DOI:
10.1039/D4RA06037G
(Paper)
RSC Adv., 2024,
14, 31797-31808
Lipogenic stearoyl-CoA desaturase-1 (SCD1) targeted virtual screening for chemical inhibitors: molecular docking / dynamics simulation and in vitro assessment of anti-NAFLD efficacy
Received
20th August 2024
, Accepted 1st October 2024
First published on 8th October 2024
Abstract
Amidst rising global prevalence of metabolic syndrome, the associated risk of non-alcoholic fatty liver disease (NAFLD) is also rapidly increasing. The pathogenesis of NAFLD starts with fat accumulation and progresses through inflammation and fibrotic sequel, often involving complex molecular mechanisms involving de novo lipogenesis. Stearoyl-CoA desaturase 1 (SCD1) enzyme, expressed in liver and adipose tissue, converts saturated fatty acids to monounsaturated fatty acids (MUFAs), contributing to triglyceride and cholesterol ester formation. In this study, potential SCD1 inhibitors were screened using the ZINC database of curated medically-approved drugs by virtual screening, molecular docking, and molecular dynamics simulations. The top-scoring five ligands with strong binding affinity against SCD1 were ZINC000003831151 > ZINC000001540998 > ZINC000003830713 > ZINC000000897251 > ZINC000002005305, which showed stable protein-ligand complexation and favorable pharmacokinetic attributes. The top ligand, Montelukast, was experimentally validated for its pharmacological efficacy in an in vitro cell culture model of steatosis (NAFLD). Montelukast showed a dose-dependent decrease in hepatic fat accumulation, reduced levels of free radicals, and lowered oxidative stress (P < 0.05). These outcomes suggest Montelukast to be a potential SCD1 inhibitor, with anti-NAFLD efficacy. These findings open new avenues for therapeutic development of the top 5 ligands in metabolic disorders involving SCD1.
Introduction
Non-Alcoholic Fatty Liver Disease (NAFLD) is a liver spectrum disease with a broad canvas of conditions, from simple lipid build-up in hepatocytes (steatosis) to liver injury (steatohepatitis) and fibrosis. Some individuals eventually develop lewis cirrhosis and liver cancer in advanced stages, which is predicted to become the leading indication for liver transplantation within the next five years.1 The global prevalence of the disease is around 20–25% of the total population.2 Therefore, patients with NAFLD continue to need pharmacological options as no specific drug has been approved by the US Food and Drug Administration (US-FDA), but recently, the Drug Controller General of India (DCGI) approved Sargolitazar Mg, a dual PPARα/γ agonist for the management of NAFLD/Non-Alcoholic Steatohepatitis (NASH).3,4 The key risk factors are in tandem with metabolic syndrome (MetS), such as obesity, insulin resistance, diabetes, and cardiovascular disease.
NAFLD/NASH poses a relevant challenge in elucidating the complex molecular mechanisms that are linked to multiple factors, initiated with an abnormal accumulation of lipid droplets in hepatocytes, which is caused primarily by de novo lipogenesis, a major contributor to steatosis.5 The endoplasmic reticulum bound Δ9-desaturase enzyme, commonly known as stearoyl-CoA desaturase 1 (SCD1), has four isoforms in mice (SCD1, SCD2, SCD3, SCD4), two in rats (SCD1 and SCD2), and two in humans (SCD1 and SCD5), with SCD1 expressed in liver and adipose tissue. SCD1 is a rate-limiting enzyme in both hepatic lipogenesis and mitochondrial lipid oxidation. This enzyme converts saturated fatty acids into mono-saturated fatty acids, which subsequently form triglycerides and cholesterol esters and is thought to be a key mediator in disease pathogenesis.6
The overexpression of SCD1 in hepatic steatosis could be a potential target for metabolic disorders and its complications. In experimental research, a knockout of the SCD1 (Scd1−/−) gene in mice resulted in an increased mitochondrial beta-oxidation through activating AMPK in hepatocytes.7 Calorie restriction diet ameliorates hepatic steatosis by accelerating breakdown of fats and increase the utilization of lipid by lowering lipoprotein and triglyceride production, which is regulated by carbohydrate and SCD1 axis.8 Although, a number of known SCD1 inhibitors have been identified, only a few have advanced to clinical trials due to their lack of specificity and limited safety. Aramchol is a cholic acid and arachidic acid conjugate that has been shown to reduce fat accumulation in hepatocytes by lowering SCD1 and is in phase III clinical trial.9 There is scope and need for identifying newer desaturase inhibitors, which can be partly achieved through drug-repurposing approach of target-based screening of ligand databases.
In the present study, SCD1 target-based high-throughput virtual screening (HTVS) was performed using the FDA-approved drugs listed in the ZINC database, to identify potential SCD-1 inhibitors. Ranking of the potential SCD1 inhibitors was based on computational methods, which included multistep molecular docking, molecular dynamics simulations, and pharmacokinetic profiling. The top ligand was then experimentally validated for controlling lipid accumulation in an in vitro cell culture model of NAFLD.
Materials and methods
The computational studies were carried out using Maestro module of Schrodinger software (v13.3, Schrodinger LLC, NY, USA). Molecular dynamics (MD) simulation was performed using Desmond module of Schrodinger software developed by D. E. Shaw (ver. 6.5) on a Tyrone workstation installed with Linux platform (Ubuntu 22.04 LTS), equipped with 160 GB RAM and 11 GB of NVIDIA graphics (GeForce RTX 208 Ti).
Human hepatoma cells (HepG2) were obtained from the National Centre for Cell Science (NCCS Pune, India), antibiotic and Minimum Essential Medium (MEM) was purchased from Gibco Life Technologies (Carlsbad, USA), fetal bovine serum (FBS), oleic acid, and Oil Red O (ORO) powder were from HiMedia (Mumbai, India). Dimethyl sulfoxide (DMSO), potassium hydroxide, monopotassium phosphate, sodium hydroxide, trichloroacetic acid (TCA), and 2-thiobarbituric acid (TBA) were purchased from Sigma-Aldrich (Schnelldorf, Germany) and were of the highest analytical grade.
Protein and ligand preparation
The protein structure of Stearoyl-CoA desaturase-1 (SCD-1) (PDB code: 4ZYO, resolution: 3.25 Å) was obtained from RCSB Protein Data Bank,10 and was prepared using the Schrodinger's protein preparation workflow. The protein preparation was done by addition of hydrogen atoms, removal of water molecules, production of het states at pH 7.0 ± 2.0, formation of disulphide bonds and forming zero-order connections with metal atoms using Epik module. The pre-processed protein was optimized and minimized using Optimized Potentials for Liquid Simulations-4 (OPLS4) force field. The grid box was generated based on the native substrate pose coordinates (X = 17.42, Y = 70.80, Z = 28.91) in the workspace of Receptor grid generation wizard. The grid size (inner box = 10, 10, 10 and outer box = 35.77, 35.77, 35.77) was set and no constraints were imposed on binding pocket atoms of the protein.11 The LigPrep module of Schrödinger was used to prepare the ligands, to generate potential isomers, tautomers and isomeric forms of the 3D conformation at pH 7.0 ± 2.0, the ionization state was neutralized by addition or removal of protons from the ligand. Finally, using the OPLS4 force field, minimization of the ligands was done to complete the process.12
Virtual screening and molecular docking study
Molecular docking was performed for FDA approved drugs obtained from ZINC database (2115 molecules) using the glide module of Schrodinger.13 Initially to perform High Throughput Virtual Screening (HTVS), all prepared ligands were docked at the binding site of the target protein (PDB ID: 4ZYO). The molecules that could enter active site were further screened by performing standard precision (SP) mode, and further by extra precision (XP) mode of docking. Docking analysis was visualized using Maestro module of the software. The binding affinity of ligands towards 4ZYO's active site was calculated using docking binding energy using the relation ΔG = −RT
ln
Kd (G= docking binding energy, T = temperature, R = Boltzmann gas constant = 1.987 cal mol−1 K−1 and Kd = docking binding affinity).14 The prime MM-GBSA calculations were performed for the top 10% of XP results using default variable dielectric solvation generalized Born (VSGB) solvent model and OPLS4 force field to generate energy properties. These properties help calculate ligand and receptor strain and binding energies, which were calculated using the equation
ΔGbind = free energy utilized by ligand L to bind with protein P to form PL complex.15
Inhibition constant (Ki)
Inhibition constant (Ki), was calculated using the equation Ki = exp(ΔG/RT) where ΔG is the binding energy, R is the universal gas constant (1.985 × 10−3 kcal mol−1 K−1), and T is the temperature (298.15 K).16
In silico ADMET prediction and drug likeness study
Top scoring ligands in the docking studies were in silico evaluated for absorption, distribution, metabolism, excretion, and toxicity (ADMET) parameters, using the pkCSM online tool.17
Molecular dynamics (MD) study
Further to assess the conformational stability of the protein–ligand complex (PLC), Molecular dynamics (MD) was performed using Desmond module, D. E. Shaw research group (Academic License, Version 2020-4).18 The PLC was subjected to transferable intermolecular potential with 3 points (TIP3P) solvent model within a 10 Å boundary box. The system was further neutralized using counter ions, and achieving salt concentration of the physiological system (0.15 M). Further, the complex was minimized and equilibrated with isobaric–isothermal ensemble (NPT) set at 310 K, and pressure 1.01 bar, and the MD stimulation was run for 100 ns using OPLS force field.19,20 Conclusively, a stimulation interaction diagram (SID) was generated which provided parameters such as Root Mean Square Deviation (RMSD), Root Mean Square Fluctuation (RMSF) of the complex and protein–ligand interaction data of the dynamics study.
Experimental induction of steatosis in HepG2 cells in vitro
To induce the steatosis model, HepG2 cells were seeded into a 96-well plate at a density of 5 × 105 cells per well and grown to 80% confluency. The cells were then serum-starved for 24 h. Next, they were treated with MEM media containing 1 mM oleic acid conjugated with 1% BSA and incubated for 48 h.
Cell viability assay
The 3-(4, 5-dimethylthiazolyl-2)-2, 5-diphenyltetrazolium bromide (MTT) assay was done in HepG2 cells to evaluate cellular cytotoxicity induced by Montelukast. Once confluency was reached, cells were trypsinized (0.25% trypsin) and seeded into a 96-well plate with a density of 8 × 103 cells per well. Montelukast was dissolved in dimethyl sulfoxide (DMSO remained below 1%) and further diluted in culture media to final concentrations ranging from 5 μg mL−1 to 25 μg mL−1. After 24 h incubation with Montelukast, the cells were treated with MTT dye (5 mg mL−1 in PBS) and incubated for an additional 3 h. Metabolically active cells converted the yellow MTT substrate into purple formazan crystals, which were dissolved using DMSO. The amount of formazan produced, proportional to the number of viable cells, was measured spectrophotometrically at 570 nm. Then, cell viability was calculated using the formula: (mean absorbance of viable cells)/(mean absorbance of control cells) × 100.
Oil Red O (ORO) staining
Following the induction of steatosis in HepG2 cells, the non-cytotoxic dosage of Montelukast (10 μg mL−1 and 15 μg mL−1) was administered to cells for 24 h. Oil Red O (ORO) staining was performed to assess lipid droplet accumulation in steatotic-HepG2 cells. The cells were fixed with 4% paraformaldehyde at 37 °C for 15–20 min. After fixation, the cells were washed with phosphate buffer to remove any residual fixative in the well. The fixed cells were then incubated with a 0.3% ORO working solution prepared by diluting in isopropanol for 30 min. Following incubation, the cells were carefully washed twice with autoclaved distilled water to remove any unattached dye from the monolayer's surface. The stained lipid droplets in the hepatocytes were visualized and examined under an inverted light microscope. Finally, the lipid-bound dye was dissolved with a 100% isopropanol solution and quantified at 490 nm using a spectrophotometer.
Lipid peroxidation assay
To assess the impact of Montelukast on oxidative stress associated antioxidant activity, the thiobarbituric acid reactive substances (TBARS) assay was performed in a steatotic model. This assay measures malondialdehyde (MDA) levels, a marker of lipid peroxidation and oxidative damage. Steatotic HepG2 cells were treated with Montelukast (10 μg mL−1 and 15 μg mL−1) for 24 h and compared with the untreated steatotic disease model. While, the control group consisted of the untreated disease model. Following treatment, cells were washed and lysed using lysis buffer. The resulting lysate was homogenized, centrifuged and the supernatant was collected, and protein content was normalized for each group. A mixture of 350 μl of Tris buffer, 50 μl of monopotassium phosphate, and 50 μl of cell lysate was mixed and incubated to assess lipid peroxidation. Subsequently, 250 μl of trichloroacetic acid (TCA) and 375 μl of thiobarbituric acid (TBA) were added, and the resulting mixture was heated in a water bath for 15 min to generate a pink-coloured adduct. The absorbance of the adduct was measured spectrophotometrically at 530 nm, and the obtained values were compared to a standard MDA curve.21
2′,7′-Dichlorodihydrofluorescein diacetate (DCFDA) assay
A DCFDA assay was performed to evaluate the intracellular reactive oxygen species (ROS) and reactive nitrogen species (RNS) levels. Steatotic HepG2 cells were treated with Montelukast (10 μg mL−1 and 15 μg mL−1) for 24 h, with an untreated disease model serving as the control group. Following treatment, the cells were loaded with 10 μM DCFDA and incubated in the dark for 60 min. The fluorescence intensity, which reflects the levels of ROS/RNS, was measured using a microplate reader with excitation at 485 nm and emission at 535 nm.
Statistical analysis
The in vitro experiments were performed in three independent experiments, each in triplicate sets. Statistical analysis was performed using one-way ANOVA to compare the experimental groups, and their statistically significant differences in values were expressed as P < 0.05 (*/#), P < 0.01 (**/#), and P < 0.001 (***/###).
Results and discussion
Molecular docking study
The molecular docking analysis reveals significant binding interactions between the ligands and the target protein, taking Aramchol as a reference standard. Table 1 shows docking, MMGBSA scores, and inhibition constant (Ki) of the top five ligands, and Table 2 includes amino acid interactions of the top ligands. The first top-hit compound ZINC000003831151 (Montelukast, a drug used for the treatment of chronic asthma and the prevention of exercise-induced bronchoconstriction), exhibited the highest binding affinity with a docking score of −13.577 kcal mol−1 and MMGBSA dG bind of −104.22 kcal mol−1. It forms hydrogen bonds with ARG 74, ASN 148, and GLH 152, a salt bridge with ARG 74, aromatic bonds with TRP 153, and Pi–Pi interactions with TRP 184 and HIS 171. The second top hit compound ZINC000001540998 (Pemetrexed, new-generation antifolate, approved for the treatment of mesothelioma and non-small cell lung cancer) has a docking score of −12.330 kcal mol−1 and MMGBSA dG bind of −53.31 kcal mol−1, interacting through hydrogen bonds with ARG 74, ASN 148, THR 261, and TRP 262, a salt bridge with ARG 74, and Pi–Pi interactions with TRP 153 and TRP 184. The third top-hit compound, ZINC000003830713 (Dinoprostone, drug used to induce labor or abortion as well as to treat non-metastatic gestational trophoblastic disease), showed a docking score of −11.760 kcal mol−1 and MMGBSA dG bind of −78.36 kcal mol−1, forming hydrogen bonds with ASN 148, HIS 171, and ASN 265. The fourth top-hit compound ZINC000000897251 (Donepezil, drug used for the treatment of dementia of the Alzheimer's disease), has a docking score of −11.704 kcal mol−1 and MMGBSA dG bind of −78.90 kcal mol−1, with Pi–cation interactions with PHE 146, hydrogen bonds with ASN 148, and Pi–Pi interactions with TRP 153 and TRP 184. The fifth top-hit compound ZINC000002005305 (levomefolic acid, drug used for the treatment and prevention of folate deficiency and antidote against folic acid antagonists), displayed a docking score of −11.333 kcal mol−1 and MMGBSA dG bind of −74.40 kcal mol−1, interacting via hydrogen bonds with ASN 75, TRP 153, and ASN 265, aromatic bonds with PHE 146 and ASN 148, and Pi–Pi interactions with TRP 184. The reference standard, Aramchol (FDA approved first-in-class, potentially disease modifying treatment for NASH) showed the lowest docking score of −8.687 kcal mol−1 but a relatively strong MMGBSA dG bind of −82.78 kcal mol−1, interacting through hydrogen bonds with ARG 74, LYS 189, and LYS 194, and forming a salt bridge and hydrogen bond with GLH 152.
Table 1 Docking results and inhibition constant (Ki) of top five ligands
S. no. |
Ligands |
Docking score (kcal mol−1) |
MMGBSA (dG bind) |
Inhibition constant (Ki) nM |
1 |
ZINC000003831151 (Montelukast) |
−13.57 |
−104.22 |
0.111 |
2 |
ZINC000001540998 (Pemetrexed) |
−12.33 |
−53.31 |
0.905 |
3 |
ZINC000003830713 (Dinoprostone) |
−11.76 |
−78.36 |
2.37 |
4 |
ZINC000000897251 (Donepezil) |
−11.70 |
−78.90 |
2.62 |
5 |
ZINC000002005305 (Levomefolic acid) |
−11.33 |
−74.40 |
4.90 |
6 |
Reference (Aramchol) |
−8.68 |
−82.78 |
430 |
Table 2 Amino acid interactions of top five ligands
S. no. |
Ligands |
Residue |
Type of interaction |
Distance (Å) |
1 |
Montelukast |
ARG 74 |
H-bond |
1.72 |
ASN 148 |
Salt bridge |
2.81 |
GLH 152 |
Aromatic bond |
2.40 |
TRP 153 |
H-bond |
2.26 |
TRP 184 |
Pi–Pi |
3.97, 4.27, 4.39 |
HIS 171 |
Pi–Pi |
5.17 |
|
Pi–Pi |
4.95 |
2 |
Pemetrexed |
ARG 74 |
H-bond |
1.63 |
ASN-148 |
Salt-bridge |
3.53 |
TRP 153 |
Aromatic bond |
3.28 |
TRP 184 |
Pi–Pi |
3.93 |
THR 261 |
Pi–Pi |
5.06 |
TRP 262 |
H-bond |
2.54, 2.04 |
|
H-bond |
2.13 |
3 |
Dinoprostone |
ASN 148 |
H-bond |
2.42, 2.18 |
HIS 171 |
H-bond |
2.22 |
ASN 265 |
H-bond |
1.97 |
4 |
Donepezil |
PHE 146 |
Pi–cation |
4.53 |
ASN 148 |
H-bond |
2.29 |
TRP 153 |
Pi–Pi |
4.12 |
TRP 184 |
Pi–Pi |
5.43 |
5 |
Levomefolic acid |
ASN 75 |
H-bond |
1.81 |
PHE 146 |
Aromatic bond |
2.61 |
ASN 148 |
Aromatic bond |
3.02 |
TRP 153 |
H-bond |
2.24 |
TRP 184 |
Pi–Pi |
4.31, 3.84 |
ASN 265 |
Pi–Pi |
5.17, 5.07 |
|
H-bond |
1.79 |
6 |
Reference (Aramchol) |
ARG 74 |
H-bond |
2.16 |
LYS 189 |
H-bond |
1.74 |
LYS 194 |
H-bond |
1.97 |
GLH 152 |
Salt bridge |
4.69 |
|
H-bond |
2.21 |
Common interactions among these ligands include hydrogen bonds with key residues like ARG 74 and ASN 148, and Pi–Pi interactions with TRP 153 and TRP 184. These residues appear crucial for strong binding, highlighting potential hotspots for effective binding in the protein target. The robust interaction network, especially in top hit ligands like Montelukast, underscores their superior binding affinities compared to the reference standard Aramchol. Fig. 1 shows the 2D ligand–protein interaction diagrams of the top-scoring ligands.
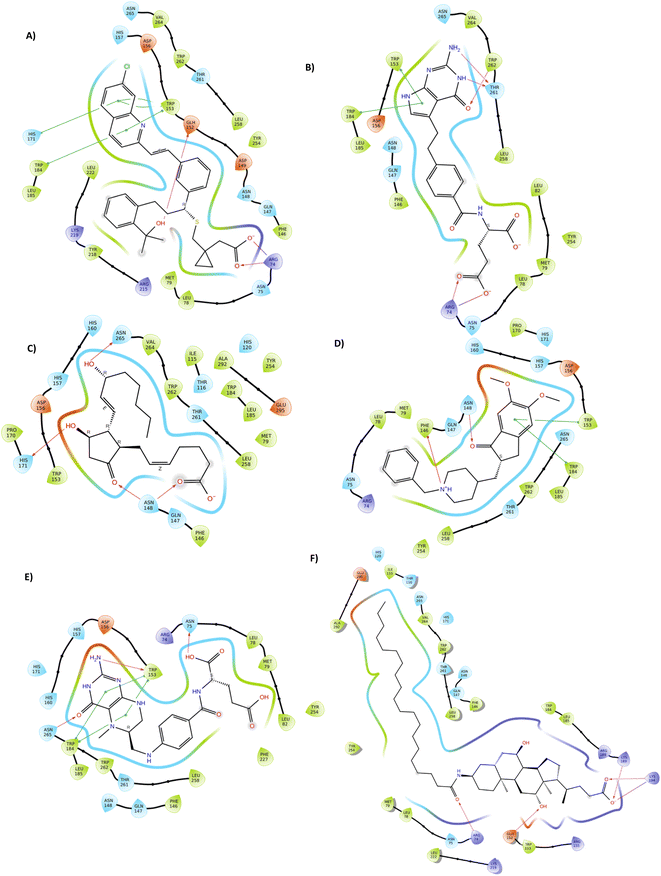 |
| Fig. 1 Representation of 2D docked poses of top scoring ligands and reference standard with 4ZYO ((A)-Montelukast, (B)-Pemetrexed, (C)-Dinoprostone, (D)-Donepezil, (E)-Levomefolic acid, (F)-Aramchol). | |
The inhibition constant (Ki) reflects the molecular affinity between the enzyme's active site and the inhibitor, with Ki being inversely proportional to enzyme affinity.22,23 Consequently, a lower Ki value indicates a stronger inhibitor. In this study, the Ki values for the SCD1 inhibitors ranked as follows: Montelukast > Pemetrexed > Dinoprostone > Donepezil > Levomefolic acid > Aramchol.
In silico ADMET prediction and drug-likeness study
ADMET characteristics play a crucial role in determining if a compound will be suitable for potential drug candidate. For a drug to be successful, it must meet specific ADMET criteria, yet more than half of all drugs fail to meet these standards. Drug absorption is assessed by considering factors like skin permeability, intestinal absorption, and whether the drug is a P-glycoprotein substrate or inhibitor. Drug distribution is influenced by parameters such as blood–brain barrier permeability (log
BB), CNS permeability, and volume of distribution (VDss). Metabolism is predicted using CYP models for various enzymes (CYP2D6, CYP3A4, CYP1A2, CYP2C19, CYP2C9, and CYP2D6). Excretion is determined by renal clearance and Organic Cation Transporter-2 (OCT2) substrate properties. Drug toxicity is evaluated based on human Ether-à-go-go-Related Gene (hERG) inhibition, hepatotoxicity, skin sensitization, and Salmonella typhimurium reverse mutation assay (AMES) toxicity. Several parameters and their corresponding standard values were considered in the current analysis. High Caco-2 permeability is indicated by a predicted value >0.90, poor intestinal absorption is < 30%, low VDss is < 0.71 L kg−1, high VDss is > 2.81 L kg−1, BBB permeability (log
BB) > 0.3 indicates ability to cross the BBB, and log
BB < −1 indicates poor distribution. CNS permeability, measured by log
PS, is > −2 for CNS penetration, while log
PS < −3 indicates inability to permeate the CNS.
Table 3 summarizes the predicted ADMET properties of the top ligands. Based on the ADMET analysis, the top hit compound Montelukast exhibits a high predicted intestinal absorption rate of 91.674%, ensuring good absorption. Although, it has low Caco-2 permeability (0.253) and a low distribution volume (VDss of −1.137), indicating limited distribution, it cannot cross the blood–brain barrier (log
BB of −1.137) or permeate the central nervous system (log
PS of −1.508), this could be advantageous for targeting non-CNS conditions. The ligand Donepezil exhibits high Caco-2 permeability (1.321), good intestinal absorption (92.768%), and the ability to cross the blood–brain barrier (BBB) with a log
BB of 1.179. However, it cannot permeate the central nervous system (CNS) with a log
PS of −1.445. Levomefolic acid also shows high Caco-2 permeability (0.931) but poor intestinal absorption (5.645%) and cannot cross the BBB (log
BB of −0.565), although it can permeate the CNS (log
PS of 4.212). The drug Dinoprostone has high Caco-2 permeability (0.962) and good intestinal absorption (53.297%) but cannot cross the BBB or permeate the CNS. The drugs Pemetrexed and Montelukast both demonstrate low Caco-2 permeability and cannot cross the BBB or permeate the CNS, and the compound Pemetrexed also has poor intestinal absorption (15.709%). The reference standard Aramchol, while having good intestinal absorption (74.202%), shows low Caco-2 permeability (0.321) and cannot cross the BBB or permeate the CNS.
Table 3 In silico predicted ADMET properties of top scoring ligands and reference standard Aramchola
|
Lipinski's rule of five |
Absorption |
Distribution |
Metabolism |
Excretion |
Toxicity |
MW |
Log P |
RB |
HBA |
HBD |
Water solubility (log mol L−1) |
Caco2 permea-bility (% absorbed) |
Intestinal permea-bility (% absorbed) |
Skin permeability (log Kp) |
P-glycol-protein subst-rate |
P-glycol-protein inhibi-tor |
VDss (log L kg−1) |
Fraction unbound (Fu) |
BBB permeability (log BB) |
CNS permeability (log PS) |
CYP substrate |
CYP inhibitor |
Total clearance (log mg per kg per day) |
Renal OCT2 subst-rate |
AMES toxicity |
Hepato-toxicity |
MW – molecular weight, log P – partition coefficient, RB – rotatable bond, HBA – hydrogen bond acceptor, HBD – hydrogen bond donor, Caco2 – Cancer coli-2, BBB – blood–brain barrier, VDss – volume of distribution at steady state, CNS – central nervous system, OCT2 – organic cation transporter 2, CYP – cytochromes P450. |
Montelukast |
586.19 |
8.94 |
12 |
4 |
2 |
−4.43 |
0.25 |
91.67 |
−2.73 |
Yes |
No |
−1.13 |
0.07 |
−0.07 |
−1.51 |
Yes |
No |
−0.14 |
No |
Yes |
Yes |
Pemetrexed |
427.41 |
0.66 |
9 |
6 |
6 |
−2.90 |
−0.82 |
15.70 |
−2.73 |
Yes |
No |
−0.83 |
0.20 |
−1.59 |
−4.03 |
Yes |
No |
0.33 |
No |
No |
Yes |
Dinoprostone |
352.47 |
3.25 |
12 |
4 |
3 |
−2.79 |
0.96 |
53.29 |
−2.73 |
No |
No |
−1.04 |
0.22 |
−0.94 |
−2.91 |
No |
No |
1.75 |
No |
No |
No |
Donepezil |
379.50 |
4.36 |
6 |
4 |
0 |
−4.63 |
1.32 |
92.76 |
−2.64 |
Yes |
Yes |
1.17 |
0.01 |
0.48 |
−1.44 |
Yes |
Yes |
0.99 |
Yes |
No |
Yes |
Levomefolic acid |
459.46 |
−0.25 |
9 |
9 |
7 |
−2.85 |
−0.93 |
5.65 |
−2.73 |
Yes |
No |
−0.56 |
0.47 |
−1.89 |
−4.21 |
No |
No |
0.16 |
No |
No |
Yes |
Aramchol |
702.12 |
10.61 |
23 |
4 |
4 |
−3.68 |
0.32 |
74.20 |
−2.73 |
Yes |
Yes |
−1.53 |
0.12 |
−1.21 |
−2.61 |
Yes |
No |
0.89 |
No |
No |
Yes |
Molecular dynamics (MD) simulation studies
The MD study was performed for Montelukast, Pemetrexed, Dinoprostone, Donepezil and Levomefolic acid, these top molecules as they showed higher or comparable MMGBSA energy to that of reference standard Aramchol. Fig. 2A and B shows RMSD of protein and ligands.
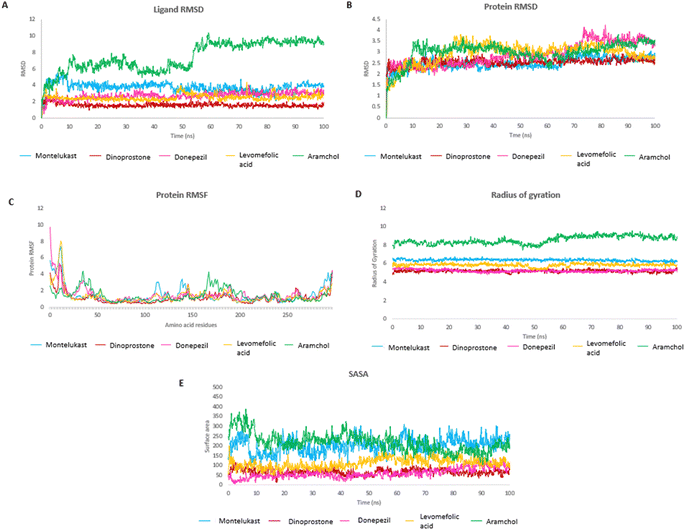 |
| Fig. 2 Analysis of molecular dynamics results (A) ligand RMSD plot (B) protein RMSD plot (C) protein RMSF plot (D) protein radius of gyration (Rg) plot (E) solvent accessible surface area (SASA) plot. | |
The protein RMSD was found to be in a range of 1–4 Å. The compounds Montelukast, and Dinoprostone showed similar RMSD range between 1.5–3.0 Å during the initial 40 ns. The compounds Montelukast and Dinoprostone stayed in the same range, up to 100 ns, whereas compound Donepezil fluctuated up to 4 Å at 76 ns. The compound Levomefolic acid and standard Aramchol showed higher protein RMSD values compared to others. The compound Levomefolic acid took 20 ns for stabilization whereas Aramchol took 10 ns, and further both showed protein RMSD values between 2.5–3.5 Å till the end of the stimulation period.
In case of ligand RMSD values, Montelukast in the initial 10 ns reached up to 5 Å then post 10 ns it got stabilized and RMSD was between 3.0–4.5 Å throughout the stimulation period. It showed low RMSD values from 2–4 Å between 75 to 90 ns and eventually it stabilizes at the end. The compound Dinoprostone initially after stabilization up to 10 ns showed RMSD between 1–2 Å, with in between minute spikes at 53 and 57 ns up to 2.5 Å. The compound Donepezil showed a stable RMSD between 2.0–3.5 Å throughout the stimulation after the initial stabilization. The compound Levomefolic acid showed RMSD between 2–3 Å from 1 to 50 ns. There were spikes up to 3.5 Å which towards the end from 80 to 100 ns stabilized and RMSD stayed between 2–3 Å. Aramchol showed higher RMSD values compared to the ligands up to 10 Å at 60 ns, further it reached a stable period till the end of stimulation period it showed a stable RMSD between 8–10 Å.
In case of Montelukast, it showed key interactions with TRP 262 (H-bond, 92%), TRP 184 (Pi–Pi, 33%), PHE 146 (Pi–Pi, 42%) and HIS 171 (Pi–Pi, 10%). Other water mediated interactions were showed by ASN 75 (11%), ARG 74 (17%), LYS 189 (13%) and LYS 219 (14%). While Dinoprostone showed major interactions with HIS 171 (H-bond, 97%), HIS 157 (H-bond, 92%) and ASN 148 (H-bond, 82%). Other residues which showed water mediated interactions were PHE 146 (39%), GLN 147 (34%), HIS 298 (30%), ASP 156 (66%) and HIS 269 (19%). The compound Donepezil showed H-bond interactions with ASN 148 (33%), HIS 157 (12%), ASN 265 (11%). Hydrophobic Pi–Pi interactions were shown by TRP 153 (75%), TRP 184 (55%) and PHE 146 (29%). Water mediated interactions were shown by ASP 156 (61%) and LEU 185 (49%). Major H-bond interactions shown by the compound Levomefolic acid were TRP184 (96%), ASN 265 (58%), ASN 75 (57%) and GLN 147 (19%). Other hydrophobic interactions were shown by HIS 157 (37%), TYR 254 (10%) and HIS 171 (20%). Standard Aramchol showed key interactions with TRP 262 (H-bond, 45%), GLU 152 (H-bond, 40%), ASN 148 (H-bond, 34%), LYS 194 (H-bond, 29%) and ASN 75 (H-bond, 25%). Some other water mediated interactions were shown by ARG 155 (31%), TYR 254 (28%) and ARG 74 (10%). Fig. 3A and B shows the 2D interaction diagram and ligand–protein contact plot of the ligands, respectively.
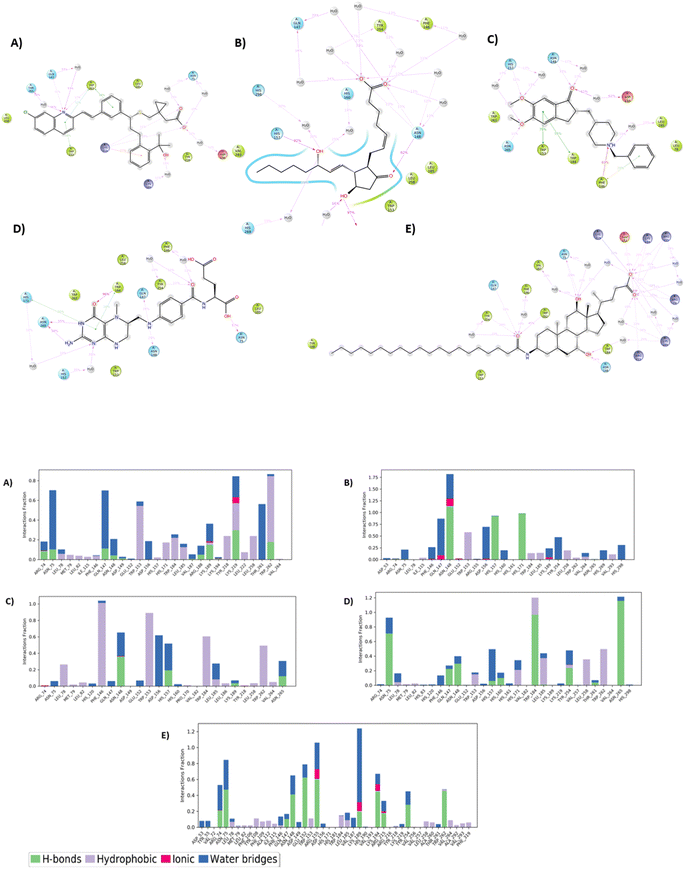 |
| Fig. 3 (A) 2D amino acid residue interaction diagrams of ligands after molecular dynamics stimulation (A-Montelukast, B-Dinoprostone, C-Donepezil, D-Levomefolic acid, E-Aramchol). (B) Protein ligand contact plot of the ligands (A-Montelukast, B-Dinoprostone, C- Donepezil, D-Levomefolic acid, E-Aramchol). | |
The RMSF plot helps to determine stability of the interacting residues of the protein chain. Fig. 2C shows the RMSF plot of the protein residues. Residues at the start and terminal of chain showed higher fluctuations. The RMSF of interacting residues of the test compounds were within 3 Å, Aramchol showed RMSF up to 4 Å for some residues.
The radius of gyration (Rg) determines the compactness of the protein during the stimulation. Fig. 2D shows that reference standard Aramchol showed higher radius of gyration 7.5–8.5 Å compared to other ligands. The compounds Montelukast and Levomefolic acid showed radius of gyration between 5.5–6.5 Å. The compounds Donepezil and Dinoprostone showed radius of gyration between 4–6 Å. Fig. 2E represents the Solvent accessible surface area (SASA) plot of the molecules. It represents that the complexes were stable throughout the stimulation period without any significant conformational changes. ZINC00000383115 and Aramchol showed higher SASA values than other compounds. Fig. 4 shows the snapshots of time-frame analysis performed in order to determine the conformational changes of montelukast throughout the MD simulation trajectory at regular time intervals. Observations at every 20 ns were analysed for the variation of ligand from its binding pose. The results suggest that all five complexes of montelukast studied contribute to the high stability with minimal conformational changes at the active site of SCD1. Fig. 5 depicts the timeline of amino residue contacts with montelukast throughout the MD stimulation duration.
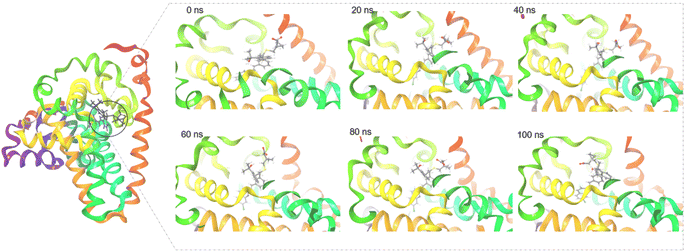 |
| Fig. 4 The surface view of SCD1 with Montelukast snapshots taken at 0 ns (docked pose), 20 ns, 40 ns, 60 ns, 80 ns and 100 ns of the MD stimulation. | |
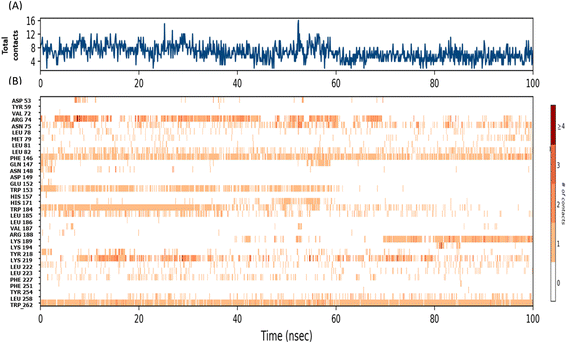 |
| Fig. 5 A timeline representation of the interactions and contacts with amino acid residues during MD simulations (A) Represents the total contacts between the amino acid residues and the drug (B) Represents the details of specific amino acid contacts during the course of MD simulations of 100 ns. | |
Montelukast suppresses intracellular neutral-lipid accumulation and oxidative stress in HepG2 cells
Before evaluating the anti-steatotic effect of Montelukast, its safe dosage was determined by assessing dose-dependent effects on HepG2 cell viability using an MTT assay. We identified non-cytotoxic doses of Montelukast (10 and 15 μg mL−1) for further in vitro studies (Fig. 6A). Steatosis was induced in hepatocytes through oleic acid supplementation. Treatment with different doses of the drug for 24 h led to a concentration-dependent decrease in lipid accumulation, evidenced by reduced ORO staining. ORO specifically stains neutral lipids, such as triglycerides and cholesteryl esters, which were quantified spectrophotometrically at 490 nm. Fig. 6B and C demonstrates a percentage decrease in lipid depots with increasing concentrations of Montelukast. Dosages of 10 and 15 μg mL−1 resulted in 6.39% and 15.43% decrease in lipid accumulation in the steatotic cells, respectively.
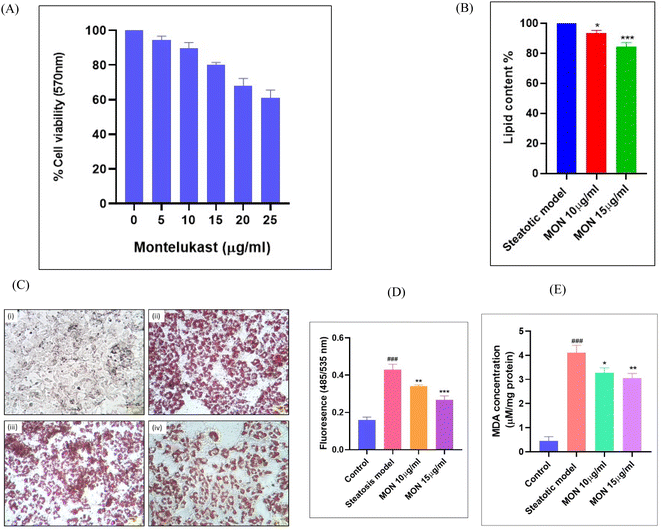 |
| Fig. 6 Inhibitory effect of Montelukast on lipid accumulation and its antioxidant efficacy in an in vitro model of NAFLD (A) dose-dependent changes in cell viability on HepG2 cells exposed to different concentrations of Montelukast. (B) Quantification of ORO staining showed dose-dependent inhibition of lipid accumulation. It has been expressed as percentage reduction in lipid content compared to steatotic model. (C) Photomicrographs (40×, scale bar 50 μm) reveal differential ORO staining between control, steatotic cells, and in treated cells as follows – (i) non-steatotic HepG2 cells (control), (ii) steatotic cells, (iii and iv) steatotic cells treated with 10–15 μg mL−1 of Montelukast. (D) Quantification of total ROS/RNS levels by DCFDA assay in the Montelukast-treated groups (E) reduction in MDA levels in the treated groups, confirming the anti-NAFLD protection by Montelukast. Non-steatotic HepG2 cells were used as the control cells. Values are expressed as mean ± S.D. in three independent experiments, each done in triplicate. Statistical comparisons are as follows: #-with respect to control; *-with respect to steatosis model. ###P < 0.001, relative to the control group. *P < 0.05, **P < 0.01 and ***P < 0.001, relative to the steatosis model. | |
Using the DCFDA assay, we identified that fluorescence intensity, reflecting total intracellular ROS/RNS production, was significantly higher (P < 0.001) in the steatosis model. Treatment with montelukast led to a dose-dependent reduction in fluorescence intensity of 20.59% and 28.94% with 10 and 15 μg mL−1 of Montelukast, respectively shown in Fig. 6D. The lipotoxicity induced by steatosis resulted in oxidative stress in the hepatocytes, as indicated by a significant increase (P < 0.001) in malonaldehyde levels in the disease group (Fig. 6E). This oxidative stress-mediated lipid peroxidation was suppressed in the Montelukast-treated cells. Here, we observed a reduction in lipid peroxidation by 20.34% and 25.76% when treated with 10 and 15 μg mL−1 of Montelukast, respectively.
Montelukast, a leukotriene receptor antagonist FDA-approved drug primarily used for asthma and allergic rhinitis, is now gaining attention for its potential in treating metabolic syndrome. Previously, it has been reported that Montelukast possesses anti-inflammatory and antioxidant properties, which aid in reducing systemic inflammation and oxidative stress,24–26 both being key factors in MetS. A recent clinical trial investigating Montelukast as a treatment for NASH demonstrated significant improvements, wherein participants showed reduced liver enzymes (aminotransferases), decreased liver stiffness, and improved metabolic parameters. Additionally, the trial reported a decrease in levels of inflammatory markers (TGF-β1 and TNF-α), as well as a reduction in oxidative stress.27 Earlier reports demonstrated that Montelukast exhibits free radical scavenging properties, thereby protecting the liver from lipopolysaccharide-induced hepatotoxicity and oxidative stress in rats.28,29
To summarize, the in silico findings provide the basis for pharmacological inhibition of SCD through favourable binding interactions with the enzyme active site and stabilization of the enzyme drug complex followed by a safe pharmacokinetic profile. The experimental outcomes of the present study provide a potential mechanism for the above protective anti-steatotic mechanism of Montelukast through inhibition of the lipogenic enzyme, SCD1. The oxidative stress and inflammatory sequel of steatosis is also ameliorated with Montelukast treatment.
Conclusion
SCD1, one of the key lipogenic enzymes, is garnering interest as a molecular target in metabolic diseases. Here, using drug repurposing approach of screening large database of clinically approved drugs, five top scoring ligands were identified as potential inhibitors of SCD1, wherein the hit candidate was Montelukast. The drug interaction with the SCD1 protein showed stable complex formation during the MD simulations, and a safe ADMET profile. The in vitro validation of Montelukast demonstrated significant reduction in hepatic fat accumulation, decreased levels ROS and oxidative stress, in steatotic HepG2 cells, suggesting potential anti-NAFLD efficacy. The results of this study point to further exploration of SCD1 as a target and Montelukast as a potential pharmacological agent in treating MetS and its complications such as cancers.
Abbreviations
SCD1 | Stearoyl-CoA Desaturase 1 |
NAFLD | Non-Alcoholic Fatty Liver Disease |
NASH | Non-Alcoholic Steato-Hepatitis |
MetS | Metabolic Syndrome |
MD | Molecular Dynamics |
HTVS | High Throughput Virtual Screening |
PLC | Protein Ligand Complex |
SP | Standard Precision |
XP | Extra Precision |
PDB | Protein Data Bank |
OPLS | Optimized Potentials for Liquid Simulations |
ROS | Reactive Oxygen Species |
DCFDA | 2′,7′-Dichlorodihydrofluorescein Diacetate |
MDA | Malondialdehyde |
ORO | Oil red O |
MTT | 3-(4,5-Dimethylthiazol-2-yl)-2,5-diphenyltetrazolium bromide |
Data availability
The original contributions presented in the study are included in the article. Further inquiries can be directed to the corresponding author.
Author contributions
PRD, SM, SP, SK: conceptualization; SP, SK, MMR, SKM: methodology & experimentation; SP, SK, MMR, SKM: data curation software; SP, SK, PRD, SM: writing and original draft preparation; SP, SK: software and data validation; PRD, SM, PKS: visualization, investigation supervision; SP, SK, MMR, SKM, PRD, SM, PKS: writing – reviewing and editing.
Conflicts of interest
The authors declare no conflict of interest.
Acknowledgements
This work was supported by a research grant from the Indian Council of Medical Research (ICMR), New Delhi (Grant No. 52/13/2022-BIO/BMS). SP, SK, MMR, and SKM acknowledge the Institute Fellowship from BITS Pilani. The authors are grateful to the administration of BITS Pilani, Pilani campus, for providing necessary providing research support and computational infrastructure.
References
- V. W. S. Wong, L. A. Adams, V. de Lédinghen, G. L. H. Wong and S. Sookoian, Nat. Rev. Gastroenterol. Hepatol., 2018, 15, 461–478 CrossRef PubMed.
- Z. M. Younossi, J. Hepatol., 2019, 70, 531–544 CrossRef PubMed.
- S. Gawrieh, M. Noureddin, N. Loo, R. Mohseni, V. Awasty, K. Cusi, K. V Kowdley, M. Lai, E. Schiff, D. Parmar, P. Patel and N. Chalasani, Hepatology, 2021, 74, 1809–1824 CrossRef PubMed.
- M. R. Jain, S. R. Giri, B. Bhoi, C. Trivedi, A. Rath, R. Rathod, R. Ranvir, S. Kadam, H. Patel, P. Swain, S. S. Roy, N. Das, E. Karmakar, W. Wahli and P. R. Patel, Liver Int., 2018, 38, 1084–1094 CrossRef PubMed.
- C. Postic and J. Girard, J. Clin. Invest., 2008, 118, 829–838 CrossRef PubMed.
- S. M. Jeyakumar and A. Vajreswari, World J. Hepatol., 2022, 14, 168–179 CrossRef PubMed.
- P. Dobrzyn, A. Dobrzyn, M. Miyazaki, P. Cohen, E. Asilmaz, D. G. Hardie, J. M. Friedman and J. M. Ntambi, Proc. Natl. Acad. Sci. U. S. A., 2004, 101, 6409–6414 CrossRef CAS PubMed.
- T. Uebanso, Y. Taketani, M. Fukaya, K. Sato, Y. Takei, T. Sato, N. Sawada, K. Amo, N. Harada, H. Arai, H. Yamamoto and E. Takeda, Am. J. Physiol.: Endocrinol. Metab., 2009, 297, 76–84 CrossRef PubMed.
- D. Bhattacharya, B. Basta, J. M. Mato, A. Craig, D. Fernández-Ramos, F. Lopitz-Otsoa, D. Tsvirkun, L. Hayardeny, V. Chandar, R. E. Schwartz, A. Villanueva and S. L. Friedman, JHEP Reports, 2021, 3, 100237 CrossRef PubMed.
- H. Wang, M. G. Klein, H. Zou, W. Lane, G. Snell, I. Levin, K. Li and B.-C. Sang, Nat. Struct. Mol. Biol., 2015, 22, 581–585 CrossRef CAS.
- S. Release, 4: LigPrep, Schrödinger, LLC, New York, NY, 2019 Search PubMed.
- S. K. Mandal, S. Puri, B. K. Kumar, M. Muzaffar-Ur-Rehman, P. K. Sharma, M. Sankaranarayanan and P. R. Deepa, Mol. Diversity, 2023, 1–16 Search PubMed.
- D. E. Shaw, J. P. Grossman, J. A. Bank, B. Batson, J. A. Butts, J. C. Chao, M. M. Deneroff, R. O. Dror, A. Even and C. H. Fenton, in SC14: Proceedings of the International Conference for High Performance Computing, Networking, Storage and Analysis, IEEE, 2014, pp. 41–53 Search PubMed.
- W. L. Jorgensen, D. S. Maxwell and J. Tirado-Rives, J. Am. Chem. Soc., 1996, 118, 11225–11236 CrossRef CAS.
- E. Wang, H. Sun, J. Wang, Z. Wang, H. Liu, J. Z. H. Zhang and T. Hou, Chem. Rev., 2019, 119, 9478–9508 CrossRef CAS.
- A. Ali, G. J. Mir, A. Ayaz, I. Maqbool, S. B. Ahmad, S. Mushtaq, A. Khan, T. M. Mir and M. U. Rehman, J. Mol. Model., 2023, 29, 171 CrossRef CAS.
- D. E. V Pires, T. L. Blundell and D. B. Ascher, J. Med. Chem., 2015, 58, 4066–4072 CrossRef PubMed.
- M. P. Jacobson, R. A. Friesner, Z. Xiang and B. Honig, J. Mol. Biol., 2002, 320, 597–608 CrossRef CAS PubMed.
- S. J. Stuart, R. Zhou and B. J. Berne, J. Chem. Phys., 1996, 105, 1426–1436 CrossRef CAS.
- M. Ikeguchi, J. Comput. Chem., 2004, 25, 529–541 CrossRef CAS PubMed.
- S. Puri, S. K. Mandal, H. Jain, P. K. Sharma and P. R. Deepa, J. Food Drug Anal., 2024, 32, 174–189 CrossRef.
- E. Jenwitheesuk and R. Samudrala, Antivir. Ther., 2005, 10, 157–166 CrossRef CAS.
- P. R. Deepa, S. Vandhana, S. Muthukumaran, V. Umashankar, U. Jayanthi and S. Krishnakumar, J. Ocul. Biol. Dis. Infor., 2010, 3, 117–128 CrossRef CAS.
- H. Dursun, F. Albayrak, M. Bilici, F. Koc, H. H. Alp, T. Candar and O. Kukula, Yakugaku Zasshi, 2009, 129, 861–869 CrossRef CAS.
- F. Dilek, E. Ozkaya, A. Kocyigit, M. Yazici, S. Kesgin, A. H. Gedik and E. Cakir, Int. Arch. Allergy Immunol., 2015, 167, 119–126 CrossRef CAS.
- R. M. Mansour, M. A. E. Ahmed, A. E. El-Sahar and N. S. El Sayed, Toxicol. Appl. Pharmacol., 2018, 358, 76–85 CrossRef CAS PubMed.
- M. S. Abdallah, A. H. Eldeen, S. S. Tantawy and T. M. Mostafa, Eur. J. Pharmacol., 2021, 906, 174295 CrossRef CAS PubMed.
- A. M. Mohamadin, A. A. Elberry, M. A. Elkablawy, H. S. Abdel and F. A. Al-abbasi, Pathophysiology, 2011, 18, 235–242 CrossRef CAS PubMed.
- I. I. Hegab, H. E. El-Horany, M. M. Elbatsh and D. S. Helal, J. Biochem. Mol. Toxicol., 2019, 33, e22231 CrossRef PubMed.
|
This journal is © The Royal Society of Chemistry 2024 |
Click here to see how this site uses Cookies. View our privacy policy here.