DOI:
10.1039/D4SC05226A
(Edge Article)
Chem. Sci., 2024,
15, 18411-18418
Multiplexed detection of respiratory pathogens using a portable device combining a CREM strategy†
Received
5th August 2024
, Accepted 7th October 2024
First published on 8th October 2024
Abstract
Rapid and precise detection of respiratory pathogens is crucial for clinical diagnosis and treatment of respiratory infections. In this study, the multiplex and visual detection of respiratory pathogens is facilitated by specifically designed engineered CRISPR RNA (en-crRNA) to activate the trans-cleavage activity of Cas12a, along with a homemade portable device. The en-crRNA comprised an original crRNA and a DNA reporter molecule that is labelled with both a fluorophore and a quencher. Moreover, the DNA is partially complementary to the variable region of the original crRNA. The proof of concept was demonstrated by simultaneously identifying distinct respiratory pathogens with a detection limit of 102 copies per μL. The visual discrimination was subsequently achieved using a homemade portable device that was seamlessly integrated with a smartphone. The specificity of the strategy was validated by comparing with qPCR assays for clinical sample detection, demonstrating exceptional accuracy with areas under the ROC curves of 0.98 for all targets. The research provides a promising avenue for the development of rapid, specific, and on-site detection techniques aimed at multiplex identification of respiratory pathogens.
Introduction
Acute respiratory tract infection constitutes a prominent cause of morbidity and mortality among the pediatric population.1,2 Respiratory pathogens are detected in up to 80% of cases,3 presenting a significant threat to both the physical and mental well-being of children. Despite presenting similar clinical symptoms and signs, infections caused by diverse pathogens (such as viruses, bacteria, mycoplasma, etc.) necessitate distinct treatment approaches.4–6 In addition, the presence of multiple co-infections with pathogens further complicates the accurate diagnosis.7 Therefore, strategies enabling precise identification of respiratory pathogens are essential for accurate diagnosis and treatment.8
Routine clinical etiological examinations, including culture, immunological detection, nucleic acid detection, and gene sequencing, have gained widespread acceptance as valuable tools for identifying common pathogens.9 As the current gold standard, the culture-based technique requires a prolonged processing time, which is unsuitable for application in urgent clinical settings.10 On the other hand, the potential false negative outcomes in immunological tests cannot be disregarded.11 The real-time quantitative PCR (qPCR) and gene sequencing techniques exhibit remarkable sensitivity and specificity.12 However, their implementation requires highly trained personnel and specialized equipment, which limits their suitability for expedited screening during emergency scenarios,13 particularly when rapid field testing is essential.14,15 Hence, the urgent need for a multi-pathogen detection platform that facilitates sensitive, specific, and rapid analysis arises from the necessity of efficient diagnosis in complex on-site conditions.
CRISPR technology has emerged as a promising tool for the detection of nucleic acid molecules due to its simplicity, robustness, and exceptional specificity.16–19 Unfortunately, the nonspecific catalytic activity of Cas proteins poses a challenge in detecting multiple targets.20–22 The introduction of multiple Cas proteins with distinct trans-cleavage activities in a single reaction has been implemented to overcome this challenge and ultimately achieve multi-path nucleic acid detection.23–26 For instance, the SHERLOCKv2 system was enhanced with the integration of various Cas proteins (LwaCas13a, PsmCas13b, CcaCas13b, and AsCas12a) and distinctive fluorescent labeling to enable the detection of four specific targets.27 The limited availability and complexity associated with the utilization of multiple Cas proteins in such a strategy, however, may potentially undermine the practicality of the system. The utilization of droplet microfluidic technology has also been employed to facilitate CRISPR systems for multiple and quantitative detection.28 The presence of individual Cas proteins within parallel droplets allows for the identification of a wide range of targets (with at most one copy in each droplet), thereby facilitating multiplexed detection29,30 and enhancing the limit of detection.31 The requirement of specialized instruments and complex infrastructure, however, limits their suitability for point-of-care testing (POCT) in emergency situations.
The present study introduces a novel CRISPR and en-crRNA based multiplex detection platform, termed the CREM platform, for precise and simultaneous identification of respiratory pathogens. Taking two sensing channels as an example, in general, the presence of targets A and B will trigger trans-cleavage mediated by Cas12a, resulting in the generation of distinct fluorescent signals. This enables simultaneous and independent detection using dual-channel technology. The performance of the CREM system was evaluated in four scenarios (Fig. 1a and b): (1) undetectable fluorescence signal in the absence of both targets; (2) Alexa Fluor 488 signal collected in the presence of target A; (3) Cy5 signal collected in the presence of target B; (4) Alexa Fluor 488 and Cy5 signals simultaneously collected in the presence of both targets. The interaction between each target and its complementary crRNA, labeled with spectra-discriminative fluorophores, was specific and devoid of any cross-reactivity. This facilitated the effective identification of multiple target nucleic acids within a single reaction tube. An enzymatic recombinase amplification (ERA) strategy can further enhance the sensitivity of clinical sample detection. Additionally, a 3D-printed device incorporating temperature control and an optical system has been seamlessly integrated for portable application, facilitating real-time monitoring of multiple fluorescence signals from the samples using a smartphone.
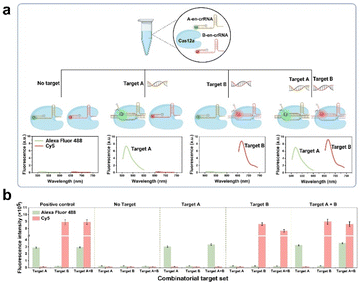 |
| Fig. 1 Cas12a/en-crRNA-based multiplex detection strategy: (a) schematic illustration of the CREM system, taking two channels' detection as an example. Illustrations created by Biorender. (b) Fluorescence signals collected toward different analysis scenarios. | |
Results and discussion
En-crRNA-assisted regulation of Cas12a trans-cleavage activity
The design incorporates an en-crRNA to augment the selectivity of Cas12a in cleaving ssDNA, thereby reducing non-specific cleavage.32 The classic en-crRNA comprises a conventional crRNA and a DNA reporter labeled with a fluorophore–quencher pair. The reporter was designed to complement the variable region of the original crRNA. The strategy can contribute to minimizing the background fluorescence signal, with the exception of cases where the target is bound to the crRNA spacer region. The DNA reporter is typically released in the presence of a target and undergoes Cas12a-mediated trans-cleavage, resulting in an increased distance between the fluorophore and quencher. This leads to the generation of a robust fluorescence signal (Fig. 2a).
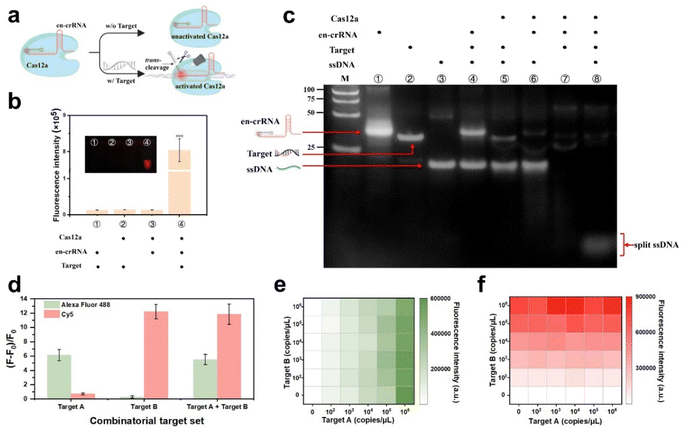 |
| Fig. 2 En-crRNA-assisted regulation of Cas12a trans-cleavage activity. (a) Schematic illustration of the en-crRNA-assisted regulation of Cas12a trans-cleavage activity. Illustrations created by Biorender. (b) Plots depicting the reaction of Cas12a with en-crRNA in various components. Values represent mean ± standard deviation (n = 3). (c) PAGE analysis of the en-crRNA-assisted regulation of Cas12a trans-cleavage reaction. (d) The (F − F0)/F0 values of Alexa Fluor 488 and Cy5 after the detection of synthetic targets using the CREM system. F and F0 represent the fluorescence intensities obtained from the sample and the no target control (NTC), respectively. Values represent mean ± standard deviation (n = 3). (e) The fluorescence heat-map analysis for the detection of target A at diverse concentrations in the presence of target B. (f) The fluorescence heat-map analysis for the detection of target B at various concentrations in the presence of target A. | |
The successful construction of the en-crRNA was verified through polyacrylamide gel electrophoresis (PAGE) analysis (Fig. S1†). Cas12a complexed with en-crRNA exhibited potent trans-cleavage activity on the reporter DNA, resulting in an enhanced fluorescence signal. The low fluorescence signal observed in Fig. 2b was attributed to the absence of reactive components, as further confirmed by PAGE analysis (Fig. 2c). The presence of the target significantly enhanced the trans-cleavage efficiency when employing the en-crRNA and Cas12a ribonucleoprotein (RNP) complex, in stark contrast to the absence of the target. The ssDNA fragments can be observed at the bottom of the gel (line 8), demonstrating the effective modulation of Cas12a trans-cleavage activity by en-crRNA.
Multiplex detection in a single-tube
The RNPs, consisting of en-crRNA and Cas12a, effectively address the issue of non-specific cleavage caused by the Cas protein, thereby demonstrating their potential for multiplex detection in a single reaction (see Fig. S2†). Synthetic targets A and B that matched the individual en-crRNAs were prepared to validate the performance of the system at first (Table S1†). The experiment entailed incubating two distinct types of en-crRNAs and Cas12a with various combinations of target nucleic acids, as illustrated in Fig. S3a.† The bar graph depicted in Fig. 2d illustrates the variation in fluorescence intensity (F − F0)/F0 across different targets. The distinct fluorescence signals emitted by Alexa Fluor 488 (λex/λem = 490/520 nm) and Cy5 (λex/λem = 643/667 nm) were individually observed in the presence of target A and target B. The dual-responsive fluorescent signal could be simultaneously detected in both channels when targets A and B were present (Fig. 2d and S3b†), while the absence of either targets resulted in a significantly diminished signal.
The performance of the system prepared in advance was further investigated in terms of its ability to handle varying target concentrations. As shown in Fig. 2e and f, the system demonstrated its capability to generate specific fluorescence responses to each target at different target concentrations, indicating the potential of simultaneous and accurate detection of diverse types of targets. Meanwhile, the interference caused by non-target substances can be effectively mitigated. In addition, the (F − F0)/F0 value exhibited robust linear correlations with the concentrations of target A (R2 = 0.9989) and target B (R2 = 0.9877) respectively, thereby confirming its exceptional quantitative detection capability (Fig. S4†).
Optimization of the CREM system
The cleavage activity of Cas12a protease is highly reliant on the optimal concentrations of both Cas12a and crRNA, which are necessary for the formation of RNPs.33 The ratio of (F − F0)/F0 exhibited an increase with higher concentrations of Cas12a and en-crRNA, resulting in the identification of distinct peaks at 100 nM (Fig. S5a†) and 750 nM (Fig. S5b†), respectively. Besides, the (F − F0)/F0 ratio reaches its plateau value at a temperature of 40 °C (Fig. S5c†) and after 60 minutes of reaction time (Fig. S5d†). Additionally, the trans-cleavage activities were significantly enhanced by TOLO Buffer compared to alternative buffers, as shown in Fig. S6.† Furthermore, the signals obtained from Cas12a-based sensing were compared to those generated by Cy5 double-labeled en-crRNA, individually labeled en-crRNA, and a combination of multiple en-crRNAs (Fig. S7†). The detection limit of the en-crRNA system was determined to be 103 copies per μL by incorporating a single Cy5 fluorophore labeled at the 5′ end of DNA. The suboptimal performance of the en-crRNA system featuring two fluorophores may be attributed to fluorescence quenching resulting from the close proximity of these fluorophores.34
Considering the advantage of simultaneous activation of multiple crRNAs targeting distinct regions within the same nucleic acid molecule,35 further investigation was conducted on the CREM system's contribution to enhanced sensitivity. Fig. S8a† presents two distinct target sequences, each designed with two en-crRNAs for a specific site. The combination of en-crRNA 1 and 2 results in an increased fluorescence intensity for the target at three different concentrations (3 × 103 copies per μL, 1 × 104 copies per μL, and 1 × 105 copies per μL). Additionally, based on Fig. S8c,† there was a slight increase in fluorescence intensity observed in the system with individually labeled en-crRNA when a small quantity of target (3 × 103 copies per μL) was present. However, significant enhancement can be achieved by combining en-crRNAs. The experimental results have validated that the incorporation of crRNA can effectively enhance the system's sensitivity.
Identification of three gene types using the CREM system
Respiratory infections are the most common infectious diseases in children.36,37 Following the pandemic, there has been a discernible surge in the prevalence of diverse respiratory ailments.38 Children afflicted with respiratory infections frequently experience co-infections caused by multiple pathogens, underscoring the criticality of accurately identifying distinct pathogen types for efficacious treatment and control. In this section, three pathogens including S. pneumoniae, H. influenzae, and M. pneumoniae were utilized as models to explore the potential of the CREM system in multiplex detection.
Based on the conserved genes of these pathogens reported in previous studies,39–41 specific en-crRNAs targeting cpsB, siaT, and P1 genes were designed (Fig. S9†). As depicted in Fig. S10,† successful differentiation of these genes could be confirmed using the PAGE analysis. By incubating Cas12a with the en-crRNAs and target genes, distinct fluorescence signals corresponding to each pathogen can be observed in principle (Fig. 3a). The preparation of Cas12a with three types of en crRNAs and various combinations of target genes was conducted according to the procedure outlined in Fig. S11.† As shown in Fig. 3b and c, the green fluorescence signal corresponds to the cpsB gene from S. pneumoniae (2, 5, 6, and 8 tubes), while the orange fluorescence represents the siaT gene from H. influenzae (3, 5, 7, and 8 tubes). Additionally, the red fluorescence represents the P1 gene from M. pneumoniae (4, 6, 7, and 8 tubes). The study simultaneously analyzed the three genes by utilizing the (F − F0)/F0 ratio (Fig. 3d). The significantly enhanced fluorescence signal of Alexa Fluor 488 (λex = 490 nm and λem = 520 nm) indicates the presence of the cpsB gene. Similarly, increased Alexa Fluor 568 (λex = 578 nm and λem = 603 nm) and Cy5 signals (λex = 643 nm and λem = 667 nm) can be observed, indicating the presence of the siaT gene and P1 gene, respectively. The findings suggest that the CREM system exhibits the capacity to simultaneously identify distinct targets and selectively cleave attached reporters that are labeled with diverse fluorophores.
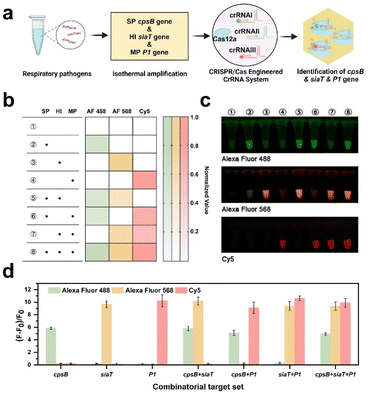 |
| Fig. 3 Identification of three gene types using the CREM system. (a) Schematic illustration of the identification of three gene types using the CREM system. Illustrations by Biorender. (b) Fluorescence signals of the CREM system in the absence and presence of three genes. (c) Fluorescence images of reaction tubes after detecting three genes. (d) The (F − F0)/F0 values of Alexa Fluor 488, Alexa Fluor 568, and Cy5 after the detection of distinct genes using the CREM system. Values represent mean ± standard deviation (n = 3). | |
Assessment of the sensitivity and specificity of the CREM system for respiratory pathogen detection
Subsequently, the sensitivity and specificity of the CREM system were assessed for the detection of three gene types from diverse pathogens, based on optimal conditions. The system demonstrated a detection sensitivity of 102 copies per μL. Notably, the fluorescence signal exhibited a significant linear correlation (R2 = 0.9978) with the concentration of the cpsB gene, ranging from 1 × 102 to 5 × 105 copies per μL (Fig. 4a). A similar linear relationship was found for the siaT gene concentration (102–106 copies per μL, R2 = 0.9919) (Fig. 4b). The detection limit of the P1 gene using the CREM system was determined to be 3 × 102 copies per μL, with a dynamic range spanning from 3 × 102 to 5 × 105 copies per μL (R2 = 0.9952, Fig. 4c). Furthermore, the specificity of the CREM system was confirmed by successfully detecting nucleic acids derived from a wide range of human respiratory pathogens (S. pneumoniae, H. influenzae, M. pneumoniae, S. agalactiae, S. aureus, E. coli, K. pneumoniae, and P. aeruginosa). The fluorescence signals of Alexa Fluor 488, Alexa Fluor 568, and Cy5 confirmed the presence of cpsB, siaT, and P1 genes, respectively, whereas other samples exhibited only a faint signal in close proximity to background levels (Fig. 4d). The fluorescence images provided additional evidence to substantiate the exceptional selectivity of the CREM system (Fig. 4d, inset). The obtained results have confirmed that the as-constructed CREM system does not exhibit any cross-reactivity with the tested human respiratory pathogens, thereby demonstrating the exceptional specificity. Furthermore, Table S2† presents a range of previously reported assays for pathogen detection, thereby confirming the robust applicability of the CREM system.
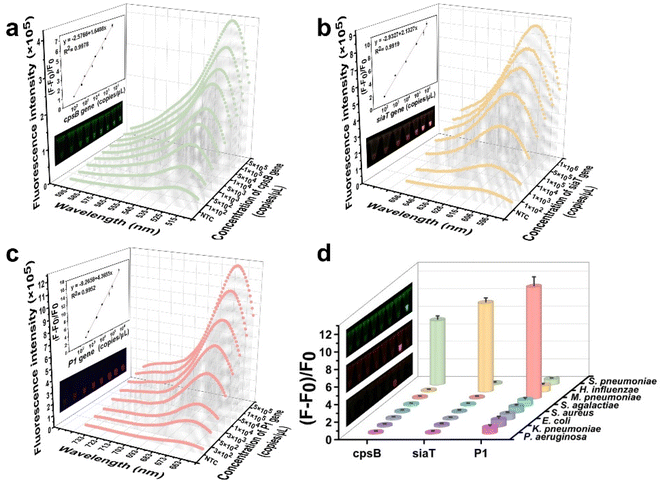 |
| Fig. 4 Evaluation of the sensitivity and specificity of the CREM system on respiratory pathogens. (a) Relationship between the fluorescence intensity and various concentrations of the cpsB gene. (b) Relationship between the fluorescence intensity and various concentrations of the siaT gene. (c) Relationship between the fluorescence intensity and various concentrations of the P1 gene. The inset shows the linear plot of the (F − F0)/F0versus various gene concentrations and their corresponding fluorescence images. (d) (F − F0)/F0 values after the detection of various pathogen nucleic acids using the CREM. Values represent mean ± standard deviation (n = 3). | |
Fluorescence signal read on a chip using a handheld device
The current readout of fluorescence intensity often requires complex optical systems, which limits its practical applicability in point-of-care testing (POCT). To address this issue, a handheld device with a temperature control and optical system was designed for fluorescence imaging acquisition. The original design blueprint is presented in Fig. S12.† The positioning of the two LED flats should be duly noted, as they are placed orthogonally and inclined at a 45-degree angle relative to the horizontal plane. The high throughput parallel detection of the multi-samples was facilitated by utilizing a PDMS-fabricated array chip. The precision of our custom-built setup is validated by the uniform irradiation intensity observed across all arrays, as demonstrated in Fig. 5c through a preliminary analysis of fluorescence.
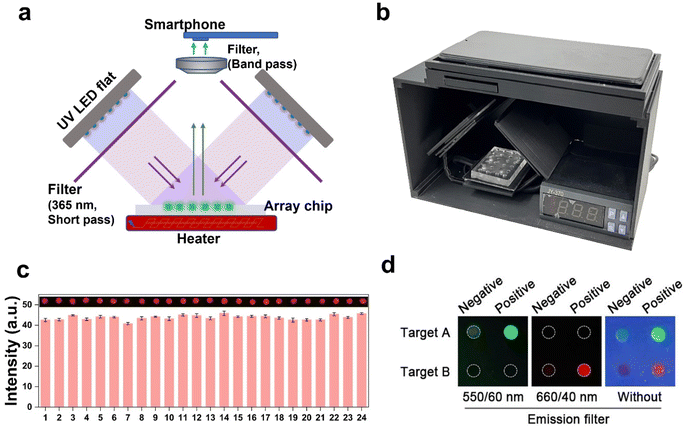 |
| Fig. 5 Design of a portable multi-channel detection device. (a) Schematic illustration of the optical system. (b) The picture of a homemade portable device and smart phone. (c) Measurement of fluorescent intensity in each well. The values of intensity represent mean ± standard deviation (n = 3) with a relative standard deviation <3.6%. (d) Evaluation of the proposed system by imaging signals using the POCT device in (b). | |
The schematic diagram and visual representation of the device are depicted in Fig. 5a and b. The excited light is directed onto the sample after passing through a 365 nm filter, and subsequently, the resulting fluorescent signal can be captured by the phone using either the 550/60 nm or 660/40 nm band-pass filters (Fig. 5d). The loading of samples and acquisition of results can be easily accomplished with the utilization of this device. The fluorescence images captured by the smartphone and processed using Image J provide visually intuitive data for result discrimination, thereby mitigating potential misjudgments arising from visual biases. The detection threshold was determined by calculating the mean value plus three times of standard deviation of pixel intensity obtained from the NTC sample. The sample was classified as positive if the fluorescence pixel intensity exceeded the detection threshold; otherwise, it was categorized as negative.
The visual device was employed to demonstrate its multi-detection capability, wherein three respiratory pathogens were loaded and fluorescent signals were recorded following a 60-minute incubation period. The results depicted in Fig. S13† demonstrate that the analytical sensitivity of the device corresponds well to the data obtained from the fluorometer (Fig. 3c). Therefore, the device facilitates the simple and rapid detection of multiple nucleic acids, providing a reliable, visual, and user-friendly approach for on-site molecular diagnosis.
Clinical sample analysis
After undergoing preclinical validation, artificial nasopharyngeal swabs containing the aforementioned three pathogens were subjected to CREM using qPCR to confirm their efficacy (Fig. 6a). The assay results for S. pneumoniae, H. influenzae, and M. pneumoniae in 28 samples were basically consistent with the qPCR results (Fig. S17–S19†), with the exception of three cases: false positives for M. pneumoniae in sample #14, S. pneumoniae and M. pneumoniae in sample #15, and H. influenzae in sample #25 (Fig. 6b). Significant statistical difference between positive and negative groups for the three pathogens has been identified successfully via the CREM system (Fig. 6c and S14†). A specificity of 95.2%, 95.7%, and 90.5% for the three pathogens was determined by receiver operating characteristic (ROC) curve analysis, respectively; Similarly, areas under the curves of 0.98, 0.98, and 0.986 were observed (Fig. 6d), providing further evidence for the clinical potential of the CREM. The portable device effectively detected clinical samples, as illustrated in Fig. S15,† yielding identical outcomes to those obtained by the off-device test (Fig. S16†). The findings presented herein provide compelling evidence that supports the effectiveness of the proposed assay as a diagnostic tool for detecting infections caused by multiple pathogens.
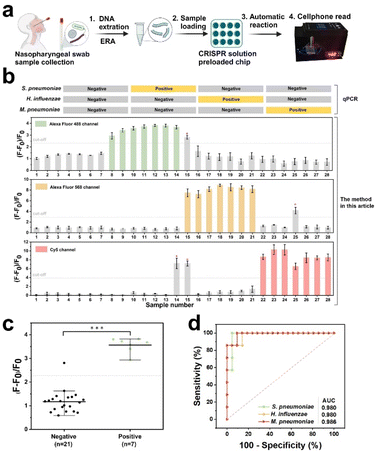 |
| Fig. 6 Clinical sample analysis using the CREM system. (a) Schematic illustration of clinical sample analysis using the CREM system. Illustrations by Biorender. (b) qPCR results and CREM signals for three pathogens. Cut-off values in each channel were calculated from ROC curves (2.35, 2.96 and 3.96 for (F – F0)/F0 of AF488, AF568, and Cy5, respectively). Values represent mean ± standard deviation (n = 3). (c) Scatter plot showing (F − F0)/F0 of 21 negative and 7 positive samples for S. pneumoniae in the Alexa Fluor 488 channel. The statistical significance of the data was determined using two-tailed unpaired Student's t-test (***p < 0.001). (d) ROC curve analysis of the CREM signals. | |
Experimental
Validation of the CREM system for simultaneous multiple target detection
Validation of Cas12a/en-crRNA system-mediated target cleavage experiments was conducted using fluorescence and PAGE electrophoresis. The Cas12a/en-crRNA system was tested in a 20 μL reaction volume containing 100 nM Cas12a, 750 nM en-crRNA labeled with Alexa Fluor 488, 750 nM en-crRNA labeled with Cy5, and varying concentrations of nucleic acid fragments in TOLO buffer. The mixture was then incubated at 40 °C for 1 hour. Finally, the fluorescence intensity was recorded with an Edinburgh FS5 fluorescence spectrofluorometer. Specifically, the excitation wavelength for Alexa Fluor 488 fluorescence was set at 490 nm, with an emission wavelength of 520 nm. For Cy5 fluorescence, the excitation and emission wavelengths were set at 643 nm and 667 nm respectively. The fluorescence images were captured using a Tanon fully automated digital imaging system, employing distinct emission filters for the Alexa Fluor 488 and Cy5 channels.
Portable multi-channel device fabrication
A portable device was designed for sample analysis, consisting of two UV LED panels, excitation and emission filters, heaters, and a smartphone (Fig. 5a and b). The blueprint of the device was designed using Sketchup and printed by a 3D printer using the PLA material. The UV LED panel is comprised of 20 LED beads (5 V, 2 W). The excitation filter selectively permits the transmission of UV light at a wavelength of 365 nm. The selection of emission filters with different band passes is based on the fluorescent group's emission peak wavelength, including 550/60 nm, 605/55 nm, and 660/40 nm. The reaction chip was prepared using the polydimethylsiloxane (PDMS) precursor following the previous work.42 The reaction reagents were loaded into the chip, followed by 60-minute incubation on the heater and subsequent multichannel testing. The fluorescence image was then recorded by the smartphone and analyzed by image J.
Clinical samples
The CREM system was clinically validated using a total of 28 human nasopharyngeal swab samples, which were subsequently confirmed by qPCR. The sensitivity and specificity of the CREM system were assessed by generating Receiver Operating Characteristic (ROC) curves with IBM SPSS Statistics.
Conclusions
The present study underscores the efficacy and feasibility of employing a multiple detection strategy for respiratory pathogens utilizing CRISPR/Cas12a and en-crRNA. The platform is capable of simultaneously identifying three distinct respiratory bacterial pathogens simultaneously, with a detection limit of 102 copies per μL. Enhanced sensitivity was achieved by targeting distinct regions with multiple crRNAs in the same target, thereby augmenting the trans-cleavage activity of Cas12a. The areas under the ROC curves for detecting all three pathogens can achieve a remarkable value of 0.98. Furthermore, a custom-designed 3D-printed apparatus integrated with a PDMS array chip facilitates the continuous real-time monitoring of fluorescence signals using a smartphone, enabling the realization of portable and efficient detection. The achievement demonstrates the potential of a multiplex strategy for diagnosing respiratory pathogens in a single reaction tube using a single Cas protein under isothermal conditions, providing a promising tool for detecting respiratory infections in children.
Data availability
The data that support the findings of this study are available on request from the corresponding author.
Author contributions
Xijuan Gu: conceptualization, methodology, investigation, writing – review & editing; Anli Pan: scientific experiment; Lingwei Wu: data curation, formal analysis; Jing Zhang: conceptualization, methodology; Zixun Xu: 3D printed device design; Tao Wen: scientific experiment; Miaomiao Wang: 3D printed device printing; Xiuying Shi: bacteria preparation; Li Wu: conceptualization, funding acquisition; Yuling Qin: supervision, writing – reviewing and editing, project administration, funding acquisition.
Conflicts of interest
There are no conflicts to declare.
Acknowledgements
This work was supported by the National Natural Science Foundation of China (82373456 and 32171452), Jiangsu Innovative and Entrepreneurial Research Team Program (JSSCTD202348), Excellent Youth Foundation of Jiangsu Scientific Committee (BK20220060), Natural Science Re-search Project of Higher Education in Jiangsu Province (23KJB150028), and Large Instruments Open Foundation of Nantong University.
References
- M. Echavarría, D. N. Marcone, M. Querci, A. Seoane, M. Ypas, C. Videla, C. O'Farrell, S. Vidaurreta, J. Ekstrom and G. Carballal, J. Clin. Virol., 2018, 108, 90–95 CrossRef PubMed.
- Y. Li, X. Fu, J. Ma, J. Zhang, Y. Hu, W. Dong, Z. Wan, Q. Li, Y.-Q. Kuang, K. Lan, X. Jin, J.-H. Wang and C. Zhang, Nat. Commun., 2019, 10, 2288 CrossRef PubMed.
- H. E. Al-Romaihi, M. K. Smatti, H. A. Al-Khatib, P. V. Coyle, N. Ganesan, S. Nadeem, E. A. Farag, A. A. Al Thani, A. Al Khal, K. M. Al Ansari, M. A. Al Maslamani and H. M. Yassine, Int. J. Infect. Dis., 2020, 95, 133–141 CrossRef PubMed.
- C. K. Y. Chan, J. Tao, O. S. Chan, H.-B. Li and H. Pang, Adv. Nutr., 2020, 11, 979–988 CrossRef PubMed.
- G.-S. Liu, P.-H. Niu, S.-C. Zhao, R.-J. Lu and W.-J. Tan, J. Med. Virol., 2019, 91, 564–569 CrossRef CAS PubMed.
- M. Gromala, D. Neufeld Josh and J. McConkey Brendan, Appl. Environ. Microbiol., 2021, 87, e02914–e02920 CrossRef CAS PubMed.
- C. Zhang, J. Gu, Q. Chen, N. Deng, J. Li, L. Huang and X. Zhou, PLoS Med., 2020, 17, e1003130 CrossRef CAS PubMed.
- P. Q. M. Nguyen, M. Wang, N. Ann Maria, A. Y. Li, H. Y. Tan, G. M. Xiong, M.-K. M. Tan, A. A. S. Bhagat, C. W. M. Ong and C. T. Lim, Microsyst. Nanoeng., 2022, 8, 82 CrossRef CAS PubMed.
- W. Kim Jeong, S. Hong Seong, S. Lee In, Y. Chi Hyun, S.-O. Kim, N. Kim Hyeong and P. Hong Sun, J. Clin. Microbiol., 2020, 58, e01500–e01519 Search PubMed.
- B. Waites Ken, L. Xiao, Y. Liu, F. Balish Mitchell and T. P. Atkinson, Clin. Microbiol. Rev., 2017, 30, 747–809 CrossRef CAS PubMed.
- J. Stærk-Østergaard, C. Kirkeby, L. E. Christiansen, M. A. Andersen, C. H. Møller, M. Voldstedlund and M. J. Denwood, J. Med. Virol., 2022, 94, 4754–4761 CrossRef PubMed.
- H. G. Lee, W. Choi, S. Y. Yang, D.-H. Kim, S.-G. Park, M.-Y. Lee and H. S. Jung, Sens. Actuators, B, 2021, 326, 128802 CrossRef CAS.
- D. Treggiari, C. Piubelli, S. Caldrer, M. Mistretta, A. Ragusa, P. Orza, B. Pajola, D. Piccoli, A. Conti, C. Lorenzi, V. Serafini, M. Boni and F. Perandin, J. Med. Virol., 2022, 94, 1190–1195 CrossRef CAS PubMed.
- E. Savvateeva, O. Smoldovskaya, G. Feyzkhanova and A. Rubina, Crit. Rev. Clin. Lab Sci., 2021, 58, 17–28 CrossRef CAS PubMed.
- J. Shin, T. Yoon, J. Park and K. S. Park, Sens. Actuators, B, 2022, 365, 131871 CrossRef CAS.
- J. S. Gootenberg, O. O. Abudayyeh, J. W. Lee, P. Essletzbichler, A. J. Dy, J. Joung, V. Verdine, N. Donghia, N. M. Daringer, C. A. Freije, C. Myhrvold, R. P. Bhattacharyya, J. Livny, A. Regev, E. V. Koonin, D. T. Hung, P. C. Sabeti, J. J. Collins and F. Zhang, Science, 2017, 356, 438–442 CrossRef CAS PubMed.
- J. S. Chen, E. Ma, L. B. Harrington, M. Da Costa, X. Tian, J. M. Palefsky and J. A. Doudna, Science, 2018, 360, 436–439 CrossRef CAS PubMed.
- S.-Y. Li, Q.-X. Cheng, J.-M. Wang, X.-Y. Li, Z.-L. Zhang, S. Gao, R.-B. Cao, G.-P. Zhao and J. Wang, Cell Discov., 2018, 4, 20 CrossRef PubMed.
- X. Gu, Q. Tang, Y. Zhu, C. Sun, L. Wu, H. Ji, Q. Wang, L. Wu and Y. Qin, Biosens. Bioelectron., 2024, 261, 116449 CrossRef CAS PubMed.
- R. Marquez-Costa, R. Montagud-Martinez, M. C. Marques, E. Albert, D. Navarro, J. A. Daros, R. Ruiz and G. Rodrigo, Anal. Chem., 2023, 95, 9564–9574 CrossRef CAS PubMed.
- E. Xiong, L. Jiang, T. Tian, M. Hu, H. Yue, M. Huang, W. Lin, Y. Jiang, D. Zhu and X. Zhou, Angew. Chem., Int. Ed., 2020, 60, 5307–5315 CrossRef PubMed.
- X. Gu, Q. Tang, X. Kang, H. Ji, X. Shi, L. Shi, A. Pan, Y. Zhu, W. Jiang, J. Zhang, J. Liu, M. Wu, L. Wu and Y. Qin, Talanta, 2024, 271, 125678 CrossRef CAS PubMed.
- J. Fu, L. Zhang, Y. Long, Z. Liu, G. Meng, H. Zhao, X. Su and S. Shi, Anal. Chem., 2023, 95, 16089–16097 CrossRef CAS PubMed.
- J. Han, J. Shin, E. S. Lee, B. S. Cha, S. Kim, Y. Jang, S. Kim and K. S. Park, Biosens. Bioelectron., 2023, 232, 115323 CrossRef CAS PubMed.
- Z. Shiying, Z. Shuyu, H. Zhen, C. Jian, L. Jiawei, Y. Mei, J. Liang, H. Danqun and H. Changjun, Chem. Commun., 2023, 59, 11987–11990 RSC.
- T. Tian, Z. Qiu, Y. Jiang, D. Zhu and X. Zhou, Biosens. Bioelectron., 2022, 196, 113701 CrossRef CAS PubMed.
- J. S. Gootenberg, O. O. Abudayyeh, M. J. Kellner, J. Joung, J. J. Collins and F. Zhang, Science, 2018, 360, 439–444 CrossRef CAS PubMed.
- S. Yuting, X. Gaowa, L. Jiaxu, L. Yuxuan, L. Yongning, C. Shulang and L. Jin-Ming, Biosens. Bioelectron., 2023, 243, 115771 Search PubMed.
- D. Cai, Y. Wang, J. Zou, Z. Li, E. Huang, X. Ouyang, Z. Que, Y. Luo, Z. Chen, Y. Jiang, G. Zhang, H. Wu and D. Liu, Adv. Sci., 2023, 10, 2205863 CrossRef CAS PubMed.
- C. M. Ackerman, C. Myhrvold, S. G. Thakku, C. A. Freije, H. C. Metsky, D. K. Yang, S. H. Ye, C. K. Boehm, T.-S. F. Kosoko-Thoroddsen, J. Kehe, T. G. Nguyen, A. Carter, A. Kulesa, J. R. Barnes, V. G. Dugan, D. T. Hung, P. C. Blainey and P. C. Sabeti, Nature, 2020, 582, 277–282 CrossRef CAS PubMed.
- T. Tian, B. Shu, Y. Jiang, M. Ye, L. Liu, Z. Guo, Z. Han, Z. Wang and X. Zhou, ACS Nano, 2021, 15, 1167–1178 CrossRef CAS PubMed.
- Z. Weng, Z. You, J. Yang, N. Mohammad, M. Lin, Q. Wei, X. Gao and Y. Zhang, Angew. Chem., Int. Ed., 2023, 62, e202214987 CrossRef CAS PubMed.
- D. Jinying, W. Xiaoya, H. Qiushi, S. Chongsi, L. Jiahao, S. Peng, S. Yan and Z. Lei, Biosens. Bioelectron., 2023, 241, 115673 CrossRef PubMed.
- S. J. Bunce, Y. Wang, K. L. Stewart, A. E. Ashcroft, S. E. Radford, C. K. Hall and A. J. Wilson, Sci. Adv., 2019, 5, eaav8216 CrossRef PubMed.
- P. Fozouni, S. Son, M. Díaz de León Derby, G. J. Knott, C. N. Gray, M. V. D'Ambrosio, C. Zhao, N. A. Switz, G. R. Kumar, S. I. Stephens, D. Boehm, C.-L. Tsou, J. Shu, A. Bhuiya, M. Armstrong, A. R. Harris, P.-Y. Chen, J. M. Osterloh, A. Meyer-Franke, B. Joehnk, K. Walcott, A. Sil, C. Langelier, K. S. Pollard, E. D. Crawford, A. S. Puschnik, M. Phelps, A. Kistler, J. L. DeRisi, J. A. Doudna, D. A. Fletcher and M. Ott, Cell., 2021, 184, 323–333 CrossRef CAS PubMed.
- C. Mengelle, J. M. Mansuy, A. Pierre, I. Claudet, E. Grouteau, P. Micheau, K. Sauné and J. Izopet, J. Clin. Virol., 2014, 61, 411–417 CrossRef CAS PubMed.
- T. T. Lam, J. W. Tang, F. Y. Lai, H. Zaraket, G. Dbaibo, S. Bialasiewicz, S. Tozer, J. M. Heraud, S. J. Drews, T. Hachette, P. K. Chan, E. S. Koay, H. K. Lee, K. K. Tee, Y. Liu, P. Fraaij, L. Jennings, M. Waris, M. Krajden, A. Corriveau, H. Jalal, H. Nishimura, P. Nymadawa, D. Badarch, A. Watanabe, A. Kabanda, T. Sloots, J. Kok, D. E. Dwyer and M. Koopmans, J. Infect., 2019, 79, 373–382 CrossRef PubMed.
- F. Xie, T. Cai, B. Jin, L. Gan, B. Cai, Y. Gao, S. Cao, L. Lei and L. Zhou, Heliyon, 2023, 9, e19592 CrossRef PubMed.
- M.-L. Zhou, Z.-R. Wang, Y.-B. Li, T. Kudinha, J. Wang, Y. Wang, M. Xiao, Y.-C. Xu, Z.-Y. Liu and P.-R. Hsueh, J. Microbiol. Immunol. Infect., 2022, 55, 870–879 CrossRef CAS PubMed.
- D. Marasini, J. Whaley Melissa, T. Jenkins Laurel, F. Hu, W. Jiang, N. Topaz, A. Chen, S. Schmink, J. Dolan Thomas, H. Harcourt Brian, H. Marjuki and X. Wang, J. Clin. Microbiol., 2022, 60, e02111–e02121 CrossRef PubMed.
- D. Vizarraga, A. Kawamoto, U. Matsumoto, R. Illanes, R. Pérez-Luque, J. Martín, R. Mazzolini, P. Bierge, O. Q. Pich, M. Espasa, I. Sanfeliu, J. Esperalba, M. Fernández-Huerta, M. P. Scheffer, J. Pinyol, A. S. Frangakis, M. Lluch-Senar, S. Mori, K. Shibayama, T. Kenri, T. Kato, K. Namba, I. Fita, M. Miyata and D. Aparicio, Nat. Commun., 2020, 11, 5188 CrossRef CAS PubMed.
- Y. Qin, L. Wu, T. Schneider, G. S. Yen, J. Wang, S. Xu, M. Li, A. L. Paguirigan, J. L. Smith, J. P. Radich, R. K. Anand and D. T. Chiu, Angew. Chem., Int. Ed., 2018, 57, 11378–11383 CrossRef CAS PubMed.
|
This journal is © The Royal Society of Chemistry 2024 |
Click here to see how this site uses Cookies. View our privacy policy here.