmood, W. Guo, H. Tabassum and R. Zou, Adv. Energy Mater., 2016, 6, 1600423 CrossRef
.
- D. Sheberla, J. C. Bachman, J. S. Elias, C.-J. Sun, Y. Shao-Horn and M. Dincă, Nat. Mater., 2017, 16, 220–224 CrossRef CAS PubMed
.
- K. Fujie, R. Ikeda, K. Otsubo, T. Yamada and H. Kitagawa, Chem. Mater., 2015, 27, 7355–7361 CrossRef CAS
.
- L. J. Murray, M. Dincă and J. R. Long, Chem. Soc. Rev., 2009, 38, 1294–1314 RSC
.
- D. Alezi, Y. Belmabkhout, M. Suyetin, P. M. Bhatt, J. Weseliński, V. Solovyeva, K. Adil, I. Spanopoulos, P. N. Trikalitis, A.-H. Emwas and M. Eddaoudi, J. Am. Chem. Soc., 2015, 137, 13308–13318 CrossRef CAS PubMed
.
- J. A. Mason, M. Veenstra and J. R. Long, Chem. Sci., 2014, 5, 32–51 RSC
.
- H. Demir and S. Keskin, Mol. Syst. Des. Eng., 2021, 6, 627–642 RSC
.
- Q. Qian, P. A. Asinger, M. J. Lee, G. Han, K. Mizrahi Rodriguez, S. Lin, F. M. Benedetti, A. X. Wu, W. S. Chi and Z. P. Smith, Chem. Rev., 2020, 120, 8161–8266 CrossRef CAS PubMed
.
- S. Qiu, M. Xue and G. Zhu, Chem. Soc. Rev., 2014, 43, 6116–6140 RSC
.
- T. Leelasree, V. Selamneni, T. Akshaya, P. Sahatiya and H. Aggarwal, J. Mater. Chem. B, 2020, 8, 10182–10189 RSC
.
- M. K. Smith and K. A. Mirica, J. Am. Chem. Soc., 2017, 139, 16759–16767 CrossRef CAS PubMed
.
- M. K. Smith, K. E. Jensen, P. A. Pivak and K. A. Mirica, Chem. Mater., 2016, 28, 5264–5268 CrossRef CAS
.
- N. Rabiee, M. Atarod, M. Tavakolizadeh, S. Asgari, M. Rezaei, O. Akhavan, A. Pourjavadi, M. Jouyandeh, E. C. Lima, A. Hamed Mashhadzadeh, A. Ehsani, S. Ahmadi and M. R. Saeb, Microporous Mesoporous Mater., 2022, 335, 111670 CrossRef CAS
.
- J. Chen, F. Cheng, D. Luo, J. Huang, J. Ouyang, A. Nezamzadeh-Ejhieh, M. S. Khan, J. Liu and Y. Peng, Dalton Trans., 2022, 51, 14817–14832 RSC
.
- J. Chen, Y. Zhu and S. Kaskel, Angew. Chem., Int. Ed., 2021, 60, 5010–5035 CrossRef CAS PubMed
.
- A. Khezerlou, M. Tavassoli, B. Khalilzadeh, A. Ehsani and H. Kazemian, Food Control, 2023, 153, 109965 CrossRef CAS
.
- A. Soni, R. Sharma, D. S. Rana, D. Singh and N. Gupta, Coord. Chem. Rev., 2023, 494, 215343 CrossRef CAS
.
- D. Wang, C. Yuan, C. Yang, P. Wang, Y. Zhan, N. Guo, L. Jiang, Z. Wang and Z. Wang, Sep. Purif. Technol., 2023, 326, 124765 CrossRef CAS
.
- E.-X. Chen, H. Yang and J. Zhang, Inorg. Chem., 2014, 53, 5411–5413 CrossRef CAS PubMed
.
- G. Lu and J. T. Hupp, J. Am. Chem. Soc., 2010, 132, 7832–7833 CrossRef CAS PubMed
.
- E.-X. Chen, H.-R. Fu, R. Lin, Y.-X. Tan and J. Zhang, ACS Appl. Mater. Interfaces, 2014, 6, 22871–22875 CrossRef CAS PubMed
.
- Y. Kobayashi, B. Jacobs, M. D. Allendorf and J. R. Long, Chem. Mater., 2010, 22, 4120–4122 CrossRef CAS
.
- F. Gándara, F. J. Uribe-Romo, D. K. Britt, H. Furukawa, L. Lei, R. Cheng, X. Duan, M. O'Keeffe and O. M. Yaghi, Chem. – Eur. J., 2012, 18, 10595–10601 CrossRef PubMed
.
- T. C. Narayan, T. Miyakai, S. Seki and M. Dincă, J. Am. Chem. Soc., 2012, 134, 12932–12935 CrossRef CAS PubMed
.
- L. Sun, T. Miyakai, S. Seki and M. Dincă, J. Am. Chem. Soc., 2013, 135, 8185–8188 CrossRef CAS PubMed
.
- A. A. Talin, A. Centrone, A. C. Ford, M. E. Foster, V. Stavila, P. Haney, R. A. Kinney, V. Szalai, F. El Gabaly, H. P. Yoon, F. Léonard and M. D. Allendorf, Science, 2014, 343, 66–69 CrossRef CAS PubMed
.
- D. Sheberla, L. Sun, M. A. Blood-Forsythe, S. Er, C. R. Wade, C. K. Brozek, A. Aspuru-Guzik and M. Dincă, J. Am. Chem. Soc., 2014, 136, 8859–8862 CrossRef CAS PubMed
.
- S. S. Park, E. R. Hontz, L. Sun, C. H. Hendon, A. Walsh, T. Van Voorhis and M. Dincă, J. Am. Chem. Soc., 2015, 137, 1774–1777 CrossRef CAS PubMed
.
- L. Sun, C. H. Hendon, M. A. Minier, A. Walsh and M. Dincă, J. Am. Chem. Soc., 2015, 137, 6164–6167 CrossRef CAS PubMed
.
- M. G. Campbell, D. Sheberla, S. F. Liu, T. M. Swager and M. Dincă, Angew. Chem., Int. Ed., 2015, 54, 4349–4352 CrossRef CAS PubMed
.
- M. G. Campbell, S. F. Liu, T. M. Swager and M. Dincă, J. Am. Chem. Soc., 2015, 137, 13780–13783 CrossRef CAS PubMed
.
- S. Chen, J. Dai and X. C. Zeng, Phys. Chem. Chem. Phys., 2015, 17, 5954–5958 RSC
.
- B. Zhao, J. Zhang, W. Feng, Y. Yao and Z. Yang, Phys. Rev. B: Condens. Matter Mater. Phys., 2014, 90, 201403 CrossRef
.
- T. C. Wang, W. Bury, D. A. Gómez-Gualdrón, N. A. Vermeulen, J. E. Mondloch, P. Deria, K. Zhang, P. Z. Moghadam, A. A. Sarjeant, R. Q. Snurr, J. F. Stoddart, J. T. Hupp and O. K. Farha, J. Am. Chem. Soc., 2015, 137, 3585–3591 CrossRef CAS PubMed
.
- O. K. Farha, A. M. Shultz, A. A. Sarjeant, S. T. Nguyen and J. T. Hupp, J. Am. Chem. Soc., 2011, 133, 5652–5655 CrossRef CAS PubMed
.
- J. Gao, X. Qian, R.-B. Lin, R. Krishna, H. Wu, W. Zhou and B. Chen, Angew. Chem., Int. Ed., 2020, 59, 4396–4400 CrossRef CAS PubMed
.
- M.-S. Yao, X.-J. Lv, Z.-H. Fu, W.-H. Li, W.-H. Deng, G.-D. Wu and G. Xu, Angew. Chem., Int. Ed., 2017, 56, 16510–16514 CrossRef CAS PubMed
.
- Z. Li, H. Li, Z. Wu, M. Wang, J. Luo, H. Torun, P. Hu, C. Yang, M. Grundmann, X. Liu and Y. Fu, Mater. Horiz., 2019, 6, 470–506 RSC
.
- S. Wang, J. Liu, H. Zhao, Z. Guo, H. Xing and Y. Gao, Inorg. Chem., 2018, 57, 541–544 CrossRef CAS PubMed
.
- M. E. Dmello, N. G. Sundaram, A. Singh, A. K. Singh and S. B. Kalidindi, Chem. Commun., 2019, 55, 349–352 RSC
.
- T. Omori, T. Kusama, S. Kawata, I. Ohnuma, Y. Sutou, Y. Araki, K. Ishida and R. Kainuma, Science, 2013, 341, 1500–1502 CrossRef CAS PubMed
.
- I. Stassen, J.-H. Dou, C. Hendon and M. Dincă, ACS Cent. Sci., 2019, 5, 1425–1431 CrossRef CAS PubMed
.
- Z. Meng, A. Aykanat and K. A. Mirica, J. Am. Chem. Soc., 2019, 141, 2046–2053 CrossRef CAS PubMed
.
- M. Wang, Z. Zhang, H. Zhong, X. Huang, W. Li, M. Hambsch, P. Zhang, Z. Wang, P. S. Petkov, T. Heine, S. C. B. Mannsfeld, X. Feng and R. Dong, Angew. Chem., Int. Ed., 2021, 60, 18666–18672 CrossRef CAS PubMed
.
- Y. Lin, W.-H. Li, Y. Wen, G.-E. Wang, X.-L. Ye and G. Xu, Angew. Chem., Int. Ed., 2021, 60, 25758–25761 CrossRef CAS PubMed
.
- A. Aykanat, Z. Meng, R. M. Stolz, C. T. Morrell and K. A. Mirica, Angew. Chem., Int. Ed., 2022, 61, e202113665 CrossRef CAS PubMed
.
- L. Liu, Y. Wang, Y. Liu, S. Wang, T. Li, S. Feng, S. Qin and T. Zhang, Microsyst. Nanoeng., 2022, 8, 85 CrossRef CAS PubMed
.
- D. Degler, U. Weimar and N. Barsan, ACS Sens., 2019, 4, 2228–2249 CrossRef CAS PubMed
.
- K. Jayaramulu, M. Esclance Dmello, K. Kesavan, A. Schneemann, M. Otyepka, S. Kment, C. Narayana, S. B. Kalidindi, R. S. Varma, R. Zboril and R. A. Fischer, J. Mater. Chem. A, 2021, 9, 17434–17441 RSC
.
- L. K. Macreadie, E. J. Mensforth, R. Babarao, K. Konstas, S. G. Telfer, C. M. Doherty, J. Tsanaktsidis, S. R. Batten and M. R. Hill, J. Am. Chem. Soc., 2019, 141, 3828–3832 CrossRef CAS PubMed
.
- H. Tian, H. Fan, M. Li and L. Ma, ACS Sens., 2016, 1, 243–250 CrossRef CAS
.
- J. Gonzalez-Chavarri, L. Parellada-Monreal, I. Castro-Hurtado, E. Castaño and G. G. Mandayo, Sens. Actuators, B, 2018, 255, 1244–1253 CrossRef CAS
.
- W.-T. Koo, S. Qiao, A. F. Ogata, G. Jha, J.-S. Jang, V. T. Chen, I.-D. Kim and R. M. Penner, ACS Nano, 2017, 11, 9276–9285 CrossRef CAS PubMed
.
- T. Zhou, Z. Qin, X. Wang, C. Wu, X. Tang, T. Zhang, H. Wang, C. Xie and D. Zeng, Chem. Commun., 2019, 55, 11045–11048 RSC
.
- M. Weber, J.-H. Kim, J.-H. Lee, J.-Y. Kim, I. Iatsunskyi, E. Coy, M. Drobek, A. Julbe, M. Bechelany and S. S. Kim, ACS Appl. Mater. Interfaces, 2018, 10, 34765–34773 CrossRef CAS PubMed
.
- M. P. M. Poschmann, L. Siebert, C. Lupan, O. Lupan, F. Schütt, R. Adelung and N. Stock, ACS Appl. Mater. Interfaces, 2023, 15, 38674–38681 CrossRef CAS PubMed
.
- M. E. Dmello, N. G. Sundaram and S. B. Kalidindi, Chem. – Eur. J., 2018, 24, 9220–9223 CrossRef CAS PubMed
.
- P. Wang, X. Zou, H. Tan, S. Wu, L. Jiang and G. Zhu, J. Mater. Chem. C, 2018, 6, 5412–5419 RSC
.
- H. Shen, H. Li, Z. Yang and C. Li, Green Energy Environ., 2022, 7, 1161–1198 CrossRef CAS
.
- G. Lu, S. Li, Z. Guo, O. K. Farha, B. G. Hauser, X. Qi, Y. Wang, X. Wang, S. Han, X. Liu, J. S. DuChene, H. Zhang, Q. Zhang, X. Chen, J. Ma, S. C. J. Loo, W. D. Wei, Y. Yang, J. T. Hupp and F. Huo, Nat. Chem., 2012, 4, 310–316 CrossRef CAS PubMed
.
- W.-T. Koo, S.-J. Kim, J.-S. Jang, D.-H. Kim and I.-D. Kim, Adv. Sci., 2019, 6, 1900250 CrossRef CAS PubMed
.
- F. Qu, S. Zhang, C. Huang, X. Guo, Y. Zhu, T. Thomas, H. Guo, J. P. Attfield and M. Yang, Angew. Chem., Int. Ed., 2021, 60, 6561–6566 CrossRef CAS PubMed
.
- H. Li, J. Zhang, G. Li, F. Tan, R. Liu, R. Li, T. Zhang, H. Jin and Q. Li, Carbon, 2014, 66, 369–376 CrossRef CAS
.
- K. A. Mirica, J. M. Azzarelli, J. G. Weis, J. M. Schnorr and T. M. Swager, Proc. Natl. Acad. Sci. U.S. A., 2013, 110, E3265–E3270 CrossRef CAS PubMed
.
- B. Yoon, S. F. Liu and T. M. Swager, Chem. Mater., 2016, 28, 5916–5924 CrossRef CAS
.
- S. F. Liu, S. Lin and T. M. Swager, ACS Sens., 2016, 1, 354–357 CrossRef CAS PubMed
.
- Y. Seekaew, W. Pon-On and C. Wongchoosuk, ACS Omega, 2019, 4, 16916–16924 CrossRef CAS PubMed
.
- J.-C. Chiou, C.-C. Wu and T.-M. Lin, Polymers, 2019, 11(3), 423 CrossRef PubMed
.
- T. D. Bennett, F.-X. Coudert, S. L. James and A. I. Cooper, Nat. Mater., 2021, 20, 1179–1187 CrossRef CAS PubMed
.
- S. J. Yang, J. Y. Choi, H. K. Chae, J. H. Cho, K. S. Nahm and C. R. Park, Chem. Mater., 2009, 21, 1893–1897 CrossRef CAS
.
- A. Huang, Q. Liu, N. Wang, Y. Zhu and J. Caro, J. Am. Chem. Soc., 2014, 136, 14686–14689 CrossRef CAS PubMed
.
- P. Wen, P. Gong, J. Sun, J. Wang and S. Yang, J. Mater. Chem. A, 2015, 3, 13874–13883 RSC
.
- J. H. Lee, S. Kang, J. Jaworski, K.-Y. Kwon, M. L. Seo, J. Y. Lee and J. H. Jung, Chem. – Eur. J., 2012, 18, 765–769 CrossRef CAS PubMed
.
- M. Saraf, R. Rajak and S. M. Mobin, J. Mater. Chem. A, 2016, 4, 16432–16445 RSC
.
- R. Sun, R. Lv, Y. Li, T. Du, L. Chen, Y. Zhang, X. Zhang, L. Zhang, H. Ma, H. Sun and Y. Qi, Food Control, 2023, 145, 109491 CrossRef CAS
.
- S. Horike, S. Shimomura and S. Kitagawa, Nat. Chem., 2009, 1, 695–704 CrossRef CAS PubMed
.
- P. Freund, L. Mielewczyk, M. Rauche, I. Senkovska, S. Ehrling, E. Brunner and S. Kaskel, ACS Sustainable Chem. Eng., 2019, 7, 4012–4018 CrossRef CAS
.
- M. Kalaj, K. C. Bentz, S. Ayala, Jr., J. M. Palomba, K. S. Barcus, Y. Katayama and S. M. Cohen, Chem. Rev., 2020, 120, 8267–8302 CrossRef CAS PubMed
.
- T. Uemura, N. Uchida, A. Asano, A. Saeki, S. Seki, M. Tsujimoto, S. Isoda and S. Kitagawa, J. Am. Chem. Soc., 2012, 134, 8360–8363 CrossRef CAS PubMed
.
- Q.-X. Wang and C.-Y. Zhang, Macromol. Rapid Commun., 2011, 32, 1610–1614 CrossRef CAS PubMed
.
- N. Yanai, T. Uemura, M. Ohba, Y. Kadowaki, M. Maesato, M. Takenaka, S. Nishitsuji, H. Hasegawa and S. Kitagawa, Angew. Chem., Int. Ed., 2008, 47, 9883–9886 CrossRef CAS PubMed
.
- T. Uemura, Y. Kadowaki, N. Yanai and S. Kitagawa, Chem. Mater., 2009, 21, 4096–4098 CrossRef CAS
.
- I. Dědek, V. Kupka, P. Jakubec, V. Šedajová, K. Jayaramulu and M. Otyepka, Appl. Mater. Today, 2022, 26, 101387 CrossRef
.
- C. Rösler and R. A. Fischer, CrystEngComm, 2015, 17, 199–217 RSC
.
- P.-H. Tong, L. Zhu, Y. Zang, J. Li, X.-P. He and T. D. James, Chem. Commun., 2021, 57, 12098–12110 RSC
.
- T. Hanawa, S. Kuwabata, H. Hashimoto and H. Yoneyama, Synth. Met., 1989, 30, 173–181 CrossRef CAS
.
- B. Le Ouay, M. Boudot, T. Kitao, T. Yanagida, S. Kitagawa and T. Uemura, J. Am. Chem. Soc., 2016, 138, 10088–10091 CrossRef CAS PubMed
.
- M. Shaik, V. K. Rao, A. K. Sinha, K. S. R. C. Murthy and R. Jain, J. Environ. Chem. Eng., 2015, 3, 1947–1952 CrossRef CAS
.
- S.-J. Choi, T.-H. Kwon, H. Im, D.-I. Moon, D. J. Baek, M.-L. Seol, J. P. Duarte and Y.-K. Choi, ACS Appl. Mater. Interfaces, 2011, 3, 4552–4556 CrossRef CAS PubMed
.
- D. Kwon, T.-I. Lee, J. Shim, S. Ryu, M. S. Kim, S. Kim, T.-S. Kim and I. Park, ACS Appl. Mater. Interfaces, 2016, 8, 16922–16931 CrossRef CAS PubMed
.
- P. Chowdhury, S. Mekala, F. Dreisbach and S. Gumma, Microporous Mesoporous Mater., 2012, 152, 246–252 CrossRef CAS
.
- K. Hwang, J. Ahn, I. Cho, K. Kang, K. Kim, J. Choi, K. Polychronopoulou and I. Park, ACS Appl. Mater. Interfaces, 2020, 12, 13338–13347 CrossRef CAS PubMed
.
- A. Cadiau, J. S. Lee, D. Damasceno Borges, P. Fabry, T. Devic, M. T. Wharmby, C. Martineau, D. Foucher, F. Taulelle, C.-H. Jun, Y. K. Hwang, N. Stock, M. F. De Lange, F. Kapteijn, J. Gascon, G. Maurin, J.-S. Chang and C. Serre, Adv. Mater., 2015, 27, 4775–4780 CrossRef CAS PubMed
.
- A. K. Geim, Science, 2009, 324, 1530–1534 CrossRef CAS PubMed
.
- V. Georgakilas, M. Otyepka, A. B. Bourlinos, V. Chandra, N. Kim, K. C. Kemp, P. Hobza, R. Zboril and K. S. Kim, Chem. Rev., 2012, 112, 6156–6214 CrossRef CAS PubMed
.
- K. S. Novoselov, V. I. Fal'ko, L. Colombo, P. R. Gellert, M. G. Schwab and K. Kim, Nature, 2012, 490, 192–200 CrossRef CAS PubMed
.
- C. Tan, X. Cao, X.-J. Wu, Q. He, J. Yang, X. Zhang, J. Chen, W. Zhao, S. Han, G.-H. Nam, M. Sindoro and H. Zhang, Chem. Rev., 2017, 117, 6225–6331 CrossRef CAS PubMed
.
- K. Jayaramulu, K. K. R. Datta, C. Roesler, M. Petr, M. Otyepka, R. Zboril and R. A. Fischer, Angew. Chem., Int. Ed., 2016, 55, 1178–1182 CrossRef CAS PubMed
.
- K. Jayaramulu, D. P. Dubal, A. Schneemann, V. Ranc, C. Perez-Reyes, J. Straska, S. Kment, M. Otyepka, R. A. Fischer and R. Zboril, Adv. Funct. Mater., 2019, 29, 1902539 CrossRef
.
- K. Jayaramulu, F. Geyer, M. Petr, R. Zboril, D. Vollmer and R. A. Fischer, Adv. Mater., 2017, 29, 1605307 CrossRef PubMed
.
- K. Jayaramulu, F. Geyer, A. Schneemann, S. Kment, M. Otyepka, R. Zboril, D. Vollmer and R. A. Fischer, Adv. Mater., 2019, 31, 1900820 CrossRef PubMed
.
- K. Jayaramulu, M. Horn, A. Schneemann, H. Saini, A. Bakandritsos, V. Ranc, M. Petr, V. Stavila, C. Narayana, B. Scheibe, S. Kment, M. Otyepka, N. Motta, D. Dubal, R. Zboril and R. A. Fischer, Adv. Mater., 2021, 33, 2004560 CrossRef CAS PubMed
.
- K. Jayaramulu, J. Masa, O. Tomanec, D. Peeters, V. Ranc, A. Schneemann, R. Zboril, W. Schuhmann and R. A. Fischer, Adv. Funct. Mater., 2017, 27, 201770196 CrossRef
.
- K. Jayaramulu, S. Mukherjee, D. M. Morales, D. P. Dubal, A. K. Nanjundan, A. Schneemann, J. Masa, S. Kment, W. Schuhmann, M. Otyepka, R. Zboril and R. A. Fischer, Chem. Rev., 2022, 122, 17241–17338 CrossRef CAS PubMed
.
- R. Kumar, K. Jayaramulu, T. K. Maji and C. N. R. Rao, Chem. Commun., 2013, 49, 4947–4949 RSC
.
- R. Kumar, K. Jayaramulu, T. K. Maji and C. N. R. Rao, Dalton Trans., 2014, 43, 7383–7386 RSC
.
- M. Majumder, H. Saini, I. Dedek, A. Schneemann, N. R. Chodankar, V. Ramarao, M. S. Santosh, A. K. Nanjundan, S. Kment, D. Dubal, M. Otyepka, R. Zboril and K. Jayaramulu, ACS Nano, 2021, 15, 17275–17298 CrossRef CAS PubMed
.
- H. Saini, N. Srinivasan, V. Sedajova, M. Majumder, D. P. Dubal, M. Otyepka, R. Zboril, N. Kurra, R. A. Fischer and K. Jayaramulu, ACS Nano, 2021, 15, 18742–18776 CrossRef CAS PubMed
.
- E. Vermisoglou, D. Panacek, K. Jayaramulu, M. Pykal, I. Frebort, M. Kolar, M. Hajduch, R. Zboril and M. Otyepka, Biosens. Bioelectron., 2020, 166, 112436 CrossRef CAS PubMed
.
- Y.-Y. Yin, Y. Xing, M.-W. Li, Y.-N. Li, J.-N. Wang, T. Li and L.-X. Zhang, Inorg. Chem. Commun., 2018, 97, 49–55 CrossRef CAS
.
- M.-S. Yao, J.-W. Xiu, Q.-Q. Huang, W.-H. Li, W.-W. Wu, A.-Q. Wu, L.-A. Cao, W.-H. Deng, G.-E. Wang and G. Xu, Angew. Chem., Int. Ed., 2019, 58, 14915–14919 CrossRef CAS PubMed
.
- X. Chen, Y. Lu, J. Dong, L. Ma, Z. Yi, Y. Wang, L. Wang, S. Wang, Y. Zhao, J. Huang and Y. Liu, ACS Appl. Mater. Interfaces, 2020, 12, 57235–57244 CrossRef CAS PubMed
.
- M.-S. Yao, P. Wang, Y.-F. Gu, T. Koganezawa, H. Ashitani, Y. Kubota, Z.-M. Wang, Z.-Y. Fan, K.-I. Otake and S. Kitagawa, Dalton Trans., 2021, 50, 13236–13245 RSC
.
- Y. Yue, P. Cai, X. Xu, H. Li, H. Chen, H.-C. Zhou and N. Huang, Angew. Chem., Int. Ed., 2021, 60, 10806–10813 CrossRef CAS PubMed
.
- A.-Q. Wu, W.-Q. Wang, H.-B. Zhan, L.-A. Cao, X.-L. Ye, J.-J. Zheng, P. N. Kumar, K. Chiranjeevulu, W.-H. Deng, G.-E. Wang, M.-S. Yao and G. Xu, Nano Res., 2021, 14, 438–443 CrossRef CAS
.
- S. Park, Z. Zhang, H. Qi, B. Liang, J. Mahmood, H.-J. Noh, M. Hambsch, M. Wang, M. Wang, K. H. Ly, Z. Wang, I. M. Weidinger, S. Zhou, J.-B. Baek, U. Kaiser, S. C. B. Mannsfeld, X. Feng and R. Dong, ACS Mater. Lett., 2022, 4, 1146–1153 CrossRef CAS
.
- D. H. Yang, T. T. T. Nguyen, S. T. Navale, L. H. T. Nguyen, Y. T. Dang, N. X. D. Mai, T. B. Phan, J.-Y. Kim, T. L. H. Doan, S. S. Kim and H. W. Kim, Sens. Actuators, B, 2022, 368, 132120 CrossRef CAS
.
- Z. Xue, J.-J. Zheng, Y. Nishiyama, M.-S. Yao, Y. Aoyama, Z. Fan, P. Wang, T. Kajiwara, Y. Kubota, S. Horike, K.-I. Otake and S. Kitagawa, Angew. Chem., Int. Ed., 2023, 62, e202215234 CrossRef CAS PubMed
.
- Y. Wang, Y. Lü, W. Zhan, Z. Xie, Q. Kuang and L. Zheng, J. Mater. Chem. A, 2015, 3, 12796–12803 RSC
.
- M.-S. Yao, W.-X. Tang, G.-E. Wang, B. Nath and G. Xu, Adv. Mater., 2016, 28, 5229–5234 CrossRef CAS PubMed
.
- W.-T. Koo, S.-J. Choi, S.-J. Kim, J.-S. Jang, H. L. Tuller and I.-D. Kim, J. Am. Chem. Soc., 2016, 138, 13431–13437 CrossRef CAS PubMed
.
- W.-T. Koo, J.-S. Jang, S.-J. Choi, H.-J. Cho and I.-D. Kim, ACS Appl. Mater. Interfaces, 2017, 9, 18069–18077 CrossRef CAS PubMed
.
- W.-T. Koo, S. Yu, S.-J. Choi, J.-S. Jang, J. Y. Cheong and I.-D. Kim, ACS Appl. Mater. Interfaces, 2017, 9, 8201–8210 CrossRef CAS PubMed
.
- X.-Z. Song, Y.-L. Meng, Z. Tan, L. Qiao, T. Huang and X.-F. Wang, Inorg. Chem., 2017, 56, 13646–13650 CrossRef CAS PubMed
.
- J.-S. Jang, W.-T. Koo, S.-J. Choi and I.-D. Kim, J. Am. Chem. Soc., 2017, 139, 11868–11876 CrossRef CAS PubMed
.
- T. Zhou, Y. Sang, X. Wang, C. Wu, D. Zeng and C. Xie, Sens. Actuators, B, 2018, 258, 1099–1106 CrossRef CAS
.
- W.-T. Koo, S.-J. Choi, J.-S. Jang and I.-D. Kim, Sci. Rep., 2017, 7, 45074 CrossRef CAS PubMed
.
- J.-S. Jang, W.-T. Koo, D.-H. Kim and I.-D. Kim, ACS Cent. Sci., 2018, 4, 929–937 CrossRef CAS PubMed
.
- Y.-M. Jo, T.-H. Kim, C.-S. Lee, K. Lim, C. W. Na, F. Abdel-Hady, A. A. Wazzan and J.-H. Lee, ACS Appl. Mater. Interfaces, 2018, 10, 8860–8868 CrossRef CAS PubMed
.
- W.-T. Koo, J.-H. Cha, J.-W. Jung, S.-J. Choi, J.-S. Jang, D.-H. Kim and I.-D. Kim, Adv. Funct. Mater., 2018, 28, 1802575 CrossRef
.
- T. Zhang, X. Tang, J. Zhang, T. Zhou, H. Wang, C. Wu, X. Xia, C. Xie and D. Zeng, Langmuir, 2018, 34, 14577–14585 CrossRef CAS PubMed
.
- H. Yuan, S. A. A. A. Aljneibi, J. Yuan, Y. Wang, H. Liu, J. Fang, C. Tang, X. Yan, H. Cai, Y. Gu, S. J. Pennycook, J. Tao and D. Zhao, Adv. Mater., 2019, 31, 1807161 CrossRef PubMed
.
- M.-S. Yao, L.-A. Cao, Y.-X. Tang, G.-E. Wang, R.-H. Liu, P. N. Kumar, G.-D. Wu, W.-H. Deng, W.-J. Hong and G. Xu, J. Mater. Chem. A, 2019, 7, 18397–18403 RSC
.
- Z. Yang, D. Zhang and H. Chen, Sens. Actuators, B, 2019, 300, 127037 CrossRef CAS
.
- X. Wu, S. Xiong, Y. Gong, Y. Gong, W. Wu, Z. Mao, Q. Liu, S. Hu and X. Long, Sens. Actuators, B, 2019, 292, 32–39 CrossRef CAS
.
- Z. Li, Y. Zhang, H. Zhang, Y. Jiang and J. Yi, ACS Appl. Mater. Interfaces, 2020, 12, 37489–37498 CrossRef CAS PubMed
.
- T. T. Tung, M. T. Tran, J.-F. Feller, M. Castro, T. Van Ngo, K. Hassan, M. J. Nine and D. Losic, Carbon, 2020, 159, 333–344 CrossRef CAS
.
- X. Wang, S. Li, L. Xie, X. Li, D. Lin and Z. Zhu, Ceram. Int., 2020, 46, 15858–15866 CrossRef CAS
.
- S. Xu, X. Liu, J. Wu and J. Wu, ACS Sens., 2023, 8, 2348–2358 CrossRef CAS PubMed
.
- X. Liu, D. Huang, C. Lai, G. Zeng, L. Qin, H. Wang, H. Yi, B. Li, S. Liu, M. Zhang, R. Deng, Y. Fu, L. Li, W. Xue and S. Chen, Chem. Soc. Rev., 2019, 48, 5266–5302 RSC
.
- K. Geng, T. He, R. Liu, S. Dalapati, K. T. Tan, Z. Li, S. Tao, Y. Gong, Q. Jiang and D. Jiang, Chem. Rev., 2020, 120, 8814–8933 CrossRef CAS PubMed
.
- A. P. Côté, A. I. Benin, N. W. Ockwig, M. O'Keeffe, A. J. Matzger and O. M. Yaghi, Science, 2005, 310, 1166–1170 CrossRef PubMed
.
- S. Y. Ding and W. Wang, Chem. Soc. Rev., 2013, 42, 548–568 RSC
.
- H. M. El-Kaderi, J. R. Hunt, J. L. Mendoza-Cortés, A. P. Côté, R. E. Taylor, M. O'Keeffe and O. M. Yaghi, Science, 2007, 316, 268–272 CrossRef CAS PubMed
.
- X. Feng, X. Ding and D. Jiang, Chem. Soc. Rev., 2012, 41, 6010–6022 RSC
.
- S. Lin, C. S. Diercks, Y. B. Zhang, N. Kornienko, E. M. Nichols, Y. Zhao, A. R. Paris, D. Kim, P. Yang, O. M. Yaghi and C. J. Chang, Science, 2015, 349, 1208–1213 CrossRef CAS PubMed
.
- P. J. Waller, F. Gándara and O. M. Yaghi, Acc. Chem. Res., 2015, 48, 3053–3063 CrossRef CAS PubMed
.
- F. Xie, C. Bie, J. Sun, Z. Zhang and B. Zhu, J. Mater. Sci. Technol., 2024, 170, 87–94 CrossRef
.
- Y. Xu, S. Jin, H. Xu, A. Nagai and D. Jiang, Chem. Soc. Rev., 2013, 42, 8012–8031 RSC
.
- H. Singh, V. K. Tomer, N. Jena, I. Bala, N. Sharma, D. Nepak, A. De Sarkar, K. Kailasam and S. K. Pal, J. Mater. Chem. A, 2017, 5, 21820–21827 RSC
.
- Z. Meng, R. M. Stolz and K. A. Mirica, J. Am. Chem. Soc., 2019, 141, 11929–11937 CrossRef CAS PubMed
.
- Y. Yue, H. Li, H. Chen and N. Huang, J. Am. Chem. Soc., 2022, 144, 2873–2878 CrossRef CAS PubMed
.
- A. Mei, W. Chen, Z. Yang, M. Zhou, W. Jin, S. Yang, K. Chen and Y. Liu, Angew. Chem., Int. Ed., 2023, 62, e202301440 CrossRef CAS PubMed
.
- S. J. Pomfret, P. N. Adams, N. P. Comfort and A. P. Monkman, Polymer, 2000, 41, 2265–2269 CrossRef CAS
.
- A. L. Pang, A. Arsad and M. Ahmadipour, Polym. Adv. Technol., 2021, 32, 1428–1454 CrossRef CAS
.
- A. G. MacDiarmid and A. J. Epstein, Synth. Met., 1994, 65, 103–116 CrossRef CAS
.
- M. Liu, Y.-J. Chen, X. Huang, L.-Z. Dong, M. Lu, C. Guo, D. Yuan, Y. Chen, G. Xu, S.-L. Li and Y.-Q. Lan, Angew. Chem., Int. Ed., 2022, 61, e202115308 CrossRef PubMed
.
- Y.-J. Chen, Y.-Y. Wen, W.-H. Li, Z.-H. Fu, G.-E. Wang and G. Xu, Nano Lett., 2023, 23, 3614–3622 CrossRef CAS PubMed
.
- A. J. Bard, J. Photochem., 1979, 10, 59–75 CrossRef CAS
.
- V. Krishnaveni, M. Esclance Dmello, P. Sahoo, N. Thokala, V. R. Bakuru, K. Vankayala, K. Basavaiah and S. B. Kalidindi, ACS Appl. Nano Mater., 2023, 6, 10960–10966 CrossRef CAS
.
- Y. L. Wong, J. M. Tobin, Z. Xu and F. Vilela, J. Mater. Chem. A, 2016, 4, 18677–18686 RSC
.
- S. Wang, H. Li, H. Huang, X. Cao, X. Chen and D. Cao, Chem. Soc. Rev., 2022, 51, 2031–2080 RSC
.
- M. Barawi, L. Collado, M. Gomez-Mendoza, F. E. Oropeza, M. Liras and V. A. de la Peña O'Shea, Adv. Energy Mater., 2021, 11, 2101530 CrossRef CAS
.
- F. M. Wisser, J. Grothe and S. Kaskel, Sens. Actuators, B, 2016, 223, 166–171 CrossRef CAS
.
- K. Yang, W. Yuan, Z. Hua, Y. Tang, F. Yin and D. Xia, ACS Appl. Mater. Interfaces, 2020, 12, 3919–3927 CrossRef CAS PubMed
.
- S. Maiti, B. Mandal, M. Sharma, S. Mukherjee and A. K. Das, Chem. Commun., 2020, 56, 9348–9351 RSC
.
- W. C. Ko, M.-S. Kim, Y. J. Kwon, J. Jeong, W. R. Kim, H. Choi, J. K. Park and Y. K. Jeong, J. Mater. Chem. A, 2020, 8, 19246–19253 RSC
.
- N. Sharma, N. Sharma, P. Srinivasan, S. Kumar, J. B. Balaguru Rayappan and K. Kailasam, J. Mater. Chem. A, 2018, 6, 18389–18395 RSC
.
- F. Niu, Z.-W. Shao, L.-M. Tao and Y. Ding, Sens. Actuators, B, 2020, 321, 128513 CrossRef CAS
.
- R.-B. Lin, Y. He, P. Li, H. Wang, W. Zhou and B. Chen, Chem. Soc. Rev., 2019, 48, 1362–1389 RSC
.
- B. Wang, R.-B. Lin, Z. Zhang, S. Xiang and B. Chen, J. Am. Chem. Soc., 2020, 142, 14399–14416 CrossRef CAS PubMed
.
- X. Song, Y. Wang, C. Wang, D. Wang, G. Zhuang, K. O. Kirlikovali, P. Li and O. K. Farha, J. Am. Chem. Soc., 2022, 144, 10663–10687 CrossRef CAS PubMed
.
- Y. Wang, D. Liu, J. Yin, Y. Shang, J. Du, Z. Kang, R. Wang, Y. Chen, D. Sun and J. Jiang, Chem. Commun., 2020, 56, 703–706 RSC
.
- W.-G. Lee, T.-U. Yoon, Y.-S. Bae, K. S. Kim and S. B. Baek, RSC Adv., 2019, 9, 36808–36814 RSC
.
- D. Akinwande, N. Petrone and J. Hone, Nat. Commun., 2014, 5, 5678 CrossRef CAS PubMed
.
- G. R. Bhimanapati, Z. Lin, V. Meunier, Y. Jung, J. Cha, S. Das, D. Xiao, Y. Son, M. S. Strano, V. R. Cooper, L. Liang, S. G. Louie, E. Ringe, W. Zhou, S. S. Kim, R. R. Naik, B. G. Sumpter, H. Terrones, F. Xia, Y. Wang, J. Zhu, D. Akinwande, N. Alem, J. A. Schuller, R. E. Schaak, M. Terrones and J. A. Robinson, ACS Nano, 2015, 9, 11509–11539 CrossRef CAS PubMed
.
- S. Z. Butler, S. M. Hollen, L. Cao, Y. Cui, J. A. Gupta, H. R. Gutierrez, T. F. Heinz, S. S. Hong, J. Huang, A. F. Ismach, E. Johnston-Halperin, M. Kuno, V. V. Plashnitsa, R. D. Robinson, R. S. Ruoff, S. Salahuddin, J. Shan, L. Shi, M. G. Spencer, M. Terrones, W. Windl and J. E. Goldberger, ACS Nano, 2013, 7, 2898–2926 CrossRef CAS PubMed
.
- W. Choi, N. Choudhary, G. H. Han, J. Park, D. Akinwande and Y. H. Lee, Mater. Today, 2017, 20, 116–130 CrossRef CAS
.
- N. Mounet, M. Gibertini, P. Schwaller, D. Campi, A. Merkys, A. Marrazzo, T. Sohier, I. E. Castelli, A. Cepellotti, G. Pizzi and N. Marzari, Nat. Nanotechnol., 2018, 13, 246–252 CrossRef CAS PubMed
.
- B. Radisavljevic, A. Radenovic, J. Brivio, V. Giacometti and A. Kis, Nat. Nanotechnol., 2011, 6, 147–150 CrossRef CAS PubMed
.
- Y. Shao, J. Wang, H. Wu, J. Liu, I. A. Aksay and Y. Lin, Electroanalysis, 2010, 22, 1027–1036 CrossRef CAS
.
- Y. Zhang, Y.-W. Tan, H. L. Stormer and P. Kim, Nature, 2005, 438, 201–204 CrossRef CAS PubMed
.
- A. Botta, W. De Donato, V. Persico and A. Pescapé, Future Gener. Comput. Syst., 2016, 56, 684–700 CrossRef
.
- A. G. Frank, L. S. Dalenogare and N. F. Ayala, Int. J. Prod. Econ., 2019, 210, 15–26 CrossRef
.
- J. Gubbi, R. Buyya, S. Marusic and M. Palaniswami, Future Gener. Comput. Syst., 2013, 29, 1645–1660 CrossRef
.
- S. M. R. Islam, D. Kwak, M. H. Kabir, M. Hossain and K. S. Kwak, IEEE Access, 2015, 3, 678–708 Search PubMed
.
- M. A. Khan and K. Salah, Future Gener. Comput. Syst., 2018, 82, 395–411 CrossRef
.
- S. Li, L. D. Xu and S. Zhao, Inf. Syst. Front., 2015, 17, 243–259 CrossRef
.
- J. Lin, W. Yu, N. Zhang, X. Yang, H. Zhang and W. Zhao, IEEE Internet Things J., 2017, 4, 1125–1142 Search PubMed
.
- Y. Mao, J. Zhang and K. B. Letaief, IEEE J. Sel. Areas Commun., 2016, 34, 3590–3605 Search PubMed
.
- R. Mijumbi, J. Serrat, J. L. Gorricho, N. Bouten, F. De Turck and R. Boutaba, IEEE Commun. Surv. Tutor., 2016, 18, 236–262 Search PubMed
.
- M. Mozaffari, W. Saad, M. Bennis, Y. H. Nam and M. Debbah, IEEE Commun. Surv. Tutor., 2019, 21, 2334–2360 Search PubMed
.
- C. Perera, A. Zaslavsky, P. Christen and D. Georgakopoulos, IEEE Commun. Surv. Tutor., 2014, 16, 414–454 Search PubMed
.
- U. Raza, P. Kulkarni and M. Sooriyabandara, IEEE Commun. Surv. Tutor., 2017, 19, 855–873 Search PubMed
.
- M. Satyanarayanan, Computer, 2017, 50, 30–39 Search PubMed
.
- E. Sisinni, A. Saifullah, S. Han, U. Jennehag and M. Gidlund, IEEE Trans. Ind. Inform., 2018, 14, 4724–4734 Search PubMed
.
- J. A. Stankovic, IEEE Internet Things J., 2014, 1, 3–9 Search PubMed
.
- F. Tao, H. Zhang, A. Liu and A. Y. C. Nee, IEEE Trans. Ind. Inform., 2019, 15, 2405–2415 Search PubMed
.
- L. D. Xu, E. L. Xu and L. Li, Int. J. Prod. Res., 2018, 56, 2941–2962 CrossRef
.
- A. Zanella, N. Bui, A. Castellani, L. Vangelista and M. Zorzi, IEEE Internet Things J., 2014, 1, 22–32 Search PubMed
.
- R. Y. Zhong, X. Xu, E. Klotz and S. T. Newman, Engineering, 2017, 3, 616–630 CrossRef
.
- J. Liu, G. Han, D. Zhao, K. Lu, J. Gao and T.-S. Chung, Sci. Adv., 2020, 6, eabb1110 CrossRef CAS PubMed
.
- R. Shevate and D. L. Shaffer, ACS Nano, 2022, 16, 2407–2418 CrossRef CAS PubMed
.
- A. Giri, G. Shreeraj, T. K. Dutta and A. Patra, Angew. Chem., Int. Ed., 2023, 62, e202219083 CrossRef CAS PubMed
.
|
This journal is © The Royal Society of Chemistry 2024 |
Click here to see how this site uses Cookies. View our privacy policy here.