Optical properties and molecular differences in dissolved organic matter at the Bermuda Atlantic and Hawai'i ALOHA time-series stations†
Received
27th November 2023
, Accepted 26th March 2024
First published on 26th March 2024
Abstract
Optically active components, namely marine chromophoric DOM (CDOM) and fluorescent DOM (FDOM), have been used as proxies for refractory DOM (RDOM) in the world's oceans, and numerous studies using ultrahigh resolution mass spectrometry (HRMS) approaches have supplied a tremendous amount of data on the chemical complexity and diversity of DOM. Here, we collected and analyzed high-resolution depth profiles of DOM throughout the water column in the North Atlantic Gyre at the Bermuda Atlantic Time-series Study (BATS) station in August 2019 and in the North Pacific Gyre at station ALOHA (A Long-term Oligotrophic Habitat Assessment) used by the Hawaii Ocean Time-series (HOT) in July 2021. Water samples were collected at 200 m depth intervals from 4530 m at BATS and 4700 m at ALOHA up to the surface and DOM was isolated by solid-phase extraction (SPE). Parallel factor analysis modeled EEM fluorescence revealed changes of “humic-like” and “protein-like” FDOM (FDOMH and FDOMP, respectively) in SPE-DOM throughout the water column with higher fluorescence intensities present at ALOHA. Dissolved organic phosphorous (DOP) and dissolved organic sulfur (DOS) concentrations were always higher in SPE-DOM at BATS than at ALOHA, except for DOP in the surface at ALOHA. Negative mode electrospray ionization (ESI) Fourier transform ion cyclotron resonance mass spectrometry (FT-ICR MS) data also revealed fundamental differences between BATS and ALOHA. A novel machine learning algorithm (SOFAR) was implemented and revealed much higher overall oxygen to carbon (O/C) ratio molecular signatures at BATS as the major difference and also much more DOS signatures at BATS when compared to ALOHA. Furthermore, we extracted for the first-time weak anion exchange (WAX) amendable DOM, and the results also showed drastic differences between the two stations. The optical and FT-ICR MS data, converged and supported the idea that DOM in the Atlantic and Pacific basins are fundamentally different when looked at through these analytical windows, at least at these long-term monitoring stations. This finding suggests that the marine DOM is likely turning over at different rates at ALOHA versus BATS and that geographical differences in DOM composition are likely a compounding factor in DOM reactivity.
Environmental significance
Marine DOM is a major global element reservoir but remains enigmatic and poorly understood. Linking sources and deciphering the molecular composition of DOM constituents is desperately needed in order to better predict overall DOM reactivity and better define the substantial role it plays within global elemental cycles. This study showed in detail fundamental differences in DOM composition form the two longest running oceanic time series stations, one located close to Bermuda in the western Atlantic, and one near the Hawaiian Islands in the central Pacific Ocean. We used numerous complementary analytical techniques to shed light on multiple aspects of the nature of the observed fundamental DOM differences. We combined optical properties, ultrahigh resolution mass spectrometry, and elemental analysis using triple quadrupole ICP-MS. We also implemented two fundamentally different DOM isolation techniques. The data from all these analytical windows paint a clear picture of fundamental differences in DOM composition, which is a critical piece of information for better understanding DOM composition, its sources and also its turnover.
|
Introduction
The question still stands:1 “Why marine dissolved organics matter?”.1 This material makes up about 662 Pg carbon in the world's oceans,2 and small additional annual mineralization would likely produce carbon dioxide on the order of the entire annual carbon dioxide produced by anthropogenic sources.
Despite the significance of this carbon pool, we know relatively little about the dynamics of dissolved organic matter (DOM) decomposition, and specifically of the refractory DOM (RDOM) pool, which turns over on longer, but unknown timescales. For example, the chemical composition, molecular structures, and age of marine DOM are still hotly debated. Although the bulk age of DOM in the deep ocean is ∼4500–6000 yBP, its turnover time could be more rapid with the input of pre-aged sources of dissolved organic carbon (DOC) to the deep ocean.3 A recent study of the radiocarbon age of marine DOM in the Indian Ocean concluded that there may be significant input of young DOC from dissolution of surface-derived POC to the deep ocean which is also reflected in the presence of a large oxygen deficiency zone (ODZ).4 Furthermore, evidence has been given that hydrothermal systems may influence dissolved radiocarbon measurements.5,6
New approaches/techniques are critically needed to better resolve basin scale differences in the DOM turnover and the reactivity of the ill-defined RDOM pool. Emerging studies suggest that the assumption of a 2-endmember model for DOC cycling may be too simplistic given potential subsidies of aged DOM to the deep ocean. 14C-free sources have been identified in recent years, and the incorporation of radiocarbon-free organic matter into DOM has also been described.5–9 Consequently, 14C measurements of marine bulk DOM cannot be directly used to infer the residence time of DOM in the ocean, and it remains feasible that a portion of deep ocean marine DOM may be younger than the age of the bulk DOM.3,4,9–11 However, we currently lack the methods to reliably quantify the degradation rates of individual DOM molecules in the deep ocean. In addition, the absence of direct comparisons of DOM composition, with the same analytical methodologies, between deep waters of different oceanic areas restricts our capacity to make further progress.
Ideally multiple independent diagnostic tools with discrete analytical windows should be used to characterize DOM as the convergence of data becomes substantially more powerful to resolve the overall appearance and composition of marine DOM. Optical property analysis paired with high resolution mass spectrometry (HRMS) has become valuable in this light, because they are based on fundamentally different analytical principles. The analysis of ultraviolet-visible (UV-vis) absorbance paired with fluorescence measurements gained popularity in recent decades.12–15 Multiple parameters were developed using UV-vis absorbance data alone. Specific parameters derived from marine chromophoric dissolved organic matter (CDOM) UV-vis spectroscopy are spectral slopes (S) and slope ratios, which have been used to provide insight into the chemical composition of DOM.12 The ratio of absorption at 250 to 365 nm (E2/E3) has been used to track changes in the relative size of DOM molecules.16,17 Fluorescent DOM (FDOM), and specifically excitation emission matrix (EEM) fluorescence spectroscopy, is another tool to describe marine DOM based on its optical properties. Several fluorescent regions within the contour plots of EEM data have been previously described using additional tools such as parallel factor analysis (PARAFAC) modelling.18,19 EEM PARAFAC components and their distributions are documented in the oceans15,20–24 and have been used as proxies for the refractory dissolved organic carbon (RDOC) pool.
HRMS approaches alone may provide a tremendous amount of data on the chemical complexity and diversity of DOM with typically more than ten thousand molecular signatures identified in a single HRMS spectrum. However, these semi-quantitative approaches have been challenging due to the requirement to run all comparative samples concurrently to establish adequate reproducibility, avoid sample carryover and analyse hundreds of samples efficiently. Additionally, sample preparation, including severe desalting, is necessary prior to direct infusion HRMS. Solid-phase extraction has been the primary choice for desalting, and specifically, the usage of polymeric resins such as the proprietary polystyrene vinyl resin sold as Agilent Bond Elut PPL has become most popular in aquatic sciences. The use of alternative resins based on fundamentally different adsorption mechanisms has been rare, but one promising candidate is the weak anion exchange (WAX) resin, which is compatible with acidified samples, and could be used sequentially with other polymeric resins to shed light on this highly specific weak anion exchange DOM (WAX-DOM) fraction.
Non-targeted HRMS data have suggested that marine DOM looks remarkably similar across ocean basins. The reasoning for this observation is likely caused by the extreme complexity and the large coverage of possible hydrogen to carbon (H/C) and oxygen to carbon (O/C) ratios in a solubility framework often described in van Krevelen space. Despite these challenges, previous studies employing HRMS have been able to discern compositional differences throughout the water column and along oceanic transects25–30 suggesting that semi-quantitative approaches using direct-infusion HRMS are feasible, despite the relatively low precision in intensities of individual m/z ions when spectra are compared. Overall small observed and consistent differences between samples would require a substantial change in composition within the observed DOM. To better understand the processing and distribution of DOM in the North Atlantic and North Pacific Oceans, we investigated depth-related changes of DOM, CDOM, and FDOM using optical properties and FT-ICR MS analysis on solid-phase extracted DOM. Temperature, depth, salinity, oxygen, and chlorophyll fluorescence were also measured using a conductivity, temperature, depth (CTD) profiler aboard the research vessels.
The Bermuda Atlantic Time-series Study (BATS) station in the Western Atlantic and station ALOHA (A Long-term Oligotrophic Habitat Assessment) in the Central Pacific Ocean were chosen due to a wealth of available historic data as well as their comparable geographical latitudes, but are located in the Atlantic and Pacific Oceans, respectively. BATS was established as a long-term biogeochemical time series study in 1988 and is located south-east of Bermuda within the Sargasso Sea. Station ALOHA is located north of Hawai'i and was also established in 1988 to start the long-term oligotrophic habitat assessment within the Hawai'i Ocean Time-series (HOT).
The focus of this study was to illuminate potential differences, using independent analytical tools, in the composition of marine DOM between the long-term monitoring stations of BATS and ALOHA, which would be a prerequisite to infer differences in the reactivity of marine DOM, depending on locale and water depth.
Materials and methods
Sample collection
Two research cruises were undertaken, and DOM samples were collected from two long-term time-series stations: Bermuda Atlantic Time-series Study (BATS) station and Hawai'i Ocean Time-series Station ALOHA in August 2019 and July 2021, respectively. Both sampling stations are located within major ocean gyres and are readily accessible. Furthermore, a tremendous amount of historical data exists for both stations. However, they may not be representative of the entire North Atlantic or North Pacific Gyres, respectively. For example, BATS is in the northwest of the North Atlantic Gyre and is influenced by eddies of the Gulf Stream and experiences a strong seasonal cycle due to inputs of nutrients during winter mixing and the formation of a shallow seasonal thermocline and mixed layer during the summer. ALOHA is known to have relatively high primary production relative to the overall North Pacific Gyre, but a much weaker seasonal signal relative to BATS and strong vertical gradients. However, both stations have ocean-specific representative DOC concentrations in the deep ocean of 38 μM C kg−1 at ALOHA and 42 μM C kg−1 at BATS. The DOC depth profiles at BATS and ALOHA, compiled from historic data, are provided in ESI Online Materials (Fig. S1†). Aboard both cruises, 10 L samples were collected in five-gallon polycarbonate drinking water containers after rinsing three times using seawater samples. Bottles were marked using a measured volume of 10 L for volume reference. The samples were then acidified with pure grade 32% hydrochloric acid from Acros Organics to a pH of 2 prior to filtration and solid-phase extraction (SPE).
R/V Atlantic Explorer 2019 – Atlantic sample collection.
BATS is located at the coordinates 31°40′N and 64°10′W, approximately eighty-two kilometers southeast of Bermuda's island of St. George. BATS has been in operation since 1988 and is often used to represent the western North Atlantic subtropical gyre and is located within the Sargasso Sea. Water samples were collected from aboard the RV Atlantic Explorer in August 2019. For each sample depth at 200 m depth intervals from the surface to the seafloor at 4530 m using the Niskin sampler, 10 L water samples were collected. In total, three full depth profiles were obtained to be able to have true field replicate samples. A CTD profiler was attached to the rosette holding the Niskin sampling bottles and the attached sensors measured depth (m), temperature (°C), oxygen and chlorophyll a fluorescence.
R/V Kilo Moana 2021 – Pacific sample collection.
The R/V Kilo Moana was used in July of 2021 for a research cruise to the Long-Term Oligotrophic Habitat Assessment (ALOHA) station. ALOHA is located at around a 10 kilometer radius circle centered at 22 45′N and 158 W, approximately 100 kilometers north of O'ahu of the Hawai'ian Islands. The Hawaii Ocean Time Series (HOT) study has been in operation since 1988 for oceanographic research and data which is considered to represent the oligotrophic North Pacific Ocean. Three full profiles collecting 10 L water samples were obtained at 200 m depth intervals from the surface to just above the seafloor at 4730 m using Niskin water collection bottles for a total of seventy-eight samples. A similar CTD profiler attached to the water sampling rosette was used as described for BATS.
Solid phase extraction (SPE)
DOM samples were isolated through two fundamentally different types of solid phase extraction procedures. The collected 10 L water samples were in-line filtered through pre-ashed 47 mm and 0.7 μm Whatman GF/F glass microfiber filters. Solid-phase extraction was conducted sequentially using 1 g of Agilent Bond Elut PPL in 6 mL cartridges and 500 mg of Waters Oasis WAX resin also in 6 mL cartridges. Both methanol (MeOH) and 0.1% (v/v) formic acid (FA) water used for SPE in the field were of LC/MS grade, and the ultrapure water (RO water) used for rinsing in the field was from systems maintained aboard the research vessels. Details about each extraction are given below.
Extraction procedure using the proprietary polystyrene vinyl resin (PPL).
PPL SPE is commonly used for the relatively high DOC extraction efficiency of about 40–50% of oceanic DOM, and PPL can retain more polar compounds than other commonly used reverse phase resins. However, small organic acids and highly polar compounds, including polyols and saccharides, are still not retained using this technique. Agilent Bond Elut 1 g PPL cartridges were first activated with 10 mL MeOH, rinsed with 5 mL 0.1% FA water, and then loaded via lines running through pre-ashed 0.7 μm glass microfiber filters by gravity or a peristaltic pump as necessary at a flow rate of less than 10 mL min−1. After sample loading, the cartridge exterior was rinsed with ultrapure water and the sorbent was thoroughly rinsed with 20 mL 0.1% FA water to completely remove salts. Cartridges were then dried, and eluted with 10 mL MeOH into new, trace contaminant certified or acid-washed and combusted 40 mL VOA glass vials. The methanolic SPE extracts of DOM (SPE-DOM) were stored in the dark at −20 °C prior to further analyses. We determined DOC concentrations for extracts reconstituted in Milli-Q water for samples collected at 1000 m. Assuming bulk seawater has a DOC concentration of 38 μM at this depth, the DOC extraction efficiency averaged 49.8 ± 0.6% (n = 3). We also extracted 10 L Milli-Q blank samples and prepared these extracts in the same manner as seawater samples. Negligible DOC was found in Milli-Q blank extracts prepared in the same manner, confirming that the FA washing step did not add significant DOC to our extracts.
Extraction procedure using weak anion exchange resin (WAX).
WAX cartridges were attached in sequence to each PPL cartridge in the first profile at each site to recover additional DOM constituents that were not extractable by the PPL resin. This is the first time that this sequential method has been used in a high-resolution marine-DOM profile study, and this approach has the potential to extend the molecular level characterization to this highly specific weak anion exchange amendable fraction of marine DOM. Water Oasis WAX 500 mg cartridges were first activated with 10 mL 0.1% ammonium hydroxide MeOH, rinsed with 5 mL 0.1% FA water, and then loaded via lines running through pre-ashed 0.7 μm glass microfiber filters by gravity or a peristaltic pump as necessary at a flow rate of less than 10 mL min−1. After sample loading, the cartridge exterior was rinsed with ultrapure water and the sorbent was thoroughly rinsed with 20 mL 0.1% FA water to completely remove salts. Cartridges were then dried and eluted with 10 mL 0.1% ammonium hydroxide MeOH into certified or acid-washed and combusted 40 mL VOA glass vials. The ammonium hydroxide/methanolic SPE extracts of DOM (SPE-DOM) were stored in the dark at −20 °C prior to further analyses. Milli-Q blank WAX extracts had relatively high DOC concentrations post extraction, likely due to the FA washing step and the fact that WAX can retain some strong organic acids. We also tested DOC concentrations in 1000 m WAX extract reconstituted in water, and after blank correction, determined a DOC extraction efficiency of 4.4%.
Characterization of optical properties
The samples of the methanolic SPE-PPL extracts were re-dissolved in 5 mL of ultrapure water for optical property analysis after drying 0.5 mL of the methanolic SPE-DOM sample using ultrahigh purity nitrogen. UV-vis absorbance spectra and fluorescence EEMs were measured over a 10 s integration time in an excitation wavelength range of 250–700 nm and emission wavelength range of 240–600 nm at 3 nm intervals using a Horiba Aqualog spectrofluorometer, which is capable of measuring UV-vis absorbance and EEMs simultaneously. All absorbance and fluorescence spectra were blank corrected using scans of ultrapure water. Absorbance spectra (A(λ)) were converted to Napierian absorption coefficients (a(λ)) using the equation a(λ) = 2.303 × A(λ)/L, where L is the pathlength of the cuvette in m (0.01 m), and A is the raw measured absorbance.31 The concentration factor of the re-dissolved sample versus the 10 L extraction was one hundred and therefore the absorption coefficients and EEM data were divided by this factor to account for the concentration during SPE. Rayleigh scattering and inner filter effects were corrected using the Aqualog software and custom-designed post-processing scripts programmed in MATLAB. Spectra were normalized using the Raman scattering of pure water and are therefore reported in water Raman units (RU). SPE-DOM EEMs were analyzed using statistical PARAFAC modeling using the MATLAB DrEEM toolbox.18 A 4-component model was generated to explain the data well, which was split-half validated using four random splits. Unit normalization was reversed to recover true scores (intensities) of the components. The maximum fluorescence (Fmax) values of components were summed for each sample and each Fmax value was calculated to explain representation by these statistical components.
Ultrahigh resolution mass spectrometry
All SPE DOM samples, including all replicates, from BATS and ALOHA were diluted 1
:
20 with pure methanol and then directly injected using an autosampler with multiple wash steps between samples. The flow rate was 2 μL min−1 and 500 scans were averaged at 4 megaword using a 12 Tesla Solarix Fourier transform ion cyclotron resonance mass spectrometer (FT-ICR MS). The samples were ionized in negative mode electrospray ionization (ESI) with an applied voltage of 3600 volts. Initial calibration was carried out using known m/z signatures of arginine clusters. Post-calibration was undertaken using known and previously identified unambiguous molecular signatures of marine DOM. The mass accuracy was always better than 200 ppb and unambiguous molecular formula assignments were calculated using a previously established and proprietary network approach32 using atoms of 12C1–∞, 1H1–∞, 16O1–∞, 14N0–10, and 32S0–2. The autosampler approach, severe cleaning between samples to avoid carryover, as well as running all samples within a week using the same method to minimize instrument drifts allowed the direct comparison of a large data set from BATS and ALOHA. We also want the reader to keep in mind that FT-ICR MS will always only address a portion of the DOM pool. It is well established that Ft-ICR MS is biased towards the strongest ionizers and that weak ionizers are likely going in and out of the baseline, which also complicates presence and absence analysis. For this work, we wanted to emphasize differences in ions found in all samples, especially to highlight that differences can even be found in the so called “island of stability.”
Calculations and statistical analyses
Apparent oxygen utilization (AOU) was calculated using the equation AOU = O′2 − O2 by subtracting the measured in situ oxygen concentration (O2) from the theoretical oxygen saturation (O′2) at any given temperature and pressure (depth). Datasets were fitted with linear regressions using Microsoft Excel. Spectral slopes S (275–295 nm), S (350–400 nm), slope ratios (SR) (S (275–295 nm)/S (350–400 nm)) and absorption ratios E2/E3 (a (250)/a (365))were calculated using the reference wavelength, and a nonlinear least–squares curve fitting procedure was applied to the absorption spectrum exponential model.12 Hierarchical clustering of log transformed and centered FT-ICR MS data was undertaken using the freeware Cluster 3.0 and Python Treeview. Additionally, a novel sparse orthogonal factor regression (SOFAR) machine learning method33 was also implemented for the first time using FT-ICR MS and EEM-PARAFAC data. The algorithm works by modifying the standard multivariate regression model and estimating a sparse singular value decomposition of the regression coefficients, ensuring low rank solutions and sparsity:
where Y is the response matrix, X is the predictor matrix, C is the coefficient matrix, and E is the error matrix. SOFAR uses singular value decomposition (SVD) to break up the coefficient matrix, C, into three matrices each with unique properties:
D is a diagonal matrix of nonzero singular values and U and VT are sparse, orthonormal matrices of singular vectors. Through a penalty, the coefficient matrix C is constrained to be of low rank. The algorithm is available in the open-source R package ‘rrpack’.34
SOFAR was run on the entire FT-ICR MS dataset only using molecular ions that were present in all samples (combined dataset from BATS and ALOHA), which ensured that all entries in the matrix were non-zero. This reduced the FT-ICR MS matrix from 6149 to 2201 columns, increasing accuracy of the SOFAR model and focusing on the most relevant molecular signatures that appear to be ubiquitous in marine DOM. The Ft-ICR MS matrix was normalized by a natural logarithm transformation, centered by the column means, and scaled by the column inter-quantile range. Normalization was conducted in order to reduce bias in favor of m/z ions with larger values-effectively placing every molecular signature on the same level of importance to the algorithm. We applied SOFAR to two unique problems, (1) bi-clustering of the FT-ICR MS dataset alone and (2) an association network between FT-ICR MS and the EEM-PARAFAC fluorescence datasets.
In the association network problem, the FT-ICR MS dataset is the predictor matrix, X, while the fluorescence dataset is the response matrix, Y. SOFAR assumes that three latent associations exist between the predictor variables and the response variables. This assumption enables dimension reduction and grouping of molecular signatures based on their connections with the fluorescence dataset.
Dissolved organic sulfur (DOS) and dissolved organic phosphorus (DOP) analysis
The samples of the methanolic SPE-PPL extracts were dried under high purity N2 (as above for optical property analyses) and diluted 100× using ultrapure Milli-Q water. DOS and DOP concentrations were then determined for these SPE-PDOM samples using a triple quadrupole inductively coupled plasma-mass spectrometer (ICP-MS/MS, Agilent 8900 ICP-QQQ), following a procedure similar to that in previous work.35 Briefly, to improve both S and P detection and to minimize interferences, oxygen gas was reacted with both sulfur and phosphorus to form 32S16O and 31P16O, respectively, in the collision/reaction cell. S was determined using m/z 32 (MS1) and m/z 48 (MS2) and P was determined using m/z 31 (MS1) and m/z 47 (MS2) with an integration time of 0.5 ms, 1 point per peak, 3 replicates and 50 sweeps per replicate. The ICP was tuned weekly using a multi-element tuning (Agilent) diluted to 1 μg L−1 with pure water, and 59Co (Agilent) was used as an internal standard in all samples, blanks, and standards at a final concentration of 0.5 μg L−1. S and P standard curves were prepared from 0 to 50 ppb using dilutions of a 10 ppm multi-element calibration mix (Agilent). Reported SPE-DOS and SPE-DOP concentrations were converted to molar concentrations using 30.974 g P mol−1 and 32.066 g S mol−1 and scaled for extraction volume and dilution.
Results and discussion
Ship-based measurements
Depth profile CTD measurements from BATS and ALOHA are given in ESI Online Material (Fig. S1†). Temperature measurements were found to be higher at the BATS surface before decreasing more gradually compared to the sharp decrease at ALOHA. Salinity at BATS was overall higher than at ALOHA. At BATS, a slight oxygen minimum (OM) occurred at 785 m and it only reached 132.20 μmol kg−1 whereas the OM occurred at 670 m at ALOHA and it reached a low value of 26.92 μmol kg−1 (Fig. S1†). The minimum oxygen saturation at BATS was 49.34% and at ALOHA it was only 8.72%. Below the OM, values increased again as one would expect below the ODZ. However, the oxygen levels at ALOHA gradually increased with depth whereas at BATS, they increased more rapidly and remained steady at higher levels before slightly decreasing near the bottom. The pronounced ODZ at ALOHA may suggest a much higher microbial respiration and lower ventilation when compared to at BATS.
Historical DOC concentrations
Historically, surface DOC concentrations are consistently higher at ALOHA with an average of 76 μmol C kg−1at ALOHA compared to that of 65 μmol C kg−1 at BATS (ESI Online Material Fig. S1†). These elevated DOC inventories at ALOHA suggest site specific differences in DOC production and removal pathways, when compared to BATS, that promote the accumulation of excess DOC in the surface layer.36 This was also confirmed by an earlier study that showed that ALOHA has higher primary production than BATS on average over an 11 year dataset,37 although the annual variability is high and differences are rather small so that on average primary productivity may be somewhat similar between ALOHA and BATS. At BATS productivity is dominated by a seasonal cycle fuelled by nutrients from deep winter mixing, diffusive mixing, eddies, and N2 fixation whereas productivity at ALOHA exhibits far less seasonality and is rather stable as it is fuelled by nutrients from diffusive mixing and nitrogen fixation.
Elevated DOC concentrations in the ALOHA photic zone likely contribute to enhanced microbial oxidation resulting in lowered oxygen levels at ODZ, which is consistent with a 5 times greater contribution of DOC to organic matter degradation at ALOHA relative to BATS.38 Furthermore, the higher surface DOC at ALOHA does not translate to higher DOC concentration with depth. In fact, the deep ocean DOC concentration at station ALOHA (38 μmol C kg−1) is substantially lower than that of the deep Atlantic (43 μmol C kg−1) (ESI Online Material Fig. S2†). It was previously argued that slow degradation of DOC transiting between the Atlantic and Pacific Oceans during meridional overturning circulation may be responsible.2,39 However, there is much debate about the true age distribution of deep ocean DOC and its inherent reactivity.3,40 For example, different rates of DOM mineralization may result from substantial compositional differences and may also translate into differences in the deep ocean DOC concentrations. Furthermore, a recent study found that both bulk DOC and SPE-DOC behaved conservatively using a two-end member mixing model between the Atlantic and the Pacific, but more than 70% of the molecular ions identified using FT-ICR MS behaved conservatively.41 Therefore 10–20% of the molecular ions that were either added or removed highlight differences in DOM composition, and therefore reactivity, between ocean basins.
Optical property analysis
Previous studies have demonstrated that absorption at 300 nm and spectral slopes are indicators of CDOM molecular information.12,42–45 The absorption coefficient at 300 nm (a (300 nm)) at BATS was 0.083 m−1 at 10 m depth before increasing to 0.143 m−1 at 1000 m and reaching a maximum of 0.154 m−1 at 4000 m (ESI Online Material Fig. S3†). At the ALOHA surface, a (300 nm) was only 0.061 m−1 at 10 m and increased to a local maximum of 0.137 m−1 at 600 m. At depths greater than 600 m, the absorbance showed an overall decreasing trend and reached a local minimum of 0.094 m−1 at a final depth of 4730 m at ALOHA. Absorption coefficients across all wavelengths (e.g., 250–500 nm) were overall higher at BATS than at ALOHA. The spectral slope of 275–295 nm (S 275–295) at BATS was 0.041 nm−1 at the surface (10 m). Below 1000 m, S 275–295 at BATS showed rather constant values of around 0.028 nm−1 (Fig. S3†). At the ALOHA surface, S 275–295 was the highest at 0.046 nm−1, but below the mixed layer, values increased with depth, which contrasted with BATS where no such trend was observed. Changes in S 275–295 as well as the slope ratio (SR) (Fig. S3†) might be indicative of changes in molecular weight (MW) in freshwater/tidal areas where CDOM absorption coefficients correlate well with DOC concentrations.12 In the open ocean, it is unclear if similar interpretations can be made because there appears to be no relationship between CDOM and DOC in the major ocean basins.46 The E2/E3 increased at ALOHA between 500 m and roughly 3000 m, which was not observed at BATS (Fig. S3†). Nonetheless, the differences in optical properties between BATS and ALOHA could indicate differences in DOM processing and composition between these two locations. Depth profiles of the humic-like fluorescence (FDOMH) and protein-like components (FDOMP) have been previously described in detail and revealed an overall increase in FDOMH and decrease in FDOMP with depth.15,20,47 The terms humic-like and protein-like are often misleading, because the marine FDOM that mimics humic-like FDOM from freshwater systems is likely derived from marine primary producers and heterotrophic bacteria22,48,49 rather than terrestrially derived humic substances. Similarly, other compound classes have been discovered that fluoresce in the protein-like fluorescent area. Our data supported the previously established decreasing trend of FDOMP and an increasing trend of FDOMH with depth.15 EEM fluorescence components of the PPL SPE-DOM showed variations that were best fitted to a four component EEM-PARAFAC model (Fig. 1). Three out of the four validated components with maximum emission wavelengths follow the signal: Fmax 1 (453 nm), Fmax 2 (400 nm), and Fmax 4 (493 nm) fit the FDOMH descriptions. The Fmax 3 (325 nm) component with a maximum emission wavelength of 325 nm was optically similar to what was historically the FDOMP or protein-like component.
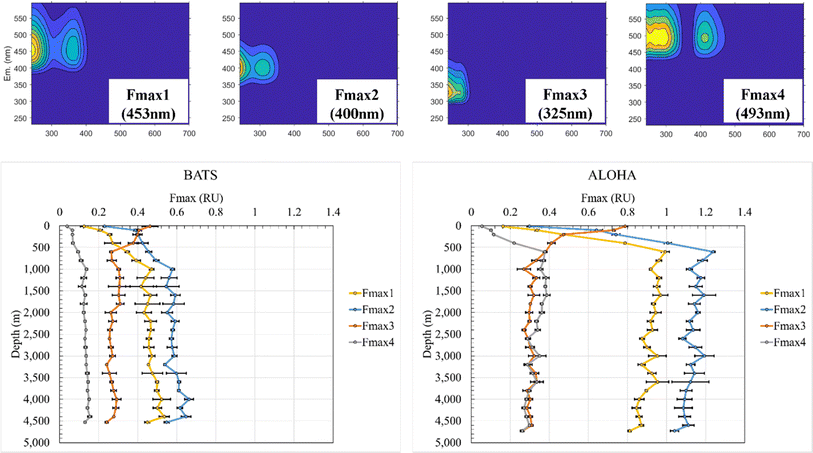 |
| Fig. 1 EEM-PARAFAC components of PPL SPE-DOM for BATS and ALOHA including maximum emission wavelength (top panel) and depth profiles of PARAFAC components 1–4 at BATS (bottom, left panel) and ALOHA (bottom, right panel). | |
F
max depth profiles at BATS and ALOHA revealed fundamental differences in the FDOM composition. Fmax 1 (453 nm) at BATS showed an increase in fluorescence from the surface to a local maximum of 0.47 RU at 1000 m before incrementally increasing with depth to a maximum of 0.54 RU at 4400 m and then decreasing to a local minimum of 0.45 RU just above the seafloor at 4530 m (Fig. 1). Fmax 1 (453 nm) at ALOHA showed a much greater increase in fluorescence from the surface to 600 m with a maximum of 0.99 RU before gradually decreasing to the minimum of 0.81 RU at the seafloor. Notably, Fmax 1 (453 nm) showed a rather distinct decrease between 4600 m and the seafloor at 4720 m, similar to the observed trend at BATS.
F
max 2 (400 nm) at BATS increased from the surface to a local maximum of 0.58 RU at 1000 m and a maximum of 0.66 RU at 4000 m and decreased to 0.54 RU at the bottom. Fmax 2 (400 nm) at ALOHA increased from the surface to a maximum of 1.24 RU at 600 m before gradually decreasing to a local minimum of 1.04 RU close to the seafloor. Fmax 3 (325 nm) was indicative of FDOMP and decreased at BATS from a maximum of 0.46 RU at the surface to 0.26 RU at 600 m before remaining relatively consistent with depth. Fmax 3 (325 nm) at ALOHA decreased from the maximum of 0.79 RU at the surface to a local minimum at 0.27 RU at 1000 m and remained relatively constant with depth. However, the notable decrease between the bottom samples collected just 10 m above the sediment was again observed for both stations. Fmax 4 (493 nm) at BATS increased from 0.04 RU from the surface to the local maximum of 0.14 RU at 1000 m before increasing gradually to the maximum at 0.15 RU at 4400 m and decreasing to 0.13 RU at the bottom. Fmax 4 (493 nm) at ALOHA increased from 0.05 RU to a local maximum at 0.38 RU at 600 m before gradually decreasing but with an increase to the maximum of 0.39 RU at 1600 m and a local minimum of 0.26 RU at the bottom. Once again, this component also showed this marked decrease just above the seafloor. Overall, all FDOMH components behaved similarly with depth, but FDOM values are roughly double at ALOHA than at BATS, except for the FDOMP.
The FDOMH EEM fluorescence intensity at surface waters was found to be depleted, while the FDOMP component was enriched, consistent with previous studies.15 These typical FDOMH components have been previously shown to increase with depth and were relatively stable below 1000–1400 m in bathypelagic layers of the global ocean.14,26,46 The FDOMP or protein-like component decreased in fluorescence with depth at both BATS and ALOHA. These results were also consistent with findings of previous studies.15 However, a detailed assessment of the results further illuminated the intricate dynamics of marine FDOM between and within the Atlantic and Pacific oceans. Specifically, the divergent behavior of FDOMH at depth with a continued decrease at ALOHA and increase at BATS below 1000 m is intriguing and warrants further investigation. One possible explanation is the injection of North Atlantic Deep Water (NADW) at BATS. At BATS the increase in FDOMH at depth may reflect Arctic-derived terrigenous DOC entrained during deep water formation, which is not the case in the Pacific Ocean. Further evidence is given in the higher UV-vis absorbance, but much lower overall fluorescence at BATS than at ALOHA, which is indicative of fundamental differences in the apparent fluorescence quantum yields. Hence, it is another complementary indicator for drastic differences in the underlaying molecular composition of CDOM at ALOHA when compared to BATS.
Apparent oxygen utilization and its correlation with FDOM
The AOU has been used as an indicator of microbial activity in the dark ocean,22,47 and has been previously correlated with FDOM in the Pacific and Indian Oceans but the relationship has not been explored in the Atlantic.15,47 We compared Fmax values for SPE-DOM to AOU in the water column at each site in order to provide new insights based on our high resolution data (ESI Online Material Fig. S4 and S5†). The FDOMH components: Fmax 1 (453 nm), Fmax 2 (400 nm) and Fmax 4 (493 nm) showed overall strong, positive correlations with AOU in the ALOHA profile, which is consistent with previous studies.47,50,51
F
max 1 (453 nm) and Fmax 2 (400 nm) showed positive correlations with AOU at ALOHA but, in contrast at BATS, no correlations were found throughout the entire water column (ESI Online Material Fig. S5†). Fmax 4 (493 nm) showed the strongest correlations at ALOHA, but it was also apparent that samples within the ODZ at BATS strongly influenced the correlation between BATS samples and AOU, which was not the case at ALOHA (Fig. S5†). To further assess the correlations, we only looked at samples below the ODZ and samples below 1200 m and a very strong correlation (R2 = 0.98) was found between Fmax 4 (493 nm) and AOU in the combined dataset from ALOHA and BATS, suggesting that EEM PARAFAC components very well reflect the overall AOU in both ocean basins at depth (Online ESI Material, Fig. S6†). The notable strong correlation between AOU and Fmax 4 (493 nm) at both stations has not been previously described and might indicate the specific usefulness of this EEM-PARAFAC component in describing AOU-FDOM relationships in the deep ocean.
Dissolved organic sulfur and phosphorus
Significant differences in DOM composition were also revealed by analyzing concentrations of two heteroatoms in SPE-DOM samples, i.e., DOS and DOP. SPE-DOS concentrations were more variable between replicates than SPE-DOP concentrations, but general trends were still observed. For instance, while SPE-DOS decreased until ∼1000 m and was relatively constant with depth at both stations, SPE-DOS concentrations were always higher at BATS compared to ALOHA (Fig. 2). For example, SPE-DOS averaged 154 ± 27 nM (n = 6) in samples collected above 200 m and 95.1 ± 16 nM (n = 24) in samples collected below 1000 m at BATS. At ALOHA, SPE-DOS averaged 101 ± 12 nM for samples <200 m (n = 6) and 56.8 ± 7.8 nM for samples collected below 1000 m. SPE-DOS concentrations have not been determined at BATS or ALOHA previously but are in good agreement with those determined during a large-scale survey in the East Atlantic Ocean and the Southern Ocean.52 In this work, SPE-DOS averaged 140 ± 20 nM and 80 ± 10 nM in samples collected between 0 and 100 m in the East Atlantic and Southern Ocean, respectively. SPE-DOS averaged 70 ± 10 nM for all samples collected below 1000 m during this large-scale survey,52 again in good agreement with SPE-DOS in deep samples at ALOHA (∼57 nM) and BATS (∼95 nM). Unfortunately, extraction efficiencies for DOS have not been determined, but these differences in PPL-extractable DOS between BATS, ALOHA, and the East Atlantic and Southern Ocean52 point towards variability in DOS composition across ocean basins.
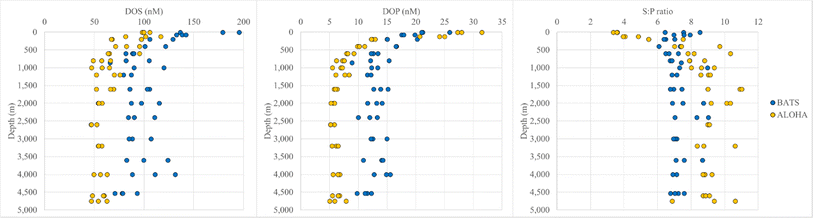 |
| Fig. 2 SPE-DOS (nM) (left panel), DOP (nM) (center panel) and molar S : P ratios (right) panel in SPE-DOM at Station ALOHA and BATS. | |
SPE-DOP concentrations showed a different trend compared to SPE-DOS concentrations and were higher at ALOHA in samples <200 m (26.1 ± 3.7 nM) than at BATS (20.6 ± 3.0 nM) (Fig. 2). Like DOC concentrations for bulk samples (ESI Online Material Fig. S1†), higher DOP concentrations have been reported for surface waters at ALOHA (∼200 nM) versus BATS (60 nM).53 Based on these reported DOP values for bulk seawater samples and our SPE-DOP values, DOP extraction efficiencies may be ∼10% at ALOHA and ∼30% at BATS. Recently, an improved UV photo-oxidation method for DON and DOP was introduced, allowing for higher resolution measurements of these species, especially in the deep ocean.54 Using this approach, DOP concentrations averaged 49 ± 4 nM in samples collected between 900 and 4800 m at ALOHA.54
SPE-DOP determined during our study decreased more rapidly with depth at ALOHA, so that SPE-DOP averaged for deep samples >1000 m and were lower at ALOHA (6.16 ± 0.86 nM) than at BATS (12.8 ± 1.6 nM). Again, comparing our SPE-DOP values to DOP concentrations before extraction54 suggests a DOP extraction efficiency of about 13% for deep samples at station ALOHA. While differences in extraction efficiency may occur with depth for these species, both DOC and DON extraction efficiencies did not change significantly with depth in previous studies.52 If this is the case, the implied difference in DOP extraction efficiency between ALOHA and BATS again points towards fundamentally different DOM compositions between these two sites. Moreover, molar SPE-DOS
:
SPE-DOP (S
:
P) ratios were fairly constant at BATS, averaging 7.32 ± 0.73 (n = 43) for the entire water column (Fig. 2). S
:
P ratios were far more variable at ALOHA, averaging 8.12 ± 2.1 (n = 45) for the entire water column, largely driven by the lower S
:
P ratios for shallow samples <200 m (3.90 ± 0.53) versus deep samples >1000 m (9.27 ± 0.93), again highlighting the differences in DOM composition between these two stations.
Similar to AOU trends, strong correlations were observed between Fmax values and SPE-DOP and S
:
P ratios at ALOHA, but not at BATS. Slopes of linear regressions between Fmax values and DOS, DOP, and S
:
P ratios are reported in ESI Online Material Table S1.† At ALOHA, FDOMH showed a negative correlation with SPE-DOP (R2 values of 0.86, 0.86, and 0.68 for Fmax 1, 2, and 4, respectively) and a positive correlation with S
:
P (R2 values of 0.74, 0.71, and 0.63 for Fmax 1, 2, and 4 respectively) (Fig. S7†). Fmax 3 had a strong positive correlation with SPE-DOP (R2 = 0.90) and a negative correlation with S
:
P (R2 = 0.76) and even correlated with SPE-DOP and S
:
P ratios at BATS, although these relationships were weaker (R2 value of 0.51). FDOMHFmax values also showed weaker, yet significant correlations with SPE-DOS at ALOHA (R2 values of 0.54, 0.52, and 0.45, respectively).
Ultrahigh resolution mass spectrometry
FT-ICR MS analysis of all samples collected at BATS and ALOHA were hierarchically clustered and a complete separation between BATS and ALOHA samples was observed apart from a surface SPE-DOM cluster that was composed of DOM signatures at both sample locations (ESI Online Material Fig. S8†). To further resolve the drivers of DOM compositional changes between BATS and ALOHA, we used large-scale association network learning and sparse orthogonal factor regression (SOFAR). The problem of bi-clustering large data matrices was framed as an unsupervised learning problem via a multivariate regression model on the FT-ICR MS matrix.55 SOFAR's model of the common signature dataset is of rank 7 with an R2 value (defined as the percent of total variation explained by the regression) of 0.866%. The first layer was visualized as shown in Fig. 3A. Layer 1 of SOFAR bi-clustering solution is visualized as shown in Fig. 3A. Because the R2 is 86.6%, this layer 1 explained (0.559 × 86.6)% or 48.4% of the FT-ICR MS data. The mass variable is on the x-axis and sorted by loading values. This layer indicated that BATS and ALOHA are associated with different molecular signatures. The molecular signatures are split into three groups and once again BATS and ALOHA samples were well separated with the most expressed molecular signatures for the BATS samples in group 1 and in group 3 for ALOHA samples, respectively (Fig. 3A). Loadings from the SOFAR analysis indicated that the molecular signatures that were higher at BATS had correspondingly higher O/C ratios overall, likely indicative of more microbially processed DOM56,57 (Fig. 3C). In contrast, ALOHA showed relatively lower O/C ratio signatures, which in turn would indicate a fresher and less oxygenated material (Fig. 3B). This trend was observed in the CHO and CHNO pool, with much more CHNO signatures highly expressed in the ALOHA samples. The CHOS pool seemed a special case where BATS still had relatively higher oxygenated CHOS signatures, but ALOHA only showed three CHOS signatures that were more expressed when compared to BATS (Fig. 3), which was consistent with the quantification of DOS (see Fig. 2 and ESI Online Material Fig. S9†). A more in-depth analysis of homologous series underlines the notion of overall increased abundances of highly oxygenated molecular signatures at BATS versus ALOHA within the CHO and CHNO pool (online ESI Material Fig. S9†).
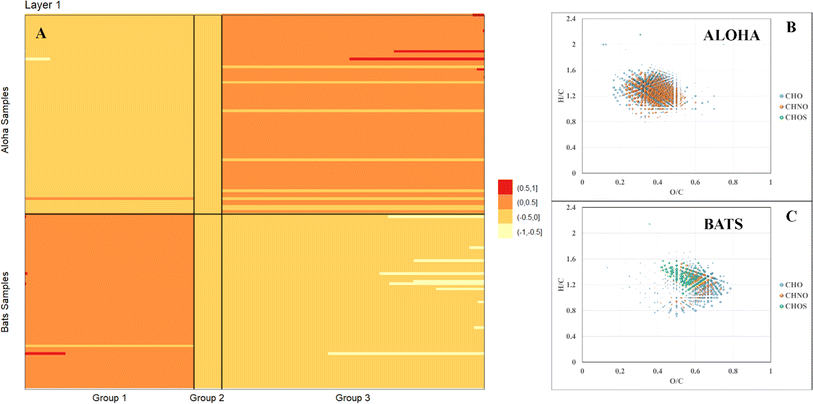 |
| Fig. 3 Image plot of the first Sparse orthogonal factor regression (SOFAR) layer of common molecular signatures derived from FT-ICR MS data of all BATS and ALOHA samples (A) and associated with most expressed signatures in van Krevelen space of ALOHA (B) and BATS (C) samples. Note: the bubble size corresponds to loadings from the SOFAR analysis. | |
Depth-dependencies of molecular signatures.
Molecular signatures and their assigned exact molecular formulae that showed either a substantial increase or decrease with depth were isolated and examples of depth profiles of individual formulae with increasing or decreasing abundances of their associated m/z ions are shown (Fig. 4). An increase in a specific molecular formula is indicative of the accumulation of DOM which is, at least temporally, refractory to microbial decomposition, whereas a decrease in a molecular formula suggests its loss, potentially through microbial degradation. Consequently, structural elucidation of these molecular formulae may provide the critical step in developing DOM reactivity tracers to determine DOM turnover times, which remains a fundamental gap in our understanding of the marine carbon cycle.
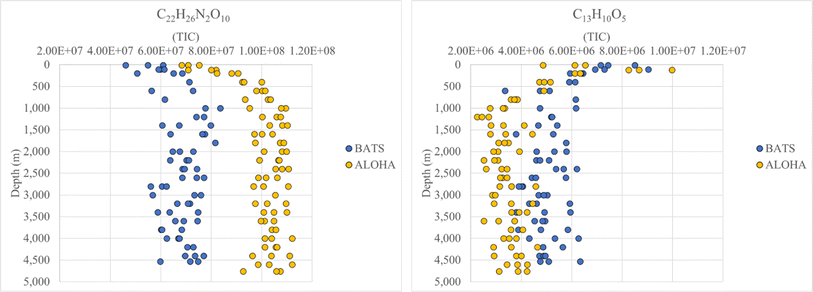 |
| Fig. 4 Examples of molecular signatures at BATS and ALOHA, which either substantially increase (left panel) or decrease (right panel) with depth. | |
Correlations between molecular signatures and FDOM
FT-ICR MS data have been previously compared and correlated with optical properties,58–61 but no detailed molecular formulae were given that likely could explain, at least in part, the observed fluorescence in freshwater, estuarine or marine environments. We further investigated the combined data of BATS and ALOHA to extract strongly correlating m/z ions with EEM-PARAFAC components, and three long homologous series centred around the assigned neutral molecular formulae C22H26O7 ± nCH2, C22H26N2O10 ± nCH2, and C22H23NO9 ± nCH2 are visualized (Fig. 5). The depth profiles highlighted the distinct differences in abundance between BATS and ALOHA with an increase in abundance with depth observed at both stations (Fig. 5). A linear regression analysis of Fmax 1, Fmax 2 and Fmax 4versus the combined abundances of all members of the homologous series of the above-described exact molecular formulae revealed strong correlations (Fig. 6). It is noteworthy that two of the three isolated homologous series are nitrogen-containing compounds, and one has 2 nitrogen atoms. Previous work suggested a potential picocyanobacterial source of marine FDOM,48 and the study also described the abundance of compounds containing 2 nitrogen atoms after long-term incubation experiments. These three exact molecular formulae and their associated homologous series are again excellent targets for structural elucidation and may reveal aromatic and conjugated structures that can explain in part FDOM distributions. To expand this analysis through statistical means, we again utilized the SOFAR approach to determine the possible linkage between FT-ICR MS data and EEM-PARAFAC components. At a factor loading of a magnitude greater than 0.1, group 1 corresponded to humic-like fluorescence components 1,2 and 4 and group 2 was associated with the protein-like component 3. Group 1 contained 1350 associated molecular signatures and group 2 contained 269 associated molecular signatures. The results from this analysis supported the much more pronounced influence of nitrogen-containing signatures in explaining the fluorescence data. The strongest linkages between FT-ICR MS and fluorescence components are provided in van Krevelen space (ESI Online Material, Fig. S10†). Although the structural elucidation of compounds within extremely complex mixtures is very challenging, it is not without hope and here we recommend some target molecular signatures for further investigation.
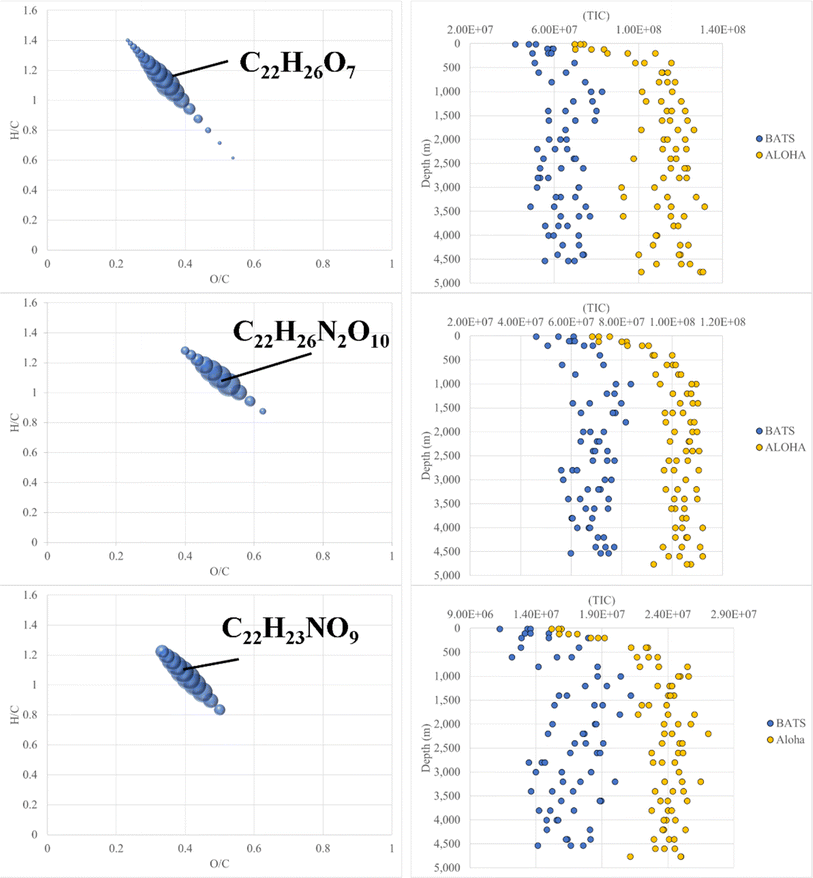 |
| Fig. 5 Van Krevelen diagram (left panel) and selected depth profiles (right panel) of homologous series based on CH2 spacing that increase with water depth, correlate well with FDOM and hence show significant differences between BATS and ALOHA. | |
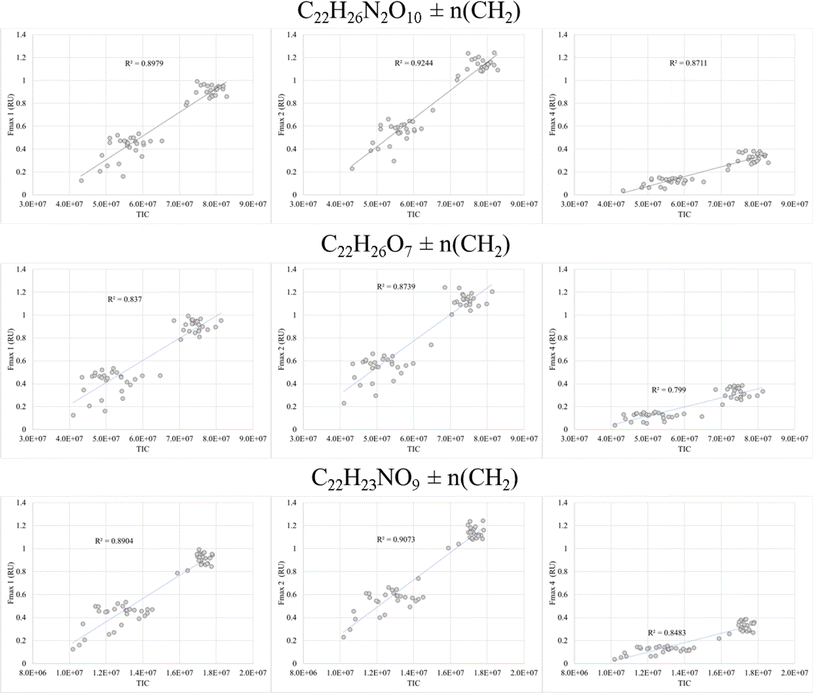 |
| Fig. 6 Linear regression of Fmax PARAFAC components versus combined intensities of all homologous series members centered around formula C22H26N2O10 (top panel), C22H26O7 (center panel) and C22H23NO9 (bottom panel). | |
Weak anion-exchange amendable DOM
A final piece of information, which once again provides evidence of fundamental compositional differences within the marine DOM pool at BATS and ALOHA was provided by the weak anion exchange (WAX) fraction of DOM. For the first time, this fraction is described in marine DOM and a list of assigned molecular formulae is given (ESI Online Material, Table S2†). This WAX fraction is highly specific and only the anionically adsorbed material was considered (methanol wash first and disgarded, followed by ammonium hydroxide/methanol elution; see material and method section). The assigned molecular formulae were highly clustered in van Krevelen space and showed expected high O/C ratios but seemed not to be aliphatic based on H/C ratios of as low as 0.9 (Fig. 7). This highly specific pool of likely aromatic, but WAX amendable DOM showed much higher abundances at BATS than at ALOHA, which was interesting given that primary production was much higher at ALOHA during sampling as inferred from chlorophyll-a measurements and AOU. This highlights a perhaps much higher production of this specific DOM at BATS than at ALOHA (Fig. 7). More work is needed to better understand the molecular composition of this highly polar marine DOM.
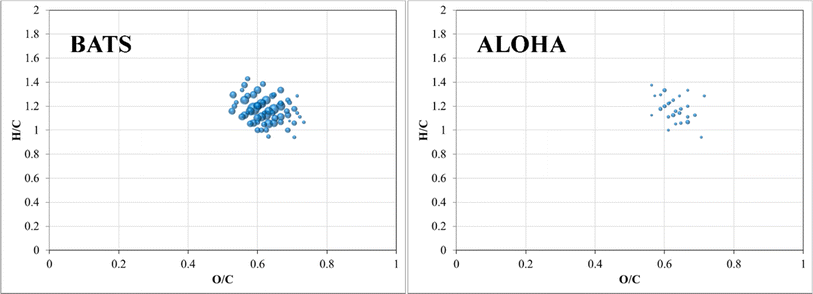 |
| Fig. 7 Van Krevelen diagrams of surface WAX SPE-DOM at BATS (left) and ALOHA (right). Note: bubble size indicates absolute abundance in total ion counts of negatively charged m/z ions. | |
Conclusions
The fundamentally different analytical windows of optical properties and FT-ICR MS converged and showed an overall coherence in results. This study supplied evidence to make a case that DOM at ALOHA is profoundly different than that at BATS. For the first time, exact molecular formula series are given that have the potential to explain marine FDOM in the deep ocean. These molecular formulae serve as a starting point for structural elucidation to better predict deep ocean DOM turnover and the overall turnover of marine FDOM. The challenge to solve the reactivity riddle of deep ocean carbon cycling has only begun.
Author contributions
The corresponding author was responsible for funding acquisition, conceptualization, methodology, supervision of co-author Madeline Lahm, visualization, writing, review and editing. Co-author Lahm performed formal analysis, data curation, writing draft. Co-author Powers was responsible for data curation, methodology, writing, review, editing, visualization and formal analysis. Co-author Feng was involved in project administration, funding acquisition, and writing and editing. Co-author McCallister contributed to conceptualization, writing, review and editing. Co-author Liang assisted in formal analysis, software, and visualization. Co-author Guinan performed formal analysis, validation, visualization, and writing. Co-author Schmitt-Kopplin supplied resources and assisted in writing, review, and editing.
Conflicts of interest
There are no conflicts to declare.
Acknowledgements
We thank the captains and crew of the R/V Atlantic Explorer and R/V Kilo Moana for their assistance in collecting samples at the Bermuda Atlantic Time-series Station and Hawai'i Oceanic Time-series station ALOHA, respectively. The project was funded by the National Science Foundation NSF award OCE 1829888 and partially supported by the NSF funded REU progam (OCE-1756244) to support REU student Grace Guinan.
References
-
A. Ridgwell and S. Arndt, in Biogeochemistry of Marine Dissolved Organic Matter, ed. D. A. Hansell and C. A. Carlson, Academic Press, Boston, 2nd edn, 2015, pp. 1–20, DOI:10.1016/B978-0-12-405940-5.00001-7.
- D. A. Hansell, C. A. Carlson, D. J. Repeta and R. Schlitzer, Dissolved organic matter in the ocean: A controversy stimulates new insights, Oceanography, 2009, 22, 202–211 CrossRef.
- M. Gonsior, L. Powers, M. Lahm and S. L. McCallister, New Perspectives on the Marine Carbon Cycle – The Marine Dissolved Organic Matter Reactivity Continuum, Environ. Sci. Technol., 2022, 56, 5371–5380 CrossRef CAS PubMed.
- E. R. M. Druffel, C. B. Lewis, S. Griffin, A. Flaherty, M. Rudresh, N. E. Hauksson, R. M. Key, A. P. McNichol, J. Hwang and B. D. Walker, Dissolved Organic Radiocarbon in the West Indian Ocean, Geophys. Res. Lett., 2023, 50, e2023GL104732 CrossRef CAS.
- E. R. M. Druffel, S. Griffin, C. B. Lewis, M. Rudresh, N. G. Garcia, R. M. Key, A. P. McNichol, N. E. Hauksson and B. D. Walker, Dissolved Organic Radiocarbon in the Eastern Pacific and Southern Oceans, Geophys. Res. Lett., 2021, 48, e2021GL092904 CrossRef CAS.
- G. W. Luther III, Hydrothermal Vents Are a Source of Old Refractory Organic Carbon to the Deep Ocean, Geophys. Res. Lett., 2021, 48, e2021GL094869 CrossRef.
- J. W. Pohlman, J. E. Bauer, E. A. Canuel, K. S. Grabowski, D. L. Knies, C. S. Mitchell, M. J. Whiticar and R. B. Coffin, Methane sources in gas hydrate-bearing cold seeps: Evidence from radiocarbon and stable isotopes, Mar. Chem., 2009, 115, 102–109 CrossRef CAS.
- M. D. McCarthy, S. R. Beaupre, B. D. Walker, I. Voparil, T. P. Guilderson and E. R. M. Druffel, Chemosynthetic origin of 14C-depleted dissolved organic matter in a ridge-flank hydrothermal system, Nat. Geosci., 2011, 4, 32–36 CrossRef CAS.
- C. L. Follett, D. J. Repeta, D. H. Rothman, L. Xu and C. Santinelli, Hidden cycle of dissolved organic carbon in the deep ocean, Proc. Natl. Acad. Sci. U. S. A., 2014, 111, 16706–16711 CrossRef CAS PubMed.
- P. K. Zigah, A. P. McNichol, L. Xu, C. Johnson, C. Santinelli, D. M. Karl and D. J. Repeta, Allochthonous sources and dynamic cycling of ocean dissolved organic carbon revealed by carbon isotopes, Geophys. Res. Lett., 2017, 44, 2407–2415 CrossRef CAS.
- T. A. B. Broek, B. D. Walker, T. P. Guilderson, J. S. Vaughn, H. E. Mason and M. D. McCarthy, Low Molecular Weight Dissolved Organic Carbon: Aging, Compositional Changes, and Selective Utilization During Global Ocean Circulation, Global Biogeochem. Cycles, 2020, 34, e2020GB006547 CrossRef CAS.
- J. R. Helms, A. Stubbins, J. D. Ritchie, E. C. Minor, D. J. Kieber and K. Mopper, Absorption spectral slopes and slope ratios as indicators of molecular weight, source, and photobleaching of chromophoric dissolved organic matter, Limnol. Oceanogr., 2008, 53, 955–969 CrossRef.
- R. Del Vecchio and N. V. Blough, Spatial and seasonal distribution of chromophoric dissolved organic matter and dissolved organic carbon in the Middle Atlantic Bight, Mar. Chem., 2004, 89, 169–187 CrossRef CAS.
-
C. A. Stedmon and N. B. Nelson, in Biogeochemistry of Marine Dissolved Organic Matter, ed. D. A. Hansell and C. A. Carlson, Academic Press - Elsevier, 2nd edn, 2015, pp. 481–503 Search PubMed.
- L. Jørgensen, C. A. Stedmon, T. Kragh, S. Markager, M. Middelboe and M. Sondergaard, Global trends in the fluorescence characteristics and distribution of marine dissolved organic matter, Mar. Chem., 2011, 126, 139–148 CrossRef.
- J. Peuravuori and K. Pihlaja, Molecular size distribution and spectroscopic properties of aquatic humic substances, Anal. Chim. Acta, 1997, 337, 133–149 CrossRef CAS.
- P. Li and J. Hur, Utilization of UV-vis spectroscopy and related data analyses for dissolved organic matter (DOM) studies: A review, Crit. Rev. Environ. Sci. Technol., 2017, 47, 131–154 CrossRef CAS.
- K. R. Murphy, C. A. Stedmon, D. Graeber and R. Bro, Fluorescence spectroscopy and multi-way techniques. PARAFAC, Anal. Methods, 2013, 5, 6557–6566 RSC.
- C. A. Stedmon and R. Bro, Characterizing dissolved organic matter fluorescence with parallel factor analysis: a tutorial, Limnol. Oceanogr.: Methods, 2008, 6, 572–579 CrossRef CAS.
- T. S. Catalá, I. Reche, A. Fuentes-Lema, C. Romera-Castillo, M. Nieto-Cid, E. Ortega-Retuerta, E. Calvo, M. Álvarez, C. Marrasé, C. A. Stedmon and X. A. Álvarez-Salgado, Turnover time of fluorescent dissolved organic matter in the dark global ocean, Nat. Commun., 2015, 6, 5986 CrossRef PubMed.
-
C. A. Stedmon and N. B. Nelson, in Biogeochemistry of Marine Dissolved Organic Matter, ed. C. A. Carlson, Academic Press, Boston, 2nd edn, 2015, pp. 481–508, DOI:10.1016/B978-0-12-405940-5.00010-8.
- X. L. Xiao, Y. Yamashita, M. Gonsior and N. Z. Jiao, The efficiency of the microbial carbon pump as seen from the relationship between apparent oxygen utilization and fluorescent dissolved organic matter, Prog. Oceanogr., 2023, 210, 102929 CrossRef.
- Y. Yamashita, R. M. Cory, J. Nishioka, K. Kuma, E. Tanoue and R. Jaffé, Fluorescence characteristics of dissolved organic matter in the deep waters of the Okhotsk Sea and the northwestern North Pacific Ocean, Deep Sea Res., Part II, 2010, 57, 1478–1485 CrossRef CAS.
- Y. Yamashita, A. Tsukasaki, T. Nishida and E. Tanoue, Vertical and horizontal distribution of fluorescent dissolved organic matter in the Southern Ocean, Mar. Chem., 2007, 106, 498–509 CrossRef CAS.
- M. Gonsior, J. Luek, P. Schmitt-Kopplin, J. M. Grebmeier and L. W. Cooper, Optical Properties and Molecular Diversity of Dissolved Organic Matter in the Bering Strait and Chukchi Sea, Deep Sea Res., Part II, 2017, 104–111 CrossRef CAS.
- S. A. Timko, A. Maydanov, S. L. Pittelli, M. H. Conte, W. J. Cooper, B. P. Koch, P. Schmitt-Kopplin and M. Gonsior, Depth-dependent Photodegradation of Marine Dissolved Organic Matter, Front. mar. sci., 2015, 2 Search PubMed.
- O. J. Lechtenfeld, G. Kattner, R. Flerus, S. L. McCallister, P. Schmitt-Kopplin and B. P. Koch, Molecular transformation and degradation of refractory dissolved organic matter in the Atlantic and Southern Ocean, Geochim. Cosmochim. Acta, 2014, 126, 321–337 CrossRef CAS.
- R. Flerus, O. J. Lechtenfeld, B. P. Koch, S. L. McCallister, P. Schmitt-Kopplin, R. Benner, K. Kaiser and G. Kattner, A molecular perspective on the ageing of marine dissolved organic matter, Biogeosciences, 2012, 9, 1935–1955 CrossRef CAS.
- M. Seidel, S. P. B. Vemulapalli, D. Mathieu and T. Dittmar, Marine Dissolved Organic Matter Shares Thousands of Molecular Formulae Yet Differs Structurally across Major Water Masses, Environ. Sci. Technol., 2022, 56, 3758–3769 CrossRef CAS PubMed.
- N. Hertkorn, M. Harir, B. P. Koch, B. Michalke and P. Schmitt-Kopplin, High-field NMR spectroscopy and FTICR mass spectrometry: powerful discovery tools for the molecular level characterization of marine dissolved organic matter, Biogeosciences, 2013, 10, 1583–1624 CrossRef CAS.
- M. S. Twardowski, E. Boss, J. M. Sullivan and P. L. Donaghay, Modeling the spectral shape of absorption by chromophoric dissolved organic matter, Mar. Chem., 2004, 89, 69–88 CrossRef CAS.
- D. Tziotis, N. Hertkorn and P. Schmitt-Kopplin, Kendrick-Analogous Network Visualisation of Ion Cyclotron Resonance Fourier Transform Mass Spectra: Improved Options for the Assignment of Elemental Compositions and the Classification of Organic Molecular Complexity, Eur. J. Mass Spectrom., 2011, 17, 415–421 CrossRef CAS PubMed.
- Y. Uematsu, Y. Fan, K. Chen, J. Lv and W. Lin, SOFAR: Large-Scale Association Network Learning, IEEE Trans. Inf. Theory, 2019, 65, 4924–4939 Search PubMed.
-
K. Chen and W. Wang, rrpack: Reduced-Rank Regression. R package version 0.1–13, https://cran.r-project.org/web/packages/rrpack.%3ci%3eJournal%3c/i%3e, 2022 Search PubMed.
- L. C. Powers, L. L. Lapham, S. Y. Malkin, A. Heyes, P. Schmitt-Kopplin and M. Gonsior, Molecular and optical characterization reveals the preservation and sulfurization of chemically diverse porewater dissolved organic matter in oligohaline and brackish Chesapeake Bay sediments, Org. Geochem., 2021, 161, 104324 CrossRef CAS.
- C. A. Carlson, H. W. Ducklow and A. F. Michaels, Annual flux of dissolved organic carbon from the euphotic zone in the northwestern Sargasso Sea, Nature, 1994, 371, 405–408 CrossRef CAS.
- D. Karl, J. Dore, R. Lukas, A. Michaels, N. Bates and A. Knap, Building the Long-Term Picture: The U. S. JGOFS Time-Series Programs, Oceanography, 2001, 14, 6–17 CrossRef.
- S. Emerson, Annual net community production and the biological carbon flux in the ocean, Global Biogeochem. Cycles, 2014, 28, 14–28 CrossRef CAS.
- J. E. Bauer, P. M. Williams and E. R. M. Druffel, 14C activity of dissolved organic carbon fractions in the north-central Pacific and Sargasso Sea, Nature, 1992, 357, 667–670 CrossRef CAS.
- F. Baltar, X. A. Alvarez-Salgado, J. Arístegui, R. Benner, D. A. Hansell, G. J. Herndl and C. Lønborg, What Is Refractory Organic Matter in the Ocean?, Front. mar. sci., 2021, 8 Search PubMed.
- S. K. Bercovici, T. Dittmar and J. Niggemann, Processes in the Surface Ocean Regulate Dissolved Organic Matter Distributions in the Deep, Global Biogeochem. Cycles, 2023, 37, e2023GB007740 CrossRef CAS.
- A. A. Andrew, R. Del Vecchio, A. Subramaniam and N. V. Blough, Chromophoric dissolved organic matter (CDOM) in the Equatorial Atlantic Ocean: Optical properties and their relation to CDOM structure and source, Mar. Chem., 2013, 148, 33–43 CrossRef CAS.
- C. M. Sharpless and N. V. Blough, The importance of charge-transfer interactions in determining chromophoric dissolved organic matter (CDOM) optical and photochemical properties, Environ. Sci.: Processes Impacts, 2014, 16, 654–671 RSC.
- E. S. Boyle, N. Guerriero, A. Thiallet, R. Del Vecchio and N. V. Blough, Optical Properties of Humic Substances and CDOM: Relation to Structure, Environ. Sci. Technol., 2009, 43, 2262–2268 CrossRef CAS PubMed.
- B. J. Dalzell, E. C. Minor and K. M. Mopper, Photodegradation of estuarine dissolved organic matter: a multi-method assessment of DOM transformation, Org. Geochem., 2009, 40, 243–257 CrossRef CAS.
- N. B. Nelson and D. A. Siegel, The Global Distribution and Dynamics of Chromophoric Dissolved Organic Matter, Annu. Rev. Mar. Sci., 2013, 5, 447–476 CrossRef PubMed.
- Y. Yamashita and E. Tanoue, Production of bio-refractory fluorescent dissolved organic matter in the ocean interior, Nat. Geosci., 2008, 1, 579–582 CrossRef CAS.
- Z. Zhao, M. Gonsior, J. Luek, S. Timko, H. Ianiri, N. Hertkorn, P. Schmitt-Kopplin, X. Fang, Q. Zeng, N. Jiao and F. Chen, Picocyanobacteria and deep-ocean fluorescent dissolved organic matter share similar optical properties, Nat. Commun., 2017, 8, 15284 CrossRef CAS PubMed.
- D. K. Steinberg, N. B. Nelson, C. A. Carlson and A. C. Prusak, Production of chromophoric dissolved organic matter (CDOM) in the open ocean by zooplankton and the colonial cyanobacterium Trichodesmium spp, Mar. Ecol.: Prog. Ser., 2004, 267, 45–56 CrossRef CAS.
- R. H. R. Stanley, S. C. Doney, W. J. Jenkins and I. D. E. Lott, Apparent oxygen utilization rates calculated from tritium and helium-3 profiles at the Bermuda Atlantic Time-series Study site, Biogeosciences, 2012, 9, 1969–1983 CrossRef CAS.
- N. B. Nelson, D. A. Siegel, C. A. Carlson and C. M. Swan, Tracing global biogeochemical cycles and meridional overturning circulation using chromophoric dissolved organic matter, Geophys. Res. Lett., 2010, 37, L03610 CrossRef.
- K. B. Ksionzek, O. J. Lechtenfeld, S. L. McCallister, P. Schmitt-Kopplin, J. K. Geuer, W. Geibert and B. P. Koch, Dissolved organic sulfur in the ocean: Biogeochemistry of a petagram inventory, Science, 2016, 354, 456–459 CrossRef CAS PubMed.
- Z. Liang, K. McCabe, S. E. Fawcett, H. J. Forrer, F. Hashihama, C. Jeandel, D. Marconi, H. Planquette, M. A. Saito, J. A. Sohm, R. K. Thomas, R. T. Letscher and A. N. Knapp, A global ocean dissolved organic phosphorus concentration database (DOPv2021), Sci. Data, 2022, 9, 772 CrossRef CAS PubMed.
- R. K. Foreman, K. M. Björkman, C. A. Carlson, K. Opalk and D. M. Karl, Improved ultraviolet photo-oxidation system yields estimates for deep-sea dissolved organic nitrogen and phosphorus, Limnol. Oceanogr.: Methods, 2019, 17, 277–291 CrossRef CAS.
- M. Lee, H. Shen, J. Z. Huang and J. S. Marron, Biclustering via Sparse Singular Value Decomposition, Biometrics, 2010, 66, 1087–1095 CrossRef PubMed.
- R. S. Horvath, Microbial co-metabolism and the degradation of organic compounds in nature, Bacteriol. Rev., 1972, 36, 146–155 CrossRef CAS PubMed.
-
P. S. Phale, H. Malhotra and B. A. Shah, in Advances in Applied Microbiology, ed. G. M. Gadd and S. Sariaslani, Academic Press, 2020, vol. 112, pp. 1–65 Search PubMed.
- A. M. Martínez-Pérez, M. Nieto-Cid, H. Osterholz, T. S. Catalá, I. Reche, T. Dittmar and X. A. Álvarez-Salgado, Linking optical and molecular signatures of dissolved organic matter in the Mediterranean Sea, Sci. Rep., 2017, 7, 3436 CrossRef PubMed.
- M. Gonsior, J. Valle, P. Schmitt-Kopplin, N. Hertkorn, D. Bastviken, J. Luek, M. Harir, W. Bastos and A. Enrich-Prast, Chemodiversity of dissolved organic matter in the Amazon Basin, Biogeosciences, 2016, 13, 4279–4290 CrossRef CAS.
- L. C. Powers, J. L. Luek, P. Schmitt-Kopplin, B. J. Campbell, C. Magen, L. W. Cooper and M. Gonsior, Seasonal changes in dissolved organic matter composition in Delaware Bay, USA in March and August 2014, Org. Geochem., 2018, 122, 87–97 CrossRef CAS.
- A. Stubbins, J. F. Lapierre, M. Berggren, Y. T. Prairie, T. Dittmar and P. A. del Giorgio, What's in an EEM? Molecular Signatures Associated with Dissolved Organic Fluorescence in Boreal Canada, Environ. Sci. Technol., 2014, 48, 10598–10606 CrossRef CAS PubMed.
|
This journal is © The Royal Society of Chemistry 2024 |
Click here to see how this site uses Cookies. View our privacy policy here.