DOI:
10.1039/D4SC05518G
(Edge Article)
Chem. Sci., 2024,
15, 18419-18430
A simplified and efficient extracellular vesicle-based proteomics strategy for early diagnosis of colorectal cancer†
Received
16th August 2024
, Accepted 8th October 2024
First published on 15th October 2024
Abstract
Colorectal cancer (CRC) is a major cause of cancer-related death worldwide and an effective screening strategy for diagnosis of early-stage CRC is highly desired. Although extracellular vesicles (EVs) are expected to become some of the most promising tools for liquid biopsy of early disease diagnosis, the existing EV-based proteomics methods for practical application in clinical samples are limited by technical challenges in high-throughput isolation and detection of EVs. In the current study, we have developed a simplified and efficient EV-based proteomics strategy for early diagnosis of CRC. DSPE-functionalized beads were specifically designed that enabled direct capture of EVs from plasma samples in 10 minutes with good reproducibility and comprehensive proteome coverage. The single-pot, solid-phase-enhanced sample-preparation (SP3) technology was then combined with data-independent acquisition mass spectrometry (DIA-MS) for in-depth analysis and quantification of EV proteomes. From a cohort with 30 individuals including 11 healthy controls, 8 patients with adenomatous polyp and 11 patients with early-stage CRC, our streamlined workflow reproducibly quantified over 800 proteins from their plasma-derived EV samples, from which dysregulated protein signatures for molecular diagnosis of CRC were revealed. We selected a panel of 10 protein markers to train a machine learning (ML) model, which resulted in accurate prediction of polyp and early-stage CRC in an independent and single-blind validation cohort with excellent diagnostic ability of 89.3% accuracy. Our simplified and efficient clinical proteomic strategy will serve as a valuable tool for fast, accurate, and cost-effective diagnosis of CRC that can be easily extended to other disease samples for discovery of unique EV-based biomarkers.
Introduction
Colorectal cancer (CRC) represents the most common gastrointestinal tumor, with 1.8 million new cases (10% of the world's cancer total) and 940
000 deaths (9.4% of the world's cancer total) annually, which poses a great threat to human health worldwide.1 CRC carcinogenesis is a multistep and slow process that begins with the initial development from a normal epithelium to mild adenomatous polyp and finally progressing to invasive carcinoma, and a lengthy period was needed for the malignant transformation, which offers significant opportunities for the early diagnosis of CRC.2 If the disease can be diagnosed early at the stage of precancerous lesions (polyp) or early CRCs, curative treatments with endoscopic resection or surgical intervention can be applied resulting in improved prognosis and long-term survival.3–5 On the other hand, the best opportunity for treatment will be missed after the tumors already developed distant metastases, leading to a poor survival rate.6 Therefore, it is critical and highly desired to develop effective screening strategies for detecting precancerous polyp and early CRC.
Currently, colonoscopy combined with histopathology remains the gold standard and the most widely used method in clinical practice, however, it involves a highly invasive procedure, costly expenses and potential complications, resulting in poor patient compliance and limited resource availability for patients.4 Molecular diagnostics aims at the discovery of specific molecular phenotype changes with disease in biological fluids when there are no obvious pathological symptoms, which allows more accurate screening, diagnosis, prognosis and disease monitoring.7 In principle, it can serve as a less invasive, safe, and affordable strategy for molecular screening of CRC at the early stages. For example, enzyme-linked immunosorbent assay (ELISA),8 secretomics9 and gene expression profile analysis10 have been performed to discover various biomarkers for CRC, however, their diagnostic power still needs to be improved.
Extracellular vesicles (EVs) are lipid bilayer-enclosed bioactive vesicles of endocytic origin that are secreted by various types of cells and present in almost all biological fluids.11 Their cargo includes proteins, nucleic acids and metabolites, which are involved in intercellular communication processes and associated with disease progression.12 As the pathophysiological roles of EVs are increasingly recognized, EV-based proteomics have become popular tools for liquid biopsy of early disease diagnosis.13,14 Recently, Melo et al. reported that glypican-1 enriched on plasma-derived EVs could serve as a screening biomarker to detect the early stages of pancreatic cancer with 100% accuracy and sensitivity.15 Moreover, it was demonstrated that distinct protein expression patterns in tumor-derived EVs determine the organ sites of future metastasis.16 Specifically for CRC, Zheng and coworkers17 have introduced a DIA-based diagnostic tool and identified FGA in EVs as an early diagnostic biomarker of CRC with satisfactory accuracy, however, the ultracentrifugation method used for isolation of EVs is time-consuming, limiting its high-throughput application in clinical cohort samples.
Several approaches have been developed to isolate EVs with good specificity including ultracentrifugation (UC), size exclusion chromatography (SEC), polymer-based precipitation, immunoprecipitation (IP) and microfluidic chips.18 Nevertheless, they either require time-consuming operations, or are associated with high reagent costs, which are not generally compatible with large-scale clinical applications.19–21 A supramolecular exosome array has been reported by Tao and colleagues, which enables efficient capture and high-throughput analysis of exosomes.22 In particular, they introduced an amphiphile-dendrimer supramolecular probe (ADSP) onto magnetic beads or nitrocellulose membranes and captured exosomes from trace amounts of clinical samples for biomarker validation in hepatocellular carcinoma (HCC) patients. More recently, the same group combined the functionalized beads with phosphoproteomics to analyze EVs in cerebrospinal fluid (CSF) for monitoring the outcome of chemotherapy of primary central nervous system lymphoma (PCNSL), further demonstrating the potential of EV-based proteomics for clinical applications.23
Inspired by the aforementioned studies, we herein reported the development of a simplified and highly efficient EV-based proteomics strategy for early diagnosis of CRC (Fig. 1). By functionalizing beads with EV-targeting chemical moieties, we were able to isolate EVs from the plasma samples of patients and healthy controls in only a few minutes. The samples were then prepared by an optimized single-pot, solid phase-enhanced sample-preparation (SP3) technology and analyzed by DIA-MS. A machine learning model was developed based on a panel of protein biomarkers and was able to differentiate healthy individuals, polyp patients and early-stage CRC patients with good accuracy. The predictive model was subsequently evaluated using an independent and single-blind cohort to demonstrate its generality. Our streamlined protocol provides a robust tool to discover significantly differentiated protein markers for a quick, accurate and cost-effective diagnosis of CRC.
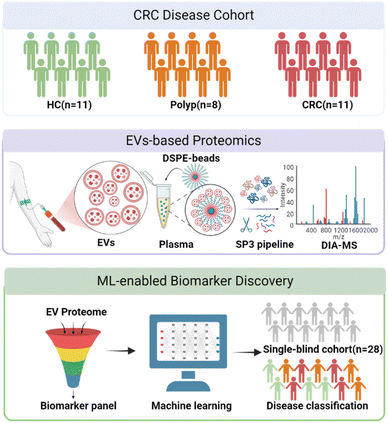 |
| Fig. 1 A general framework for clinical biomarker discovery based on EV proteomics for early diagnosis of CRC. A high-throughput, EV-based proteomics strategy was used to profile plasma samples from a cohort with individuals including healthy controls, patients with pre-malignant adenomas (polyp) and patients with early-stage CRC. Dysregulated EV proteomes were screened to identify stage-relevant protein signatures. A machine learning model was built to differentiate polyp and the early stages of CRC. Lastly, the model performance was validated in a single-blind and independent cohort. | |
Results and discussion
The design, synthesis and characterization of DSPE-functionalized beads
To facilitate a rapid and high-throughput workflow for the isolation of EVs, we developed a special agarose resin functionalized with 1,2-distearoyl-sn-glycero-3-phosphoethanolamine (DSPE) (Fig. 2A), which has been shown to capture EVs through inserting its hydrophobic structure of fatty acid esters into the lipid bilayer of EVs.24 By covalent conjugation of the EV-targeting probe DSPE onto agarose beads, we could generate a “multivalent interface” on the surface of the agarose resins to accomplish a streamlined enrichment of a substantial quantity of EVs within less than 10 minutes, circumventing the requirement for costly equipment and reagents. The DSPE-functionalized beads were conveniently synthesized utilizing a one-step coupling reaction between NHS ester-modified beads and NH2-PEG2000-DSPE (Fig. 2B). To examine whether DSPE-functionalized beads can effectively separate EVs from plasma, we systematically characterized the isolated EVs by common standards and features as reported in the literature.19,21 Firstly, we evaluated the presence of the commonly used EV biomarkers including CD63, TSG101, HSP70 and Alix and the absence of exclusion marker Calnexin by western blotting. As expected, we observed that EVs derived from plasma isolated by DSPE-Beads exhibited positivity for these EV biomarkers while demonstrating negative existence of Calnexin. These results confirmed the efficacy of our isolation protocol and the purity of the EV preparations, effectively ruling out contamination (Fig. 2C, loading controls for these western blots are provided in Fig. S1†). Additionally, we employed scanning electron microscopy (SEM) to visualize the beads' surface after EV isolation. Representative SEM images showed a smooth surface on the blank beads (without incubation with plasma) while the beads after EV capture could be clearly observed with uniform distribution of nanoparticles with a size of about 100 nm in diameter, which was consistent with the regular size of EVs (Fig. 2D).
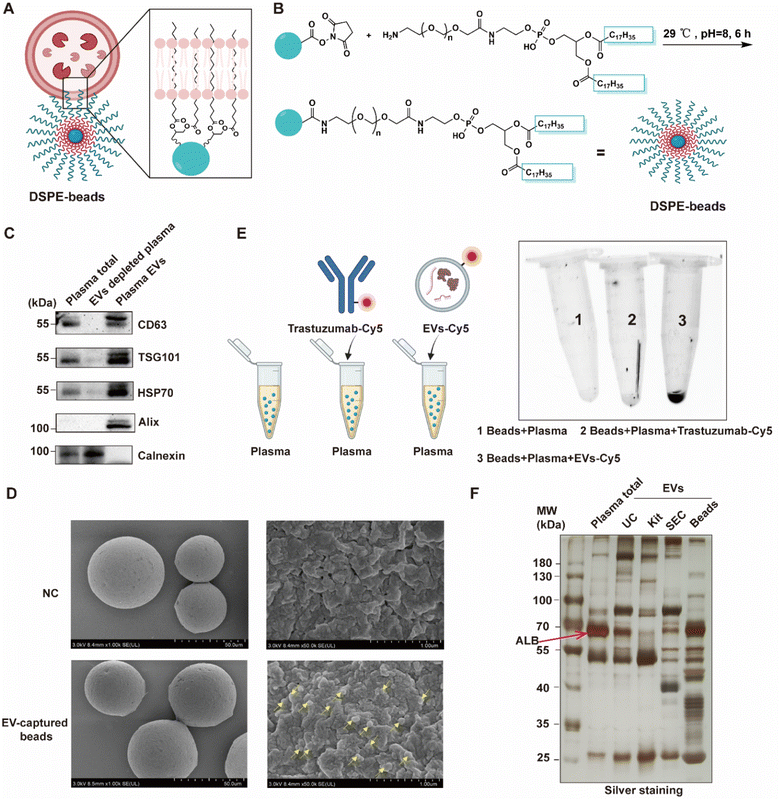 |
| Fig. 2 The design, synthesis and characterization of DSPE-functionalized beads. (A) Purification principle of EVs captured by DSPE-functionalized beads. (B) The DSPE-functionalized beads were synthesized by one-step coupling of DSPE-PEG2000-NH2 with NHS ester coated beads. (C) Western-blot analysis of well-known marker proteins from plasma, EV-depleted plasma and plasma EVs isolated by DSPE-functionalized beads. (D) The SEM image of prepared DSPE-functionalized beads and EVs captured on beads. (E) Enrichment of Cy5 fluorophore-conjugated EVs using DSPE-functionalized beads. Only tubes with the Cy5 fluorophore-conjugated EVs (tube 3) displayed bright fluorescence while no background fluorescence was observed in the control tubes (right). (F) Silver staining of gel-based protein profiles for plasma and plasma-derived EVs isolated by ultracentrifugation methods (“UC”), polymer precipitation (“Kit”), DSPE-functionalized beads (“Beads”) and size-exclusion chromatography (“SEC”). | |
To validate the method specificity, we spiked plasma samples with Cy5-labeled EVs or Cy5-labeled antibodies, while using regular plasma as a negative control. These samples were subjected to a DSPE-functionalized bead-based enrichment protocol. The results showed that Cy5-labeled EVs, but not Cy5-labeled antibodies, were successfully enriched from plasma, suggesting that our beads had low non-specific binding toward free proteins existing in biological fluids (Fig. 2E). To assess the versatility of our method, we extended this assay to other biological fluids, including urine and saliva. Similar results were obtained across all tested matrices, demonstrating the broad applicability of our DSPE-functionalized beads for EV isolation from various biological fluids (Fig. S2†).
To further evaluate our method, we conducted a comparative analysis of EV isolation techniques. We extracted EVs from plasma samples using four distinct methods: our DSPE-functionalized bead-based approach, ultracentrifugation (UC), a polymer-based precipitation kit (Kit), and size-exclusion chromatography (SEC). Subsequently, we performed a systematic characterization of the isolated EVs (Fig. S3†). Interestingly, the in-gel silver staining revealed that EVs extracted by the DSPE-functionalized beads contain diverse proteins compared to total plasma and EVs isolated by the other three methods (Fig. 2F), which will greatly facilitate subsequent proteomic studies.
Proteome composition of EVs isolated by DSPE-functionalized beads
The above-mentioned observations motivated us to explore the potential advantages of our method over conventional approaches at the proteomic level. We next performed a label-free quantitative proteomic analysis using DIA to compare the proteome compositions of EVs isolated by the DSPE-functionalized beads versus other common methods. Firstly, EVs were isolated from 200 μL of plasma from healthy donors at Peking University Shougang Hospital by the DSPE-functionalized beads, UC, Kit and SEC, and the BCA assay indicated that they contained 40, 40, 3000 and 20 μg protein per 200 μL of plasma, respectively (Fig. S4†). After normalization, 1 μg of each sample was subjected to proteomic analysis by mass spectrometry and the EV samples enriched with the DSPE-beads exhibited the greatest number of proteins on average compared with those prepared by the other three methods (Fig. 3A, Table S1 and S2†). Furthermore, the quantified proteins displayed a broad dynamic range (Fig. S5†). Notably, the proteomic profile of EVs enriched using our DSPE-functionalized beads encompassed over 85% of the proteins identified by all other methods (Fig. 3B). This extensive overlap, coupled with the detection of additional unique proteins, demonstrated that our DSPE-bead-based method achieved superior depth of proteome coverage. Concurrent with its superior protein identification capabilities, our DSPE-functionalized bead-based method also demonstrated excellent quantitative reproducibility. Label-free quantification analysis across three independently prepared and enriched samples revealed that over 80% of the total identified proteins exhibited a coefficient of variation (CV) below 20% (Fig. 3C). This high level of quantitative stability is comparable to that observed with the other three well-established EV isolation methods. Moreover, we utilized the top 100 EV proteins from the Vesiclepedia database (http://www.microvesicles.org/) as a benchmark, and assessed the detection rate of these well-known EV proteins across all four isolation methods. Our DSPE-functionalized bead-based approach demonstrated superior performance, identifying more canonical EV proteins compared to the other three methods (Fig. 3D). Building upon recent advancements in EV protein profiling, we assessed the ability of each isolation method to identify the 22 novel EV proteins that were recently reported as universally enriched across various cell types.21 Our method consistently identified 8 of these EV markers across all replicates, which are comparable to UC and SEC (both identifying 7). All three methods significantly outperformed the commercial kit, which identified only 1 (Fig. 3E). Meanwhile, the abundance of the top 100 high-abundance proteins in human plasma was much lower and not even detected in EVs isolated by the DSPE-functionalized beads as compared to those by UC, SEC and Kit isolation (Fig. 3F). However, due to the specific molecular structure of DSPE, our method inevitably enriched the family of lipoproteins from plasma as revealed by quantitative proteomics (Fig. 3F and S6†). Nevertheless, the cellular compartment analysis of the bead-enriched proteome dataset showed that the identified proteins are still predominantly localized in the extracellular exosomes, extracellular region, or extracellular space (Fig. 3G), consistent with the profiles observed for EVs isolated by the other three methods (Fig. S7†). Moreover, we conducted a functional enrichment analysis of the proteins identified by each method, focusing on the top 3 enriched molecular pathways. While the other three methods have primarily enriched proteins related to immune pathways, our DSPE-bead method uniquely showed significant enrichment in both immune-related and proteolysis-related pathways (Fig. S8†), which suggests that our method effectively captures EVs with more diverse functional properties. Collectively, these results demonstrated that the DSPE-functionalized beads have robust performance, high specificity and reproducibility in isolating EV samples from plasma, which offers a more comprehensive and accurate representation of the proteomic profile of EVs for potential disease biomarker discovery.
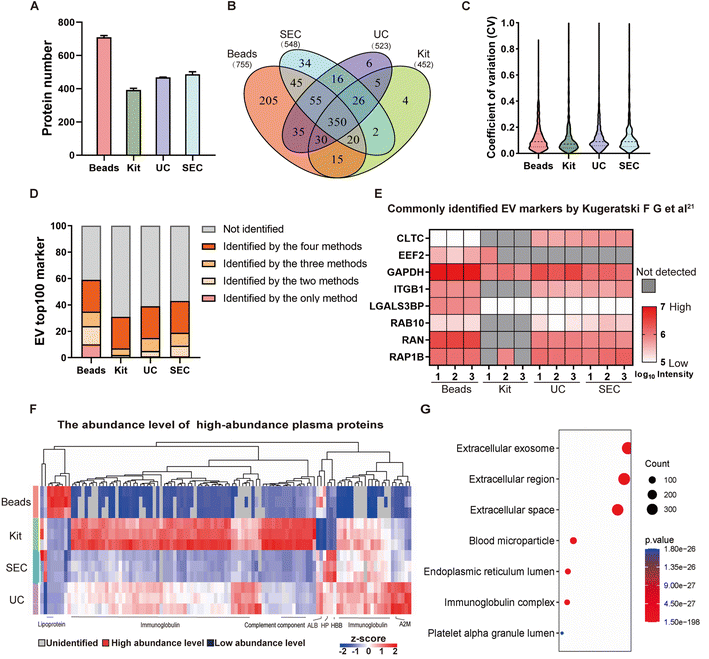 |
| Fig. 3 Performance evaluation of DSPE-functionalized beads to isolate EVs. (A) The number of proteins identified by the label-free MS from EVs isolated by different techniques. (B) Venn diagram analysis revealed a substantial overlap in the proteins identified by the different isolation techniques. (C) Distribution of coefficient of variation (CV) for proteins quantified from EVs isolated by different techniques. (D) Number of top 100 EV marker proteins (as defined in the Vesiclepedia database) identified from EVs isolated by different methods. (E) Heatmap of MS intensities of commonly identified EV markers by different methods from the dataset reported by Kugeratski F. G. et al.21 (F) Heatmap of the abundance level of the top 100 high-abundance plasma proteins identified from EV samples isolated by different methods. (G) Gene ontology of cellular components' annotations for the identified proteins in EV samples isolated by the DSPE-functionalized beads. | |
In addition, we also compared our one-step method with the previously reported two-step method which utilized the DSPE-biotin for EV labeling followed by neutravidin bead-mediated enrichment.24 Our method showed significant improvements in protein identification, quantification stability, missing value reduction, and non-specific binding reduction (Fig. S9 and Table S3†). Moreover, our approach led to a considerable reduction in the per-sample cost from about $10 to $0.28, thereby substantially cutting down the overall assay expenses for clinical applications. Furthermore, it shortened the total analysis time to 10 minutes, consequently augmenting both efficiency and productivity.
Optimization of DIA-based plasma EVs' proteome profiling
We next applied the DSPE-functionalized beads together with the data-independent acquisition (DIA) strategy25 aiming to establish a robust and high-throughput MS-based proteomics workflow for CRC biomarker discovery based on the EV proteome profiling (Fig. 1). DIA has become prevalent in large-scale clinical proteomic studies26,27 due to its capability of recording fragment ion information for all precursors within the sequential isolation window and allowing accurate and retrospective proteome quantification. Our approach was thoroughly optimized at all steps from sample preparation to data acquisition. Firstly, different lysis and extraction buffers were tested, including RIPA, 1% SDS, 8 M urea and 0.1% RapiGest SF. The results showed that RIPA yielded the best performance with over 600 unique proteins quantified (Fig. S10A†). Secondly, since the high-throughput proteolytic digestion of samples is indispensable for clinical proteomics, we also compared two common techniques for rapid sample preparation, including the filter aided sample preparation (FASP)28 method and the single-pot, solid phase-enhanced sample-preparation (SP3)29 technology. The results demonstrated that SP3 serves as a better choice (Fig. S10B†), which not only identified more proteins but also enabled a streamlined sample preparation process within a single tube. In addition, we employed the 40 min LC gradient for minimizing the analysis time without compromising protein identification capabilities (Fig. S10C†). Lastly, we further compared the performance of two pieces of DIA data analysis software and found that DIA-NN30 resulted in much more protein identification than Spectronaut with better reproducibility (Fig. S10D†).
Plasma EV proteome profiling of patients with early CRC
We then applied the DIA-based plasma EV proteome profiling for biomarker discovery in the diagnosis of early CRC. We collected plasma samples from an early CRC cohort consisting of subjects diagnosed with pre-malignant polyp (polyp, n = 8) or early-stage (stage I and II) colorectal cancer (CRC, n = 11) and healthy controls (HC, n = 11). The demographic information of these 30 cases is summarized in Table S4†. We extracted EVs from these plasma samples using the DSPE-functionalized beads and subjected them to the proteomic workflow as mentioned above. In total, 826 EV proteins were accurately quantified by DIA-MS across all 30 samples (Table S5†). The label-free quantification (LFQ) intensity of proteins identified in three groups spanned near six orders of magnitude (Fig. 4A), suggesting a wide range of protein abundance within and across the groups. For these samples, we first investigated the overall variance using the unsupervised principal component analysis (PCA) and found clear cluster separation between early-stage CRC patients and non-cancer population (HC and polyp), however, the distinction between the polyp and HC groups was not obvious (Fig. 4B). To further explore the potential markers between different groups, orthogonal partial least-squares discriminant analysis (OPLS-DA), receiver operating characteristic curve (ROC) analysis and Mann–Whitney U tests (MWU) were employed to search for those significantly altered proteins (OPLS-DA, VIP >1; ROC, AUC >0.85; MWU, P < 0.05) that contributed more substantially to differentiate HC, polyp and CRC. Such a differential analysis revealed 219 unique proteins, accounting for 27% of the total quantified EV proteomes. Among them, 40 proteins exhibited differential expression between HC and polyp, 154 proteins between HC and CRC, and 93 proteins between polyp and CRC (Fig. 4C), demonstrating significant abundance changes across various stages of disease progression (Fig. 4D and Table S6†).
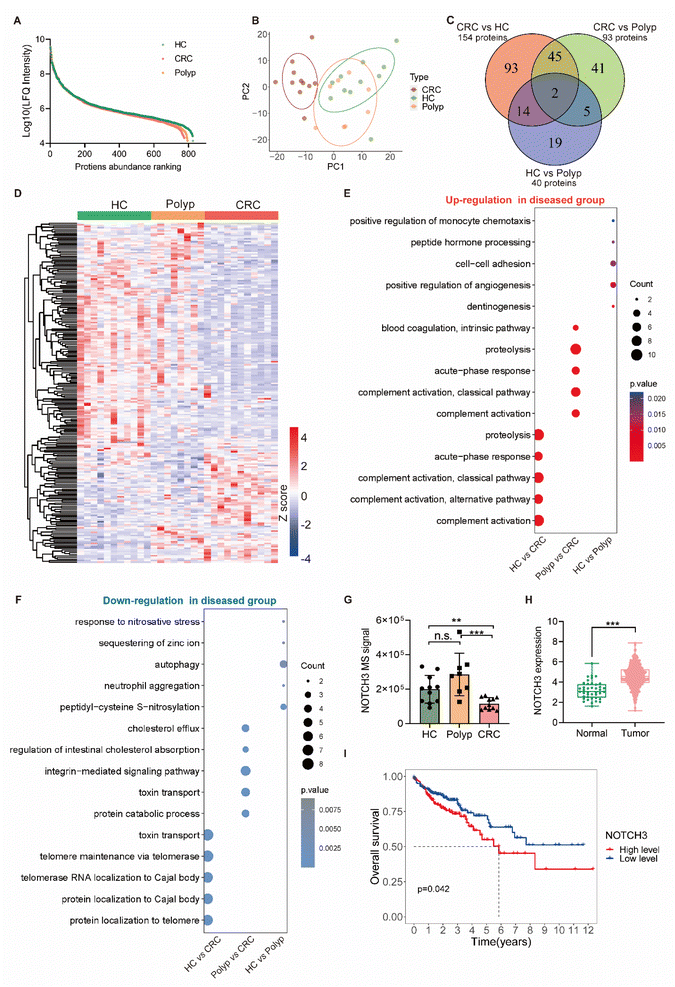 |
| Fig. 4 EV-based proteomic profiling from a clinical CRC cohort. (A) Dynamic ranges of the EV proteomes of the three groups in terms of the average protein label-free quantification (LFQ) intensity. (B) Principal component analysis (PCA) of EV proteomes among the healthy controls (HC), pre-malignant polyp (polyp) and early-stage colorectal cancer (CRC). (C) Venn diagram showing the number of potential protein biomarkers with abundance changes in different subgroups. (D) Supervised cluster analysis of proteins dysregulated (VIP >1, p < 0.05, AUC >0.85) in EV proteomes across the different groups during disease progression. (E and F) KEGG pathway analysis of proteins that showed significantly increased (E) and decreased (F) abundances in EV proteomic profiling. (G and H) Distribution of the expression levels of NOTCH3 in plasma derived EVs (G) and tissues (H) from the TCGA colon adenocarcinoma cohort, respectively. The Mann–Whitney U test was used to define statistical significance (p < 0.01 (**), p < 0.001 (***), p < 0.0001 (****)). (I) Overall survival (OS) analyses of CRC patients with high or low expression levels of NOTCH3 (according to The Cancer Genome Atlas database, https://portal.gdc.cancer.gov/). | |
Among these discovered targets, there were several previously reported protein markers, such as inflammation-associated C-reactive protein (CRP),8 latent-transforming growth factor beta-binding protein 1 (LTBP1),9 fibronectin (FN1),31 and matrix metalloproteinase-9 (MMP9),32 all of which were dysregulated during disease progression (Fig. S11†), supporting the reliability of our EV proteomics-based approach in biomarker discovery. Gene ontology (GO) analysis revealed the top 5 significantly upregulated and downregulated biological pathways across the different groups (Fig. 4E and F), from which we observed distinct and shared dysfunctions arising from intestinal lesions. For example, we found consistent and significant upregulation of complement activation, acute-phase response and proteolysis in both HC vs. CRC and polyp vs. CRC (Fig. 4E). Furthermore, we identified certain pathways that were uniquely downregulated in the HC vs. CRC group, such as those governing the localization of proteins or RNAs to telomere or Cajal body (Fig. 4F).
Among our identified indicators, we observed a significant downregulation of NOTCH3 in the CRC group relative to the non-cancer group (Fig. 4G). Intriguingly, the expression pattern of NOTCH3 in tumor tissues exhibited an inverse trend to that observed in normal tissues, as supported by data from The Cancer Genome Atlas database (https://portal.gdc.cancer.gov/) (Fig. 4H). We reasoned that these differences could be attributed to many complex factors. For example, the increased uptake of tumor cells in the cancer tissue might affect the level of plasma-derived EVs. Furthermore, we found a close association between the expression level of NOTCH3 and CRC patient survival, indicating its potential as a prognostic biomarker in CRC (Fig. 4I). Collectively, our analysis provides a comprehensive plasma EV proteome profile of patients with early intestinal malignant transformation and identified hundreds of altered EV proteins that might serve as potential biomarkers to effectively predict disease development at the early stages.
EV biomarker discovery for patients with early CRC
For the purpose of distinguishing pre-malignant polyp and early CRC, we measured the classification performance of each protein using the random forest algorithm and ROC analysis (Fig. S12 and Table S7†), and finally selected a panel of ten protein markers (Fig. 5A). Among the 10 unique proteins (Fig. 5B), apolipoprotein A-IV (APOA4), alpha-1-antichymotrypsin (SERPINA3) and glutathione peroxidase 3 (GPX3) were previously identified as candidate markers for the CRC diagnosis.33–36 Notably, the expression levels of both alpha-synuclein (SNCA) and synaptobrevin homolog YKT6 (YKT6) displayed a progressive decline in association with the exacerbation of intestinal malignant transformation, representing promising CRC marker candidates due to their roles in the regulation of tumor apoptosis and autophagy processes37,38 and disease stage-dependent change in the circulation. Additionally, the GTP-binding nuclear protein Ran (RAN) and the ectonucleotide pyrophosphatase/phosphodiesterase family member 2 (ENPP2) also exhibited significant downregulation in the disease groups compared to healthy controls. Interestingly, these two proteins were previously proposed as predictive biomarkers for CRC progression to hepatic metastasis,39 however, their functional roles and the specific molecular mechanisms contributing to CRC pathogenesis and metastasis remain poorly defined. Both anthrax toxin receptor 1 (ANTXR1) and thrombospondin-4 (THBS4) exhibited obviously decreased abundance during malignant progression from HC to CRC, which seemingly contradicts with existing literature reporting that they play contributing roles in tumor cell attachment and migration.40,41 The remaining protein, secreted phosphoprotein 24 (SPP2), has not been reported to be associated with CRC and could serve as a novel biomarker for early screening for the disease. For SNCA and GPX3, a high degree of correlation was observed between the protein expression level in the plasma-derived EVs and the mRNA transcript level within the cancerous and adjacent non-cancerous regions of tumor tissues (according to The Cancer Genome Atlas database, https://portal.gdc.cancer.gov/), suggesting that the detected differences may originate from the tumors themselves (Fig. S13†). To validate our findings, we selected ANTXR1 as a representative marker for further verification. Western blot analysis was performed on 12 samples across the spectrum of colorectal malignancy. The results demonstrated a gradual downregulation of ANTXR1 in DSPE-bead-enriched EV samples as colorectal malignant transformation progressed (Fig. S14A†). This trend strongly correlated with our MS data (Pearson correlation coefficient r = 0.73) (Fig. S14B†), confirming both the proteomics results and the reliability of our EV enrichment workflow. Intriguingly, analysis of the whole plasma sample from three healthy individuals revealed that only 5 out of 10 identified biomarkers were detectable without EV isolation (Fig. S15†). This result emphasizes the critical role of EV enrichment in uncovering proteins with higher diagnostic value, which might be overlooked in whole plasma analysis.
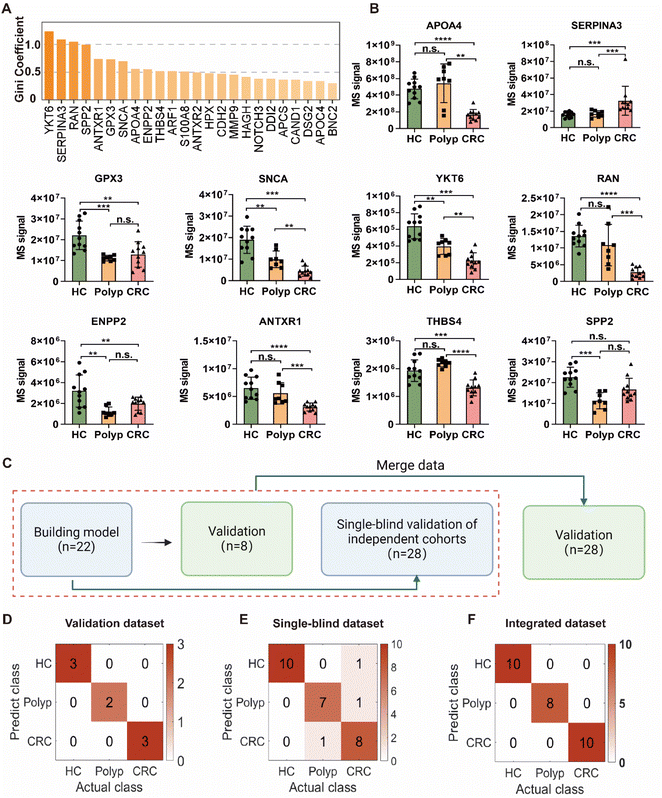 |
| Fig. 5 SVM-based machine learning classification for differentiating HC, polyp, and CRC. (A) The random forest algorithm was employed to prioritize the features based on their Gini coefficient. (B) Distribution of the expression levels of ten protein markers with considerable contribution to classification in different groups. Mann–Whitney U tests were used to define statistical significance (p < 0.01 (**), p < 0.001 (***), p < 0.0001 (****)). (C) Multiple strategies have been employed to assess the generalization capability of our developed model. (D–F) Confusion matrix of the prediction results for the different datasets. Specifically, (D) corresponds to predictions on the cross-validation dataset, (E) represents predictions on a single blind dataset, and (F) illustrates results from cross-validation conducted after integrating both datasets. The number of samples identified is noted in each box. | |
CRC stage classification based on EV biomarkers using machine learning
To facilitate potential clinical application of our findings, we sought to develop a predictive model based on the 10-protein biomarker panel (Fig. 5B). Concerning the machine learning (ML) model investigation, we systematically assessed the performance of several classification algorithms, including Classification Tree, K-Nearest Neighbors (KNN), Random Forest, Naive Bayes, Support Vector Machine (SVM) and Backpropagation Neural Network. We split the entire dataset into the training set (70%) and the validation set (30%), and the model performance was evaluated using both training and validation datasets, with accuracy as the primary metric. Notably, the Naive Bayes, SVM, and Random Forest models achieved 100% accuracy in both training and validation sets (Table S8†). Considering our relatively small sample size, we selected SVM for our final model, as it typically demonstrates more robust performance on smaller datasets. The SVM model demonstrated a perfect classification with 100% accuracy in our internal validation set as illustrated by the confusion matrix (Fig. 5C and D). Additionally, Receiver Operating Characteristic (ROC) analysis revealed a remarkable discriminatory capability of the SVM classifier in distinguishing between HC, polyp, and CRC groups, achieving an Area Under the Curve (AUC) of 1.0 (Fig. S16†).
While these results were highly promising, we recognized the importance of rigorous external validation to assess the true translational potential of our biomarker panel. We performed a single-blind test using an independent cohort, which included another 28 plasma samples consisting of healthy controls (HC, n = 10), polyp (n = 8), and CRC (n = 10) (Table S9† and Fig. 5C). We conducted high-throughput EV proteomic analysis of these samples without the knowledge of their specific disease diagnosis and patient demographic information (“single blindness”), and applied our established machine learning model for predictions. The 10-protein biomarker panel was able to accurately predict 25 out of these 28 cases, achieving an effective accuracy of 89.3% (Fig. 5E). Among them, all HC samples were predicted with 100% accuracy without any misclassification. For the disease (polyp and CRC) groups, our predictive model achieved an accuracy of 94.4% with only one patient misclassified as healthy. Furthermore, our prediction model also exhibited satisfactory performance in distinguishing the polyp and CRC groups. The AUC values from the ROC curves that differentiate polyp from HC, CRC from HC, and CRC from polyp were 1, 0.97 and 0.94, respectively (Fig. S17†). These data demonstrated that the prediction model can be effectively and generally applicable to new patients and achieve desirable accuracy without overfitting.
Owing to the unbiased recording of all precursor and fragment ions, DIA-MS holds a unique advantage of seamlessly integrating datasets from different batches of clinical samples together for retrospective analysis, which may reveal more “previously hidden” biomarkers. To preliminarily explore this direction, we combined these two batches of clinical cohort samples and subjected them to the data processing workflow as described above (Table S10† and Fig. 5C). The results showed that, in comparison to our initial model, the new model established with the integrated data could achieve a perfect classification accuracy (100%) with only seven biomarkers (Fig. S18† and 5F). Projecting forward, we will continue to increase the sample size in future to obtain more sensitive and robust biomarkers.
Conclusions
In this work, we have developed a simplified, fast and efficient EV-based proteomics strategy to enable biomarker discovery for early diagnosis of CRC. By covalent conjugation of an EV-targeting probe, DSPE, to agarose beads, we achieved one-step enrichment of EVs from plasma fluids in 10 minutes without requirement of expensive equipment and reagents, demonstrating its potential and compatibility in clinical applications. Furthermore, to facilitate large-scale clinical screening, the samples were then prepared by the optimized SP3 pipeline and analyzed by DIA-MS for in-depth, robust and reproducible proteomic analysis of plasma-derived EVs. We applied the method to investigate the changes of plasma-derived EV proteomes associated with intestinal pathology, and discovered circulating protein markers of diagnostic value in detection of polyp and early CRC. By employing a feature selection algorithm, we selected a panel of 10 protein markers to construct an ML model, which can effectively predict pre-malignant polyp or early-stage CRC with an excellent diagnostic ability (89.3% accuracy) from an independent and single-blind validation cohort. Inherently, DIA-MS technology holds the advantage of retrospective protein quantification, which allows data integration from different batches or even multi-center studies to reveal more robust biomarkers. Moreover, a well-trained ML model will give automatic feedback that can practically aid bedside diagnosis. We envisioned that the reported simplified EV-based proteomic strategy should be readily applicable to biomarker discovery for other malignant tumorigenesis and diseases.
Data availability
The mass spectrometry proteomics data have been deposited to the ProteomeXchange Consortium (https://proteomecentral.proteomexchange.org) via the iProX partner repository42,43 with the dataset identifier PXD054231.
Author contributions
C. W., J. G., and J. L. designed and supervised the project, J. Z., W. X., N. J. and J. Z. performed the probe synthesis, EV characterization and proteomic experiments, and Z. G., X. J. and D. L. collected and managed the clinical samples. J. Z., Z. G., X. W. and F. Z. analyzed the data, and J. Z. and C. W. wrote the paper with input from all authors.
Conflicts of interest
The authors declare no competing financial interest.
Acknowledgements
We thank the Computing Platform of the Center for Life Science for supporting the proteomic data analysis. We thank Mr Feng Zhang and Dr Yuan Liu for discussion. This work is supported by the National Natural Science Foundation of China (no. 22321005, 21925701 and 92153109), the Natural Science Foundation of Sichuan Province, China (no. 2024YFFK0335) and the National Key Research and Development Projects (no. 2022YFA1304700) to C. W. This work is also supported by the National Natural Science Foundation of China (82274034) to J. L. and National Natural Science Foundation of China (82073223) to J. G. C. W. acknowledges the support by the Joint Laboratory of Medicine and Chemistry at Peking University Thrid Hospital. The graphical abstract image and Fig. 1 were created with https://www.biorender.com/.
Notes and references
- H. Sung, J. Ferlay, R. L. Siegel, M. Laversanne, I. Soerjomataram, A. Jemal and F. Bray, Global Cancer Statistics 2020: GLOBOCAN Estimates of Incidence and Mortality Worldwide for 36 Cancers in 185 Countries, Ca-Cancer J. Clin., 2021, 71, 209–249 CrossRef PubMed.
- R. J. Davies, R. Miller and N. Coleman, Colorectal cancer screening: prospects for molecular stool analysis, Nat. Rev. Cancer, 2005, 5, 199–209 CrossRef CAS PubMed.
- N. Keum and E. Giovannucci, Global burden of colorectal cancer: emerging trends, risk factors and prevention strategies, Nat. Rev. Gastroenterol. Hepatol., 2019, 16, 713–732 CrossRef PubMed.
- A. Shaukat and T. R. Levin, Current and future colorectal cancer screening strategies, Nat. Rev. Gastroenterol. Hepatol., 2022, 19, 521–531 CrossRef PubMed.
- E. J. Kuipers, W. M. Grady, D. Lieberman, T. Seufferlein, J. J. Sung, P. G. Boelens, C. J. van de Velde and T. Watanabe, Colorectal cancer, Nat. Rev. Dis. Prim., 2015, 1, 15065 CrossRef PubMed.
- N. Akimoto, T. Ugai, R. Zhong, T. Hamada, K. Fujiyoshi, M. Giannakis, K. Wu, Y. Cao, K. Ng and S. Ogino, Rising incidence of early-onset colorectal cancer - a call to action, Nat. Rev. Clin. Oncol., 2021, 18, 230–243 CrossRef.
- X. Song, X. Yang, R. Narayanan, V. Shankar, S. Ethiraj, X. Wang, N. Duan, Y. H. Ni, Q. Hu and R. N. Zare, Oral squamous cell carcinoma diagnosed from saliva metabolic profiling, Proc. Natl. Acad. Sci. U. S. A., 2020, 117, 16167–16173 CrossRef CAS.
- A. Loktionov, A. Soubieres, T. Bandaletova, N. Francis, J. Allison, J. Sturt, J. Mathur and A. Poullis, Biomarker measurement in non-invasively sampled colorectal mucus as a novel approach to colorectal cancer detection: screening and triage implications, Br. J. Cancer, 2020, 123, 252–260 CrossRef CAS PubMed.
- J. Robles, L. Pintado-Berninches, I. Boukich, B. Escudero, V. de Los Rios, R. A. Bartolome, M. Jaen, A. Martin-Regalado, M. J. Fernandez-Acenero, J. I. Imbaud and J. I. Casal, A prognostic six-gene expression risk-score derived from proteomic profiling of the metastatic colorectal cancer secretome, J. Pathol. Clin. Res., 2022, 8, 495–508 CrossRef CAS PubMed.
- T. Zhang, K. Yuan, Y. Wang, M. Xu, S. Cai, C. Chen and J. Ma, Identification of Candidate Biomarkers and Prognostic Analysis in Colorectal Cancer Liver Metastases, Front. Oncol., 2021, 11, 652354 CrossRef CAS.
- R. Kalluri and V. S. LeBleu, The biology, function, and biomedical applications of exosomes, Science, 2020, 367, eaau6977 CrossRef CAS.
- G. van Niel, G. D'Angelo and G. Raposo, Shedding light on the cell biology of extracellular vesicles, Nat. Rev. Mol. Cell Biol., 2018, 19, 213–228 CrossRef CAS PubMed.
- B. Zhou, K. Xu, X. Zheng, T. Chen, J. Wang, Y. Song, Y. Shao and S. Zheng, Application of exosomes as liquid biopsy in clinical diagnosis, Signal Transduction Targeted Ther., 2020, 5, 144 CrossRef CAS PubMed.
- G. Szabo and F. Momen-Heravi, Extracellular vesicles in liver disease and potential as biomarkers and therapeutic targets, Nat. Rev. Gastroenterol. Hepatol., 2017, 14, 455–466 CrossRef CAS.
- S. A. Melo, L. B. Luecke, C. Kahlert, A. F. Fernandez, S. T. Gammon, J. Kaye, V. S. LeBleu, E. A. Mittendorf, J. Weitz, N. Rahbari, C. Reissfelder, C. Pilarsky, M. F. Fraga, D. Piwnica-Worms and R. Kalluri, Glypican-1 identifies cancer exosomes and detects early pancreatic cancer, Nature, 2015, 523, 177–182 CrossRef CAS PubMed.
- A. Hoshino, B. Costa-Silva, T. L. Shen, G. Rodrigues, A. Hashimoto, M. Tesic Mark, H. Molina, S. Kohsaka, A. Di Giannatale, S. Ceder, S. Singh, C. Williams, N. Soplop, K. Uryu, L. Pharmer, T. King, L. Bojmar, A. E. Davies, Y. Ararso, T. Zhang, H. Zhang, J. Hernandez, J. M. Weiss, V. D. Dumont-Cole, K. Kramer, L. H. Wexler, A. Narendran, G. K. Schwartz, J. H. Healey, P. Sandstrom, K. J. Labori, E. H. Kure, P. M. Grandgenett, M. A. Hollingsworth, M. de Sousa, S. Kaur, M. Jain, K. Mallya, S. K. Batra, W. R. Jarnagin, M. S. Brady, O. Fodstad, V. Muller, K. Pantel, A. J. Minn, M. J. Bissell, B. A. Garcia, Y. Kang, V. K. Rajasekhar, C. M. Ghajar, I. Matei, H. Peinado, J. Bromberg and D. Lyden, Tumour exosome integrins determine organotropic metastasis, Nature, 2015, 527, 329–335 CrossRef CAS PubMed.
- X. Zheng, K. Xu, B. Zhou, T. Chen, Y. Huang, Q. Li, F. Wen, W. Ge, J. Wang, S. Yu, L. Sun, L. Zhu, W. Liu, H. Gao, L. Yue, X. Cai, Q. Zhang, G. Ruan, T. Zhu, Z. Wu, Y. Zhu, Y. Shao, T. Guo and S. Zheng, A circulating extracellular vesicles-based novel screening tool for colorectal cancer revealed by shotgun and data-independent acquisition mass spectrometry, J. Extracell. Vesicles, 2020, 9, 1750202 CrossRef CAS PubMed.
- Q. Niu, Y. Shu, Y. Chen, Z. Huang, Z. Yao, X. Chen, F. Lin, J. Feng, C. Huang, H. Wang, H. Ding, C. Yang and L. Wu, A Fluid Multivalent Magnetic Interface for High-Performance Isolation and Proteomic Profiling of Tumor-Derived Extracellular Vesicles, Angew Chem. Int. Ed. Engl., 2023, 62, e202215337 CrossRef CAS PubMed.
- A. Hoshino, H. S. Kim, L. Bojmar, K. E. Gyan, M. Cioffi, J. Hernandez, C. P. Zambirinis, G. Rodrigues, H. Molina, S. Heissel, M. T. Mark, L. Steiner, A. Benito-Martin, S. Lucotti, A. Di Giannatale, K. Offer, M. Nakajima, C. Williams, L. Nogues, F. A. Pelissier Vatter, A. Hashimoto, A. E. Davies, D. Freitas, C. M. Kenific, Y. Ararso, W. Buehring, P. Lauritzen, Y. Ogitani, K. Sugiura, N. Takahashi, M. Aleckovic, K. A. Bailey, J. S. Jolissant, H. Wang, A. Harris, L. M. Schaeffer, G. Garcia-Santos, Z. Posner, V. P. Balachandran, Y. Khakoo, G. P. Raju, A. Scherz, I. Sagi, R. Scherz-Shouval, Y. Yarden, M. Oren, M. Malladi, M. Petriccione, K. C. De Braganca, M. Donzelli, C. Fischer, S. Vitolano, G. P. Wright, L. Ganshaw, M. Marrano, A. Ahmed, J. DeStefano, E. Danzer, M. H. A. Roehrl, N. J. Lacayo, T. C. Vincent, M. R. Weiser, M. S. Brady, P. A. Meyers, L. H. Wexler, S. R. Ambati, A. J. Chou, E. K. Slotkin, S. Modak, S. S. Roberts, E. M. Basu, D. Diolaiti, B. A. Krantz, F. Cardoso, A. L. Simpson, M. Berger, C. M. Rudin, D. M. Simeone, M. Jain, C. M. Ghajar, S. K. Batra, B. Z. Stanger, J. Bui, K. A. Brown, V. K. Rajasekhar, J. H. Healey, M. de Sousa, K. Kramer, S. Sheth, J. Baisch, V. Pascual, T. E. Heaton, M. P. La Quaglia, D. J. Pisapia, R. Schwartz, H. Zhang, Y. Liu, A. Shukla, L. Blavier, Y. A. DeClerck, M. LaBarge, M. J. Bissell, T. C. Caffrey, P. M. Grandgenett, M. A. Hollingsworth, J. Bromberg, B. Costa-Silva, H. Peinado, Y. Kang, B. A. Garcia, E. M. O'Reilly, D. Kelsen, T. M. Trippett, D. R. Jones, I. R. Matei, W. R. Jarnagin and D. Lyden, Extracellular Vesicle and Particle Biomarkers Define Multiple Human Cancers, Cell, 2020, 182, 1044–1061 CrossRef CAS PubMed.
- F. Cocozza, E. Grisard, L. Martin-Jaular, M. Mathieu and C. Thery, SnapShot: Extracellular Vesicles, Cell, 2020, 182, 262 CrossRef CAS PubMed.
- F. G. Kugeratski, K. Hodge, S. Lilla, K. M. McAndrews, X. Zhou, R. F. Hwang, S. Zanivan and R. Kalluri, Quantitative proteomics identifies the core proteome of exosomes with syntenin-1 as the highest abundant protein and a putative universal biomarker, Nat. Cell Biol., 2021, 23, 631–641 CrossRef CAS PubMed.
- X. Feng, A. Iliuk, X. Zhang, S. Jia, A. Shen, W. Zhang, L. Hu and W. A. Tao, Supramolecular Exosome Array for Efficient Capture and In Situ Detection of Protein Biomarkers, Anal. Chem., 2023, 95, 2812–2821 CrossRef CAS PubMed.
- J. Sun, Q. Li, Y. Ding, D. Wei, M. Hadisurya, Z. Luo, Z. Gu, B. Chen and W. A. Tao, Profiling Phosphoproteome Landscape in Circulating Extracellular Vesicles from Microliters of Biofluids through Functionally Tunable Paramagnetic Separation, Angew Chem. Int. Ed. Engl., 2023, 62, e202305668 CrossRef CAS PubMed.
- Y. Wan, G. Cheng, X. Liu, S. J. Hao, M. Nisic, C. D. Zhu, Y. Q. Xia, W. Q. Li, Z. G. Wang, W. L. Zhang, S. J. Rice, A. Sebastian, I. Albert, C. P. Belani and S. Y. Zheng, Rapid magnetic isolation of extracellular vesicles via lipid-based nanoprobes, Nat. Biomed. Eng., 2017, 1, 0058 CrossRef CAS PubMed.
- L. C. Gillet, P. Navarro, S. Tate, H. Rost, N. Selevsek, L. Reiter, R. Bonner and R. Aebersold, Targeted data extraction of the MS/MS spectra generated by data-independent acquisition: a new concept for consistent and accurate proteome analysis, Mol. Cell. Proteomics, 2012, 11, O111.016717 CrossRef PubMed.
- O. Karayel, S. Virreira Winter, S. Padmanabhan, Y. I. Kuras, D. T. Vu, I. Tuncali, K. Merchant, A. M. Wills, C. R. Scherzer and M. Mann, Proteome profiling of cerebrospinal fluid reveals biomarker candidates for Parkinson's disease, Cell Rep. Med., 2022, 3, 100661 CrossRef CAS PubMed.
- L. Niu, M. Thiele, P. E. Geyer, D. N. Rasmussen, H. E. Webel, A. Santos, R. Gupta, F. Meier, M. Strauss, M. Kjaergaard, K. Lindvig, S. Jacobsen, S. Rasmussen, T. Hansen, A. Krag and M. Mann, Noninvasive proteomic biomarkers for alcohol-related liver disease, Nat. Med., 2022, 28, 1277–1287 CrossRef CAS PubMed.
- J. R. Wiśniewski, A. Zougman, N. Nagaraj and M. Mann, Universal sample preparation method for proteome analysis, Nat. Methods, 2009, 6, 359–362 CrossRef PubMed.
- C. S. Hughes, S. Moggridge, T. Muller, P. H. Sorensen, G. B. Morin and J. Krijgsveld, Single-pot, solid-phase-enhanced sample preparation for proteomics experiments, Nat. Protoc., 2019, 14, 68–85 CrossRef CAS PubMed.
- V. Demichev, C. B. Messner, S. I. Vernardis, K. S. Lilley and M. Ralser, DIA-NN: neural networks and interference correction enable deep proteome coverage in high throughput, Nat. Methods, 2019, 17, 41–44 CrossRef PubMed.
- J. Chantaraamporn, V. Champattanachai, A. Khongmanee, C. Verathamjamras, N. Prasongsook, K. Mingkwan, V. Luevisadpibul, S. Chutipongtanate and J. Svasti, Glycoproteomic Analysis Reveals Aberrant Expression of Complement C9 and Fibronectin in the Plasma of Patients with Colorectal Cancer, Proteomes, 2020, 8, 26 CrossRef CAS PubMed.
- N. V. Yunusova, E. A. Zambalova, M. R. Patysheva, E. S. Kolegova, S. G. Afanas'ev, O. V. Cheremisina, A. E. Grigor'eva, S. N. Tamkovich and I. V. Kondakova, Exosomal Protease Cargo as Prognostic Biomarker in Colorectal Cancer, Asian Pac. J. Cancer Prev. APJCP, 2021, 22, 861–869 CrossRef CAS PubMed.
- J. Barbazan, Y. Dunkel, H. Li, U. Nitsche, K. P. Janssen, K. Messer and P. Ghosh, Prognostic Impact of Modulators of G proteins in Circulating Tumor Cells from Patients with Metastatic Colorectal Cancer, Sci. Rep., 2016, 6, 22112 CrossRef CAS PubMed.
- L. Niu, C. Gao and Y. Li, Identification of potential core genes in colorectal carcinoma and key genes in colorectal cancer liver metastasis using bioinformatics analysis, Sci. Rep., 2021, 11, 23938 CrossRef CAS PubMed.
- S. Bosch, A. Acharjee, M. N. Quraishi, I. V. Bijnsdorp, P. Rojas, A. Bakkali, E. E. Jansen, P. Stokkers, J. Kuijvenhoven, T. V. Pham, A. D. Beggs, C. R. Jimenez, E. A. Struys, G. V. Gkoutos, T. G. de Meij and N. K. de Boer, Integration of stool microbiota, proteome and amino acid profiles to discriminate patients with adenomas and colorectal cancer, Gut Microb., 2022, 14, 2139979 CrossRef PubMed.
- V. Voronova, P. Glybochko, A. Svistunov, V. Fomin, P. Kopylov, P. Tzarkov, A. Egorov, E. Gitel, A. Ragimov, A. Boroda, E. Poddubskaya and M. Sekacheva, Diagnostic Value of Combinatorial Markers in Colorectal Carcinoma, Front. Oncol., 2020, 10, 832 CrossRef PubMed.
- Y. X. Li, Z. W. Yu, T. Jiang, L. W. Shao, Y. Liu, N. Li, Y. F. Wu, C. Zheng, X. Y. Wu, M. Zhang, D. F. Zheng, X. L. Qi, M. Ding, J. Zhang and Q. Chang, SNCA, a novel biomarker for Group 4 medulloblastomas, can inhibit tumor invasion and induce apoptosis, Cancer Sci., 2018, 109, 1263–1275 CrossRef CAS PubMed.
- K. McGrath, S. Agarwal, M. Tonelli, M. Dergai, A. L. Gaeta, A. K. Shum, J. Lacoste, Y. Zhang, W. Wen, D. Chung, G. Wiersum, A. Shevade, S. Zaichick, D. B. van Rossum, L. Shuvalova, J. N. Savas, S. Kuchin, M. Taipale, K. A. Caldwell, G. A. Caldwell, D. Fasshauer and G. Caraveo, A conformational switch driven by phosphorylation regulates the activity of the evolutionarily conserved SNARE Ykt6, Proc. Natl. Acad. Sci. U. S. A., 2021, 118, e2016730118 CrossRef CAS PubMed.
- Y.-D. Miao, W.-X. Quan, X. Dong, J. Gan, C.-F. Ji, J.-T. Wang and F. Zhang, Prognosis-related metabolic genes in the development of colorectal cancer progress and perspective, Gene, 2023, 862, 147263 CrossRef CAS.
- F. Feng, B. Cheng, B. Cheng, Y. Jia, M. Zhang and F. Xu, ANTXR1 as a potential sensor of extracellular mechanical cues, Acta Biomater., 2023, 158, 80–86 CrossRef CAS PubMed.
- M. S. Kim, H. S. Choi, M. Wu, J. Myung, E. J. Kim, Y. S. Kim, S. Ro, S. E. Ha, A. Bartlett, L. Wei, H. S. Ryu, S. C. Choi, W. C. Park, K. Y. Kim and M. Y. Lee, Potential Role of PDGFRbeta-Associated THBS4 in Colorectal Cancer Development, Cancers, 2020, 12, 2533 CrossRef CAS.
- J. Ma, T. Chen, S. Wu, C. Yang, M. Bai, K. Shu, K. Li, G. Zhang, Z. Jin, F. He, H. Hermjakob and Y. Zhu, iProX: an integrated proteome resource, Nucleic Acids Res., 2018, 47, D1211–D1217 CrossRef.
- T. Chen, J. Ma, Y. Liu, Z. Chen, N. Xiao, Y. Lu, Y. Fu, C. Yang, M. Li, S. Wu, X. Wang, D. Li, F. He, H. Hermjakob and Y. Zhu, iProX in 2021: connecting proteomics data sharing with big data, Nucleic Acids Res., 2021, 50, D1522–D1527 CrossRef PubMed.
|
This journal is © The Royal Society of Chemistry 2024 |
Click here to see how this site uses Cookies. View our privacy policy here.