DOI:
10.1039/D4RA04454A
(Paper)
RSC Adv., 2024,
14, 28569-28584
Dual inhibitory potential of ganoderic acid A on GLUT1/3: computational and in vitro insights into targeting glucose metabolism in human lung cancer†
Received
18th June 2024
, Accepted 7th August 2024
First published on 6th September 2024
Abstract
Human glucose transporters (GLUTs) facilitate the uptake of hexoses into cells. In cancer, the increased proliferation necessitates higher expression of GLUTs, with particular emphasis on GLUT1 and GLUT3. Thus, inhibiting GLUTs holds promise as an anticancer therapy by starving these cells of fuel. Ganoderic acid A (GAA), a triterpene found in Ganoderma lucidum, has anticancer and antidiabetic properties. Recent studies show that GAA reduces glucose uptake in cancer cells, which indicates that GAA may affect GLUT1/GLUT3 by inhibiting glucose uptake. Therefore, this study aimed to inspect whether GAA could target GLUT1/GLUT3 and play an inhibitory role in changing their endofacial and exofacial conformations. To this end, AlphaFold2 was employed to model the endofacial and exofacial conformations of GLUT3 and GLUT1, respectively. Molecular docking, molecular dynamics simulation, cell viability, cellular thermal shift assays (CETSA), glucose uptake, qPCR, and western blotting were harnessed. In comparison to the endofacial (cytochalasin B) and exofacial (phloretin) GLUT1/3 inhibitors, the computational findings unveiled GAA's capacity to bind and stabilize GLUT1/3 in their two conformational states, with a preference for binding the endofacial conformation. A low, non-cytotoxic dose of GAA thermally stabilized both transporters and inhibited glucose uptake in human lung cancer cells, similar to cytochalasin B and phloretin. In conclusion, this study has unearthed novel functionalities of GAA, suggesting its potential utility in cancer therapy by targeting glucose metabolism.
1. Introduction
Cancer cells require a significant amount of energy to sustain their rapid growth and division. To meet this energy demand, they rely more on anaerobic glycolysis pathways than on mitochondrial oxidative phosphorylation, in contrast to noncancer cells. This phenomenon is commonly referred to as the Warburg effect.1 Furthermore, cancer cells upregulate the expression of glycolysis enzymes and glucose transporters, both of which correlate with the invasiveness and metastatic potential of cancers.2–4 Among these transporters, members of the glucose transporter (GLUT) family have been confirmed to be upregulated in malignant tumors.5 For instance, non-specific glucose and fructose transporters GLUT2, 5, and 12 have been identified to be linked with different cancers.6–8 On the other hand, GLUT1 is found to be the main factor of the Warburg effect, which is the main carrier of glucose transmembrane transport.9 Additionally, GLUT3, a high-affinity glucose transporter, plays a crucial role in facilitating glucose entry into neurons. The elevated expression of both GLUT1 and GLUT3 has been observed in various cancer types,10 including lung cancer.11,12 As a result, targeting GLUTs has emerged as a potential strategy for cancer treatment.13–15
Many GLUT inhibitors are small molecules, primarily from natural and semi-natural sources, belonging to various chemical categories such as alkaloids, flavonoids, and terpenoids.16,17 Triterpenes represent secondary metabolites that are widely used for phytochemical and pharmacological investigations. In the context of energy metabolism, recent studies indicated that triterpenes target several critical proteins in glucose metabolism, making them promising therapeutic agents to treat Diabetes Mellitus (DM). For instance, studies have shown that triterpenes have an inhibitory effect on aldose reductase, Glyceraldehyde-3-Phosphate Dehydrogenase (GAPDH), and glycogen phosphorylase.18 Ganoderic acid (GA) is a type of triterpene naturally found in Ganoderma lucidum, a medicinal mushroom used in traditional Chinese medicine for centuries. GA has several isoforms, including ganoderic acid A (GAA). The anticancer and antidiabetic properties of certain GA isoforms including GAA have been reported in previous studies.19–23 For instance, studies have reported that GAA exhibits a protective effect against streptozotocin-induced diabetes in rats, regulates genes associated with metabolism in the liver, and alters energy metabolism in colon cancer by inhibiting glucose uptake, as well as the production of lactate and pyruvate.24–26 Despite this, the potential role of GAA to inhibit GLUT in cancer cells remains to be testified.
Given the capacity of GA to reduce glucose uptake in cancer, we investigated the ability of GAA to bind and stabilize the endofacial conformations of GLUT1/3 and compared it with the standard endofacial inhibitor. Moreover, we also targeted the exofacial conformations of both transporters to investigate the possibility of GAA as a drug conjugator. Toward this goal, we modeled the endofacial and exofacial conformations of GLUT3 and GLUT1, respectively as a surrogate to the crystal structures using AlphaFold2. Initially, our in vitro assay confirmed GAA's capacity to inhibit glucose uptake in human lung cancer. Subsequently, in silico assay disclosed GAA's affinity for binding to GLUT1/3, a finding substantiated by the cellular thermal stability tests. Consequently, this study unveils novel functions of GAA as a potential inhibitor for glucose transporters 1 and 3, suggesting a potential reprogramming of glucose metabolism in human lung cancer.
2. Methods
2.1 Materials
A549 and H1299 cell lines were obtained from the American Type Cultures Collection (ATCC, Manassas, VA). GAA was purchased from Linyi Azeroth Bioscience Co., Ltd. Other chemicals purchased include: DMEM medium (HyClone, USA), DMEM with high glucose medium (Sevicebio; G4511-500ML), DMEM without glucose (Servicebio; G4528-500ML), Trypsin-EDTA (CORNING; 25-053-Cl), DMSO (MP Biomedicals; 196
055), CCK-8 (Dojindo, Japan), and FBS (ExCell Bio). Cytochalasin B (HY-16928), phloretin (HY-N0142) was purchased from MedChemExpress, and glucose assay kit-WST (G264) was purchased from Dojindo Molecular Technologies, Inc. In the experiments, we utilized Hifair™ V one-step RT-gDNA digestion SuperMix (Yeasen, 11142-A/B/C), SYBR Green Master Mix (Yeasen, 11184ES03), RIPA lysate (Beyotime, Shanghai, China), Protease Inhibitor Cocktail Tablets (Roche; 11
697
498
001), Protein quantification kit (Abbkine, KTD3001), Skim Milk powder (Solarbio; D8340), SDS-PAGE (Shanghai Life-iLab Bio-Technology; LFB23021), Nitrocellulose filter membrane (Pharmacia; WP4HY00010), and western blotting antibody dilution buffer (Elabscience; E-IR-R121). The polyclonal antibodies for GLUT1 (Cat No. 21829-1-AP), and GLUT3 (Cat No. A5515) were purchased from Proteintech and Selleckchem, respectively. In the research, we also used ECL Kit (Epizyme; SQ202), and HRP conjugated Affinity pure goat Anti-rabbit IgG (H + L) (Boster Biological Technology; lot: BST16I03AI8B56).
2.2 Modeling of GLUT1exo and GLUT3endo protein structure
At the time, no crystal structures were illustrating GLUT1 in an outward-open or GLUT3 in an inward-open conformation, so AlphaFold2 was employed. The protein sequences for GLUT1 and GLUT3 were obtained from UniProt with the IDs P11166 and P11169, respectively, for this investigation. The outward-open conformation of GLUT3 protein structure labeled with PDB ID: 4ZW9 has a resolution of 1.5 Å and contains a single mutation (N43T).27 To generate the WT model, this mutation was inverted using the Chimera mutagenesis tool. Subsequently, AlphaFold2 online service,28 integrated with the ChimeraX software,29 was harnessed to build the GLUT1 model in an out-ward open conformation using GLUT3 (PDB ID: 4ZW9) as a template, and the GLUT3 model in an inward-open conformation using GLUT1 (PDB ID: 5EQG) as a reference. The predicted models were then validated with the SAVES Server 6.0.30
2.3 Protein and ligands preparation
In SDF format, ganoderic acid A, cytochalasin B, and phloretin were retrieved from PubChem (https://pubchem.ncbi.nlm.nih.gov/compound/) compound database; furthermore, using the PyRx 0.8 virtual tool, the energy of the ligands was minimized by applying default settings. These settings comprised the universal force field as the force field, the conjugate optimization algorithm, a total of 200 steps with 1 step for updates, and a stopping criterion based on an energy difference of less than 0.1. After the energy minimization process, the compounds were converted to the ‘pdbqt’ file format.
The crystal structure of the inward-open conformation of GLUT1 (PDB ID 5EQG) and outward-open conformation of GLUT3 (PDB ID 4ZW9) were downloaded from the RCSB protein data bank (PDB) database and saved in PDB format. The downloaded PDB files of the target proteins were prepared using PyMol. For the GLUT1 and GLUT3 models, a quick prep in MOE 2015.10 was used to minimize and optimize the proteins. Then, the PDB files were prepared by removing the water molecules, co-crystal molecules, and heat atoms, inserting partial charges, and adding polar hydrogen to the 3-D protein moiety using the Autodock vina virtual tool. Subsequently, the PDB file was converted into a pdbqt file.
2.4 Binding pocket identification and molecular docking analysis
A binding pocket in a protein refers to a specific site on its surface or interior that can tightly bind to a ligand. The shape, location, and chemical properties of the binding pocket are determined by the specific amino acid residues that surround it. Together, these factors determine the binding pocket's overall functionality and how effectively it can interact with other molecules.31 Therefore, GLUT1/3 binding pockets, their volume, and XYZ dimensions were identified by uploading the PDB file of either the models or the crystal structures to (http://prankweb.cz/).32 Based on Prankweb's ranking, pocket 1 was selected for docking, and the grid box was assigned accordingly. The XYZ dimensions for the grid box of the studied proteins were set as follows (X: 582.68 Y: −27.03 Z: 279.73 for GLUT1endo, X: −6.11 Y: −0.81 Z: 5.16 for GLUT1exo, X: 5.48 Y: −4.43 Z: −7.15 for GLUT3endo, and X: 59.43 Y: 15.99 Z: 61.02 for GLUT3exo) with pocket volume 2582.8, 2281.1, 1019.9, and 2414.6 Å3 for pocket one in GLUT1endo, GLUT1exo, GLUT3endo, and GLUT3exo, respectively, while the residues of these pockets are shown in Table S1.†
Molecular docking is an essential method in structural biology, commonly employed in computer-aided drug design (CADD). The technique enables the prediction of the most favorable binding mode between a targeted macromolecule and a small molecule, such as a drug.33 To identify the binding mode of the target proteins with the selected ligands, molecular docking was separately performed using AutoDock Vina 1.1.2 option of PyRx 0.8 virtual screening tool.34 AutoDock Vina version 1.1.2 which is implemented in PyRx versions 0.8 as well as 0.9.7 has been extensively validated and widely used in the scientific community.35 To ensure the reliability and accuracy of the docking results, Autodock Vina in SwissDock 2024
36 using the Vina Python library (version 1.2.5) was harnessed. The PDB protein files and ligand SMILES were uploaded to https://www.swissdock.ch/ server. Docking was performed using the previously defined binding pockets, specified by XYZ coordinates and a 20 × 20 × 20 Å box size. The sampling method used was exhaustive, with a value of 4. Ligands (GAA, cytochalasin B, and phloretin) generated multiple docking poses (up to nine poses). The first pose of each ligand with the most negative binding energy (kcal mol−1) was selected for further evaluation. Protein–ligand interaction profiler37 and PyMol version 3.0 were used to visualize the interaction between ligand–protein complexes.
2.5 Molecular dynamics (MD) simulation
To determine the stability of binding, conformational changes, and interaction mechanisms of the chosen ligands (GAA, cytochalasin B, and phloretin), MD simulation was conducted on GLUT1 and GLUT3. The ligand–protein complex files underwent molecular dynamics studies using the default protocol of the Schrodinger Desmond module.38 The Desmond System Builder tool was utilized to create the water-soaked solvated system. The TIP3P model was adopted as the solvation system for this experiment. The orthorhombic simulation box has periodic boundary conditions at 10 Å from the outermost portion of the protein surface. The water-soaked solvated system was rendered neutral by the addition of the necessary counterions. By infusing the simulation setup with 0.15 M NaCl, a state of isotonic balance was established. Before the start of the simulation, the system was allowed to pass through an equilibrium phase, ensuring it reached a stable condition. For a duration of 100 and/or 200 ns, the simulation was executed at a temperature of 310 K and an ambient pressure of 1.013 bar. The force field parameters were generated using the Force Field Builder module within the Schrödinger Desmond software, with the OPLS3 force field utilized.39 The trajectory obtained from the simulations was analyzed using Desmond, VMD, and PyMOL.40,41 To conduct the principal component analysis, we transformed the simulation trajectory into a format compatible with Bio3D on the ‘R’ program platform.42,43 MM-GBSA analysis was performed utilizing the thermal_MMGBSA.py script from the Prime Desmond module in the Schrödinger suite.41 The analysis involved calculating the free binding energy by generating 0–1000 frames. For the solvent model, the VSGB 2.0 new energy model was chosen, and the force field selected was OPLS-2005. The remaining settings were kept at their default values. The MM-GBSA calculation of 100 ns MDS data involved processing and analyzing 200 frames. Subsequently, the binding free energies (in kcal mol−1) were computed.44,45
2.6 Cell viability assay
Human lung cancer cell lines (A549 and H1299) were cultured in DMEM medium with 10% fetal bovine serum and 1% penicillin/streptomycin at 37 °C in 5% CO2 for 24 hours. For cell viability experiments, approximately 7 × 104 cells were seeded in a 96-well plate and incubated under the same conditions. Following this, the cells were treated with varying concentrations of GAA (0.5, 2.5, 5, 10, and 20 μM) for 24 hours. Subsequently, 10 μl of CCK-8 solution was added to each well, and the plate was incubated for an additional hour at 37 °C. Absorbance was measured at 450 nm using a SpectraMax M5 microplate reader (Molecular Devices, USA).
2.7 Glucose consumption
The glucose concentrations in cell medium supernatants were measured as previously described.46 Briefly, 7 × 104 cells were treated with 5 μM GAA, 10 μM cytochalasin B, 100 μM phloretin, and dimethyl sulfoxide (DMSO) in DMEM containing 5 mM glucose. Culture medium was collected after 24 h incubation time. Then, glucose consumption was measured using a glucose assay kit-WST G264 (Dojindo Molecular Technologies, Inc., Japan).47,48
2.8 Cellular thermal shift assay (CETSA)
A thermal shift assay was conducted as described previously.49 In brief, A549 and H1299 cells were treated with either 5 μM GAA or dimethyl sulfoxide (DMSO) for 6 h at 37 °C. Subsequently, the intact cell suspensions were harvested using cold PBS and aliquoted into PCR tubes, with 100 μl per tube. These suspensions were then heated in a thermal cycler at seven temperature points ranging from 30 °C to 90 °C, with each temperature maintained for 5 min. Following this, protein extraction and quantification were carried out, and protein stability was assessed via western blotting.
2.9 Quantitative real-time PCR
Quantitative real-time PCR was employed to detect the relative expression of mRNA of GLUT1, and GLUT3 after treating A549 and H1299 cells with 5 μM GAA for 24 h. The cellular RNA extraction was performed using the RNA TRIzol instructions. Subsequently, cDNA synthesis was performed using Hifair™ V one-step RT-gDNA digestion SuperMix (Yeasen, 11142-A/B/C). Then, qPCR was conducted using SYBR Green Master Mix (Yeasen, 11184ES03). A Roche 480 fluorescence PCR instrument (Basel, Switzerland) was used for qPCR. The relative expression of mRNA of targeted genes was calculated using the comparative CT method (2−ΔΔCt) and normalized to β-actin. The primer sequences are provided in the Table S3.†
2.10 Western blot
A 100 μL of RIPA lysate containing 1% protease inhibitor was added to the cells of each group and lysed in an ice bath for 30 min. Subsequently, the cells were collected using a cell spatula in 1.5 mL centrifuge tubes, and centrifuged at 4 °C, 14
000 g, for 15 min. The supernatant was collected and protein concentration was determined using the BCA protein assay kit method. After normalizing the protein concentration with PBS, 5 × loading buffer was added and placed in boiling water for 10 min to denature the protein. The proteins were separated by SDS-PAGE and transferred to a PVDF membrane. The membrane was washed three times with TBST, each time for 5 min, and then blocked with 5% skimmed milk powder at room temperature for 1 h. The corresponding primary antibody was added and incubated at 4 °C overnight. The next day, after rewarming, the membrane was washed three times with TBST, each time for 5 min. Horseradish peroxidase (HRP)-labeled secondary antibody was then added and incubated at room temperature for 1 h. After washing three times with TBST for 5 min each time, ECL ultrasensitive luminescent liquids A and B were mixed thoroughly in equal volumes and added dropwise onto the PVDF membrane. The membrane was developed using a chemiluminescence imaging analysis system (UVITEC/MINI HD9) to obtain protein expression information. Data were analyzed using ImageJ software.
2.11 Statistical analysis
The mean ± SD (SD: standard deviation) values were used to represent the data, and statistical significance was determined as a P-value < 0.05. To evaluate the differences between groups, t-Test, a one-way ANOVA (ANOVA: Analysis of Variance), and Dunnett's multiple comparison tests were performed using GraphPad Software (Prism version 8, San Diego, California, USA).
3. Results
3.1 Alphafold2 modeled high confidence protein structure of GLUT1exo and GLUT3endo
According to Alphafold2 calculations, the GLUT1exo and GLUT3endo models exhibited a high level of confidence, as indicated by the predicted local distance difference test (pLDDT) and Predicted Aligned Error (PAE) as shown in (Fig. S1 and S2†).
The models were further validated by SAVES v6.0 using the PROCHECK and ERRAT analysis before and after energy minimization. Analysis of the distribution of φ and ψ angles of amino acid residues of GLUT3endo from the Ramachandran plots showed that 94.6% of the residues resided in the most preferred region, 4.5% in the additional allowed region, and only 0.9% in the generously allowed region. The overall quality factor obtained from ERRAT for the predicted GLUT3endo was found to be 98.529% which indicates to high resolution of protein structure. Furthermore, the analysis of the distribution of φ and ψ angles of amino acid residues of GLUT1exo model from the Ramachandran plots after energy minimization showed that 95.2% of the residues resided in the most favored regions, 4.3% in the additional allowed regions, only 0.5% in the generously allowed regions, and no residues observed in disallowed regions. The overall quality factors obtained from ERRAT for the predicted GLUT1exo before and after energy minimization were found to be 94.280 and 98.073%, respectively suggesting a high resolution of the protein structure (Table 1 and Fig. 1, 2).
Table 1 Ramachandran plot statistics of GLUT3endo and GLUT1exo conformations as calculated by SAVES v6.0a
Residue |
Full-length |
GLUT3endo |
GLUT1exo |
Before minimization |
After minimization |
Before minimization |
After minimization |
Number |
Percentage |
Number |
Percentage |
Number |
Percentage |
Number |
Percentage |
Based on an analysis of 118 structures of resolution of at least 2.0 Angstroms and R-factor no greater than 20%, a good quality model would be expected to have over 90% in the most favored regions. |
In most favored regions [A, B, L] |
401 |
94.6 |
401 |
94.6 |
397 |
94.3 |
401 |
95.2 |
In additional allowed regions [a, b, I, p] |
18 |
4.2 |
19 |
4.5 |
21 |
5.0 |
18 |
4.3 |
In generously allowed regions [∼a, ∼b, ∼I, ∼p] |
5 |
1.2 |
4 |
0.9 |
1 |
0.2 |
2 |
0.5 |
In disallowed regions |
0 |
0.0 |
0 |
0.0 |
2 |
0.5 |
0 |
0.0 |
Number of non-glycine and non-proline |
424 |
100.0% |
424 |
100.0% |
421 |
100.0% |
421 |
100.0% |
Number of end-residues (excl. Gly and Pro) |
2 |
|
2 |
|
2 |
|
2 |
|
Number of glycine residues (shown as triangles) |
49 |
|
49 |
|
46 |
|
46 |
|
Number of proline residues |
21 |
|
21 |
|
23 |
|
23 |
|
Total number of residues |
|
496 |
496 |
492 |
|
492 |
|
|
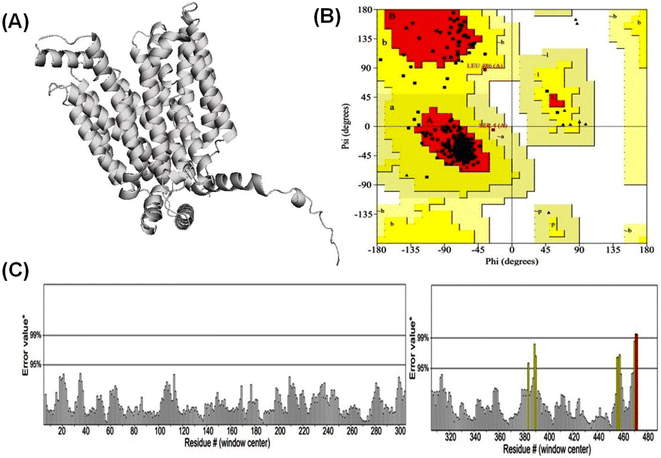 |
| Fig. 1 Validation and quality evaluation of the predicted structure of GLUT1 in exofacial conformation. Plot (A) shows the Predicted GLUT1exo model. Ramachandran plot (PROCHECK) of GLUT1exo model (B), and (C) displays ERRAT analysis of GLUT1exo model after energy minimization. | |
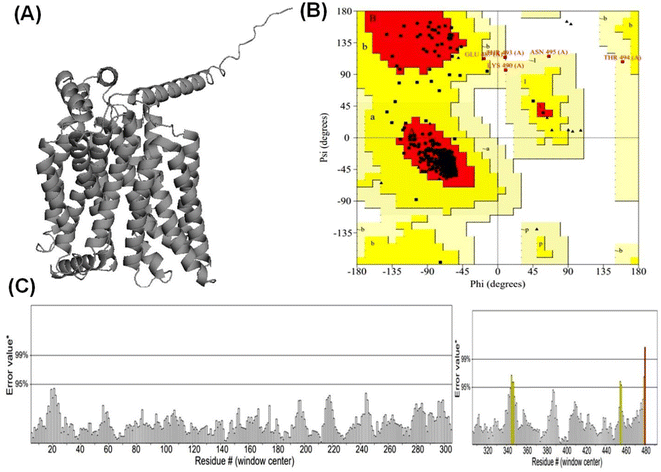 |
| Fig. 2 Validation and quality evaluation of the predicted structure of GLUT3 in endofacial conformation. Plot (A) shows the Predicted GLUT3endo model. Ramachandran plot (PROCHECK) of GLUT3endo model (B), and (C) displays ERRAT analysis of GLUT3endo model after energy minimization. | |
3.2 GLUT1/3 possesses multiple putative binding pockets in their endo- and exofacial conformations
The pocket of a target protein is made up of several binding sites that are complementary in terms of their chemical makeup, size, and shape to their ligands. Prank Web (https://prankweb.cz/) server determined four binding pockets for GLUT1endo, six pockets for GLUT3endo, five pockets for GLUT1exo, and nine pockets for GLUT3exo (Table S1 and Fig. S3†). Based on the ranking of the pockets, pocket 1 showed a higher score among other pockets in both conformational states in these proteins, therefore it was chosen for docking analysis. After analysis, we observed that the binding sites of pocket 1 are situated at the glucose binding site in GLUT1, while they are located outside the glucose binding site in GLUT3.
3.3 Ganoderic acid A binds to GLUT1/3 with high affinity to GLUT1endo conformation
The optimum intermolecular interaction between GLUT1/3 and ligands (Fig. 3) was investigated using molecular docking analysis. The ligands were docked against GLUT1 and 3 separately in endo- and exofacial states.
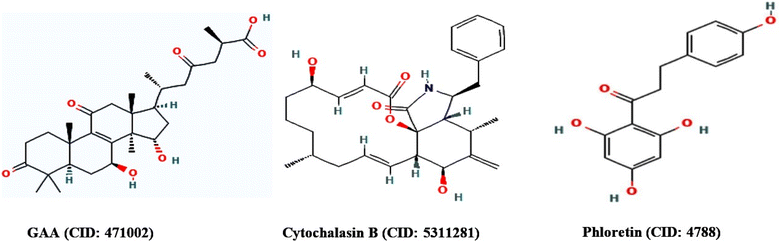 |
| Fig. 3 Two-dimensional (2D) structure of ganoderic acid A (GAA: C30H44O7), cytochalasin B (CCB: C29H37NO5), and phloretin (Phlo: C15H14O5). | |
The binding affinities of the ligands to GLUT1/3 following molecular docking are illustrated in Table 2. The binding of GAA to the endofacial conformation of GLUT1 shows higher affinities compared to its exofacial conformation, comparable to standard inhibitors (cytochalasin B and phloretin). Additionally, GAA displays a better binding affinity to GLUT1endo compared to GLUT3endo, this result aligns with the MMGBSA result (Table 2). These findings suggest that GAA exhibits differential binding to GLUT1/3 with preference affinity to GLUT1endo conformation.
Table 2 List of compound identity, binding affinity, and MMGBSA (dG) of ganoderic acid A (GAA), cytochalasin B (CCB), and phloretin (Phlo) with GLUT1/3
Ligand |
Autodock vina 1.1.2 binding affinity (kcal mol−1) |
Autodock vina 1.2.5 binding affinity (kcal mol−1) |
dG bind |
Endofacial |
Exofacial |
Endofacial |
Exofacial |
Endofacial |
Exofacial |
GLUT1 |
GLUT3 |
GLUT1 |
GLUT3 |
GLUT1 |
GLUT3 |
GLUT1 |
GLUT3 |
GLUT1 |
GLUT3 |
GLUT1 |
GLUT3 |
GAA |
−10 |
−8.3 |
−9.1 |
−8.1 |
−10.007 |
−7.021 |
−8.681 |
−7.586 |
−65.88 |
−49.28 |
−51.37 |
−63.11 |
CCB |
−10.4 |
−9.1 |
— |
— |
−8.539 |
−5.061 |
— |
— |
−74.23 |
−78.58 |
— |
— |
Phlo |
— |
— |
−8.1 |
−7.9 |
— |
— |
−8.198 |
−7.643 |
— |
— |
−34.24 |
−45.62 |
Fig. 4 shows that the residues ASN288 and HIS160 formed hydrogen bonds in the GAA-GLUT1endo complex, while GLN283, ASN288, and ASN317 in the GAA-GLUT1exo complex. Furthermore, cytochalasin B (CCB) formed hydrogen bonds with THR137 and PRO385 in GLUT1endo, whereas in the phloretin-GLUT1exo complex, six residues (GLN161, GLN172, GLN282, GLN283, TRP288, and ASN415) were observed to form hydrogen bonds.
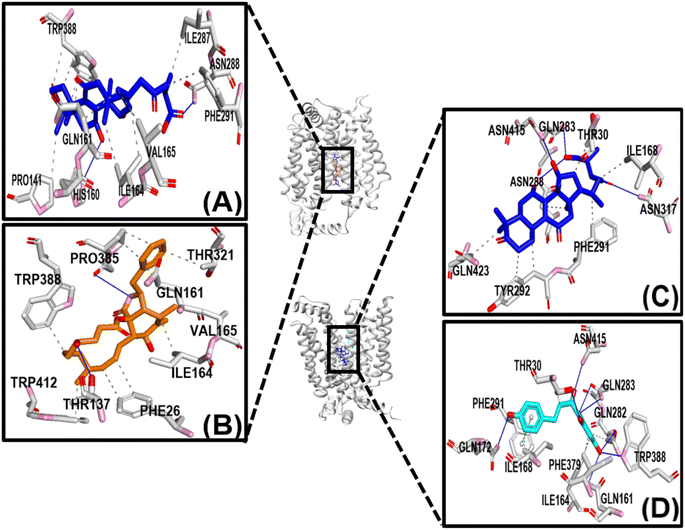 |
| Fig. 4 Protein–ligand interactions profile prediction of ligand–GLUT1 complexes. Panels (A and B) show the 3D interactions of GAA (A) and cytochalasin B (B), respectively in a complex with GLUT1endo, while panels (C and D) display the 3D interactions of GAA and phloretin, respectively in a complex with GLUT1exo. The blue line represents the hydrogen bonds. The pink and red represent the nitrogen and oxygen atoms, respectively. | |
In the ligand–GLUT3 complexes, GLN281, TYR291, and ASN413 were observed to form hydrogen bonds in the GAA-GLUT3endo complex, while THR28, GLN281, TYR291, and ASN413 were observed in the exofacial conformation of the same complex. The endofacial inhibitor cytochalasin B formed hydrogen bonds with THR28, GLN281, ASN286, and TYR386 in GLUT3. In contrast, the exofacial inhibitor phloretin formed hydrogen bonds by TYR291, and ASN413 (Fig. 5). Additionally, GAA, CCB, and Phlo were also observed to form hydrophobic interactions with GLUT1 and GLUT3 (Table S2†).
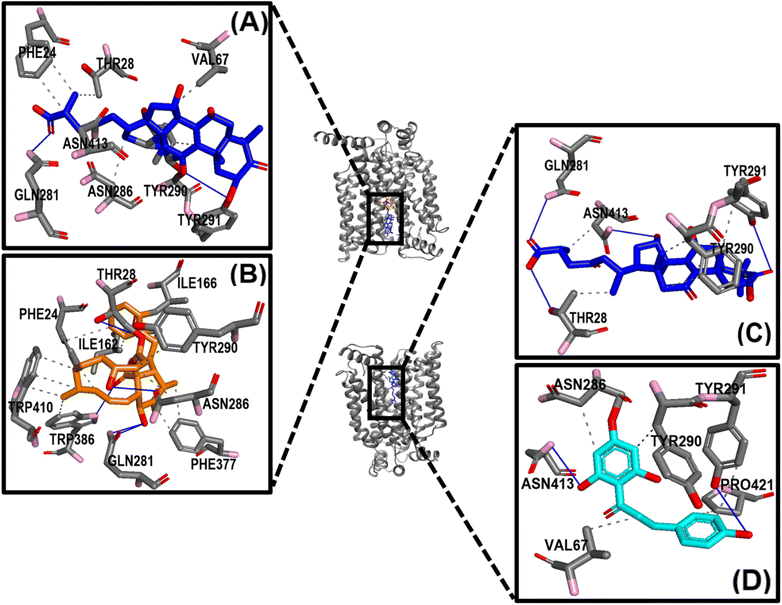 |
| Fig. 5 Protein–ligand interactions profile prediction of GLUT3 complexes. Panels (A and B) show the 3D interactions of GAA (A) and cytochalasin B (B), respectively in a complex with GLUT3endo, while panels (C and D) display the 3D interactions of GAA and phloretin, respectively in a complex with GLUT3exo. The blue line represents the hydrogen bonds. The pink and red represent the nitrogen and oxygen atoms, respectively. | |
3.4 Ganoderic acid A preferentially stabilizes the endofacial conformation of GLUT1
The Root Mean Square Deviation (RMSD) is a widely accepted measure for assessing alterations in the structural framework of proteins from their original to final conformational states. Through the analysis of trajectory deviations during simulations, it is possible to determine the stability of a protein to its conformation. In general, proteins exhibiting greater deviations are considered less stable.50 Thus, the study evaluated the stability of GLUT1 and GLUT3 upon binding to GAA in the endo- and exofacial conformations and compared with standard inhibitors. In Fig. 6A, the RMSD trajectory of GLUT1endo′s backbone exhibits a stable and significant decrease in RMSD values (1.5 Å) upon binding to GAA, compared to apo-GLUT1endo and CCB-bound GLUT1endo, which reached (2.5–3.0 Å).
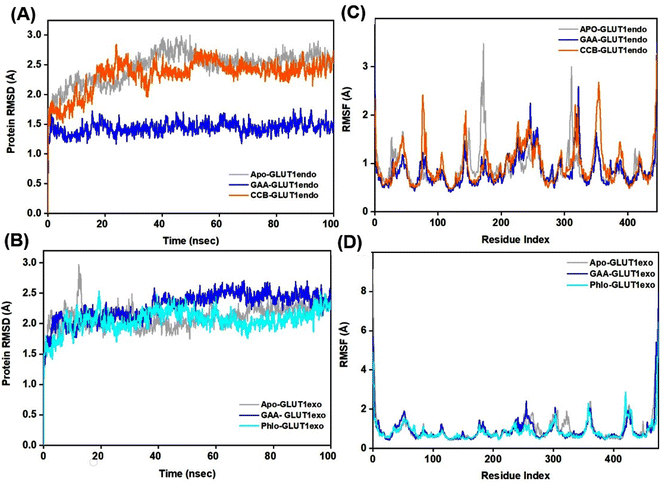 |
| Fig. 6 RMSD and RMSF study plots of ligand–GLUT1 complexes. (A and B) Represent the RMSD plots for the GLUT1endo and GLUT1exo, respectively. While (C and D) represent the RMSF plots, respectively for the GLUT1endo and GLUT1exo. Trajectories for the apo-GLUT1, and complexes with GAA, cytochalasin (CCB), and phloretin (Phlo) are shown in light grey, blue, orange, and cyan colors, respectively. | |
However, when GAA binds to the same transporter in the exofacial conformation, the trajectory remains stable until 40 ns with a slight increase in RMSD values, reaching 2.5 Å, before returning to 2.0 Å by the end of the simulation. In contrast, both apo-GLUT1exo and phloretin-bound GLUT1exo show a stable RMSD trajectory throughout the simulation, except for apo, which exhibits an increase in RMSD value, reaching 3.0 Å within the first 20 ns, before stabilizing at 2.292 Å by 100 ns (Fig. 6B). On the other hand, the RMSD trajectory of apo-GLUT3endo exhibited fluctuations and an increase in RMSD value at the first 20 ns reaching (3.5 Å), which decreased to reach (1.5 Å) at the end of the simulation time. RMSD trajectory of GAA-bound GLUT3endo showed a noticeable decrease in the value reaching (1.5–2.0 Å) compared to that of apo-GLUT3endo and CCB-bound GLUT3endo (Fig. 7A).
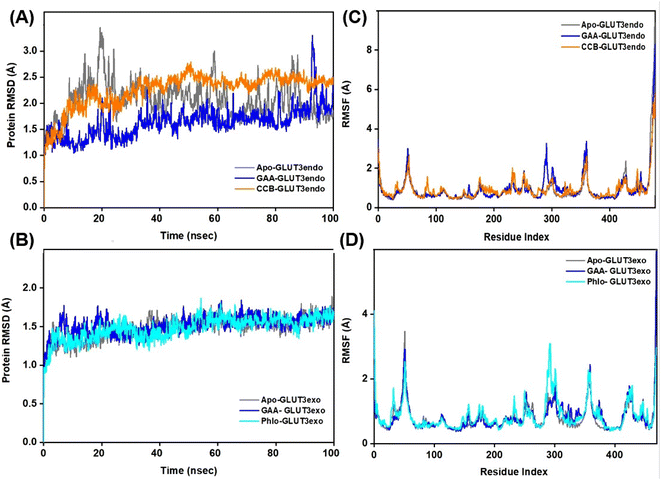 |
| Fig. 7 RMSD and RMSF study plots of ligand–GLUT3 complexes. (A and B) Represent the RMSD plots for the GLUT3endo and GLUT3exo, respectively. While (C and D) represent the RMSF plots, respectively for the GLUT3endo and GLUT3exo. Trajectories for the apo-GLUT3, and complexes with GAA, cytochalasin (CCB), and phloretin are shown in dark grey, blue, orange, and cyan colors, respectively. | |
The RMSD trajectory of apo-GLUT3exo exhibited a stable profile, starting at 0.8 Å in the beginning and then increasing to 1.5 Å by the end of the simulation. The RMSD trajectory for GAA- and phloretin-bound GLUT3exo registered remained stable throughout the 100 ns in the range of (1.0–1.5 Å), as illustrated in Fig. 7B.
Extending the MDS time by an additional 100 ns for GLUT3 in both its endo- and exofacial conformations with GAA and the inhibitors resulted in no significant differences in the RMSD values of the GLUT3 backbone compared to the 100 ns timeframe, with all fluctuations remaining below 3 Å (Fig. S9†).
The ligand's stability in the binding sites of the proteins under study was evaluated using ligand RMSD. RMSD analysis of GAA in GLUT1 in its two conformations exhibited stable and low values compared to that of the standard inhibitors (Fig. 8; Video S1–S4†). In contrast, within GLUT3, the RMSD of GAA remained high at 6.0 Å during the initial 100 ns. Extending the MDS for an additional 100 ns showed that the RMSD of GAA stayed elevated at 7.0 Å in the GLUT3 pocket, while the RMSD of the inhibitors did not. Despite this, both GAA remained in the pocket throughout the entire MDS duration (Fig. S9 and Video S5–S8†).
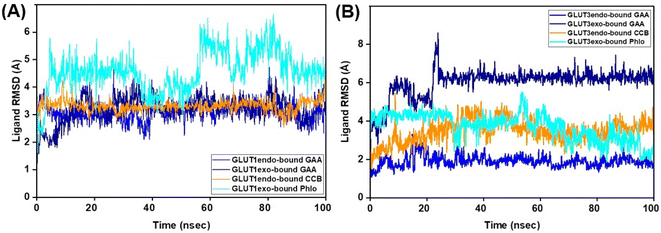 |
| Fig. 8 RMSD plots of GLUT1/3–ligand complexes. (A and B) Represent ligand RMSD plots for the GLUT3endo and GLUT3exo, respectively. Trajectories for the GLUT1/3-bound GAA in endofacial and exofacial conformation are shown in blue and dark blue, respectively, while cytochalasin (CCB), and phloretin are shown in orange, and cyan colors, respectively. | |
Root Mean Square Fluctuation (RMSF) is a critical factor to consider when evaluating the stability and adaptability of complex systems during simulation. Its primary purpose is to determine the behavior of amino acid residues upon ligand binding.51 The RMSF values were calculated and plotted for Cα atoms of GLUT1 and GLUT3 in the apo-state as well as the GAA-bound state compared to the standard inhibitors. Residues with low or negligible RMSF values, indicating their restricted movement during MD simulations, are considered more stable.52 Fig. 6C displays GLUT1endo residues fluctuation levels over the 100 ns simulation time. The RMSF graph of apo-GLUT1endo demonstrated fluctuation values (3.0–3.5 Å) for residues located at positions (100–200 and 300–400), while GAA-bound GLUT1endo shows a decrease in the fluctuation of these residues, suggesting the stabilization of GLUT1endo upon GAA binding which is comparable to the endofacial inhibitor cytochalasin B at several residues in the protein backbone. Fig. 6D displays the fluctuation levels of GLUT1exo residues over the 100 ns simulation time. The RMSF graph of apo-GLUT1exo showed no significant fluctuation except in the residues between 250–300 where the apo-GLUT1exo exhibited a slight increase in the values, which are reduced upon binding of GAA and phloretin. RMSF analysis of GLUT3 in its two conformations showed fluctuations in several sites in the protein backbone. The binding of GAA to GLUT3exo reduced the fluctuations comparably to phloretin (Fig. 7C and D). The RMSF analysis for both 100 ns and 200 ns MDS timeframes of GLUT3–ligands complexes demonstrates that the ligands exhibit stable interactions with GLUT3. The consistency in the RMSF values across both timeframes suggests that the structural flexibility and stability of the complex are well-maintained over extended simulation periods (Fig. S10†).
Further, the study analyzed the interactions of the GAA and standard inhibitors with the transporters. In the GAA-GLUT1 complexes (Fig. 9A and B), hydrogen bonding was shown with THR 30, HIS 160, GLN 161, and TYR 292 in the endofacial state, while the residues ASN34, GLN37, TRP65, GLN283, and ASN288 were participants in hydrogen bonding interactions with GAA in the exofacial conformation. Five residues (HIS160, GLN282, GLN283, ASN288, and TRP388) in the GLUT1endo form strong hydrogen bonds with cytochalasin B, while, ASN34, GLN172, GLN283, and ASN288 were identified as residues in hydrogen bonding interactions with phloretin (Fig. 9A–D). This result is highly consistent with the protein–ligand analyses of docking results (Table S1†) and ligand–protein contact map (Fig. S4†). The residues HIS160, ASN34, GLN283, and ASN288 are involved in hydrogen bonding with GAA as well as standard inhibitors.
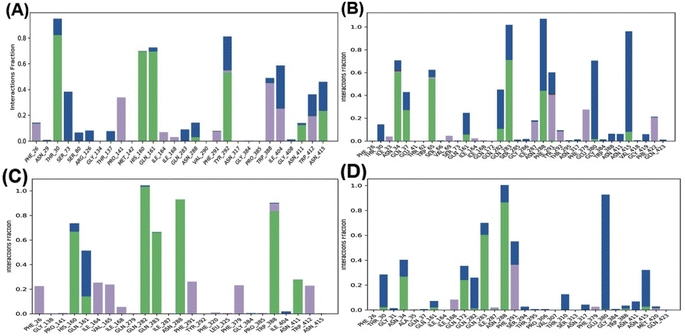 |
| Fig. 9 The histogram of GLUT1–ligand interactions. (A–D) Plots represent GAA-GLUT1endo, GAA-GLUT1exo, CCB-GLUT1endo, and Phlo-GLUT1exo, respectively. The histogram of protein–ligand interaction displays hydrogen bonding in green color, ionic in red, water bridges in blue, and hydrophobic bonds in violet. | |
Fig. 10 highlights the interactions of the ligands with GLUT3 in endofacial and exofacial conformations. GAA formed hydrogen bonding with GLN281, ASN286, and ASN413 of GLUT3 in its endofacial conformation, while ASN32 and ASN413 were in the exofacial conformation of this transporter (Fig. 10A and B). Cytochalasin B established hydrogen bonds with SER64, SER71, ASN413, and GLY417, while phloretin was involved in these interactions with TYR290 and ASN413 (Fig. 10C and D). The majority of residues predicted by docking to form hydrogen bonds were reaffirmed in this analysis. It's worth noting that, extending the MDS time to 200 ns for GLUT3 with three ligands in its endo- and exofacial conformation revealed that the residues participating in hydrogen bonding were either identical or increased in number compared to the 100 ns simulation. This suggests enhanced stability and interaction over the longer simulation period (Fig. S11†). In addition, the number of interacted amino acids and the values of RMSD, MolSA, rGyr, PSA, and SASA of GAA in GLUT1/3 in their two conformations are consistent with the aforementioned findings (Fig. S4–S7†).
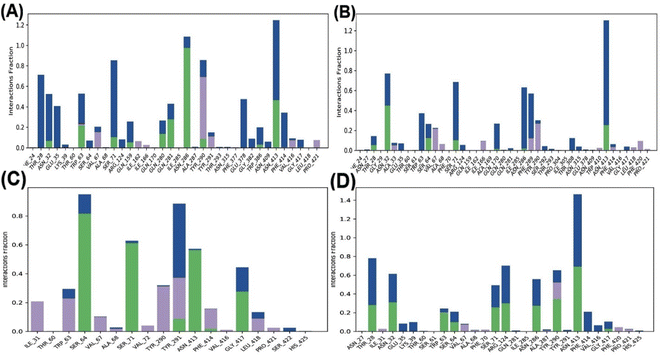 |
| Fig. 10 The histogram of GLUT3–ligand interactions. (A–D) Plots represent GAA-GLUT3endo, GAA-GLUT3exo, CCB-GLUT3endo, and Phlo-GLUT3exo, respectively. The histogram of protein–ligand interaction displays hydrogen bonding in green color, water bridges in blue, and hydrophobic bonds in violet. | |
3.5 Ganoderic acid A induces pronounced dynamics in GLUT's exofacial conformation
During the MD simulation, GLUT1 and GLUT3 show dimensional movement that can be deduced to have principal components and generate a series of eigenvalues and eigenvectors that describe the overall motion of these transporters, which can be linked to the system's stability and protein function. Here, we harnessed principal component analysis (PCA) to assess the conformational dynamics of GLUT1/3 upon binding to GAA, contrasting them with standard inhibitors. PCA divides the dimensions into their fundamental components.
Fig. 11 illustrates the first three PCAs predominantly accounted for the motion of the protein backbone based on the MD trajectories. According to PCA analysis of GAA-GLUT1, the first three eigenvectors account for 16.23%, 9.24%, and 7.07% of GLUT1endo, while for GLUT1exo, they account for 34.23%, 8.18%, and 6.77% (Fig. 11A and B). In contrast, PCA analysis shows that the first three eigenvectors account for 20.4%, 16.23%, and 11.6% of GAA with GLUT3endo, whereas for GLUT3exo, they account for 17.52%, 11.86%, and 6.6% (Fig. 11C and D). PCA analysis of GLUT3–ligand complex has been performed for 200 ns MD simulation result. As shown in Fig. S14,† there are no significant differences between PC1, PC2, and PC3 that resulted from the interaction of GLUT3 and GAA, CCB, or phloretin over 100 ns and 200 ns simulation time. The GAA-GLUT1exo complex exhibited the highest PC1 value (34.23%), signifying a more significant degree of conformational change than other GLUT1/3 conformations. Within this study, phloretin induced the highest PC1 (22.98%) in GLUT1, whereas cytochalasin B led to the highest PC1 in GLUT3 (33.03%) (Fig. S8†). The finding indicates that GAA may exhibit comparable behavior to standard inhibitors with GLUT1, but not with GLUT3.
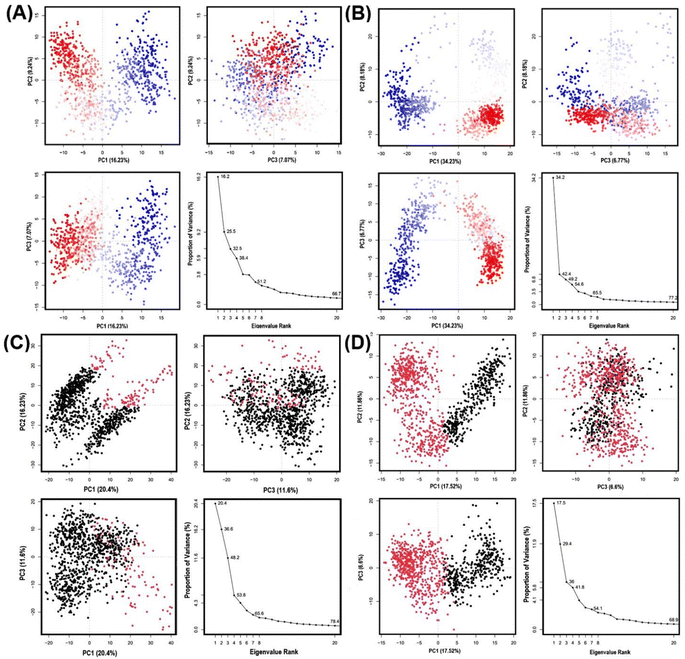 |
| Fig. 11 PCA of top three principal component analysis of 100 ns, along with eigenvalue rank plots. (A–D) Plots represent GAA-GLUT1endo, GAA-GLUT1exo, GAA-GLUT3endo, and GAA-GLUT3exo, respectively. The plot had multiple data points, where each one denoting the conformation of the protein. A color gradient indicates the initial to final stages of the simulation (blue to red for GLUT1 and red to black for GLUT3). | |
3.6 Ganoderic acid A reduces the viability of human lung cancer cells
To evaluate the effect of GAA on cell lines' viability, CCK-8 analysis was performed. A549 and H1299 were treated with GAA at concentrations of 0.5, 2.5, 5, 10, and 20 μM for 24 h. As shown in (Fig. 12A and B), GAA revealed a noticeable decline in cell viability in A549 and H1299 in a dose-dependent manner after 24 h treatment. Additionally, we found that (5 μM) of GAA showed >70% viability of A549 with a non-significant effect on H1299 cells, therefore this concentration was used in the following experiments.
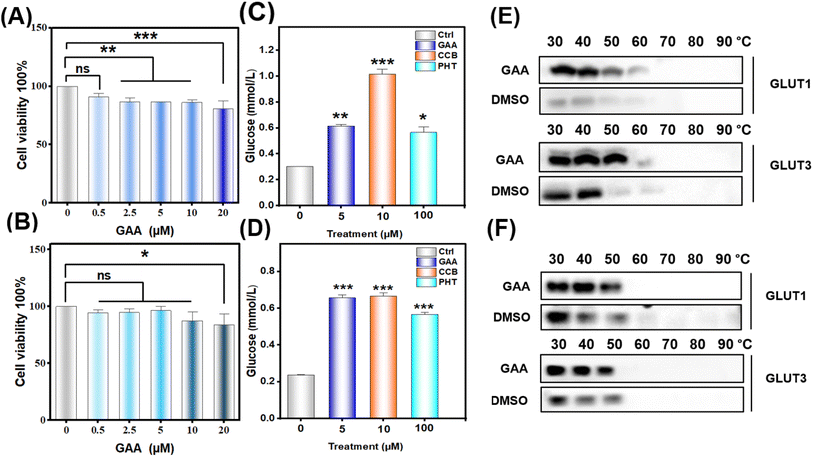 |
| Fig. 12 Cytotoxicity, glucose consumption, and cellular thermal shift assays. Panels (A and B) display the cytotoxicity effect of GAA on A549 and H1299 cells, respectively. Panels (C and D) show the impact of GAA on glucose consumption by A549 and H1299, respectively. Panels (E and F) demonstrate the thermal stability of GLUT1/3 by GAA in A549 and H1299 cells, respectively. Statistical significance is denoted as follows: *P < 0.05; **P < 0.01; ***P < 0.001. | |
3.7 Ganoderic acid A inhibits glucose consumption in human lung cancer cells
To assess whether GAA has an impact on glucose uptake in lung cancer cells, we measured the glucose consumption after 24 hours. Fig. 12C and D show that GAA had a significant effect on glucose consumption by A549 and H1299 cells comparable to cytochalasin B and phloretin. Moreover, this dose did not significantly alter the gene expression of either transporter, with the only notable elevation being in GLUT3 expression in H1299 cells (Fig. S15†).
3.8 CETSA validates the stability of GLUT1/3 proteins by GAA in human lung cancer cells
To validate the in silico result, we assessed the thermal stability of GAA-GLUT1/3 complexes in A549 and H1299 cells treated with 5 μM GAA. As shown in Fig. 12E and F, GAA thermally stabilized GLUT1 and GLUT3 compared to the control in both A549 and H1299 cells. Notably, we observed that H1299 cells express GLUT1 and GLUT3 with a molecular weight of 70 kDa, which has been previously reported as due to glycosylation.53
4. Discussion
Recently, aerobic glycolysis has emerged as a major target in anticancer drug development.54 Ganoderic acids, known for their cancer-inhibiting properties, have been found to reduce glucose uptake and regulate energy metabolism in cancer. However, the structural basis for their inhibitory role on critical proteins involved in cancer metabolism remains elusive. Here, we aimed to uncover the inhibitory potential of ganoderic acid A (GAA) on critical transporters in human lung cancer, glucose transporters 1 and 3 (GLUT1 and GLUT3). To evaluate the impact of GAA on these transporters and its function in glucose metabolism, a combination of in silico and in vitro investigations was employed. Recent advances in protein structure prediction, driven by deep-learning methods like Alphafold2, offer a solution to the lack of available experimental protein structures. Lyu et al. recently55 used Alphafold2 structures to test hundreds of new molecules, comparing the results to docking against experimental structures. Their study found that the hit rates and affinities were similarly high for both the experimental and Alphafold2 structures. Since there is no crystal structure deposited in the protein database bank for GLUT3 and GLUT1 in an endofacial and exofacial conformation, respectively so far, Alphafold2 was harnessed. Based on the validation results of the GLUT3endo and GLUT1exo structures by Alphafold2 and SAVES v6.0, the models have extremely high confidence making them suitable for further analysis.
Despite having identical substrate coordination and a fully conserved substrate-binding site, GLUT1 and GLUT3 exhibit significantly different transport affinities (∼10–20 mM and ∼3 mM, respectively) that correspond to their respective physiological roles. This suggests that certain sites beyond the glucose-binding site in GLUT3 may provide a clue to its high affinity to glucose, as reported recently.56 These sites may also be utilized as drug-binding sites to inhibit GLUT3. In light of a comparative study on the selectivity of substrate and inhibitor among human glucose transporters that used GLUT1 (PDB: 5EQG) as a model, several critical residues in GLUT proteins were identified.57 Accordingly, our study found that GAA interacts with GLN161, ASN288, and TRP388 in GLUT1endo, and with GLN281 and ASN286 in GLUT3endo. These residues are conserved in the glucose binding sites of GLUT1 and GLUT3, respectively.57 Cytochalasin B a fungal metabolite widely known for its actin polymerization inhibition, was initially recognized as a positive control for glucose uptake inhibition (GLUT endofacial inhibitor). However, further development of cytochalasin B was abandoned due to its predominant inhibitory activity on actin polymerization.58 Our study found that GAA interacts with HIS160, GLN282, and TRP388 in GLUT1, which are the residues inhibited by cytochalasin B. In contrast, GAA did not interact with any of the GLUT3 residues that are affected by cytochalasin B inhibition, suggesting that GAA may target different residues to inhibit the endofacial conformation of GLUT3. Moreover, we studied the interaction of GAA with the exofacial conformation of GLUT1 and GLUT3 based on (PDB ID 4ZW9). GAA interacted with several residues in GLUT1exo and GLUT3exo, however, GLN283, ASN288, and ASN317 in GLUT1 and GLN281 in GLUT3 are conserved and members of the glucose binding site in 4ZW9 according to.59 In comparison with phloretin the exofacial inhibitor of GLUT1/3, GAA interacts with several residues in GLUT1exo including GLN283, which is also targeted by phloretin. While in GLUT3exo, three residues (TYR290, TYR291, and ASN413) were found in binding with GAA and also targeted by phloretin.
Molecular dynamics simulation plays a vital role in both studying the dynamic movements of atoms and analyzing the effects of ligand binding on protein stability, making it a valuable tool in drug discovery.60 The RMSD, RMSF, and protein–ligand interaction analyses indicated that GAA can bind and stabilize the trajectories of GLUT1 and GLUT3 in their endofacial and exofacial conformations. However, GAA had a more potent effect on stabilizing the trajectories of GLUT1 in its endofacial conformation compared to the exofacial conformation. Moreover, the principal component analysis showed that the structures of GLUT1/3 underwent a degree of conformational changes upon binding to GAA. These changes were more pronounced in the exofacial conformation than in the endofacial conformation of GLUT1. These findings suggest that GAA may have an inhibitory effect on GLUT1 and 3 which may obstruct the glucose transport either by directly hindering glucose access to its active site or by stabilizing the inward-facing conformation and thus preventing glucose from entering its active site.
The expression of glucose transporters in particular GLUT3 is associated with increased proliferation and serves as an independent prognostic factor for overall survival in non-small cell lung cancer NSCLC.61 Thus, A549 and H1299 cell lines were used to investigate whether GAA impacts glucose metabolism by targeting GLUT1 and GLUT3. Earlier, Feng et al.62 found that triterpenes from Ganoderma lucidum have anti-cancer activity by inhibiting cell proliferation and tumor growth in A549 cells and Lewis tumor-bearing mice. In the same context, we found that GAA could inhibit the viability of A549 and H1299 cells in a dose-dependent manner, confirming their cytotoxicity to these cells. A study by Liu et al.,26 uncovered the capacity of ganoderic acid D to inhibit glucose uptake and regulate energy metabolism in colon cancer. Here, we found that GAA could also inhibit glucose uptake in A549 and H1299 cells, comparable to the effect of the endofacial and exofacial inhibitors in both cell lines, without affecting the gene expression of GLUT1/3. This finding suggests the potential of GAA to inhibit glucose uptake by binding to and stabilizing GLUT1/3, as predicted by computational analysis. Cellular thermal shift assay was devised to assess the binding of drug molecules with protein targets in cellular and tissue samples, leveraging the principle of ligand-induced thermodynamic stabilization of protein targets.63 It demonstrates superior performance across various sample types. This makes it beneficial for compounds reliant on cellular metabolism.64 GAA thermally stabilized both transporters in the intact lung cancer cells, validating the in silico result and suggesting the capacity of GAA to inhibit GLUT1 and GLUT3. Despite GAA being considered a dietary supplement and hepatoprotective agent,65 its role in inhibiting GLUT1/3 may impact essential functions in normal cells such as neurons66 and the placenta,67 given their critical roles in glucose transport. Therefore, it is worth investigating whether GAA would significantly impact the function of GLUT1 and GLUT3 in neurons and the placenta as a step to study the selectivity of GAA on GLUT1/3 in lung cancer cells using advanced delivery systems. While broad-spectrum GLUT inhibitors could be more effective in reducing cancer cell survival, they might also be more harmful to normal cells. Conversely, inhibitors that are specific to one or two predominant GLUT isoforms in certain types of cancer, when used in conjunction with other anti-cancer therapies, could offer novel therapeutic approaches that can be tailored to the metabolic reprogramming of each cancer type and stage.68 In light of our study, it appears that GAA may be a promising therapeutic agent and an advantageous treatment option for reprogramming glucose metabolism in lung cancer. This is due to the potential broad impact of GAA on glucose metabolism in lung cancer.
5. Conclusions
In summary, aerobic glycolysis has become a significant target for anticancer drug development. It is well known that cancer cells upregulate the expression of glycolysis enzymes and glucose transporters, which correlate with the invasiveness and metastatic potential of cancers. Elevated expression of both GLUT1 and GLUT3 has been observed in various cancer types, including lung cancer. Besides its anticancer activity, ganoderic acid A is considered a dietary supplement and hepatoprotective agent. Therefore, our study aimed to uncover the inhibitory potential of GAA on GLUT1 and GLUT3 in human lung cancer. Using in silico and in vitro methods, we demonstrated that GAA binds to and stabilizes these transporters, inhibiting glucose uptake in A549 and H1299 cells comparably to GLUT1/3 inhibitors. These findings highlight a new potentiality of GAA as an anticancer agent through inhibiting GLUT1/3.
Data availability
This study was carried out using publicly available data from [protein Database Bank] at [RCSB PDB: Homepage https://www.rcsb.org/] with [PDB ID 5EQG and ID 4ZW9].
Author contributions
Q. H. conceived and supervised the research. M. A. B. conducted modeling and docking analysis. M. A. B. and M. A. conducted MD simulation work, and M. A. B., S. C. S., and P. B.-Q. performed cellular analysis. M. A. B., W. A. A., H. W., and M. S. S. results visualization. All the authors participated in the research. M. A. B. wrote the manuscript draft. Q. H. edited and finalized the manuscript. All authors have approved the final version.
Conflicts of interest
The authors declare no commercial or financial conflict of interest.
Acknowledgements
This work was supported by the National Science Foundation of China (11635013). Especially, Miss Mona Alrasheed Bashir would like to express her gratitude to the Chinese government for the CSC fellowship she received.
References
- J. M. Cuezva, M. Krajewska, M. L. de Heredia, S. Krajewski, G. Santamaría, H. Kim, J. M. Zapata, H. Marusawa, M. Chamorro and J. C. Reed, Cancer Res., 2002, 62, 6674–6681 CAS.
- L. A. Villani, B. K. Smith, K. Marcinko, R. J. Ford, L. A. Broadfield, A. E. Green, V. P. Houde, P. Muti, T. Tsakiridis and G. R. Steinberg, Mol. Metab., 2016, 5, 1048–1056 CrossRef CAS PubMed.
- M. Younes, L. V. Lechago and J. Lechago, Clin. Cancer Res., 1996, 2, 1151–1154 CAS.
- L. M. Phan, S. C. Yeung and M. H. Lee, Cancer Biol. Med., 2014, 11, 1–19 CAS.
- M. Pliszka and L. Szablewski, Cancers, 2021, 13(16), 4184 CrossRef CAS PubMed.
- Y. H. Kim, D. C. Jeong, K. Pak, M. E. Han, J. Y. Kim, L. Liangwen, H. J. Kim, T. W. Kim, T. H. Kim, D. W. Hyun and S. O. Oh, Oncotarget, 2017, 8, 68381–68392 CrossRef PubMed.
- V. Douard and R. P. Ferraris, Am. J. Physiol.: Endocrinol. Metab., 2008, 295, E227–E237 CrossRef CAS PubMed.
- J. Pujol-Gimenez, F. P. de Heredia, M. A. Idoate, R. Airley, M. P. Lostao and A. R. Evans, J. Cancer, 2015, 6, 139–143 CrossRef PubMed.
- L. Szablewski, Int. J. Cardiol., 2017, 230, 70–75 CrossRef PubMed.
- M. L. Macheda, S. Rogers and J. D. Best, J. Cell. Physiol., 2005, 202, 654–662 CrossRef CAS PubMed.
- M. Younes, R. W. Brown, M. Stephenson, M. Gondo and P. T. Cagle, Cancer, 1997, 80, 1046–1051 CrossRef CAS PubMed.
- M. Masin, J. Vazquez, S. Rossi, S. Groeneveld, N. Samson, P. C. Schwalie, B. Deplancke, L. E. Frawley, J. Gouttenoire, D. Moradpour, T. G. Oliver and E. Meylan, Cancer Metabol., 2014, 2, 11 CrossRef PubMed.
- T. Amann and C. Hellerbrand, Expert Opin. Ther. Targets, 2009, 13, 1411–1427 CrossRef CAS PubMed.
- W. A. Flavahan, Q. Wu, M. Hitomi, N. Rahim, Y. Kim, A. E. Sloan, R. J. Weil, I. Nakano, J. N. Sarkaria, B. W. Stringer, B. W. Day, M. Li, J. D. Lathia, J. N. Rich and A. B. Hjelmeland, Nat. Neurosci., 2013, 16, 1373–1382 CrossRef CAS PubMed.
- R. E. Airley and A. Mobasheri, Chemotherapy, 2007, 53, 233–256 CrossRef CAS PubMed.
- Z. Yao, F. Xie, M. Li, Z. Liang, W. Xu, J. Yang, C. Liu, H. Li, H. Zhou and L. H. Qu, Cell Death Dis., 2017, 8, e2633 CrossRef CAS PubMed.
- M. K. Temre, A. Kumar and S. M. Singh, Front. Pharmacol, 2022, 13, 1035510 CrossRef CAS PubMed.
- J. Nazaruk and M. Borzym-Kluczyk, Phytochem. Rev., 2015, 14, 675–690 CrossRef CAS PubMed.
- J. Jiang, B. Grieb, A. Thyagarajan and D. Sliva, Int. J. Mol. Med., 2008, 21, 577–584 CAS.
- J. Shao, Z. Li, G. Jiao, G. Sun and Z. Zhou, Nanfang Yike Daxue Xuebao, 2015, 35, 619–624 CAS.
- X. Wang, D. Sun, J. Tai and L. Wang, Mol. Med. Rep., 2017, 16, 3894–3900 CrossRef CAS PubMed.
- C. S. Shao, N. Feng, S. Zhou, X. X. Zheng, P. Wang, J. S. Zhang and Q. Huang, Toxicol. Res., 2021, 10, 531–541 CrossRef PubMed.
- M. A. Bashir, C.-S. Shao, M. Abdalla, X. Lin, L. Li, Y. Wu and Q. Huang, J. Mol. Struct., 2024, 1301, 137431 CrossRef CAS.
- L. Ren, Saudi J. Biol. Sci., 2019, 26, 1961–1972 CrossRef PubMed.
- J. Zhu, J. Jin, J. Ding, S. Li, P. Cen, K. Wang, H. Wang and J. Xia, Chem.–Biol. Interact., 2018, 290, 77–87 CrossRef CAS PubMed.
- Z. Liu, L. Li and B. Xue, Eur. J. Pharmacol., 2018, 824, 72–77 CrossRef CAS PubMed.
- D. Deng, P. Sun, C. Yan, M. Ke, X. Jiang, L. Xiong, W. Ren, K. Hirata, M. Yamamoto, S. Fan and N. Yan, Nature, 2015, 526, 391–396 CrossRef CAS PubMed.
- J. Jumper, R. Evans, A. Pritzel, T. Green, M. Figurnov, O. Ronneberger, K. Tunyasuvunakool, R. Bates, A. Žídek, A. Potapenko, A. Bridgland, C. Meyer, S. A. A. Kohl, A. J. Ballard, A. Cowie, B. Romera-Paredes, S. Nikolov, R. Jain, J. Adler, T. Back, S. Petersen, D. Reiman, E. Clancy, M. Zielinski, M. Steinegger, M. Pacholska, T. Berghammer, S. Bodenstein, D. Silver, O. Vinyals, A. W. Senior, K. Kavukcuoglu, P. Kohli and D. Hassabis, Nature, 2021, 596, 583–589 CrossRef CAS PubMed.
- T. D. Goddard, C. C. Huang, E. C. Meng, E. F. Pettersen, G. S. Couch, J. H. Morris and T. E. Ferrin, Protein Sci., 2018, 27(1), 14–25 CrossRef CAS PubMed.
- C. Colovos and T. O. Yeates, Protein Sci., 1993, 2, 1511–1519 CrossRef CAS PubMed.
- A. Stank, D. B. Kokh, J. C. Fuller and R. C. Wade, Acc. Chem. Res., 2016, 49, 809–815 CrossRef CAS PubMed.
- D. Jakubec, P. Skoda, R. Krivak, M. Novotny and D. Hoksza, Nucleic Acids Res., 2022, 50(W1), W593–W597 CrossRef CAS PubMed.
- T. Mohammad, Y. Mathur and M. I. Hassan, Briefings Bioinf., 2021, 22(4), bbaa279 CrossRef PubMed.
- O. Trott and A. J. Olson, J. Comput. Chem., 2010, 31, 455–461 CrossRef CAS PubMed.
- N. M. Mattson, A. K. N. Chan, K. Miyashita, E. Mukhaleva, W. H. Chang, L. Yang, N. Ma, Y. Wang, S. P. Pokharel, M. Li, Q. Liu, X. Xu, R. Chen, P. Singh, L. Zhang, Z. Elsayed, B. Chen, D. Keen, P. Pirrotte, S. T. Rosen, J. Chen, M. A. LaBarge, J. E. Shively, N. Vaidehi, R. C. Rockne, M. Feng and C. W. Chen, Nat. Struct. Mol. Biol., 2024, 31, 465–475 CrossRef CAS PubMed.
- M. Bugnon, U. F. Röhrig, M. Goullieux, M. A. S. Perez, A. Daina, O. Michielin and V. Zoete, Nucleic Acids Res., 2024, 52, W324–W332 CrossRef PubMed.
- M. F. Adasme, K. L. Linnemann, S. N. Bolz, F. Kaiser, S. Salentin, V. J. Haupt and M. Schroeder, Nucleic Acids Res., 2021, 49, W530–W534 CrossRef CAS PubMed.
- K. J. Bowers, E. Chow, H. Xu, R. O. Dror, M. P. Eastwood, B. A. Gregersen, J. L. Klepeis, I. Kolossváry, M. A. Moraes, F. D. Sacerdoti, J. K. Salmon, Y. Shan and D. E. Shaw, ACM/IEEE SC 2006 Conference (SC'06), 2006, pp. 43 Search PubMed.
- M. Sarma, M. Abdalla, J. H. Zothantluanga, F. Abdullah Thagfan, A. K. Umar, D. Chetia, T. N. Almanaa and S. T. Al-Shouli, J. Biomol. Struct. Dyn., 2023, 1–17 Search PubMed.
- W. Humphrey, A. Dalke and K. Schulten, J. Mol. Graphics, 1996, 14(33–38), 27–38 Search PubMed.
- F. A. Ugbe, G. A. Shallangwa, A. Uzairu, I. Abdulkadir, E. I. Edache, W. A. I. Al-Megrin, S. T. Al-Shouli, Y. Wang and M. Abdalla, J. Biomol. Struct. Dyn., 2023, 1–24 CrossRef PubMed.
- B. J. Grant, A. P. Rodrigues, K. M. ElSawy, J. A. McCammon and L. S. Caves, Bioinformatics, 2006, 22, 2695–2696 CrossRef CAS PubMed.
- F. Nwaokorie, M. Abdalla, U. O. Edet, A. M. E. Abdalla, E. Archimedes Okpo, A. Shami, I. U. Bassey, F. J. Tayeb, D. E. Charlie, B. O. David, N. D. A. O. Hajedri, C. P. Archibong, A. B. Oyeyemi and S. C. Alaribe, J. Mol. Struct., 2024, 1316, 138733 CrossRef CAS.
- M. Rudrapal, W. A. Eltayeb, G. Rakshit, A. A. El-Arabey, J. Khan, S. M. Aldosari, B. Alshehri and M. Abdalla, Sci. Rep., 2023, 13, 8656 CrossRef CAS PubMed.
- A. S. Aloufi, A. A. El-Arabey, W. A. Eltayb, R. Elsayim, H. S. Marenga, Y. Modafer, M. E. Awadalla, P. K. Mohapatra, R. K. Mohapatra and M. Abdalla, J. Biomol. Struct. Dyn., 2024, 1–10, DOI:10.1080/07391102.2024.2314745.
- X. Wu, S. Qian, J. Zhang, J. Feng, K. Luo, L. Sun, L. Zhao, Y. Ran, L. Sun, J. Wang and F. Xu, Cancer Metabol., 2021, 9, 23 CrossRef PubMed.
- T. Matsuo, Y. Konya, E. Hirayama and Y. Sadzuka, Oncol. Lett., 2020, 20, 962–966 CrossRef CAS PubMed.
- M. Shinohara, Y. Tashiro, M. Shinohara, J. Hirokawa, K. Suzuki, M. Onishi-Takeya, M. Mukouzono, S. Takeda, T. Saito, A. Fukumori, T. C. Saido, R. Morishita and N. Sato, FASEB J., 2020, 34, 2425–2435 CrossRef CAS PubMed.
- L. Y. Peng, J. Jiang, L. Zhou, E. C. Nice and C. Huang, STAR Protoc., 2022, 3(2) DOI:10.1016/j.xpro.2022.101423.
- Y. Li, Y. Li, C. Ning, J. Yue, C. Zhang, X. He, Y. Wang and Z. Liu, Comput. Biol. Chem., 2022, 98, 107648 CrossRef CAS PubMed.
- B. Kumar, P. Parasuraman, T. P. K. Murthy, M. Murahari and V. Chandramohan, J. Biomol. Struct. Dyn., 2022, 40, 7796–7814 CrossRef CAS PubMed.
- J. Fang, P. Wu, R. Yang, L. Gao, C. Li, D. Wang, S. Wu, A. L. Liu and G. H. Du, Acta Pharm. Sin. B, 2014, 4, 430–437 CrossRef PubMed.
- T. Kitagawa, Y. Tsuruhara, M. Hayashi, T. Endo and E. J. Stanbridge, J. Cell Sci., 1995, 108(Pt 12), 3735–3743 CrossRef CAS PubMed.
- Y. Zhou, Y. Guo and K. Y. Tam, Expert Opin. Ther. Pat., 2022, 32, 441–453 CrossRef CAS PubMed.
- J. Lyu, N. Kapolka, R. Gumpper, A. Alon, L. Wang, M. K. Jain, X. Barros-Álvarez, K. Sakamoto, Y. Kim, J. DiBerto, K. Kim, I. S. Glenn, T. A. Tummino, S. Huang, J. J. Irwin, O. O. Tarkhanova, Y. Moroz, G. Skiniotis, A. C. Kruse, B. K. Shoichet and B. L. Roth, Science, 2024, 384(6702), eadn6354 CrossRef PubMed.
- T. F. Custódio, P. A. Paulsen, K. M. Frain and B. P. Pedersen, Life Sci. Alliance, 2021, 4(4), e202000858 CrossRef PubMed.
- R. S. Ferreira, J. L. Pons and G. Labesse, ACS Omega, 2019, 4, 4748–4760 CrossRef CAS PubMed.
- E. S. Reckzeh and H. Waldmann, Eur. J. Org. Chem., 2020, 2020, 2321–2329 CrossRef CAS PubMed.
- R. S. Ferreira, J.-L. Pons and G. Labesse, ACS Omega, 2019, 4, 4748–4760 CrossRef CAS PubMed.
- F. Ahammad, R. Alam, R. Mahmud, S. Akhter, E. K. Talukder, A. M. Tonmoy, S. Fahim, K. Al-Ghamdi, A. Samad and I. Qadri, Briefings Bioinf., 2021, 22(5), bbab098 CrossRef PubMed.
- B. R. Younes M, M. Stephenson, M. Gondo and P. T. Cagle, Cancer, 1997, 80, 1046–1051 CrossRef PubMed.
- L. Feng, L. Yuan, M. Du, Y. Chen, M. H. Zhang, J. F. Gu, J. J. He, Y. Wang and W. Cao, Molecules, 2013, 18, 9966–9981 CrossRef CAS PubMed.
- D. Martinez Molina, R. Jafari, M. Ignatushchenko, T. Seki, E. A. Larsson, C. Dan, L. Sreekumar, Y. Cao and P. Nordlund, Science, 2013, 341, 84–87 CrossRef PubMed.
- Z. Cui, C. Li, P. Chen and H. Yang, Theranostics, 2022, 12, 1829–1854 CrossRef CAS PubMed.
- X. C. Lv, Q. Wu, Y. J. Cao, Y. C. Lin, W. L. Guo, P. F. Rao, Y. Y. Zhang, Y. T. Chen, L. Z. Ai and L. Ni, Food Funct., 2022, 13, 5820–5837 RSC.
- W. Peng, C. Tan, L. Mo, J. Jiang, W. Zhou, J. Du, X. Zhou, X. Liu and L. Chen, Metabolism, 2021, 123, 154869 CrossRef CAS PubMed.
- C. Janzen, M. Y. Y. Lei, J. Cho, P. Sullivan, B. C. Shin and S. U. Devaskar, Placenta, 2013, 34, 1072–1078 CrossRef CAS PubMed.
- C. V. Iancu, G. Bocci, M. Ishtikhar, M. Khamrai, M. Oreb, T. I. Oprea and J.-y. Choe, Sci. Rep., 2022, 12, 1429 CrossRef CAS PubMed.
|
This journal is © The Royal Society of Chemistry 2024 |
Click here to see how this site uses Cookies. View our privacy policy here.