DOI:
10.1039/D4RA06060A
(Paper)
RSC Adv., 2024,
14, 32304-32313
Migration of total petroleum hydrocarbon and heavy metal contaminants in the soil–groundwater interface of a petrochemical site using machine learning: impacts of convection and diffusion†
Received
21st August 2024
, Accepted 30th September 2024
First published on 14th October 2024
Abstract
Convection and diffusion are key pathways for the migration of total petroleum hydrocarbons (TPH) and heavy metals (HMs) from soil to groundwater. However, the extent of their influence on pollutant migration, as well as the nonlinear relationships between these processes and pollutants, remains unclear. This study investigates the spatial distribution of TPH and HMs at a petrochemical site with complex hydrogeological conditions in southwestern China. In addition, machine learning (ML) was used to assess the effects of convection and diffusion on pollutant migration at the soil–groundwater interface. The analysis identifies and reveals TPH, Co, and Ni as the primary pollutants, with soil concentrations reaching 47.427, 7.024, and 4.766 times their respective screening values. Among various ML models, Random Forest (RF) was identified as the most effective, based on R2, and RMSE performance metrics. The RF model demonstrates that the concentrations of TPH and As are closely related to soil depth. Furthermore, importance indices calculated by RF indicate that the significance of convection and diffusion varies across different soil–groundwater systems. Specifically, at the soil–perched water interface, convection plays a more significant role than diffusion in influencing the migration of TPH and As. However, at the soil–pore water interface, diffusion more significantly influences the migration of all pollutants compared to convection. Additionally, a threshold or saturation effect was observed for the impact of the convection factor on pollutant concentrations in groundwater. These findings highlight the distinct roles of convection and diffusion across various water interfaces, providing new insights into the mechanisms governing contaminant migration and fate.
1 Introduction
With the increasing production and consumption of petroleum and its derivatives, environmental pollution from crude oil is intensifying.1–3 The contamination of soil and groundwater by petroleum hydrocarbons has garnered global attention.4,5 Approximately 33% of oil storage tanks worldwide are estimated to leak annually, contaminating the surrounding soil,6,7 and introducing substantial quantities of total petroleum hydrocarbons (TPH), heavy metals (HMs), and other hazardous waste components into the soil and groundwater. These contaminants can lead to significant ecosystem degradation.8 Current research indicates that soil and water contamination around refineries can have profound adverse effects on human health, including increased risks of coronary heart disease,9 leukemia,10 and lung cancer.11
Upon entering the soil, petroleum hydrocarbons and HMs are subjected to various environmental influences, including soil composition and other factors, leading to heterogeneous distribution patterns.12,13 These contaminants are then affected by groundwater convection and diffusion, migrating from the soil into the groundwater14 and further impact pollutant transport. However, most previous studies have focused on the application of convection and diffusion in constructing predictive equations for pollutant migration,15–18 even though research has shown that convection and diffusion are key contributors to groundwater contamination.19 Therefore, studying the influence of convection and diffusion on pollutant migration at the soil–groundwater interface is crucial for predicting pollutant dispersion and understanding pollutant fate of contaminants.20 Unfortunately, this area of research remains underexplored. Additionally, the nonlinear relationships between pollutants,19 convection, and diffusion further complicate the investigation of their impact on pollutant migration. To determine whether convection or diffusion has a greater impact on pollutant migration, and to explore the relationships between these processes and pollutants, appropriate methods are needed to investigate the complex interactions involved.
In recent years, machine learning (ML) has been widely used in environmental field research,21–24 to discover hidden patterns and infer correlations.25,26 ML not only performs well on structured data but also demonstrates strong performance on semi-structured and unstructured data.27–29 The understanding of the migration of pollutants can be enhanced by using ML,30 such as finding the pollutants that have the closest relationship with environmental factors,31 helping to reveal relationships that may not be strongly linear. In addition, it can also be used to explore the nonlinear relationships between environmental factors and pollutants.32 The training-based ML can also be used to predict the migration of pollutants at the soil–groundwater interface.19 This highlights the great potential of machine learning in exploring the complex relationships between pollutant concentrations and environmental variables, including uncovering the intricate connections between convection, diffusion, and pollutant concentrations.
Oil refineries are recognized as the second-largest source of organic pollutants.33 This study delves into the spatial distribution and environmental migration of TPH and HMs at a typical contaminated refinery site characterized by complex hydrogeological conditions in southwestern China. Employing statistical methods alongside ML, the research aims to: (1) ascertain the spatial distribution of TPH, arsenic (As), cobalt (Co), nickel (Ni), and lead (Pb) utilizing the inverse distance weighting (IDW) method. (2) Develop a robust ML model using plot data to elucidate the correlation between TPH, As, Co, Ni, and Pb concentrations and soil depth. (3) Investigate the influence of convection and diffusion processes on the transport of these pollutants within the soil–groundwater interface.
2 Methods
2.1. Study area
The abandoned refinery, a representative example of industrial pollution in southwestern China, is located at 106°7′3′′E longitude and 30°50′38′′N latitude, covering approximately 913.71 acres (Fig. 1). With over 55 years of production history, it specialized in petroleum products such as light gasoline, liquefied petroleum gas, lubricating oils, petroleum waxes, petroleum fats, and asphalt before operations ceased in 2013. The site is situated in a region characterized by a humid monsoon climate within the mid-subtropical zone, with an average temperature of 17.1 °C and an annual precipitation of approximately 1100 mm. Field data show that the site's topography features higher elevations in the northwest and lower in the southeast, with typical soil strata composed of miscellaneous fill, pulverized clay, and rounded gravel, underlain by sandy mudstone bedrock. In our preliminary field investigation of the site, groundwater flow direction was determined to be from the northeast to the southwest based on groundwater level measurements from monitoring wells (Fig. S2†). Additionally, the results of the hydrogeological survey indicated that the site has complex soil and hydrological conditions, with abundant perched water and pore water. In the more than ten years since its abandonment, the distribution and migration of pollutants in the area have been significantly influenced by perched water and pore water.
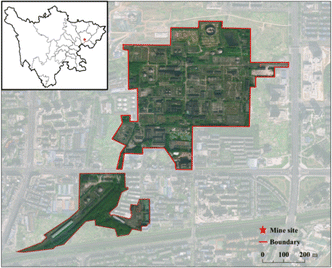 |
| Fig. 1 Study area. | |
2.2. Soil and groundwater sampling and analysis
Preliminary soil sampling indicates that areas such as the catalytic cracking and atmospheric decompression device zones are contaminated, with sampling conducted in a grid pattern not exceeding 20 m × 20 m. Conversely, regions without apparent contamination, such as the plant's open spaces and warehouses, follow a grid pattern not exceeding 40 m × 40 m. During the investigation, anomalies in soil color and odor were noted, prompting the addition of encrypted distribution points where necessary. The final sampling points are shown in Fig. S3.† SVOCs samples were collected from the original soil state, spanning depths of 0–13 m, and the 0–4 m range was subdivided into four layers: 0–0.5 m, 0.5–1.5 m, 1.5–2.5 m, and 2.5–4 m. These samples were stored in 500 mL wide-mouth glass bottles. HMs samples, initially collected as raw soil, were sealed with sealing tape and subsequently placed in an insulated box for preservation. In total, 2084 profile soil samples from 540 profiles were gathered. Groundwater sampling encompassed 115 testing points arranged in an 80 × 80 m grid, yielding 46 perched water samples and 69 diving samples. The final sampling points are shown in Fig. S4.†
2.3. Development of machine learning (ML)
ML was utilized to investigate pollutant transport within the soil–groundwater interface of an abandoned oil refinery. Eight distinct models—linear regression (LR), random forest (RF), K-nearest neighbor (KNN), artificial neural network (ANN), linear kernel support vector machine (SVM), decision tree (DTree), explanatory baseline model (EBM), and extreme gradient boosting (XGB)—were trained using the training dataset. The detailed descriptions, along with the advantages and disadvantages of the aforementioned machine learning models, are provided in Table S1.† The model development process includes the following steps:34,35 (1) using a combination of measured values of pollutant concentrations, sampling depths, geographic locations, and other relevant environmental factors as input data, (2) missing values were removed, followed by the application of a 3-standard deviation rule to filter out outliers. Afterward, the units of each variable were standardized, and the output variable was transformed into its natural logarithm (ln) form, (3) setting the ratio of training set to testing set in the input data to 9
:
1, (4) tuning the parameters of eight algorithms and identifying the optimal parameters for each algorithm, (5) setting up the commands for data quantification and comparing model performance. To reduce the risk of overfitting, 9-fold cross-validation was applied to the training dataset (Fig. S5†). The 9-fold cross-validation was also used to adjust the model parameters, and Bayesian optimization (for details see TEXT S1†) was employed to determine the optimal configuration of the models. The coefficient of determination (R2) (equn (1)) and root mean square error (RMSE) (eqn (2)) between the actual and predicted values were calculated to evaluate the prediction accuracy of each ML algorithm. The optimal algorithm was identified as the one with the highest R2 and the lowest RMSE on the validation dataset. The development and evaluation of the ML models were performed using Python. |
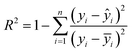 | (1) |
|
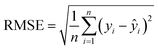 | (2) |
where n denotes the sample size, yi denotes the measured value of the ith sample, ŷi denotes the predicted value of the model for the sample point, and yi denotes the mean value. Additionally, Shapley Additive Explanations (SHAP) analysis (for details see TEXT S2†) was used to further interpret the model.
2.4. Convection and diffusion of soil pollutants
Convection and diffusion are the main modes of soil contaminant transport.36 To study the importance of convection and diffusion in the migration of pollutants, it is necessary to quantify these two processes. When pollutants are transported to groundwater via convection, the concentration of pollutants in groundwater can be approximated by the concentration of pollutants in the soil layers close to the groundwater (eqn (3)).19 Therefore, analyzing the relationship between pollutant concentrations in the corresponding soil layers and groundwater can help assess the impact of convection on pollutant migration at the soil–groundwater interface (eqn (4)).37 Analyzing the relationship between vertical gradients and groundwater concentrations can be used to assess the impact of diffusion on pollutant migration at the soil–groundwater interface. By using convection and diffusion as features in machine learning models for groundwater contamination analysis the relative impacts of convection and diffusion on groundwater contamination can be compared. Details regarding the convective and diffusive factor equations can be found in the ESI (TEXT S3).† |
 | (3) |
|
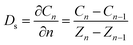 | (4) |
where Cs denotes the amount of contaminant transported from soil to groundwater; Ds represents the diffusion factor; C is the pollutant concentration; T is time. Cn, Cn−1 denote the contaminant concentration of samples n, n − 1; and Zn, Zn−1 denote the soil depth corresponding to samples n, n − 1.
2.5. Statistical analysis and data visualization
Descriptive statistics, including the maximum, minimum, and other key metrics for each pollutant, were analyzed using SPSS software (v.12). Vertical distribution maps were plotted using ArcGIS software (v.10.2). Data visualization, such as linear regression, was performed using Origin (v.2022). Data analysis and machine learning model fitting, including bootstrapped confidence intervals and various regression models, were performed using Python (v.3.11).
3 Results and discussion
3.1. TPH and HMs content in soil and groundwater at the refinery
A thorough quality assessment of soil and groundwater is essential to elucidate the mechanisms of contaminant transport and dispersion. The concentrations of TPH and HMs in 2084 soil samples and 115 groundwater samples are detailed in Tables 1 and S2.† TPH, As, Co, Ni, and Pb were prevalent in both soil and groundwater, with notably high concentrations at certain sampling sites. The peak concentrations of TPH, As, Co, Ni, and Pb in soil samples reached 39
715.032 mg kg−1, 95.890 mg kg−1, 280.953 mg kg−1, 714.864 mg kg−1, and 1204.567 mg kg−1, respectively, significantly surpassing the risk screening benchmarks. Notably, the maximum concentrations of TPH, Co, and Ni were 47.427, 7.024, and 4.766 times higher than the screening values, respectively. This trend is largely ascribed to petroleum refining activities, which generate substantial amounts of toxic metals, particularly Co and Ni. Leakages during the refining process can result in TPH, Co, and Ni seeping into the soil, thereby markedly elevating their cumulative concentrations. Furthermore, an analysis of contaminant means values across various depths revealed a progressive increase in TPH within the 0–2.5 m stratum, suggesting accumulation within the 0.5–2.5 m interval. This is consistent with existing research,30 indicating that TPH, being poorly soluble in water, tends to concentrate in intermediate horizons due to fluctuations in the water table. The mean concentration of all contaminants was significantly elevated in the 0–2.5 m horizon. The coefficient of variation (CV) for all pollutants exceeded 0.5 within this stratum. Considering the established correlation between CV values and the 0.5 threshold as an indicator of anthropogenic soil contamination,39,40 the data suggest that human activities have polluted the soil profile within the 0–2.5 m range.
Table 1 Contents of TPH and HMs in soil of abandoned refinery (mg kg−1) (n = 2084)
|
TPH |
As |
Co |
Ni |
Pb |
Risk intervention values (GB36600-2018);38 ND: not detected; SD: standard deviation; CV: coefficient of variation; OSR: over-standard rate. |
Min |
ND |
1.165 |
2.582 |
5.337 |
2.880 |
Max |
39 715.032 |
95.890 |
280.953 |
714.864 |
1204.567 |
Mean |
491.650 |
14.162 |
14.667 |
29.590 |
33.901 |
SD |
1838.586 |
5.991 |
8.302 |
23.766 |
43.227 |
CV |
3.740 |
0.423 |
0.566 |
0.803 |
1.275 |
OSR% |
9.945 |
0.492 |
0.492 |
0.492 |
0.219 |
Soil reference valuea |
826 |
40 |
40 |
150 |
400 |
In groundwater, TPH, As, Co, Ni and Pb concentrations ranged from 30–4140.0 μg L−1, 0.3–63.4 μg L−1, 0.153–2778.0 μg L−1, 0.710–2367.0 μg L−1, and 0.621–249.0 μg L−1, with mean values of contaminants in the perched water being greater than in pore water, especially for TPH, Co and Ni, which were 8.78, 5.39 and 3.41 times higher in the perched water than in the pore water. Comparison with groundwater standards revealed that the abandoned refinery was mainly contaminated with TPH (95.650% in perched water and 17.391% in pore water), followed by As (4.348% in perched water and 5.797% in pore water) and Pb (6.522% in perched water and 4.348% in pore water). The CVs of all contaminants were greater than 0.5 both in the perched water and in the pore water, thus human activities are the main influencing factor of the contaminants in the groundwater of this abandoned refinery.
3.2. Heterogeneous distribution of TPH and HMs in soil–groundwater
Since the IDW interpolation method is less affected by smoothing compared to Kriging and other methods, it can better reflect the actual pollution distribution at the contaminated site.41,42 Therefore, IDW was used to interpolate the spatial distribution of pollutants at the site. In the IDW spatial interpolation analysis of the study site, as illustrated in the Fig. 2, it is evident that the soil surface layer exhibits high concentrations of TPH contamination primarily concentrated in the production area and around the wastewater treatment facilities. Across each layer, substantial regions exceed the risk screening values, indicating that TPH can vertically migrate to depths exceeding 4 meters. As soil depth increases, the area of high concentration contamination diminishes and reveals a discrete ‘patchy’ distribution in the deeper soil layers. This distribution pattern primarily arises from the presence of multiple production zones within the site, where intermittent pollution sources contribute to discontinuous ‘hotspots’ of contamination at the soil surface. When TPH infiltrates the subsurface, groundwater disturbances further expand the range of TPH contamination.43 Notably, in the 1.5–2.5 m soil layer, certain regions exhibit unusually higher TPH concentrations compared to the 0.5–1.5 m soil layer. This phenomenon may be related to the clayey texture of the soil. Soil texture investigations at the study site revealed that the anomalous area at 1.5–2.5 meters depth consists of clay, characterized by low permeability and high adsorption capacity.44 TPH adsorption in the soil increases with higher clay content,45 and clay acts as a ‘barrier layer’ during vertical transport, leading to TPH enrichment. Apart from Pb, the elevated concentrations of the other three HMs are primarily found in the soil's surface layer. The distribution of HMs concentrations varies significantly between different soil layers, likely due to the heterogeneous distribution of primary minerals within the profile. These minerals gradually release into the surrounding environment during weathering, resulting in varying HMs contents across different layers.46,47
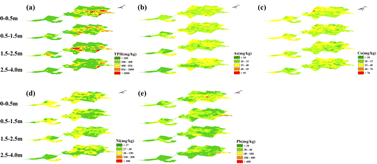 |
| Fig. 2 Vertical spatial distribution of TPH and HMs in the study area. Panels (a) through (e) correspond to TPH, As, Co, Ni, and Pb. The risk intervention values (GB36600-2018)38 for pollutants are as follows: TPH is set at 826 mg kg−1, As at 40 mg kg−1, Co at 40 mg kg−1, Ni at 150 mg kg−1, and Pb at 100 mg kg−1. The orange or red areas indicate that the pollutant levels in these regions exceed the standard values. | |
The distribution of various types of pollutants in groundwater is shown in Fig. S6,† which demonstrates that groundwater is widely polluted by TPH, with As, Co, and Ni concentrations higher in perched water than in pore water, and Pb concentrations higher in pore water than in perched water. A variety of pollutants showed significant spatial distribution differences. In the perched water, the high concentration areas of TPH and HMs were mainly located in the eastern part of the plot. The corresponding areas on the soil surface were also in high concentration areas, indicating that the soil surface was the main source of pollutants in the perched water. Overall, the pollutant concentration in the perched water showed a decreasing trend from the northeast to the southwest. In the pore water, the high value areas of TPH and As were biased to the southwest relative to the perched water, which was consistent with the flow direction of groundwater, but the high concentration areas of Co, Ni and Pb moved to the northwest. This phenomenon may be caused by soil disturbance. Historically, the north-western part of the abandoned refinery was used as a catalytic area, and the change in the functional area has resulted in multiple soil disturbances, which have led to the introduction of Co, Ni and Pb into the pore water.
3.3. Transport of TPH and HMs in soil–groundwater
3.3.1. Performance of different ML algorithms in interpreting soil data. Diverse ML models demonstrate varying efficacy in predicting soil contaminant concentrations. To thoroughly examine the characteristics of different pollutants, selecting a model with superior predictive performance is imperative. This study entailed a comprehensive comparison of eight distinct ML models, employing R2 and RMSE as the evaluative metrics. The optimal model hyperparameters obtained through Bayesian optimization are shown in Tables S4† and S3† presents the R2 and RMSE for all models. Fig. 3 clearly shows that, the RF model performs well in predicting various pollutants and demonstrates the highest stability. Upon holistic consideration of these metrics, the RF model distinguishes itself among the contenders due to its robust stability and precision. Previous research has also demonstrated the effectiveness of machine learning models in similar environmental contexts. For example, Guo et al. (2023)32 utilized XGBoost (XGB), which performed well in identifying key factors for vertical distribution of HMs in Pb/Zn smelters. Similarly, Huang et al. (2023)19 applied the Random Forest (RF) model to predict groundwater HMs concentrations in mining areas, which showed robust performance across various pollutants. In this study, RF was chosen as it exhibited strong predictive power for a variety of pollutants, including TPH, As, and Co, similar to the findings of previous research. The use of models like RF, which consistently performed well across pollutants, provides an efficient approach for investigating the nonlinear interactions between environmental factors and contaminant transport, while also ensuring model stability. Furthermore, the inherent interpretability of RF model48 facilitates a deeper understanding of the modeling process. Consequently, the RF model was chosen for subsequent exploration of pollutant infiltration dynamics at the study site.
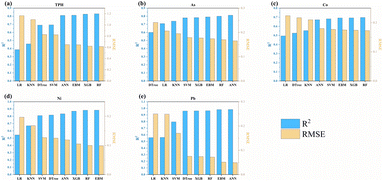 |
| Fig. 3 Performance comparison of different models for predicting pollutant levels using R2 and RMSE metrics. (a) TPH, (b) As, (c) Co, (d) Ni, (e) Pb. | |
3.3.2. Correlation of soil TPH and HMs with soil depth. Contaminants in soil typically exhibit a non-homogeneous distribution, and the RF model is adept at predicting the transport of various contaminants within soil matrices. Using the RF model, the correlation between six pollutants and soil profile depth was calculated. Analysis of significance indices between pollutants and depth revealed that TPH and As were most closely associated with depth (Fig. S7†). The Fig. 4 presents the outcomes of linear regression analyses between measured pollutant concentrations and depth. The study observed that the concentrations of TPH and HMs decrease with soil depth, which is consistent with the principle of depth attenuation. Notably, larger intercepts and steeper fitting curves for TPH and Pb suggest that these pollutants are more prevalent, exhibit greater vertical variability, and possess limited transportability in the soil surface layer. Previous research indicates that only a minor fraction of TPH migrates to the subsurface due to surface runoff, leaching, and hydrothermal heating of the soil.49 TPH partially dissolves in water, adsorbs onto solid organic particles, and forms soil gases and non-aqueous phase liquids.50 Owing to its hydrophobic nature, TPH readily adheres to organic matter within the soil.51 Pb predominantly exists as Pb(OH)2, PbCO3, PbSO4, and other solid forms in the soil, exhibiting minimal mobility.52 Consequently, TPH and Pb tend to accumulate in the upper soil layers and adhere during downward migration, resulting in significant vertical variability. The fitting curves for As, Co, and Ni content in soil versus depth are comparatively smooth, indicating minor vertical changes and robust migratory capabilities for these elements, corroborating findings from existing studies.19,30 Additionally, Fig. S8† presents the results using bootstrapped confidence intervals. It is evident that the concentrations of TPH and Pb exhibit greater variation with depth compared to the other pollutants, while the concentrations of As, Co, and Ni show less variation with depth. This is consistent with the analysis results from the linear regression.
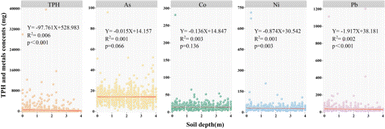 |
| Fig. 4 Fitted curves based on linear regression of TPH and HMs with soil depth. | |
3.3.3. Interpretability analysis of TPH correlation with key features. Taking TPH (the most severe contaminant at the site) as an example, RF feature importance indices and SHAP analysis were utilized for model interpretability analysis. Fig. 5(a) shows the RF feature importance indices for predicting TPH concentration in perched water. These indices help identify the most significant variables contributing to the model's predictions. The results indicate that chloride and total dissolved solids (TDS) are key variables influencing TPH migration. Notably, C(TPH) and D(TPH), representing the convection and diffusion processes for TPH, highlight the crucial role of both convection and diffusion under different environmental conditions. Fig. 5(b) presents the SHAP values, offering a more detailed explanation of the predictions in perched water. SHAP values decompose each sample's prediction, revealing that chloride and TDS are the primary variables affecting TPH convection, while D(TPH) emphasizes the importance of diffusion in TPH migration. At pore water, Fig. 5(c) shows that sulfate and turbidity are the key variables a ffecting TPH concentration, with C(TPH) and D(TPH) continuing to play significant roles in TPH transport. Fig. 5 (d) further confirms through SHAP analysis that sulfate is a crucial factor in regulating TPH diffusion in pore water. In general, a high concentration of total dissolved solids may indicate that the water body has a high degree of mineralization, which can affect the density and viscosity of the water,53 altering the partitioning of TPH to the aqueous phase54 and thereby impacting the movement and distribution of TPH in groundwater. Additionally, the activity of microorganisms can be affected by chlorides, fluoride, and dissolved solids,55 which can adversely affect TPH degradation.56,57 Additionally, a variety of solutes commonly found in groundwater systems, including various ions in TDS, may compete with TPH molecules for adsorption sites,58,59 potentially leading to increased mobility of TPH.
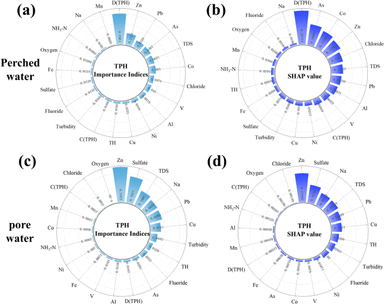 |
| Fig. 5 Factors influencing TPH concentration in perched water (a) based on importance indices and (b) based on SHAP analysis; and in pore water (c) based on importance indices and (d) based on SHAP analysis from the RF model. TDS: total dissolved solids; TH: total hardness; D(TPH): diffusion of TPH; C(TPH): convection of TPH. | |
3.3.4. The impact of convection and diffusion on pollutant migration in soil–groundwater. The roles of convection and diffusion in the transport of pollutants between soil and groundwater were compared using RF importance indices and SHAP (Fig. 6). At the soil–perched water interface, the diffusion importance indices were 0.298 and 0.014 for TPH and As, while the convection importance indices were 0.017 and 0.01, respectively. Clearly, the diffusion importance indices were higher than the convection importance indices, indicating that the migration of TPH and As was more influenced by diffusion. Conversely, the convection importance indices for Co, Ni, and Pb were 0.088, 0.095, and 0.004, while the diffusion importance indices were 0.029, 0.029, and 0.003. This suggests that the migration of Co, Ni, and Pb in perched water was more dependent on convective processes. Specifically, TPH showed the highest correlation with diffusion, whereas Ni was most closely related to convection. At the soil–pore water interface, the diffusion importance indices for TPH, As, Co, Ni, and Pb were 0.005, 0.044, 0.045, 0.375, and 0.052, respectively, and the convection importance indices were 0.002, 0.007, 0.03, 0.183, and 0.03, respectively. At this interface, diffusion generally played a more significant role than convection, indicating that the migration of TPH and HMs is more influenced by diffusion. Due to its hydrophobic characteristics,51 TPH has low solubility in water, and many small molecular components of TPH may diffuse rapidly in groundwater. As exists in water primarily in ionic form, which facilitates its entry into perched water through diffusion. HMs such as Co, Ni, and Pb may exist as larger particles or in combination with other large molecules or particles at the soil–perched water interface, making them more reliant on groundwater flow for transport. Geological investigations have shown that the soil texture in the soil–perched water interface is predominantly miscellaneous fill, whereas in the soil–pore water interface, it is predominantly clay. Clay soils, due to their strong HMs adsorption capacity,60 diminish the impact of convection on pollutant transport at the soil–pore water interface. Additionally, according to the derivation of eqn (4), when the external source of pollution disappears, pollutants gradually migrate downward over time, resulting in a decrease in the vertical gradient difference. In the shallow soil layer, the downward migration of HMs reduces the vertical concentration difference, thereby weakening the role of diffusion at the soil–perched water interface. Furthermore, the relative strength of convection and diffusion may be a significant factor in the difference in Co and Ni concentrations between perched and pore water. At the soil–pore water interface, increased diffusion makes it difficult for larger particles of HMs like Co and Ni to migrate from the soil to the pore water, resulting in lower concentrations in the pore water compared to the perched water. Simultaneously, Pb, which has the largest carbonate fraction, preempts the adsorption sites of Co and Ni, which have the highest exchange states,61 causing a large difference between the concentrations of Co and Ni in pore water and perched water, while the difference in Pb concentrations is smaller. In summary, the transport mechanisms of pollutants in the perched and pore water environments are influenced by their physicochemical properties and soil texture. TPH and As are mainly transported by diffusion in the perched water, whereas Co, Ni, and Pb are more dependent on convection. In pore water, diffusion has a greater influence on contaminant transport than convection, and the difference between convection and diffusion is a crucial factor in the differing concentrations of Co and Ni between the two groundwater types.
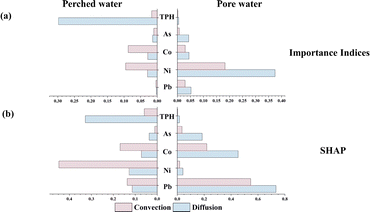 |
| Fig. 6 Correlation between convection and diffusion factors and the concentrations of TPH and HMs in groundwater, (a) based on the importance indices from the RF model; (b) based on the SHAP. | |
Fig. S13 and S14† show the dependence of pollutant concentrations on convection and diffusion factors in the RF model. From the figures (Fig. S13(a)–(e) and S14(a)–(e)†), it can be seen that the pollutant concentrations increase with the convection factor, whether in perched water or pore water. This is mainly because the higher the concentration of pollutants in the soil, the greater the number of pollutants that can enter the groundwater. Additionally, a threshold or saturation effect exists between some pollutants and convection, where pollutant concentrations change rapidly or slow down after the convection factor exceeds a certain value. For example, in perched water, Pb (Fig. S13(e)†) shows rapid changes when the convection exceeds 85, but the change slows down when it exceeds 89. This is mainly because when the pollutant concentration in the soil is low, the soil particles adsorb the pollutants,50 and the amount of pollutants carried by groundwater convection is limited. However, when the pollutant concentration in the soil reaches a certain level, the soil particles cannot fully adsorb the pollutants, and under groundwater convection, the pollutants rapidly dissolve into the groundwater. When the pollutant concentration in the soil becomes too high, the concentration of pollutants that can dissolve into the groundwater reaches saturation, and the concentration entering the groundwater through convection levels off. In the dependence plots of the diffusion factor (Fig. S13(f)–(j) and S14(f)–(j)),† it is observed that the concentration of pollutants in groundwater generally exhibits a U-shape centered around zero with respect to the diffusion factor, indicating that the larger the gradient difference, the higher the concentration of pollutants entering the groundwater.
We conducted linear regression analyses of convective factors, diffusive factors, and groundwater contaminant concentrations, and the results (Table S5†) of the study revealed some striking phenomena. The analyses showed that in the soil–perched water interface, only the TPH concentration had a significant linear relationship with the convection and diffusion factors, and the diffusion factor had a greater effect on the TPH concentration than the convection factor, suggesting that TPH enters the perched water mainly through diffusion, although convection also plays a certain role, a finding which is consistent with that of the RF analysis. However, for the other HMs pollutants, the same linear relationship was not observed in the perched water, and similarly, the concentration of TPH in the pore water did not show a significant linear relationship with the convection and diffusion factors, which is not in accordance with the expectation of Fick's law.62 We hypothesize that the reason for this discrepancy may be due to the fact that HMs may be adsorbed by the soil during their entry into the soil–groundwater interface63 or complexes formed with TPH are adsorbed on solid particles.64 At the same time, the light non-aqueous phase liquid (LNAPL) forms a ‘barrier’ between the perched water and the soil, resulting in smaller amounts of HMs entering the perched water by convection and diffusion compared to TPH. Therefore, in the perched water, we only observed significant linear relationships between TPH and convection and diffusion factors. In the soil–pore water interface, data Table S2† shows that there is no significant gradient trend in the concentration of different pollutants in the vertical direction. In addition, the geological conditions of the study area showed heterogeneity, resulting in inconsistent concentrations of pollutants released into pore water from soils at different levels and locations, which resulted in the lack of a significant linear relationship between pollutant concentrations in pore water and changes in vertical gradients in soil. This may imply the presence of a weak linear or other non-linear relationship. Because the RF model is able to capture these weak linear and non-linear relationships, it has shown effectiveness in exploring the mechanisms by which contaminant concentrations in the soil–groundwater interface are influenced by convection and diffusion.
3.3.5. Targeted remediation strategies based on pollutant migration mechanisms. Building on the observed threshold effect and the distinct roles of convection and diffusion in pollutant transport, this study underscores the need for tailored remediation approaches. For contaminants such as TPH and Pb, which exhibit more pronounced depth-dependent migration patterns, it is essential to consider strategies that account for their tendency to enter groundwater through diffusion, especially when diffusion factors exceed a critical threshold. For example, in perched water layers, In Situ Chemical Oxidation (ISCO) could be employed to target TPH hotspots by injecting oxidants to degrade pollutants directly in the affected zones,65 reducing both soil and groundwater contamination. In contrast, metals like Co and Ni, whose transport is more heavily influenced by convection, require remediation techniques that focus on controlling groundwater flow. Permeable Reactive Barriers (PRBs) could be strategically installed to intercept convective transport pathways, utilizing reactive materials such as zero-valent iron to immobilize metals before they reach critical concentrations in the groundwater. Moreover, since convection plays a lesser role at the soil–pore water interface, soil vapor extraction or phytoremediation could be considered as supplementary measures to manage pollutants like As, which primarily diffuse through soil layers.The observed threshold effect between convection and groundwater concentrations also highlights the importance of timely intervention. When convection factors exceed a certain value, pollutants enter groundwater at an accelerated rate, suggesting that remediation efforts should be implemented before this threshold is reached. Monitoring systems based on the identified thresholds can provide early warning signals, enabling proactive remediation before contaminants surpass acceptable limits.
4 Conclusions
This study investigated the spatial distribution and transport characteristics of TPH and HMs at a contaminated oil refinery site characterized by complex hydrogeological conditions, utilizing ML to analyze the roles of convection and diffusion in contaminant migration at the soil–groundwater interface. Key findings include the following: (1) TPH, Co, and Ni were identified as the primary contaminants. TPH pollution was detected throughout all strata within the 0–4 m soil depth, while HMs were primarily concentrated in the surface soil layer. Groundwater contamination was mainly caused by TPH, As, and Pb, originating predominantly from the surface soil. (2) The concentrations of all pollutants decreased with depth, consistent with the principle of depth attenuation. Notably, TPH and Pb exhibited larger intercepts and steeper fitted curves, indicating limited migration capabilities. (3) The relative effects of convection and diffusion differ between perched water and pore water. At the soil–perched water interface, diffusion had a more significant impact on the migration of TPH and As. At the soil–pore water interface, the influence of diffusion exceeds that of convection. The main cause of this result may be the differences in the soil particle properties of the site and the physicochemical properties of the pollutants. (4) A threshold effect and saturation effect were observed between convection and groundwater pollutant concentrations. (5) TPH primarily entered the groundwater system through processes occurring in perched water, whereas other HMs did not exhibit similar linear relationships, likely due to significant variations in vertical concentration gradients and nonlinear relationships between contaminants and hydrodynamic factors. However, discrepancies may occur because HMs can be adsorbed by the soil during their entry into the soil–groundwater interface, or complexes formed with TPH may adhere to solid particles. These factors could influence the transport mechanisms, and future research should focus on clarifying these processes to better understand their effects.
Data availability
The data supporting this article have been included as part of the ESI.†
Author contributions
All authors contributed to the study conception and design. The experiment was carried out by Yingdong WU, Zixin Zeng, Zhi Huang, Jie Yu. Material preparation, data collection and analysis were performed by Yingdong WU, Jiang Yu, Siwei Deng, Yinying Jiang. The first draft of the manuscript was written by Yingdong WU. All authors commented on previous versions of the manuscript. All authors read and approved the final manuscript.
Conflicts of interest
There are no conflicts to declare.
Acknowledgements
The work was supported by the National Key Research and Development Program (No. 2018YFC1802605); the Sichuan Provincial Major Science and Technology Project (No. 2022YFQ0081); the Science and Technology Program of Department of Ecology and Environment of Sichuan Province (No. 2023HB02); the Science and Technology Innovation Project of the Sichuan Academy of Eco-Environmental Sciences (No. ZX-2023106).
Notes and references
- N. A. S. Hussain and J. L. Stafford, Abiotic and biotic constituents of oil sands process-affected waters, J. Environ. Sci., 2023, 127, 169–186 CrossRef.
- S. J. Varjani, Microbial degradation of petroleum hydrocarbons, Bioresour. Technol., 2017, 223, 277–286 CrossRef PubMed.
- Z. Wei, Y. Wei, Y. Liu, S. Niu, Y. Xu, J.-H. Park and J. J. Wang, Biochar-based materials as remediation strategy in petroleum hydrocarbon-contaminated soil and water: Performances, mechanisms, and environmental impact, J. Environ. Sci., 2024, 138, 350–372 CrossRef PubMed.
- M. A. I. Khan, B. Biswas, E. Smith, R. Naidu and M. Megharaj, Toxicity assessment of fresh and weathered petroleum hydrocarbons in contaminated soil- a review, Chemosphere, 2018, 212, 755–767 CrossRef.
- Y. Wang, Y. Huang, P. Xi, X. Qiao, J. Chen and X. Cai, Interrelated effects of soils and compounds on persulfate oxidation of petroleum hydrocarbons in soils, J. Hazard. Mater., 2021, 408, 124845 CrossRef.
- C. M. Kao and J. Prosser, Evaluation of natural attenuation rate at a gasoline spill site, J. Hazard. Mater., 2001, 82, 275–289 CrossRef PubMed.
- H. Liu, M. Wu, H. Gao, N. Yi and X. Duan, Hydrocarbon transformation pathways and soil organic carbon stability in the biostimulation of oil-contaminated soil: Implications of 13C natural abundance, Sci. Total Environ., 2021, 788, 147580 CrossRef PubMed.
- K. Sivagami, D. Anand, G. Divyapriya and I. Nambi, Treatment of petroleum oil spill sludge using the combined ultrasound and Fenton oxidation process, Ultrason. Sonochem., 2019, 51, 340–349 CrossRef.
- H. Kim, N. Festa, K. Burrows, D. C. Kim, T. M. Gill and M. L. Bell, Is residential exposure to oil refineries a novel contextual risk factor for coronary heart disease?, Environ. Res., 2024, 244, 117965 CrossRef PubMed.
- D.-H. Koh, T.-W. Kim, Y.-H. Yoon, K.-S. Shin and S.-W. Yoo, Lymphohematopoietic Cancer Mortality and Morbidity of Workers in a Refinery/Petrochemical Complex in Korea, Saf. Health Work, 2011, 2, 26–33 CrossRef PubMed.
- C.-Y. Yang, B.-H. Cheng, T.-Y. Hsu, S.-S. Tsai, C.-F. Hung and T.-N. Wu, Female Lung Cancer Mortality and Sex Ratios at Birth near a Petroleum Refinery Plant, Environ. Res., 2000, 83, 33–40 CrossRef CAS PubMed.
- Y. Teng, D. Feng, L. Song, J. Wang and J. Li, Total petroleum hydrocarbon distribution in soils and groundwater in Songyuan oilfield, Northeast China, Environ. Monit. Assess., 2013, 185, 9559–9569 CrossRef PubMed.
- Q. Zhao, P. Li, F. Stagnitti, J. Ye, D. Dong, Y. Zhang and P. Li, Effects of aging and freeze-thawing on extractability of pyrene in soil, Chemosphere, 2009, 76, 447–452 CrossRef PubMed.
- S. A. Hoang, B. Sarkar, B. Seshadri, D. Lamb, H. Wijesekara, M. Vithanage, C. Liyanage, P. A. Kolivabandara, J. Rinklebe, S. S. Lam, A. Vinu, H. Wang, M. B. Kirkham and N. S. Bolan, Mitigation of petroleum-hydrocarbon-contaminated hazardous soils using organic amendments: A review, J. Hazard. Mater., 2021, 416, 125702 CrossRef PubMed.
- S. Harendra and C. Vipulanandan, Sorption and transport studies of cetyl trimethylammonium bromide (CTAB) and Triton X-100 in clayey soil, J. Environ. Sci., 2013, 25, 576–584 CrossRef PubMed.
- D. Li, F. Li and B. Xu, Multi-relaxation-time lattice Boltzmann method for anisotropic convection-diffusion equation with divergence-free velocity field, Comput. Appl. Math., 2024, 171, 1–5 CrossRef.
- A. Schneider, Z. Lin, T. Sterckeman and C. Nguyen, Comparison between numeric and approximate analytic solutions for the prediction of soil
metal uptake by roots. Example of cadmium, Sci. Total Environ., 2018, 619, 1194–1205 CrossRef PubMed.
- H. Xie, Y. Chen, H. Ke, X. Tang and R. Chen, Analysis of diffusion-adsorption equivalency of landfill liner systems for organic contaminants, J. Environ. Sci., 2009, 21, 552–560 CrossRef CAS PubMed.
- C. Huang, Z. Guo, T. Li, R. Xu, C. Peng, Z. Gao and L. Zhong, Source identification and migration fate of metal(loid)s in soil and groundwater from an abandoned Pb/Zn mine, Sci. Total Environ., 2023, 895, 165037 CrossRef CAS PubMed.
- X. Zheng, C. W. Lee and D. L. Phillips, Resonance Raman observation of the allyl cation produced after ultraviolet photodissociation of cyclopropyl bromide in acetonitrile solution, Chem. Phys. Lett., 2002, 366, 656–663 CrossRef CAS.
- Z. Huang, J. Yu, X. Shao, Y. Jiang, J. Yu, S. Deng and P. Li, Interpretable artificial intelligence for advanced oxidation systems: Principle, operations and performance, Process Saf. Environ. Prot., 2023, 180, 242–259 CrossRef CAS.
- Y. Sun, Y. Zhang, L. Lu, Y. Wu, Y. Zhang, M. A. Kamran and B. Chen, The application of machine learning methods for prediction of metal immobilization remediation by biochar amendment in soil, Sci. Total Environ., 2022, 829, 154668 CrossRef CAS.
- T. Shi, X. Hu, L. Guo, F. Su, W. Tu, Z. Hu, H. Liu, C. Yang, J. Wang, J. Zhang and G. Wu, Digital mapping of zinc in urban topsoil using multisource geospatial data and random forest, Sci. Total Environ., 2021, 792, 148455 CrossRef CAS PubMed.
- Y. Sun, S. Chen, H. Jiang, B. Qin, D. Li, K. Jia and C. Wang, Towards interpretable machine learning for observational quantification of soil heavy metal concentrations under environmental constraints, Sci. Total Environ., 2024, 926, 171931 CrossRef CAS.
- K. N. Palansooriya, J. Li, P. D. Dissanayake, M. Suvarna, L. Li, X. Yuan, B. Sarkar, D. C. W. Tsang, J. Rinklebe, X. Wang and Y. S. Ok, Prediction of Soil Heavy Metal Immobilization by Biochar Using Machine Learning, Environ. Sci. Technol., 2022, 56, 4187–4198 CrossRef PubMed.
- S. Zhong, K. Zhang, M. Bagheri, J. G. Burken, A. Gu, B. Li, X. Ma, B. L. Marrone, Z. J. Ren, J. Schrier, W. Shi, H. Tan, T. Wang, X. Wang, B. M. Wong, X. Xiao, X. Yu, J.-J. Zhu and H. Zhang, Machine Learning: New Ideas and Tools in Environmental Science and Engineering, Environ. Sci. Technol., 2021, 55, 12741–12754 Search PubMed.
- J. Xia, Q. Shi, H. Li, M. Zhou, K. Jiang and K. Wang, A novel sorting method for liquid metal batteries based on deep learning and sequential features, J. Energy Storage, 2023, 64, 107093 CrossRef.
- É. D. C. Paes, G. V. Veloso, A. A. D. Fonseca, E. I. Fernandes-Filho, M. P. F. Fontes and E. M. B. Soares, Predictive modeling of contents of potentially toxic elements using morphometric data, proximal sensing, and chemical and physical properties of soils under mining influence, Sci. Total Environ., 2022, 817, 152972 CrossRef PubMed.
- Z. Huang, J. Yu, W. He, J. Yu, S. Deng, C. Yang, W. Zhu and X. Shao, AI-enhanced chemical paradigm: From molecular graphs to accurate prediction and mechanism, J. Hazard. Mater., 2024, 465, 133355 CrossRef PubMed.
- Y. Zhang, Q. Zhang, W. Chen, W. Shi, Y. Cui, L. Chen and J. Shao, Source apportionment and migration characteristics of heavy metal(loid)s in soil and groundwater of contaminated site, Environ. Pollut., 2023, 338, 122584 CrossRef PubMed.
- S. Liu, X. Yang, B. Shi, Z. Liu, X. Yan, Y. Zhou and T. Liang, Utilizing machine learning algorithm for finely three-dimensional delineation of soil-groundwater contamination in a typical industrial park, North China: Importance of multisource auxiliary data, Sci. Total Environ., 2024, 911, 168598 CrossRef CAS PubMed.
- Z. Guo, Y. Zhang, R. Xu, H. Xie, X. Xiao and C. Peng, Contamination vertical distribution and key factors identification of metal(loid)s in site soil from an abandoned Pb/Zn smelter using machine learning, Sci. Total Environ., 2023, 856, 159264 CrossRef CAS PubMed.
- H. Rajabi, M. H. Mosleh, P. Mandal, A. Lea-Langton and M. Sedighi, Emissions of volatile organic compounds from crude oil processing – Global emission inventory and environmental release, Sci. Total Environ., 2020, 727, 138654 CrossRef CAS PubMed.
- E. Abbasi, M. R. A. Moghaddam and E. Kowsari, A systematic and critical review on development of machine learning based-ensemble models for prediction of adsorption process efficiency, J. Cleaner Prod., 2022, 379, 134588 CrossRef.
- J. Luo, X. Ma, Y. Ji, X. Li, Z. Song and W. Lu, Review of machine learning-based surrogate models of groundwater contaminant modeling, Environ. Res., 2023, 238, 117268 CrossRef CAS PubMed.
- X. Shu, Y. Wu, X. Zhang and F. Yu, Experiments and models for contaminant transport in unsaturated and saturated porous media – A review, Chem. Eng. Res. Des., 2023, 192, 606–621 CrossRef CAS.
- R. Schulin, F. Curchod, M. Mondeshka, A. Daskalova and A. Keller, Heavy metal contamination along a soil transect in the vicinity of the iron smelter of Kremikovtzi (Bulgaria), Geoderma, 2007, 140, 52–61 CrossRef CAS.
- P.R.C. Ministry of Ecology and Environment Soil Environmental Quality Risk Control Standard for Soil Contamination of Development Land, China Beijing, 2018.
- Y. Han, A. Offenhausser and S. Ingebrandt, Detection of DNA hybridization by a field-effect transistor with covalently attached catcher molecules, Surf. Interface Anal., 2006, 38, 176–181 CrossRef CAS.
- Y. Wang, X. Duan and L. Wang, Spatial distribution and source analysis of heavy metals in soils influenced by industrial enterprise distribution: Case study in Jiangsu Province, Sci. Total Environ., 2020, 710, 134953 CrossRef CAS.
- G. Y. Lu and D. W. Wong, An adaptive inverse-distance weighting spatial interpolation technique, Comput. Geosci., 2008, 34, 1044–1055 CrossRef.
- A. P. Sergeev, A. G. Buevich, E. M. Baglaeva and A. V. Shichkin, Combining spatial autocorrelation with machine learning increases prediction accuracy of soil heavy metals, Catena, 2019, 174, 425–435 CrossRef.
- S. An, S. H. Kim, H. Woo, J. W. Choi, S.-T. Yun, J. Chung and S. Lee, Groundwater-level fluctuation effects on petroleum hydrocarbons in vadose zones and their potential risks: Laboratory studies, J. Hazard. Mater., 2024, 463, 132837 CrossRef PubMed.
- L. Zhao, J. Bian, Y. Zhang, L. Zhu and Z. Liu, Comparison of the sorption behaviors and mechanisms of perfluorosulfonates and perfluorocarboxylic acids on three kinds of clay minerals, Chemosphere, 2014, 114, 51–58 CrossRef PubMed.
- M. A. Providenti, H. Lee and J. T. Trevors, Selected factors limiting the microbial degradation of recalcitrant compounds, J. Ind. Microbiol., 1993, 12, 379–395 CrossRef.
- S. Huang, Z. Li, J. Yu, J. Feng, H. Hou and R. Chi, Vertical distribution and occurrence state of the residual leaching agent (ammonium sulfate) in the weathered crust elution-deposited rare earth ore, J. Environ. Manage., 2021, 299, 113642 CrossRef.
- J. Zeng, C. B. Tabelin, W. Gao, L. Tang, X. Luo, W. Ke, J. Jiang and S. Xue, Heterogeneous distributions of heavy metals in the soil-groundwater system empowers the knowledge of the pollution migration at a smelting site, Chem. Eng. J., 2023, 454, 140307 CrossRef CAS.
- C. Wang, J. Liu, C. Qiu, X. Su, N. Ma, J. Li, S. Wang and S. Qu, Identifying the drivers of chlorophyll-a dynamics in a landscape lake recharged by reclaimed water using interpretable machine learning, Sci. Total Environ., 2024, 906, 167483 CrossRef CAS.
- L. Yuan, Y. Wu, Q. Fan, P. Li, J. Liang, Z. Wang, R. Li and L. Shi, Spatial distribution, composition, and source analysis of petroleum pollutants in soil from the Changqing Oilfield, Northwest China, Mar. Pollut. Bull., 2022, 185, 114338 CrossRef.
- I. C. Ossai, A. Ahmed, A. Hassan and F. S. Hamid, Remediation of soil and water contaminated with petroleum hydrocarbon: A review, Environ. Technol. Innovation, 2020, 17, 100526 CrossRef.
- S. Xu, J. Zhan, L. Li, Y. Zhu, J. Liu and X. Guo, Total petroleum hydrocarbons and influencing factors in co-composting of rural sewage sludge and organic solid wastes, Environ. Pollut., 2023, 319, 120911 CrossRef PubMed.
- Y. Luo, Z. Wang, Y.-D. Zhang, J.-Q. Zhang, Q.-P. Zeng, Z.-L. Zhang, D. Tian, C. Li, C.-L. Peng, K. Ye, Y.-M. Chen, F.-Y. Huang, Y.-P. Wang, X.-Y. Ma and L. Chen, Vertical migration behavior simulation and prediction of Pb and Cd in co-contaminated soil around Pb-Zn smelting slag site, J. Hazard. Mater., 2024, 469, 133990 CrossRef PubMed.
- F. M. Sani and S. Nesic, A critical review of models for density, viscosity, and diffusivity in aqueous sodium chloride solutions, Electrochim. Acta, 2024, 477, 143766 CrossRef.
- M. Wang, C. Wang and Y. Li, Petroleum hydrocarbons in a water-sediment system from Yellow River estuary and adjacent coastal area, China: Distribution pattern, risk assessment and sources, Mar. Pollut. Bull., 2017, 122, 139–148 CrossRef PubMed.
- V. Ochoa-Herrera, Q. Banihani, G. Leon, C. Khatri, J. A. Field and R. Sierra-Alvarez, Toxicity of fluoride to microorganisms in biological wastewater treatment systems, Water Res., 2009, 43, 3177–3186 CrossRef CAS PubMed.
- M. T. B. Abena, T. Li, M. N. Shah and W. Zhong, Biodegradation of total petroleum hydrocarbons (TPH) in highly contaminated soils by natural attenuation and bioaugmentation, Chemosphere, 2019, 234, 864–874 CrossRef PubMed.
- L. Rong, X. Zheng, B. T. Oba, C. Shen, X. Wang, H. Wang, Q. Luo and L. Sun, Activating soil microbial community using bacillus and rhamnolipid to remediate TPH contaminated soil, Chemosphere, 2021, 275, 130062 CrossRef CAS.
- J. Lei, Y. Han, C. Zhao, S. Zhang, F. Han, Z. Li, J. Hao and W. Zhou, Activation behavior of Cu0/FeS/N-graphene derived from waste soybean residue for peroxymonosulfate: Performance and mechanism, Sep. Purif. Technol., 2023, 324, 124591 CrossRef CAS.
- X. Xiao, X. He, C. Ji, L. Li, M. Zhou, X. Yin, Y. Shan, M. Wang and Y. Zhao, Activation of persulfate by g-C3N4/nZVI@SBC for degradation of total petroleum hydrocarbon in groundwater, J. Environ. Manage., 2024, 356, 120612 CrossRef.
- R. Novikau and G. Lujaniene, Adsorption behaviour of pollutants: Heavy metals, radionuclides, organic pollutants, on clays and their minerals (raw, modified and treated): A review, J. Environ. Manage., 2022, 309, 114685 CrossRef PubMed.
- X. Liu, H. Guo, X. Zhang, S. Zhang, X. Cao, Z. Lou, W. Zhang and Z. Chen, Modeling the transport behavior of Pb(II), Ni(II) and Cd(II) in the complex heavy metal pollution site under the influence of coexisting ions, Process Saf. Environ. Prot., 2022, 162, 211–218 CrossRef.
- S. Keesstra, V. Geissen, K. Mosse, S. Piiranen, E. Scudiero, M. Leistra and L. van Schaik, Soil as a filter for groundwater quality, Curr. Opin. Environ. Sustain., 2012, 4, 507–516 CrossRef.
- T. Yang, Y. Liao, M. Wang, Z. Zheng and M. Yao, Remediation and resource utilization of petroleum-contaminated soil by pyrite-assisted pyrolysis as bifunctional materials to adsorb heavy metal and activate peroxymonosulfate oxidation, Sci. Total Environ., 2023, 892, 164742 CrossRef PubMed.
- C. E. Choong, K. T. Wong, S. Y. Yoon, H. Kim, M. Shin, Y.-Y. Chang, J.-K. Yang, S.-H. Kim, B.-H. Jeon, Y. Yoon and M. Jang, A facile acid induced water-based solvent by improving hydrophobicity for simultaneous remediating total petroleum hydrocarbon, heavy metals and benzo(a) pyrene contaminated soil: Laboratory- and pilot-scale studies, J. Cleaner Prod., 2021, 278, 123425 CrossRef.
- Z. Han, X. Kang, K. Singha, J. Wu and X. Shi, Real-time monitoring of in situ chemical oxidation (ISCO) of dissolved TCE by integrating electrical resistivity tomography and reactive transport modeling, Water Res., 2024, 252, 121195 CrossRef CAS.
|
This journal is © The Royal Society of Chemistry 2024 |
Click here to see how this site uses Cookies. View our privacy policy here.