DOI:
10.1039/D4CS00432A
(Review Article)
Chem. Soc. Rev., 2025,
54, 367-395
Development of the design and synthesis of metal–organic frameworks (MOFs) – from large scale attempts, functional oriented modifications, to artificial intelligence (AI) predictions
Received
31st August 2024
First published on 25th November 2024
Abstract
Owing to the exceptional porous properties of metal–organic frameworks (MOFs), there has recently been a surge of interest, evidenced by a plethora of research into their design, synthesis, properties, and applications. This expanding research landscape has driven significant advancements in the precise regulation of MOF design and synthesis. Initially dominated by large-scale synthesis approaches, this field has evolved towards more targeted functional modifications. Recently, the integration of computational science, particularly through artificial intelligence predictions, has ushered in a new era of innovation, enabling more precise and efficient MOF design and synthesis methodologies. The objective of this review is to provide readers with an extensive overview of the development process of MOF design and synthesis, and to present visions for future developments.
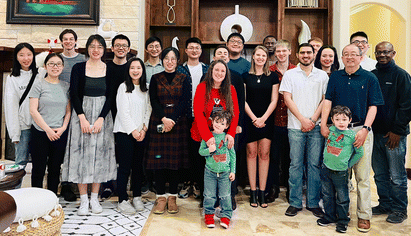 Zhou's group | The Zhou Research Group focuses on interdisciplinary research spanning Chemistry, Biochemistry, and Materials Science and Engineering with an emphasis on porous materials such as metal–organic frameworks (MOFs), porous polymer networks (PPNs), and covalent and coordination cages. By exploiting the extreme tunability of MOFs, PPNs and other porous materials, the group seeks to understand the role that material architecture and functionality has on its chemical and physical properties and how modifying these properties can lead to the development of MOFs and PPNs for applications related to gas and energy storage, separations, catalysis, enzyme immobilization and drug delivery. |
1. Introduction
Metal–organic frameworks (MOFs) are a class of crystalline materials characterized by their unique porous structures and exceptional properties.1–3 Composed of metal ions or clusters coordinated to organic ligands, MOFs feature intricate frameworks with distinct pore environments. This architecture grants MOFs an extraordinary surface area, often surpassing that of traditional porous materials, making them highly effective for a variety of applications.4–10 The versatility of MOFs arises from the vast combinatorial possibilities of metal nodes and organic linkers, allowing for tailored synthesis to meet specific needs. This adaptability has propelled MOFs to the forefront of research and industrial applications.11–15
In theoretical research, the development of MOFs has significantly expanded the fields of coordination chemistry and topology, deepening the understanding of coordination geometries and topological structures.16–20
In practical applications, the potential of MOFs is amplified by their stability and recyclability, positioning them as sustainable alternatives to conventional materials. As advancements in MOF design and synthesis continue to emerge, these materials hold promise for addressing some of the most pressing challenges in energy, environment, and health. The ongoing exploration of MOFs represents a vibrant and rapidly evolving frontier in materials science.21–25
Traditional design principles and synthesis methods heavily rely on high-throughput attempts within a continuous “trial and error” process to combine different metals and ligands, leading to the creation of various novel structures. Building on the solid foundations of primary research, functional-oriented design and modification methods have been developed, reducing the time and cost associated with numerous attempts. These advanced methods allow for the introduction and modification of target coordination fragments and functional groups at specific positions to achieve desired properties and functions. However, the vast number of possible combinations of metal nodes and organic linkers makes the experimental synthesis and modification of MOFs time-consuming and resource-intensive processes. Recently, with the evolution of computer science and technology, significant advancements in machine learning (ML) and artificial intelligence (AI) have further accelerated the design and modification of new structures for targeted applications. AI-assisted MOF synthesis can address these challenges by leveraging ML algorithms and computational models to predict optimal synthetic routes and material properties. Recent research progress has focused on developing AI models that can rapidly screen large chemical spaces, predict MOF stability and performance, and even suggest novel MOF structures with desired characteristics. These AI tools can analyse vast amounts of experimental data, helping to identify patterns and correlations that are not easily discernible by human researchers. The necessity of AI in MOF synthesis arises from the sheer complexity of the design space and the desire to accelerate the discovery and development of novel MOFs for emerging applications, reducing both the cost and time of experimentation while enhancing the precision and scalability of the synthesis process. These technologies enhance the efficiency and precision of identifying optimal combinations and configurations, thereby revolutionizing this field and opening new frontiers in materials science (Fig. 1).
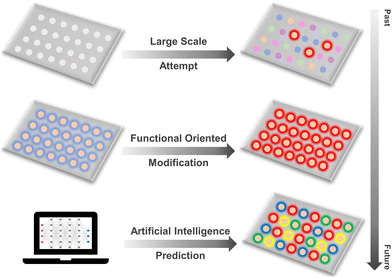 |
| Fig. 1 Schematic representation of the development of the design and synthesis of MOFs. | |
1.1 Origin and development of MOFs
The origin and development of MOFs represent a remarkable journey in the field of porous science, beginning in the late 20th century. Initially rooted in coordination chemistry, the early concepts of MOFs evolved from the synthesis of coordination polymers.26–28 The pivotal moment came in the 1990s with the pioneering work of researchers, such as Prof. O. M. Yaghi, who demonstrated that combining metal salts with organic linkers could create highly porous, crystalline structures with vast surface areas and customizable properties.29–31 This innovation provided a new class of materials with significant potential for various applications based on their unique pore properties.
The subsequent decades saw rapid advancements in MOF research, driven by improvements in synthesis techniques and computational modelling (Fig. 2). These advancements enabled the precise design and functionalization of MOFs, significantly broadening their applicability. The ability to tailor the pore size, shape, and functionality of MOFs has led to their exploration in numerous fields, including environmental remediation, energy storage, and drug delivery.32–36
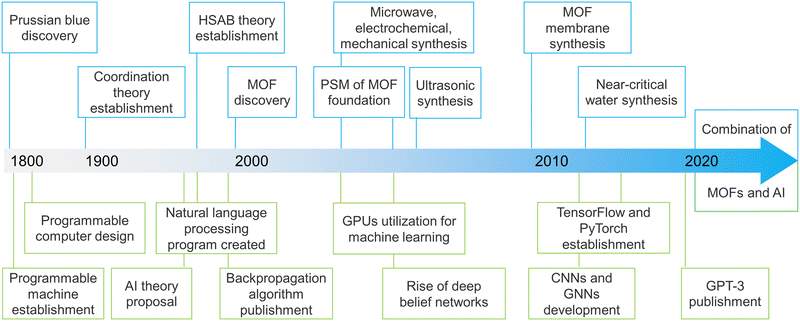 |
| Fig. 2 Simplified development timeline of MOFs and AI. HSAB stands for hard–soft acid–base, while PSM stands for post-synthetic modification. CNN stand for convolutional neural network, while GNN stands for graph neural network. | |
Nowadays, MOFs continue to be a vibrant area of research, with ongoing efforts focused on discovering new frameworks, enhancing their stability, and developing scalable production methods. The continuous innovation in this field promises to address some of the most pressing challenges in technology and industry, positioning MOFs as a cornerstone of future scientific and industrial advancements.37–40
1.2 Machine learning and artificial intelligence
The evolution of computer science and technology has catalyzed significant advancements in the fields of ML and AI. AI technology emulates the human intelligence processes, enabling machines to perform complex tasks such as natural language understanding, vision perception, and decision-making. ML, a primary state of AI, focuses on developing algorithms that allow computers to learn from data and make accurate predictions (Fig. 3).41–44
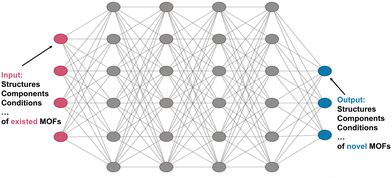 |
| Fig. 3 Schematic representation of the ML and AI-guided MOF design and synthesis. | |
With the surging development of computational power and data storage capacities, modern computers are endowed with the ability to employ ML and AI models to process and analyse vast datasets, uncovering patterns and insights beyond human reach. The iterative learning capabilities of ML and AI—through methods such as supervised, unsupervised, and reinforcement learning—have been enhanced by advancements in hardware, software, and data acquisition techniques, which drives innovation and efficiency in healthcare, finance, transportation, and beyond. In healthcare, these technologies enable breakthroughs in disease detection and personalized medicine. In finance, they improve fraud detection and algorithmic trading. Autonomous vehicles, intelligent virtual assistants, and personalized recommendation systems illustrate their broad impact on daily life.45–47
Ongoing research aims to refine ML and AI methodologies, emphasizing model interpretability, robustness, and ethical considerations. The continuous interplay between technological development and ML/AI advancements promises to address complex global challenges, augment human abilities, and foster unprecedented opportunities for innovation. This dynamic field remains at the forefront of the digital transformation, representing a pivotal area of growth in modern technology.48–50
2. Traditional design principle and synthesis method
As a class of crystalline porous materials constructed through the coordination interactions between metal ions and organic ligands, the structures of MOFs depend heavily on the selection of metals, ligands, solvents, and other reaction conditions, like temperature and pressure. Based on this, the design principles and synthesis methods of MOFs are pivotal in determining their properties and functions. Traditionally, researchers have employed high-throughput screening under a continuous “trial and error” process to construct novel MOFs with specific components and spatial structures, employing various synthesis methods. While this approach is straightforward in design, it is both time and cost consuming.
2.1 Traditional design principle
The design of MOFs revolves around the deliberate selection and combination of metal nodes and organic linkers to create frameworks with specific structures, properties, and functionalities. The key principles primarily involve the careful matching of metal salts, ligands, solvents, and reaction conditions (Fig. 4).
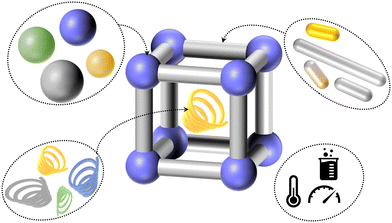 |
| Fig. 4 Design principle of MOFs through the selection of metal salts, ligands, solvents, and reaction conditions. | |
In general, the synthesis of MOFs is a complex process that typically involves three key stages: the formation of coordination bonds, nucleation, and crystal growth. Initially, metal ions or clusters interact with organic ligands to form coordination bonds, establishing the foundational framework of the material. This step defines the basic connectivity and geometry of the MOF structure. Once coordination bonds are formed, nucleation begins, where small, stable clusters—known as crystal nuclei—emerge. The conditions at this stage, such as temperature, solvent, and concentration, significantly influence the size and number of these nuclei, which in turn affects the final morphology of the MOF. After nucleation, the crystal growth phase follows, during which the nuclei expand into well-defined, extended structures as more metal–ligand units are added. The rate of crystal growth and the resulting size and quality of the MOF crystals can be controlled through various synthesis parameters, such as reaction time, temperature, and solvent selection. Together, these stages govern the overall properties of the MOF, including the porosity, stability, and surface area, which are critical for its performance in applications like catalysis, gas storage, and sensing.
The hard and soft acids and bases (HSAB) theory plays a significant role in guiding the design and synthesis of MOFs by providing a theoretical framework for understanding and predicting the interactions between metal ions and organic linkers. In essence, HSAB theory helps researchers optimize the choice of metal centers and organic ligands based on their “hard” or “soft” characteristics, which in turn affects the stability, functionality, and overall properties of the resulting MOF. For applications requiring hard or soft metals, corresponding hard or soft ligands will be selected to connect the metal centers and form stable frameworks. Similarly, for applications requiring hard or soft ligands, matching hard or soft metals will be chosen to ensure optimal compatibility and stability. The HSAB theory serves as a powerful guide for MOF design and synthesis by enabling the rational selection of metal nodes and organic linkers to optimize interactions based on their hard or soft characteristics.
The synthesis of MOFs is also governed by a delicate balance between dynamic processes and thermodynamic equilibrium. The dynamic of MOF synthesis involves kinetic processes such as nucleation, crystal growth, and reaction rates, while thermodynamic equilibrium dictates the stability of the final product and its structural phases. The challenge in MOF synthesis lies in balancing the dynamics and thermodynamics to achieve the desired structures and properties. In some cases, the kinetic pathway dominates, leading to metastable MOF phases that are kinetically trapped and may possess unique properties, such as open frameworks with high porosity but lower stability. In other cases, allowing the system to reach thermodynamic equilibrium results in more stable and crystalline MOFs with fewer structural defects. By adjusting synthesis parameters, researchers can manipulate both the dynamic and thermodynamic aspects of MOF formation.
2.1.1 Metal salt selection.
The choice of metal nodes is a critical factor in the design and synthesis of MOFs (Fig. 5). Metal nodes, which can be metal ions or clusters, serve as the central coordination points in MOFs, linking organic ligands into extended and porous structures. The selection of appropriate metal nodes significantly influences the structural, physical, chemical, and biological properties of MOFs, thereby determining their suitability for various applications. Main group metals (especially Mg, Ca, Al, In, etc.),51–54 fourth-period transition metals (particularly Ti, Cr, Fe, Co, Ni, Cu, Zn, etc.),55–61 fifth-period transition metals (notably Zr, Cd, etc.),62,63 and lanthanide metals64–66 are frequently employed as metal nodes.
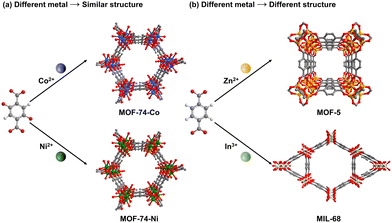 |
| Fig. 5 Metal selection influence on the structures of MOFs under similar conditions. Atom code: C, grey; O, red; H, white; Co, blue; Ni, green; Zn, yellow; In, light green. | |
Benefiting from the diverse functions of these metals, different metals are chosen based on desired properties:
• Structural property: metals like Zr, Hf, lanthanides, etc. are often selected to synthesize cluster-based MOFs.
• Physical stability: metals such as Zr, Al, Cr, Fe, etc. are usually used to construct highly stable frameworks.
• Chemical reactivity: metals like Fe, Co, Ni, Cu, etc. are frequently employed for catalytic applications.
• Biocompatibility: metals such as Mg, Ca, Fe, Zn, etc. are generally chosen for biocompatible MOFs.
Additionally, the combination of metal salts and ligands should adhere to the HSAB theory to optimize coordination and stability.67,68 As for the selection of the anions, the departure ability of the anions and the solubility in certain solvents are often regarded as the primary consideration.69,70
2.1.2 Ligand selection.
The choice of ligands is a pivotal aspect in the design and synthesis of MOFs (Fig. 6). Ligands can connect to the metal nodes to form the periodically extended network structures. The selection of appropriate ligands profoundly influences the framework's architecture, stability, porosity, and functionality, making it crucial for tailoring MOFs to target applications such as gas storage, catalysis, drug delivery, and sensing. Various ligands are often employed, mainly including the carboxylates, imidazolates, phosphonates, pyrazoles, triazoles, etc.71–75
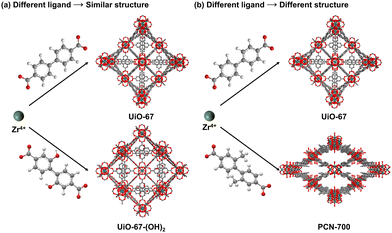 |
| Fig. 6 Ligand selection influence on the structures of MOFs under similar conditions. Atom code: C, grey; O, red; H, white; Zr, dark green. | |
Benefiting from the diverse choice of these ligands, different ligands are chosen based on desired properties:
• Architecture: ligands determine the connectivity and geometry of MOFs, mainly including the length, rigidity, number of joints, and functional groups of the ligands.
• Framework stability: the properties of the ligands affect the stability of MOFs. For example, ligands with robust chemical bonds can enhance the framework's resistance to hydrolysis, thermal degradation, and chemical attack.
• Variable porosity: the size and shape of ligands can be varied to tune the porosity of the MOFs, affecting its capacity for gas adsorption, separation, and storage.
• Functional property: ligands impart specific functionalities to MOFs with their target functional groups, which is beneficial to form excellent host–guest interactions in applications, for example, hydrogen bond, halogen bond, π⋯π interaction, etc.
Also, the choice of ligands should adhere to the HSAB theory to optimize the coordination interaction and enhance the stability. Furthermore, in some cases, the organic linkers will be transformed to their corresponding metal salts for better solubilities in certain solutions.76,77
2.1.3 Solvent selection.
The choice of solvent plays a crucial role in the synthesis of MOFs (Fig. 7). Solvents do not only act as the medium in which the reactants dissolve and interact but also influence the crystallization process, morphology, and properties of the MOFs. The selection of the appropriate solvent is essential for optimizing reaction conditions, achieving high-quality crystals, and tailoring the properties of the resulting MOFs for specific applications. Some commercial chemicals with great solubilities were used, including water, methanol/ethanol, N,N-dimethylformamide (DMF)/N,N-dimethylacetamide (DMA), chloroform, etc.78–83 Also, in many cases, the extra additions of acid or base can be beneficial for generating higher quality crystals or higher crystallization rates.84,85
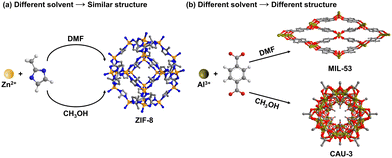 |
| Fig. 7 Solvent selection influence on the structures of MOFs under similar conditions. Atom code: C, grey; O, red; H, white; Zn, yellow; Al, dark yellow. | |
From the various solvents, there are some basic conditions for consideration:
• Solubility: solvents determine the solubility of metal salts and organic linkers, affecting the availability of reactants and the overall reaction kinetics. Suitable solvents can enhance the reactivity and facilitate the formation of desired coordination bonds.
• Saturation vapor pressure: the saturation vapor pressure of the solvent affects the pressure in the reactor, which further influences the reaction rate and the sizes and shapes of the products.
• Chemical compatibility: the solvent must be chemically compatible with the reactants and products, avoiding unwanted side reactions or decomposition.
• Environmental and safety issues: solvent toxicity, flammability, and environmental impact are also crucial factors. Green solvents such as water and ethanol are preferred for their lower environmental impact and safer handling properties.
2.1.4 Reaction condition selection.
The choice of reaction conditions can also influence a lot in MOF synthesis, mainly including the reaction temperature, reaction time, cooling steps, the synthesis methods, etc (Fig. 8).86–90 These parameters significantly influence the nucleation, crystal growth, and overall quality of the MOFs. Optimizing these conditions is essential for tailoring the structural, physical, and chemical properties of MOFs to meet specific application needs.
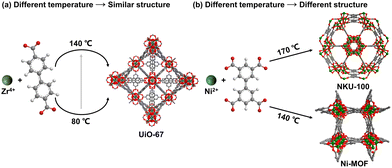 |
| Fig. 8 Temperature selection influence on the structures of MOFs under similar conditions. Atom code: C, grey; O, red; H, white; Zr, dark green; Ni, green. | |
From the various reaction conditions, there are some basic conditions for consideration:
• Reaction temperature: the synthesis temperature controls the kinetic energy of the reactants, influencing the rates of nucleation and crystal growth. Higher temperatures generally accelerate reaction rates and promote the formation of larger crystals, but they must be balanced to prevent decomposition of sensitive ligands or coordination structures.
• Reaction time: the duration of the reaction affects the extent of nucleation and crystal growth. Insufficient reaction time can lead to incomplete reactions and poor crystallinity, while excessive reaction time may cause overgrowth or degradation of the framework.
• Cooling steps: the rate at which the reaction mixture is cooled affects the crystallization process. Slow cooling allows for the formation of well-defined, high-quality crystals, whereas rapid cooling often results in smaller, less ordered structures.
• Synthesis methods: the synthesis methods generally include solvothermal method, microwave method, electrochemical synthesis, mechanical grinding, ultrasonic synthesis, and near-critical water synthesis. These methods can greatly affect the reaction time through the different energy intervention forms, which will be discussed in detail below.
• Activation methods: removing solvent residues from the pores of as-synthesized MOFs are crucial step for applications such as adsorption and catalysis. The solvent residues are typically trapped in the MOF's pores after synthesis, and removing them often involves solvent exchange and thermal activation. In solvent exchange, generally a more volatile solvent replaces the initial solvent within the pores, while thermal activation is typically performed by heating under vacuum. In some cases, supercritical CO2 drying is used to remove solvents without causing structural collapse, which usually happens with conventional drying methods. This process of activation is critical to achieving a fully open and accessible pore structure, maximizing the surface area and allowing the material to function effectively in its intended applications.
In summary, traditional design principles necessitate a deep understanding of coordination chemistry and crystallography, along with numerous pairing attempts and adjustments. This approach has led to the development of many novel coordination structures and porous frameworks.
2.2 Synthesis method
The synthesis of MOFs involves a variety of methods, each offering control over the framework's structure, property, and scalability. The primary synthesis methods mainly include solvothermal method, microwave method, electrochemical synthesis, mechanical grinding, ultrasonic synthesis, and near-critical water synthesis. The choice of synthesis methods for MOFs has profound implications for their design and application potential, as different methods directly influence the structural property, functionality, and scalability of the resulting materials. MOF design is intrinsically linked to the desired application, and selecting the appropriate synthesis approach is critical to tailoring specific properties like porosity, stability, and catalytic activity.
For instance, solvothermal method is widely used for producing highly crystalline MOFs with uniform pore structures, making them ideal for gas storage, separation, and catalysis. However, this method often requires high temperatures, long reaction time, and toxic solvents, posing challenges for scalability and environmental sustainability. Rapid synthesis techniques like microwave method, and ultrasonic synthesis have emerged as promising methods for producing MOFs quickly. These methods offer great potential for applications where time efficiency is critical, such as in commercial-scale production or the development of MOF-based sensors. However, controlling the morphology and ensuring the homogeneity of the materials synthesized through these faster methods can be difficult, which may affect their performance in applications that require precise structural control. Greener synthesis technique, such as mechanical grinding, offer more sustainable alternatives by reducing solvent usage and energy consumption, which is well-suited for applications where environmental impact and cost efficiency are critical, such as large-scale industrial processes and environmental remediation. Yet, achieving the same level of structural precision and crystallinity as traditional methods can be challenging, which may limit their application in areas requiring highly ordered frameworks, like selective catalysis or drug delivery.
Ultimately, the synthesis method must be carefully chosen to align with the desired application, as it affects not only the material's physical and chemical properties but also its feasibility for real-world use. Meanwhile, for energy-related applications, such as hydrogen storage or battery technologies, the synthesis process must prioritize high surface area, stability, and ease of mass production. Balancing these factors—efficiency, scalability, sustainability, and functional precision—remains one of the central challenges in optimizing MOF design for diverse applications.
2.2.1 Solvothermal method.
Solvothermal synthesis is the most common method for producing MOFs, where metal salts and organic linkers are dissolved in a solvent and heated in a reaction vessel to promote crystal growth. The particle size and shape can be regulated and controlled by adjusting the reaction temperature, reaction time, and cooling steps. Generally, solvothermal synthesis allows for high crystallinity and purity.91–94
Various reaction vessels are frequently used in experimental operations, mainly including glass vials, glass flasks, pressure-resistant bottles, Schlenk bottles, and Teflon autoclaves (Table 1). The primary advantage of glass vessels over Teflon autoclaves is their ability to facilitate real-time observation of crystal growth processes.
Table 1 Main pros and cons of different reaction vessels for MOF synthesis
Vessels |
Pros |
Cons |
Glass vial |
• Real-time observation |
• Low temperature and pressure |
• Cheap and convenient |
Glass flask |
• Real-time observation |
• Normally atmospheric pressure |
• Large-scale synthesis |
Pressure-resistant bottle |
• Real-time observation |
• Safety issue |
• Withstand high pressure |
Schlenk bottle |
• Real-time observation |
• Normally atmospheric pressure |
• Low humidity or oxygen-free environment |
Teflon autoclave |
• Withstand high pressure |
• Unobservable reaction process |
• High safety |
Glass vials with plastic lids are inexpensive but can hardly withstand temperatures over 100 °C and are prone to air leakage, resulting in low pressure. In contrast, vials with metal lids are more expensive but can endure higher temperatures.
Compared to other vessels, glass flasks are suitable for larger-scale synthesis, though they generally cannot be sealed for safety reasons, which highly limits the application range for the atmospheric pressure condition.
Pressure-resistant bottles offer a higher sealing capability and can withstand higher pressures, though the internal pressure must be estimated beforehand for safety, which requires a low liquid level height, high solvent boiling temperature, and low reaction temperature.
Schlenk bottles are used for some special reactions requiring low humidity or an oxygen-free environment, mainly involving some unstable metals or ligands. Also, this type of vessels mainly provides the atmospheric pressure condition.
Teflon autoclaves are among the most widely used vessels due to their excellent resistance to heat, acids, and alkalis, allowing them to withstand much higher temperatures and pressures. Besides, autoclaves are much safer due to the durable metal shell following standard operations. However, the device is relatively expensive and inconvenient.
2.2.2 Microwave method.
Microwave heating operates on the principle of dielectric heating, where the energy is absorbed by molecules in the reaction mixture, causing them to oscillate and generate heat through molecular friction. This process results in extremely fast and uniform heating, which is particularly beneficial for reactions that require precise temperature control. Microwave-assisted synthesis has emerged as a powerful and versatile technique in the field of chemical synthesis, particularly for the fabrication of MOFs. This method leverages microwave radiation to heat the reaction mixtures, offering distinct advantages over conventional heating methods. The rapid and uniform heating provided by microwaves can significantly shorten the reaction time from several days to several hours, improve product yields, and allow for better control over the physical properties of the synthesized materials, like the sizes and shapes. This technique enables the rapid screening of different metal–ligand–solvent combinations, accelerating the discovery of new MOFs with target properties and functionalities. Additionally, the ability to precisely control reaction conditions makes it possible to fine-tune the porosity, surface area, and stability of the resulting frameworks.95–97
2.2.3 Electrochemical synthesis.
Electrochemical synthesis of MOFs operates on the principle of electrolysis, where an electric current is applied to an electrolyte solution to drive the assembly of metal ions and organic ligands. This method allows for precise control over the concentration of metal ions, reaction kinetics, and deposition rates, leading to high-quality MOF samples. Electrochemical synthesis has gained prominence as an innovative and efficient method for the fabrication of MOFs, which utilizes an electrochemical cell to drive their formation, offering distinct advantages in terms of reaction control, purity of products, and environmental sustainability. During the synthesis process, metal ions are continuously supplied from the anode, enabling the controlled growth of MOFs with certain sizes and morphologies. Electrochemical synthesis has been successfully employed to produce a diverse range of MOFs with tailored properties for specific applications. This method is particularly advantageous for the synthesis of thin films and coatings of MOFs on various substrates, which are important for applications in catalysis, sensing, and energy storage. The ability to precisely control the deposition process makes it possible to engineer MOFs with desired morphologies and functionalities.98–100
2.2.4 Mechanical grinding.
Mechanical grinding has emerged as an innovative and sustainable alternative in MOF synthesis. This technique, a branch of mechanochemistry, involves the use of mechanical force to induce chemical reactions between solid reactants, typically using a ball mill or mortar and pestle. Mechanical grinding offers several advantages over conventional methods, such as reduced solvent usage, lower energy requirements, and shorter reaction times. Additionally, it often results in high product yields and can facilitate the synthesis of MOFs with unique properties unattainable through traditional routes. The mechanochemical approach for MOF synthesis is not only environmentally friendly but also opens new avenues for the design and discovery of novel materials. By leveraging the principles of green chemistry, mechanical grinding represents a paradigm shift towards more sustainable and efficient production of MOFs, aligning with the growing demand for eco-friendly and cost-effective manufacturing processes in materials science.101–103
2.2.5 Ultrasonic synthesis.
Ultrasonic synthesis also serves as a promising alternative technique for MOF fabrication, leveraging the power of ultrasonic waves to drive chemical reactions. This method, also referred to as sonochemistry, utilizes high-frequency sound waves to generate localized high-energy conditions through acoustic cavitation—the formation, growth, and implosive collapse of bubbles in a liquid medium. The extreme temperatures and pressures generated by this process facilitate rapid and efficient chemical reactions, often leading to the formation of MOFs in significantly shorter times and under milder conditions compared to solvothermal method. The cavitation effect not only accelerates the reaction kinetics but also enhances the uniformity and crystallinity of the resulting MOF structures.104–106
2.2.6 Near-critical water synthesis.
Near-critical water synthesis is a newly developed method. High-temperature water is being studied as a medium for processes such as organic reaction, waste destruction, and nanoparticle formation. This field arises because properties of water change dramatically as it approaches the critical point. For example, the dielectric constant decreases to values more typical of non-polar solvents, making organic compounds, such as the ligands used for the synthesis of MOFs, soluble. Additionally, the ionic product of the reaction solution increases by up to three orders of magnitude, reaching a maximum at around 280 °C, and creating a solvent with simultaneously raised concentrations of H+ and OH− ions. Furthermore, the water can be reused in the reaction without purification. If necessary, processes such as ion exchange can be employed to remove unreacted carboxylic acids and metal ions.107–110
In summary, the synthesis methods have effectively utilized various forms of energy intervention to achieve highly efficient energy usage, resulting in more efficient, cost-effective, and environmentally friendly synthesis processes (Fig. 9).
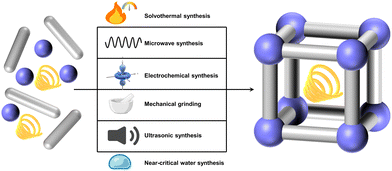 |
| Fig. 9 Classical synthesis methods of MOFs. | |
3. Functional oriented design and modification method
Functional oriented design involves the selection of appropriate metal nodes, organic linkers, and solvents for the MOF's structures, guided by the desired properties and applications, particularly in areas such as luminescence, chirality, catalysis, separation, storage and delivery, and electrochemistry. Modifications can be achieved through post-synthetic treatments, focusing primarily on the modification of metals (metal exchange and coordination modification), linkers (linker functionalization, linker scissoring, and linker exchange), and guests (guest exchange) (Fig. 10). These modifications enhance the MOF's target performance. By employing a functional-oriented design and modification strategy, researchers can optimize MOFs for specific tasks, creating bespoke materials that address complex challenges in various technological domains. This methodology not only broadens the scope of MOF applications but also paves the way for innovative solutions to contemporary scientific and industrial needs.
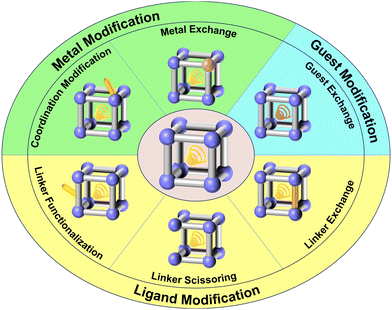 |
| Fig. 10 Classical modification methods for MOFs. | |
The HSAB theory and dynamics and thermodynamic equilibrium also play a significant role in the modification of MOFs, offering valuable insights into how different reagents interact with the metal centers and ligands within a MOF structure. By applying HSAB principles, researchers can design more post-modifications to tailor MOF properties for specific applications, such as catalysis, gas storage, and sensing. This understanding helps ensure that modifications are not only efficient but also result in stable, functional materials that perform well under the desired operating conditions. While for the dynamics and thermodynamic equilibrium, unlike the initial MOF synthesis, where the formation of coordination bonds and crystal growth are dictated by the primary synthesis conditions, the functional modification often involves chemical reactions or exchanges that occur within the pre-established framework. This requires careful control over both kinetic factors (reaction rates, diffusion of reactants) and thermodynamic principles (stability, equilibrium of products). The ability to control reaction rates, optimize conditions for achieving equilibrium, and ensure the long-term stability of modified MOFs opens new possibilities in tailoring these materials for complex tasks, such as catalysis, gas storage, sensing, and drug delivery.
3.1 Functional oriented design
Based on the accumulated experience from numerous syntheses attempts and studies of the relationships between the structures and the properties of MOFs, the functional oriented design of MOFs with targeted functions, or the modification of MOFs to achieve specific functionalities, has attracted great attention. This approach significantly expands the application prospects and reduces the time needed for exploration and development.
3.1.1 Luminescence.
The luminescent properties of MOFs make them highly attractive for applications in sensing, imaging, light-emitting devices, and photonics.111–115 These materials can exhibit diverse photophysical behaviours such as fluorescence, phosphorescence, and delayed fluorescence, depending on their composition and structure. By engineering the electronic environments of the metal nodes, the conjugation pathways of the organic linkers, and the specific properties of functional guests, researchers can create MOFs with targeted emission wavelengths and intensities tailored to certain needs.116–120
Additionally, luminescent MOFs offer unique advantages in terms of stability, porosity, and functionality compared to traditional luminescent materials. Their porous nature allows for guest encapsulation, which can lead to enhanced luminescent properties or novel sensing mechanisms. The modular design of MOFs also enables the incorporation of multiple luminescent centers within a single framework, facilitating multifunctional applications.121–125
Due to the abundant combinations of metals, ligands, and guests, MOFs can possess various luminescence sources and multiple luminescence centers, simultaneously. Metals such as Cu(I), Ag(I), Eu(III), and Tb(III) are frequently employed as luminescent centers, while other metals that do not exhibit strong competitive absorption of excitation light, like Mg(II), Zn(II), Al(III), and Zr(IV), are used as metal nodes to link luminescent ligands. Ligands with highly conjugated structures or functional groups, such as pyrene rings and amino groups, are also often used as luminescent sources. As for the guests, metal ions like Eu(III) and Tb(III), coordination units such as [Ir(ppy)2(bpy)]+, and luminescent dyes can serve as additional luminescent centers within MOFs (Fig. 11).126–130 These diverse components contribute to the versatile luminescence properties of MOFs, enabling their use in a wide range of applications. Besides the direct synthesis to introduce luminescence centers into MOFs, nearly all the classical modification methods can bring the luminescence centers into MOFs, which enriches the luminescence sources and makes it easy for MOFs to possess multiple luminescence centers.
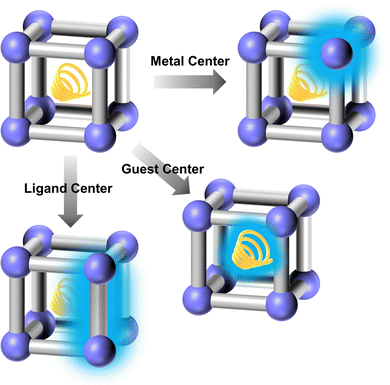 |
| Fig. 11 Various luminescent centers in MOFs. | |
3.1.2 Chirality.
Chirality, a concept widely recognized in organic chemistry, extends its intriguing characteristics to MOFs, creating a fascinating intersection of geometry, symmetry, and functionality. The chirality in MOFs can arise from several sources, including the inherent chirality of the organic linkers, the chiral arrangement of the framework itself, or the incorporation of chiral guests within the pores. This structural handedness imparts unique properties to chiral MOFs, such as the ability to differentiate between enantiomers of a compound, a feature highly desirable in pharmaceuticals, pesticides, and fine chemicals.131–135
Understanding and harnessing the chirality of MOFs involves a multidisciplinary approach, integrating principles from coordination chemistry, crystallography, and materials science. Researchers are continually developing new synthetic strategies to design and construct chiral MOFs with tailored properties, aiming to exploit their chiral nature for practical applications, such as the enantioselective recognition, separation, asymmetric catalysis, and nonlinear optics. This field is not only rich in fundamental scientific inquiry but also holds promise for innovative solutions to complex industrial challenges.136–138
The introduction of chirality into MOFs is a vibrant area of research that combines the precision of chemistry with the ingenuity of materials science. While several methodologies are employed to introduce chirality into MOFs, each leveraging different aspects of chemical design and synthesis. The most common approach is the use of pure chiral ligands during the MOF synthesis, while the organic molecules that are inherently chiral. These chiral ligands can transfer their chirality to the entire framework, resulting in chiral MOF structures. Another method involves the incorporation of achiral ligands in a way that induces a chiral arrangement within MOFs. This can be achieved through the choice of specific metal centers and reaction conditions that favour the formation of chiral frameworks. Post-synthetic modification is another powerful strategy, where an initially achiral MOF is functionalized with chiral molecules, thereby imparting chirality to the framework, which mainly includes the coordination modification to the metal nodes, the chiral linker functionalization and exchange to the ligands, and the ion/molecule exchange to the guests. Additionally, templating methods, where chiral molecules or ions are used as templates during the synthesis, can also guide the formation of chiral MOFs by influencing the spatial arrangement of the building blocks (Fig. 12).139–145
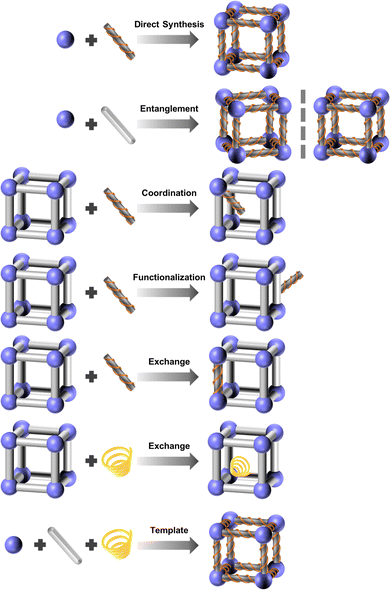 |
| Fig. 12 Classical construction methods for chiral MOFs. The original guests in the framework have been omitted for clarify. | |
The precise control over the chirality of MOFs through these methods not only enhances our understanding of chiral materials but also creates new avenues for practical applications. By tailoring the synthesis process, researchers can design chiral MOFs with specific pore sizes, shapes, and functionalities, enabling their use in a wide range of advanced technological applications.
3.1.3 Catalysis.
MOFs have emerged as a versatile class of materials in the field of catalysis, offering a unique platform for facilitating a wide range of chemical reactions, which exhibit many attributes that are particularly advantageous for catalysis. The large surface area and porosity allow for the accommodation and diffusion of reactant molecules, enhancing the contact between reactants and active sites. The abundant potential open metal sites and switchable dynamic metal sites also give rise to the sufficient opportunities for contact. The modular nature of MOFs indicates that their components can be precisely designed and synthesized to incorporate specific catalytic sites, such as metal ions, metal clusters, or functionalized organic linkers. This design flexibility enables the creation of MOFs tailored for specific catalytic processes, ranging from organic transformations and gas adsorption to photochemical and electrochemical reactions.146–150
Besides, the MOF catalysts possess the potential for heterogeneity and recyclability. Unlike many traditional homogeneous catalysts, MOFs can often be recovered and reused without significant loss of activity, offering economic and environmental benefits. Additionally, the stability of MOFs under various reaction conditions, including high temperatures and acidic or basic environments, further extends their applicability in industrial processes. Researches into MOF-based catalysis are continuously evolving, with innovative strategies being developed to enhance the performance and scope of these materials. The confluence of these advancements positions MOFs as a promising frontier in catalysis, with the potential to revolutionize processes in chemical manufacturing, environmental remediation, and sustainable energy solutions.151–154
Many functional metals and groups have been widely introduced into MOFs both through direct synthesis and the post-synthetic modification, mainly through the coordination modification and the guest exchange methods, especially for the transition metal elements Fe, Co, Ni, Cu, Ag and functional linkers porphyrin and phthalocyanine. The abundant and highly adjustable metal sites for MOFs give rise to the efficient constructions of novel catalysts for certain usage.155–160
3.1.4 Separation.
By exploiting precise control through direct synthesis or modification of pore dimensions, pore shapes, and surface functionalities, MOFs can selectively capture and separate specific ions or molecules from complex mixtures in both liquid and gaseous states, which highly relays on the pore size and inner chemical environments. This capability offers significant advancements in applications ranging from environmental remediation, clean energy production to industrial purification and biochemical processing.161–165
There are two main research directions in this field: energy utilization and environmental protection. For energy utilization, MOFs have shown great potential in separating petroleum products, including gaseous products like low-chain alkanes, olefins, and alkynes, as well as liquid products such as C6, C7, and C8 mixtures, and downstream products like xylene (Table 2). Additionally, crucial metal resources, such as Li+, Co2+, Ni2+, and Ln3+, can also be efficiently separated using MOFs.166–170
Table 2 Xylene separation abilities of selective MOFs in vapor phase
MOF |
Selectivity |
Ref. |
MAF-X8 |
p- > m- > o- > eb |
166
|
Mg-CUK-1 |
p- > o- > m- |
167
|
sql-1-Co-NCS |
o- > p- > m- > eb |
168
|
MIL-101 |
o- > eb > m- > p- |
169
|
Co2(dobdc) |
o- > eb > m- > p- |
170
|
ZU-61 |
o- > m- > p- |
171
|
MFM-300 |
m- > o- > p- |
172
|
For environmental protection, MOFs have demonstrated excellent separation effects for a series of toxic gases, ions, and persistent organic pollutants. Examples include SO2 and NO2 in automobile exhaust (Table 3), heavy metal ions in water environments, and perfluorinated compounds and dyes in natural environments. As the field continues to evolve, the integration of MOFs into separation technologies promises to enhance efficiency, reduce energy consumption, and contribute to more sustainable practices across diverse industries.173–177
Table 3 SO2 and NO2 adsorption capacities of selective MOFs at 1 bar and 298 K
MOF |
Capacity (mmol g−1) |
Ref. |
[Ir]@NU-1000 |
SO2 10.9 |
178
|
Ni(bdc)(ted)0.5 |
SO2 10.0 |
164
|
SIFSIX-1-Cu |
SO2 11.0 |
176
|
MFM-601 |
SO2 12.3 |
179
|
MIL-101(Cr) |
SO2 18.4 |
180
|
MFM-170 |
SO2 17.5 |
181
|
MFM-300-Sc |
SO2 9.4 |
182
|
ECUT-111 |
SO2 11.6 |
183
|
MFM-300-Al |
NO2 14.1 |
184
|
MFM-300(V) |
NO2 13.0 |
185
|
3.1.5 Storage and delivery.
MOFs have garnered significant attention in the fields of storage and delivery due to their unique structural and pore properties, which make MOFs ideal candidates for the efficient storage and delivery of a wide range of substances, including gases, liquids, and certain therapeutic agents, through guest exchange processes,186–190 which highly relays on the pore volume and inner chemical environments.
In the context of gas storage, MOFs offer solutions for storing and delivering hydrogen, methane, and other gases with high volumetric and gravimetric capacities, presenting promising advancements for clean energy applications. Additionally, MOFs demonstrate superior performance in storing and releasing volatile organic compounds, which is crucial for environmental monitoring and industrial processes. MOFs’ ability to encapsulate and release drugs in a controlled manner also holds significant potential for targeted drug delivery systems, improving the efficacy and reducing side effects of various treatments.191–195
As research progresses, the integration of MOFs into storage and delivery systems promises to revolutionize various industries by enhancing efficiency, increasing capacity, and providing more sustainable and controlled methods of substance management. The versatility and adaptability of MOFs underscore their potential to address contemporary challenges in energy storage, medical treatments, and environmental protection, heralding a new era of advanced materials science.
3.1.6 Electrochemistry.
MOFs have emerged as a highly promising class of materials in the field of electrochemistry, offering transformative potential for a range of applications, including energy conversion, energy storage, and energy applications.
In energy conversion, MOFs offer significant potential to enhance the efficiency, selectivity, and sustainability of various electrochemical reactions, especially in oxygen evolution reaction (OER), hydrogen evolution reaction (HER), oxygen reduction reaction (ORR), and carbon dioxide reduction reaction (CO2RR), which convert the electrical energy into chemical energy. Target metals are frequently employed as the catalyst sites, including Fe, Co, Ni, Cu, and In.196–200
In energy storage, MOFs have shown great promise in the development of advanced batteries and supercapacitors. Their high porosity and customizable surfaces enable the efficient storage and rapid transfer of ions, leading to improved charge/discharge rates and increased capacity. MOFs are also being explored for use in fuel cells and electrolysers, where their structural versatility allows for the optimization of catalytic processes, enhancing the overall efficiency of energy conversion and storage.201–205
As for the energy applications, MOFs are making significant strides in the field of electrochemical sensing and environmental monitoring. The precise control over their chemical functionalities and pore environments allows for the selective detection of various analytes, including gases, ions, and organic compounds, with high sensitivity and specificity.206–210
As researches continue to advance, the integration of MOFs into electrochemical applications promises to drive significant improvements in performance, sustainability, and functionality. This integration not only opens new avenues for energy storage and conversion but also enhances capabilities in environmental sensing and beyond, marking a pivotal step forward in the application of advanced materials in electrochemistry.
3.2 Modification method
Post-synthetic modification involves the deliberate alteration of the MOF's structure and functionality based on its initial synthesis, which allows for the exchange of metal centers, introduction of new functional groups, or the alteration of the guests within the MOF framework without disrupting its overall architecture. Target-guided modification provides a versatile toolkit for tailoring the physical and chemical environment within the pores, thus optimizing MOFs for specific applications. Post-synthetic modification allows researchers to introduce new functionalities, improve stability, or adapt the structural features of a pre-synthesized MOF without altering its underlying framework. This capability is especially valuable for applications in gas storage, catalysis, drug delivery, and sensing, where specific properties such as pore size, chemical reactivity, or surface hydrophilicity are critical. For example, modifying the metal center can enhance catalytic efficiency or improve sensing capabilities, while the modification towards the linker can alter the physical and chemical environment of the framework and pores, affecting gas storage, catalysis, and sensing performance. Additionally, the modification to the guest molecules can also influence the pore environment, leading to changes in storage capacity and delivery efficiency.
3.2.1 Metal exchange.
Metal exchange on the framework of MOFs involves replacing the metal ions within the pre-formed framework with different metal species, which mainly involves fourth row transition metals and lanthanide metals, respectively (Fig. 13). This process normally can be performed under relatively mild conditions, preserving the integrity of the MOF structure while enabling the selective substitution of metal ions, which can significantly alter the structural, electronic, and catalytic properties of MOFs, thereby opening new possibilities for customization and application-specific optimization. Metal exchange is a post-synthetic modification strategy that enables researchers to fine-tune the functionality of MOFs without the need to synthesize entirely new frameworks from scratch.211–215
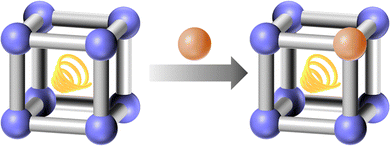 |
| Fig. 13 Metal exchange process for MOFs. | |
Metal exchange greatly expands MOFs with various properties and functions. It allows for the introduction of metals which may be challenging to incorporate during the initial synthesis due to incompatibility with the organic ligands or reaction conditions. Additionally, metal exchange can be used to create multi-metallic MOFs, which can exhibit synergistic properties that are not attainable with single-metal frameworks. This flexibility is particularly beneficial for catalytic applications, where the nature of the metal center plays a crucial role in determining the activity and selectivity of the catalyst.
3.2.2 Coordination modification.
Coordination modification involves altering the coordination environment around the metal nodes or clusters within MOFs with potential open metal sites after its initial synthesis (Fig. 14), which can significantly impact the physical and chemical properties of MOFs, allowing for fine-tuning of its functionalities to meet specific application requirements. This process can also be performed under relatively mild conditions, preserving the integrity of the MOF structure. By modifying the coordination sphere, researchers can introduce new reactive sites, adjust the electronic properties, and improve the stabilities of MOFs.216–220
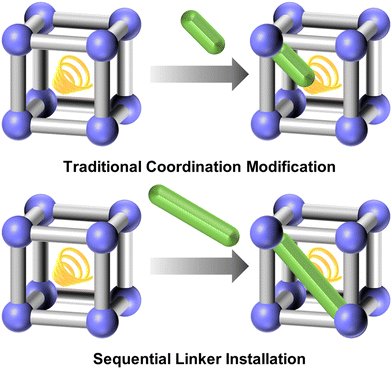 |
| Fig. 14 Coordination modification process for MOFs. | |
The advantages of coordination modification are numerous. It offers a versatile approach to introduce functional groups that may not withstand the conditions of direct MOF synthesis. This method enables the precise control of the metal–ligand interactions and the creation of coordinatively unsaturated sites, which are essential for catalytic activities and enhanced adsorption properties. Coordination modification also facilitates the incorporation of multiple types of ligands, leading to multifunctional MOFs with synergistic properties.
Traditional coordination modification often involves one-end coordination to the metal nodes. Recently, the sequential linker installation method, which aims at dual-end coordination, has attracted great attention due to their highly defined position and the great stability of the installed linkers. By enabling the precise adjustment of the organic linkers within the framework, this method allows for the creation of highly specialized MOFs tailored to meet the demands of advanced technological applications.221–225
3.2.3 Linker functionalization.
Linker functionalization involves the post-synthetic modification of the linkers within the MOF structure. This process allows for the introduction of various functional groups into the framework, tailoring the physical and chemical environment within the pores without altering the overall architecture of the MOF. By modifying the linkers, researchers can significantly regulate the properties of the MOF, including its reactivity, stability, and interaction with guest molecules. Generally, there are three main pathways for linker functionalization: organic reactions,226–230 coordination interactions,231–233 and partial exchanges234,235 (Fig. 15).
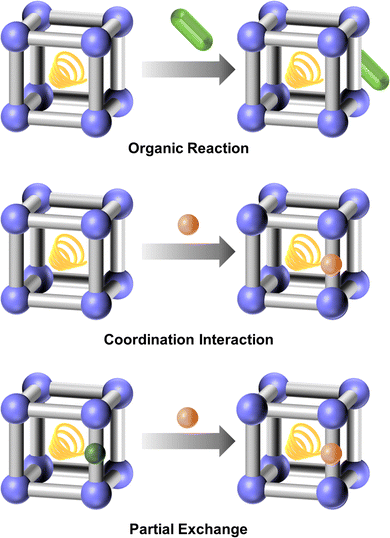 |
| Fig. 15 Linker functionalization process for MOFs. | |
For MOFs with certain functional groups, such as aldehyde group, amino group, carboxyl group, double bond, and triple bond, organic reactions can introduce molecules with complementary functional groups to enhance specific properties and functions. In MOFs that possess ligands with potential coordination sites, like carboxyl groups, hydroxyl groups, and nitrogen-containing heterocyclic units (pyridine, pyrazine, piperazine, etc.), metals can be attached to the ligands through coordination interactions. Additionally, for MOFs whose ligands contain coordination units, such as metal–porphyrin and metal–phthalocyanine, the metals within these ligands can be partially exchanged with other metals.
The advantages of linker functionalization are numerous. This technique enables the precise incorporation of functional groups that may not survive the harsh conditions of direct MOF synthesis, thus expanding the range of achievable modifications. Functionalized linkers can enhance the MOF's ability to interact with specific molecules, improving its performance in applications such as catalysis, where active sites are crucial, or in gas storage, where selectivity and capacity are paramount. Moreover, functionalization can be used to introduce responsive or stimuli-sensitive groups, creating smart materials that react to environmental changes. This functionalization strategy enables precise control over the physical and chemical environment within MOFs, facilitating the development of materials with tailored properties for advanced applications.
3.2.4 Linker scissoring.
Linker scissoring involves the post-synthetic cleavage of the organic linkers within the MOF structures (Fig. 16), which strategically breaks the linkers, altering the framework's connectivity and porosity without destroying the MOF architecture completely. By selectively scissoring the linkers, researchers can introduce novel properties and functionalities, create porous defects, or modify the pore structures, significantly influencing the material's properties and enhancing its performance in various applications.236–240
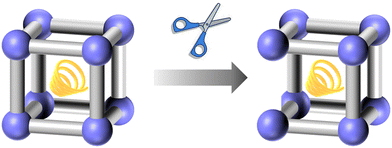 |
| Fig. 16 Linker scissoring process for MOFs. | |
Pathways for linker scissoring mainly include chemical cleavage, where specific chemical reagents are used to break the linkers; photochemical scissoring, which uses light to induce cleavage; and mechanical methods, such as applying pressure or shear forces. Each method offers unique advantages and can be tailored to achieve the desired modifications while maintaining the overall integrity of the MOF structure.
The usage of linker scissoring allows for the generation of open metal sites and the creation of hierarchical porosity, which can improve the accessibility of active sites and enhance the material's catalytic activity. This pathway also enables the introduction of functional groups at the scission sites, facilitating further chemical modifications. Additionally, linker scissoring can be used to modulate the mechanical flexibility and stability of MOFs, making them more suitable for specific applications.
3.2.5 Linker exchange.
Linker exchange involves the post-synthetic substitution of the organic linkers within a MOF structure with different linkers (Fig. 17), which allows for the introduction of new properties and functionalities without the need to synthesize new structures. The pathway of implementing this route is simple and facile, usually achieved by heating and soaking in the solvent or vapor phase of target chemicals, which provides specific advantages depending on the desired modification and the structural stabilities of MOFs.241–245
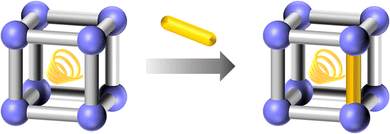 |
| Fig. 17 Linker exchange process for MOFs. | |
The advantages of linker exchange are obvious. It offers a flexible approach to modify the properties and functions of MOFs, enabling the incorporation of functional groups that may not withstand the conditions of direct synthesis. This technique can also improve the material's stability and robustness, enhance its adsorption capacity, and introduce reactive sites for catalysis. Additionally, the linker exchange method allows for the development of multifunctional MOFs with synergistic properties by combining different types of linkers within the same framework.
3.2.6 Guest exchange.
Guest exchange involves the post-synthetic replacement of ions or molecules within the pores of MOFs with different guest species (Fig. 18). This process can be performed under relatively mild conditions which allows for the dynamic modification of the MOF's properties without altering its fundamental structure. By exchanging guest molecules, researchers can fine-tune the MOF's pore environment, optimize its performance for specific applications, and introduce new functionalities.246–250
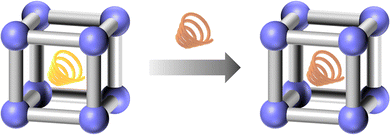 |
| Fig. 18 Guest exchange process for MOFs. | |
Guest exchange provides a flexible approach to modify the internal environment of MOFs, enabling the introduction of various functional groups or active species that can be incompatible with direct synthesis. Guest exchange can generally enhance the material's stability, reactivity, and selectivity, making it more effective for specific applications such as molecular sensing and catalysis, where the presence of target guest molecules can significantly influence catalytic activity. Additionally, guest exchange can be used to create responsive MOFs that can adapt to different environmental conditions or stimuli.
4. AI methodologies and AI-guided synthesis
The recent technological advancements in ML and AI have sparked a proliferation of new systems and methodologies across various fields. From conversational agents like ChatGPT to breakthroughs in sports analytics,251 medicine,252 and beyond, AI has become an indispensable tool for predictions, information gathering, complex calculations, and other critical applications. In chemical research, ML models harness the power of AI and vast datasets to develop increasingly accurate predictions. These predictions can be experimentally validated, with the resulting data fed back into the models to further refine their accuracy. By identifying patterns in massive datasets that would be impractical for humans to detect within a similar timeframe, ML and AI allow scientists to focus their efforts on laboratory testing and validation of model-generated insights, thus accelerating the pace of discovery and innovation.253
4.1 AI methodologies
Before diving into how ML and AI can be used to help chemists perform more efficient research, it is crucial to understand how the ML models are developed, and how they use established databases to grow their algorithm. In this section, a general overview of database establishment for ML models, as well as training ML models to develop algorithms and perform better calculations and predictions will be presented in the context of MOF research.
4.1.1 Database establishment.
To build a ML model, the system must first be fed up with data that is specific to the task which the user requires the model to perform. Instead of just explicitly coding a set knowledge base, ML allows for more fluid logic from a system by enabling the system to learn relationships through observing the data it is fed up with and finding patterns in it. This means that as more relevant data is given, not only successful trials but also failed trials, the model is able to offer better predictions.254 Database establishment is a crucial step in the process of making a functional ML model, and greatly helps the training processes. Once the model has been trained, it will then be validated to ensure accuracy before being used to solve tasks.
The four types of data that a database can be composed of are structured, unstructured, semi-structured, and metadata (Fig. 19).255 As the name suggests, structured data is highly organized. Think of an excel spreadsheet of addresses, with each column having one piece of the address. This type of data is considered structured and easy to handle by a ML model. Unstructured data does not have a predefined format, and therefore is much more difficult for the model to consume. Most normal internet media like images, portable document formats (PDFs), and word documents are considered unstructured. Semi-structured databases are not as structured as a structured database, but do have some organization pattern, such as hyper text markup language (HTML) data. Metadata is not a type of raw data, but instead is the extracted key points from a full data set. Metadata presents the most important information for the user, without diluting it with the full data set.255 Framing these types of data into the context of MOF research and database establishment, if the ML model retrieves its data from PDFs of journal manuscripts or raw experimental data, it would be considered as an unstructured database, but if it retrieves the data from the Cambridge Structural Database (CSD) or other ordered database, it would be considered metadata. Once the data has been selected, it is classified as either training data or testing data. As the names suggest, training data is used to develop and optimize the ML models, while testing data is then used to evaluate whether the model can correctly assess a problem and produce a reasonable outcome.256
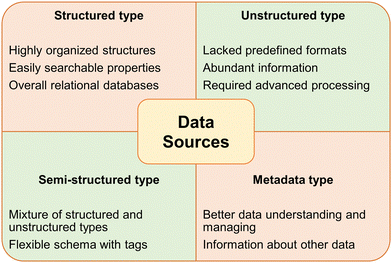 |
| Fig. 19 Four types of data for machine learning. | |
Due to the nature of chemistry, the information is often not in a format that is consumable by the ML model. For this reason, the data must first be refined to ensure it is in a language that the model can understand. Many different works may synthesize the same MOF structure, but there could be inconsistencies, missing data, or errors in some of the works. For this reason, all of the data for a single MOF must be compiled and refined into a single dataset, which can help make the model more accurate.257 Furthermore, each MOF structure must be simplified into a Simplified Molecular Input Line Entry System (SMILES), which is a language that can be understood by a ML model, and all other forms of media is translated in a similar way.258
There is an array of currently available MOF databases to be used as training and testing data for a ML model, including CoRE MOF, SynMOF, and CSD. CSD is the largest and most popular structural database available, containing not only MOF and other material structures, but also small molecules and other types of experimentally determined three-dimensional structural information, numbering over 1.4 million structures and including about 70
000 MOF structures.259,260 Many journals even require a submission of any crystallographic information file (CIF) to the CSD before publishing a manuscript, making the database a rich source for MOF data, and there are multiple examples of MOF databases built from CSD data.261,262 While CSD is a rich resource for crystal structures, it is not exclusive to MOFs and so it would be counterintuitive to train a model using the full database. By filtering the CSD down while only MOF data is included, ML models are able to efficiently gather the data needed to run whatever task is required. There is even a subset data package within the CSD specifically for MOF data.263
CoRE MOF is a large database with over 14
000 MOF structures.258,264 In some structures, bound solvent molecules can affect the structure or can be a desired use for some materials. For this reason, the database is separated into free solvent removed (FSR) and all solvent removed (ASR), allowing a ML model to draw from whichever data type it requires.258 This database also includes searches for different types of organic linkers, possible open metal sites, as well as calculations for the structures. The CoRE MOF database draws from the CSD and supplements any missing structures by using Web of Science and manually imported CIFs. The data is then refined to be ready for computations, allowing it to be directly used as a source for a ML model. This makes CoRE MOF a ready-to-use database for ML and has been used for MOF screening purposes.265–267 CoRE MOF has even been used as a training database for a ChatGPT-type program that utilizes large language models, called ChatMOF.268 CoRE MOF has also been used to establish specific database for synthesis predictions, namely SynMOF. By extracting the synthesis information (metal, linker, time, temperature, solvent, and modulator) for the MOFs contained in the CoRE MOF database and combining it with the structural information contained in the CSDs, SynMOF is able to predict the synthesis conditions required for theoretical MOF structures.269 By utilizing SynMOF, it is possible to go from a predicted structure to the synthesis conditions required to generate it, enabling chemists to better determine the synthesis conditions, and saving time and chemicals. Another popular database that utilizes CoRE MOF data is MOFX-DB.270 A unique feature of MOFX-DB compared to the previous databases is that it includes both experimentally discovered and hypothetical structures retrieved from ML models for both MOFs and zeolites. MOFX-DB combines data from CoRE MOF,258 hypothetical MOF (hMOF),271 and ToBaCCo272 for MOF data, and IZA273 and PCOD-syn274 for zeolite structures. MOFX-DB also includes the calculated adsorption data for the MOFs, totaling over 160
000 MOFs, as well as all of the necessary data to reproduce the calculated data. This makes MOFX-DB a rich source for ML training, especially for finding ideal adsorbents.
4.1.2 Algorithm and model.
Once the database has been established, the data can be used to train a ML model. There are four main ways to train a ML model: supervised, unsupervised, semi-supervised, and reinforcement learning, based on whether the data received by the system is labeled or not (Fig. 20).255 In supervised learning, the output data is labeled by a supervisor, usually a human, which is an explanation of the input data. The combination of the input and its corresponding labeled output explanation forms a training example, and a collection of these examples constitutes the training dataset. The presence of the output explanation can be crucial for advancing the logic of the ML model, and since it is labeled by a human, the model gets a head start in understanding the logic it should apply. The two main types of supervised learning models are regression models and classification models.275
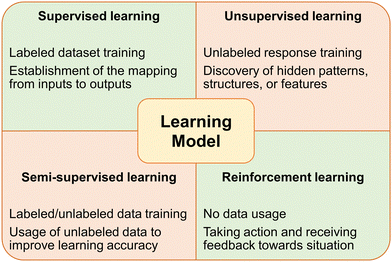 |
| Fig. 20 Four main ways to train machine learning model. | |
Unsupervised learning is defined if the output data is not manually tagged before being used for training. A lot of times, the volume of data is too large to be tagged manually, or the data cannot be tagged due to the type of data it is. When a ML model encounters this type of data, the goal is to extract trends or an underlying structure from the database. Due to the volume of most contemporary databases, ML relies heavily on unlabeled data and the trends the system can gain from it. It is possible for deep learning algorithms to be produced from unsupervised data, allowing for training from a large database, and increasing the potency of the deep learning algorithm.276
Sometimes, however, a supervisor is able to add a small sample of labeled to the system, giving the system a better understanding of how to classify the data it receives. When this method is applied, it is called semi-supervised learning, which has advantages over the previous two types. Semi-supervised learning systems are able to take unlabeled data, and by using the classification logic established by the labeled data, can classify this data in a way that the supervised system cannot. Furthermore, semi-supervised systems require less supervision, because they can become increasingly independent as the system is fed up with more unlabeled data and refines its logic.255,275
The final type of ML technique is reinforcement learning, which does not rely on existing data. Similar to classical reinforcement learning, the system observes an input state and decides to perform a certain action based on the observation. If the action is correct, the system is ‘rewarded’, while if the action is incorrect, the system is ‘reinforced’. The information about the relationship between the state and correctness of action is then stored and used as learning for the next state. As more input states are observed, the system is able to fine-tune its decision making due to the reward/reinforcement based on the action the system performs.255,275
There are many different types of computational models that can be used for the designs and predictions of MOFs, such as Grand Canonical Monte Carlo (GCMC), regression model, convolutional neural network, and graph neural network for studies, creating a toolbox for a variety of purposes. GCMC is a model for studying systems in equillibrium and analyzing related properties. It is widely employed in MOF chemistry to simulate the adsorption properties of materials. By calculating the energy exchange and particle exchange between different states of a material during adsorption, it is possible to determine which molecule gets adsorbed best, as well as the possible mechanisms of adsorption, by comparing the energy strain of each adsorption state of the material. Furthermore, the heat of adsorptions for different molecules can be calculated within a specific MOF structure.277 Two types of potential energies are usually calculated for GCMC adsorption calculations, one is based on guest–guest interactions and the other is based on host–guest interactions using the force field of atoms in the MOF. A force field provides an approximate representation of the interactions between atoms, serving as a key metric for describing the potential energies of these interactions.278 By pairing GCMC calculations with ML algorithms, it is possible to quickly screen a large sample of MOF structures, theoretical and experimental, to determine which has the best simulated adsorption capabilities for a certain substance or mixture.279–281
Another computational model that is commonly used for adsorption studies is the Gaussian Process Regression (GPR) model. GPR tends to require less data for training compared to other methods. Usually for adsorption data, temperature and pressure of the system, combined with the pore volume and surface area of the MOF are the parameters set for regression modeling.282 Other parameters can be used for other types of predictions, such as catalysis, where the pH and reactant and catalyst concentrations can be used as parameters to determine which catalyst works best for a certain pollutant. GPR is especially helpful when a non-linear relationship between input and output is present, due to the ability to have multiple kernel functions within the algorithm allowing for increased flexibility, especially when resolving uncertainties in the data. Kernel functions are multidimensional functions that quantify similarities between two pieces of data in a certain abstract data space. With a set number of parameters, no matter the size of the data space, the kernel functions can be compared to see which works best for a certain data set.283 These qualities allow GPR models to be powerful and versatile modeling tools.
With the information resulted from previous mentioned models, deep learning neural networks algorithms can be further utilized, specifically convolutional neural networks (CNNs) and graph neural networks (GNNs). By taking the calculations from GCMC or other models, and using crystal graphs, which is a representation of crystal materials that the network can understand, CNNs are able to be trained to have optimized parameters, allowing them to automatically describe the properties of crystalline materials. CNN doesn’t necessarily require fine granularity in the input data, which can increase the computational efficiency and improve the model generalization by identifying broader, more generalizable patterns in the data. Using crystal graphs, CNNs are able to predict material properties using the data they were trained on, giving CNNs flexibility similar to GPR models. By discerning the training data for a specific purpose, the researchers are able to make CNN models predict a vast array of MOFs’ performance for specific tasks, such as gas storage or separation.284–286 CNNs have even been shown to be able to characterize MOF structures from experimental X-ray diffraction patterns after being trained on mostly theoretical data.287 The other main type of neural network is the GNN. CNNs are mostly used for image-based data, including crystal structure data, while GNNs are better for graph related tasks, such as atomistic material representation.288 In order to train a GNN, the MOF structure must be portrayed in a graphical representation by turning the atoms into nodes and the bonds as edges. The nodes are labeled as a certain element by assigning input node features such as electronegativity, location on the periodic table, radius, etc. This atomistic graph is then converted into a line graph and then the training data is run with a goal of minimizing the error, usually mean squared error. Once again, based on the parameters of the training data, the GNN can be tuned to look for a specific purpose in the MOF structures, such as gas adsorption based on surface area and pore size, or catalytic efficiency based on band gaps and other desired properties. It has also been shown that traditional GCMC calculations are able to be used as a comparison to the GNN predictions, ensuring accuracy of the trained model.289
There are also examples of large language models being combined with ML algorithms to develop smart assistants for MOF discovery and optimization.290,291 These smart assistants are able to effectively gather information and screen large quantities of data to answer an input task much quicker than just using conventional computational methods such as GCMC. By combining conventional computational methods with ML models, chemists are able to quickly screen possibilities, allowing them to spend more time in the lab testing the output from the models.
4.2 AI-guided synthesis
The structure and application of MOFs can be finely adjusted by altering the metal nodes, organic linkers and the reaction conditions. This high degree of tunability poses a challenge for researchers seeking the most suitable MOF for specific applications under optimal synthesis conditions. AI offers a promising solution to streamline this process by accelerating the screening of optimal MOFs based on structure and functionality. This chapter explores AI-guided enhancements in synthesis conditions and the design of functional structures, as well as AI-guided approaches to innovate new MOF designs and synthesis methodologies.
4.2.1 AI-assisted synthesis optimization.
The synthesis of MOFs involves the self-assembly of metal nodes and organic linkers. Various synthesis methods have been developed, while multiple parameters within the synthesis conditions can be tuned, significantly influencing the crystallinity, morphology, and surface area of the final MOF products. These parameters include the choice of solvents, pH value of the solution, concentrations of metals and organic linkers, type and amount of additives, reaction time, temperature, pressure, and more. Screening optimal conditions can be a time-consuming, labor-intensive, and costly process. ML and AI offer a promising solution to reduce the effort in condition screening and facilitate the novel synthesis of pristine MOFs.
ML model can be trained using the SynMOF database, which was generated through automatic data extraction from the CoRE MOF database and literatures, recording six parameters, including metal source, linker, solvent, additive, synthesis time, and temperature, for the recorded MOFs. A web tool was developed based on this model, which, when given the CIF file of a MOF structure, can predict synthesis conditions including temperature, reaction time, solvent, and additives. This model demonstrates better performance compared to experts’ general intuition. Although the model provides only basic predictions of the synthesis conditions without detailed information such as reactant concentrations and additive amounts, it highlights the potential of this tool to accelerate the optimization of the MOF synthesis process.269 Genetic algorithm (GA) can be used to optimize the experimental synthesis conditions of HKUST-1, while nine different synthesis parameters, including volume of water, dimethylformamide, methanol, ethanol, isopropanol, ratio of reactants, reaction temperature, microwave strength and reaction time, were studied. With over 120 conditions tested, the importance between the nine parameters can be determined and a better weighted calibration can be introduced to enhance the algorithm. The algorithm was also applied to Zn-HKUST-1, which didn’t yield crystalline MOF with previously reported condition for HKUST-1. Out of 20 algorithm-generated conditions, two conditions yielded Zn-HKUST-1 crystals.292 Synthetic Conditions Finder (SyCoFinder) can also be used for the optimization of the synthesis of Al-PMOF, while the original reported solvothermal reaction for Al-PMOF had a low 40% yield and long reaction time as 16 hours. Five parameters (type of solvent, concentration, temperature, microwave strength, and reaction time) were optimized by the GA algorithms, and an alternative condition was generated for the synthesis of highly crystalline Al-PMOF. Under the new condition, the reaction time was shortened to 50 minutes, with around 80% yield achieved.293 SyCoFinder has also been proven for the synthesis optimization of HKUST-1, while five parameters including the amount of metal and linker, amount of modulator, ultrasonic cleaning time, and spray cleaning time, were optimized for the synthesis of HKUST-1 surface anchored MOFs. In two generation and a total of 30 trials, HKUST-1 SURMOFs could be synthesized with 84% crystallinity and a perfect 100% [111]-orientation. One of the challenges of synthesizing SURMOFs is the layer-by-layer growth of the MOF film. With the larger number of deposition cycles, the influence from underlying self-assembled monolayer decreased, and the uniformity of the MOF orientation is lost. However, with the algorithm-optimized condition, HKUST-1 SURMOFs retain high 92% in [111] direction with 95% crystallinity even after 80 cycles.294
Besides, ML and AI can also support the development of new process for MOF synthesis. SyCoFinder can be utilized to optimize the synthesis condition of ZIF-8 and ZIF-67 with microdroplet-based spray method, a newly developed method for efficient continuous synthesis of MOF particles. Four parameters, including the furnace temperature, operating pressure, water/methanol ratio, and linker/metal ratio, were optimized. 100 parameter sets for ZIF-8 synthesis were generated by SyCoFinder and 21 of the most diverse sets were tested. Within the experiment set of the second generation, highly crystalline ZIF-8 with the surface area of 1748 m2 g−1 was synthesized, which is among the highest reported values and predominated by MOF micropores rather than mesopores from interparticle voids. The same condition was applied for the synthesis of ZIF-67, while ZIF-67 with high crystallinity and surface area was also obtained.295
Overall, the integration of ML and AI in MOF synthesis not only enhances the efficiency and efficacy of existing methods but also paves the way for innovative synthesis techniques, thereby advancing the field of MOF research and applications.
4.2.2 Functional oriented property predictions and structure optimization.
The applications and functionalities of MOFs are intricately linked to their structural properties. Traditionally, screening datasets to uncover MOFs with desired characteristics involves high-throughput computational screening (HTCS) utilizing methods like density functional theory (DFT) or GCMC.296 However, this process is often time-consuming, expensive, and inefficient due to the vast amount of data involved. The effectiveness of a ML model hinges on various factors including dataset scope and selection, choice of ML algorithm, feature selection and weighting, and thorough model evaluation. The selection of appropriate metrics and models is contingent upon the specific objectives of the data analysis.
Leveraging their experience in AI-assisted synthesis optimization and the in-depth study of the relationships between MOFs’ structures and functions, researchers have developed an auxiliary tool for MOF design and synthesis. This tool focuses on functional-oriented property predictions and structure optimizations, significantly enhancing the efficiency of designing MOFs for specific functions. Key applications including water treatment, gas adsorption, gas separation, energy transfer, and sensing will be discussed in detail below (Fig. 21).
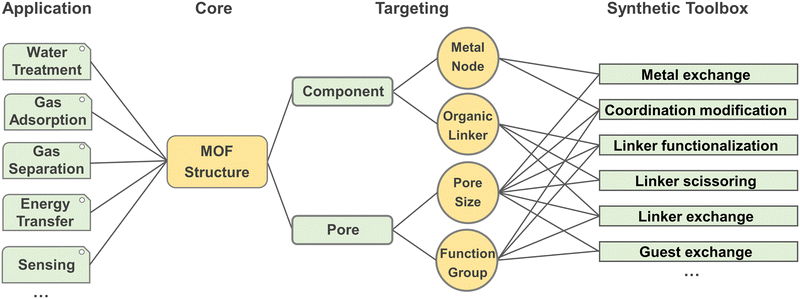 |
| Fig. 21 Simplified functional oriented property predictions and structure optimization processes. | |
For water treatment, artificial neural networks (ANNs) are excellent models due to their ability to handle many parameters with complex nonlinear relationships. In toxic and harmful ion adsorption of water treatment, the influencing parameters are complex and interrelated, which makes ANNs particularly suitable for predicting and optimizing the performance of MOFs in ion adsorption. The research on predicting the performance of MOF thin-film nanocomposite membranes through an ANN model by collecting parameters such as MOF size, loading, pore size, polyamide thickness, pressure, and salt concentration, can indicate that thickness and pore size are the most critical factors affecting performance.297 ANN model can also be employed to predict the performance in phosphate adsorption for water treatment. Several factors affecting MOF adsorption performance were analysed, including MOF types, synthesis methods, modification techniques, and operational conditions (initial concentration, adsorbent dose, pH, contact time, and temperature). The construction and evaluation of the ANN model indicate that initial phosphate concentration and modulating agents significantly impacted adsorption performance prediction.298
For gas adsorption, several energy and exhausted gases, are widely studied. For energy gases, such as H2, a dataset of approximately 98
000 real and hypothetical MOFs were used to screen their gas adsorption abilities by the Bayesian Optimization (BO) method, aiming to identify structures with high H2 storage capacity. By using this method, it is possible to find the optimal candidate MOFs by screening less than 0.027% of the database. Despite the high complexity of training, BO models have the following advantages: requiring fewer samples, identifying the global optimum rather than the local, and being suitable for black-box optimization.299 Besides, ANN model, least squares support vector machine (LS-SVM) model, and generalized regression neural network (GRNN) model can also be employed to study the H2 storage capacity, indicating that surface area and adsorption enthalpy are the most critical variables.300,301 For CH4, random forest (RF) method was trained on a dataset of over 130
000 MOFs from the hMOF database, while the percentage of metal was introduced as a variable to represent open metal sites, achieving an R2 value of 98%.302 Also, the practical application was further considered, aiming to prevent water-induced degradation of MOF structures through RF method.303 GCMC simulations and ANN regression models can also be employed to study the CH4 adsorption ability of 2224 MOFs.304 For CO2, DFT calculations and GCMC simulations can be used to study the CO2 adsorption performance of MOFs, suggesting that the –NH2 functional group has the strongest binding affinity with CO2.305 GCMC simulations also confirm that molecular selectivity is a crucial indicator for CO2 capture.306 Other gases like N2 can also be predicted, while RF method based on CoRE MOF database and DFT calculations based on ARC-MOF database can be employed for the gas adsorption performance study.307,308
For gas separation, through the comparisons of 2434 MOFs from the CoRE MOF and CSD databases, the selection of the database notably impact the gas adsorption and ideal selectivity calculations under low-pressure conditions in higher H2/CH4 Henry's coefficients.265 Multi-component-GCMC method to screen the hMOF database can be employed to identify the best materials for C2H6 and C2H4 separation, discovering that the porosity is the key factor.309 GCMC simulations with an XGBoost (eXtreme Gradient Boosting) ML model can be combined and employed for screening MOF materials for high Xe/Kr separation performance, while the porosity, density, pore volume, and pore limiting diameter are the key features influencing adsorption performance.310 5249 MOF membranes and 31
494 MOF/polymer mixed-matrix membranes (MMMs) were used for the evaluation in the separation of six gas pairs (He/H2, He/N2, He/CH4, H2/N2, H2/CH4, N2/CH4), which indicates that physical properties such as porosity have a stronger correlation with the gas adsorption and diffusion properties of MOFs, rather than chemical descriptors like atomic types and degree of unsaturation.311
For energy transfer, several highly conductive MOFs were developed through optimization. Potential conductive MOF structures can be identified by transfer learning.267 Crystal graph convolutional neural network (CGCNN) was employed to address bandgap prediction, which generates approximate crystal graphs and requires only 7 minutes to compute the properties of 13
058 MOFs, whereas traditional DFT calculations would take 1.5 million hours.312
For sensing, ANN model was used to predict glucose concentration in Huangshui, demonstrating excellent fitting and predictive performance with low root mean squared error and high residual predictive deviation, which showed superior detection performance and extended the sensor's linear detection range to 11 mM (Fig. 22).313
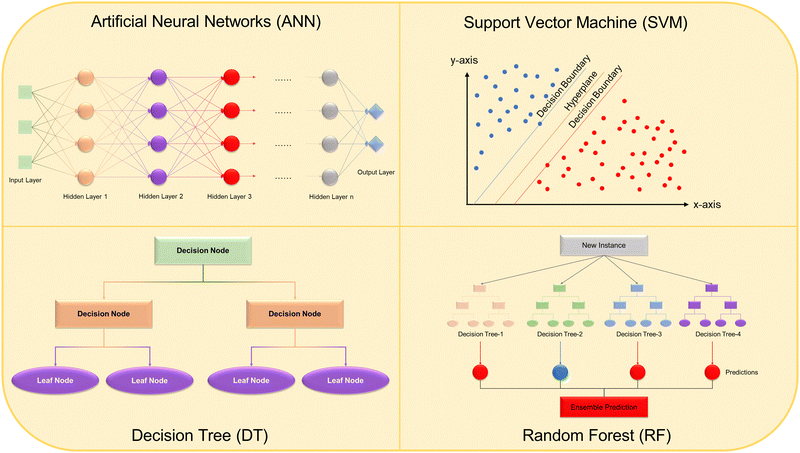 |
| Fig. 22 Classical logics for the models of machine learning. | |
4.2.3 AI-guided new MOF design and synthesis.
The AI-driven development of novel MOFs can be achieved mainly in three processes: (i) screening potential MOFs from hMOF databases; (ii) utilizing structure–activity relationship knowledge to assist structural design; (iii) employing algorithms to generate novel MOFs.
For the screening process when constructing hMOF database, the comprised MOF structures were identified by high-throughput screenings which involves simulating the performance of all candidates in the database for comparison. The construction of hMOF databases can be traced back to 2000 for the development of a method named “automated assembly of secondary building units (AASBU)” to generate MOF structures. Secondary building units (SBUs) from atoms were built by using Lennard-Jones potential to parameterize the interaction energy between atoms. Then structures were generated by calculating the interactions between SBUs with a given number of SBUs per unit cell and space group. The simulated annealing Monte Carlo algorithm was used to optimize the energy of the final structure by allowing building units to rearrange and adjusting cell size and distance between steps so that the resulting structure is stable enough to be synthesized.314 Later, geometric methods were developed as an alternative approach to minimize the energy, which can be classified as “bottom-up” and “top-down”. The “bottom-up“ approach begins with the SBUs, through sequential connection to form a periodic crystal structure.315 While the “top-down“ approach starts with a given network or topology and then maps the appropriate building blocks to the network to generate the structure.316,317 In this process, AI can accelerate the screening by introducing advanced algorithms to reduce the number of operation objects like performing a preselecting process to filter un-promising MOFs before running property simulations. The related algorithms can be mainly divided into three types, tree-based (i.e. decision trees, random forests), kernel-based (i.e. support vector machines), and artificial neural network,318–321 which provide the foundation for the subsequent structural design.
For the structure–activity relationship knowledge assistant, structure–activity relationship study is a cornerstone of modern chemistry which provides a systematic approach to understand how molecular structure influences properties, and thus enable to tailor materials for various applications. Employing AI to study structure–activity relationship can quickly identify the key features of high performance among a huge MOF database, which will be used to further generate promising MOF structures. Collectively, relative studies underscore the transformative impact of ML and AI methodologies in the rational design and synthesis of MOFs with various structures and properties.322–326
For the employment of algorithms to generate novel MOFs, introducing an evolutionary algorithm (EA) is a valuable approach. EA is one kind of ML algorithm that can start from a small set of MOFs and achieve high-performance structure discovery through continuous modification evolution. In EA, a linker is randomly chosen from the population to undergo one of several evolution operations (like linker modifications). The produced linker can be inserted back into the population only if it passes all the filters such as the torsions number need <8, and sites number = 2 to make sure it can form a MOF while its capacity should also be greater than the lowest one in the current population so that it has research value. As a result, the linkers possessing high capacity would evolve and the population could enlarge while excluding the low-performance ones.327–334 The integration of EAs with ML/AI techniques has significantly advanced the design and discovery of high-performing MOFs.
AI-assisted new MOF design is not limited to the development and optimization of the algorithm and model to increase the accuracy and speed. It is also trying to reduce the knowledge requirement to use the algorithm, which can predict MOF properties and generate MOFs with specified properties from natural language inputs. Some of the results reported and their applications have been summarized and listed in Table 4. Coefficient of determination (R2) reflects how closely the predicted values align with the ideal line, which is easy to interpret and allows for the comparison across different models and objectives. While R2 alone sometimes cannot fully capture the model's accuracy and can be misleading when comparing models with different numbers of variables. In addition, overfitting is a common issue in predictive models, which must be carefully addressed. It occurs when a model captures noise or random fluctuations in the training data instead of learning the underlying patterns. This phenomenon leads to poor performance on new, unseen data and undermines generalization of the model. To prevent overfitting, it is essential to split the available data into two parts: training set and testing set. The training set is used to build and tune the model, while the testing set, which the model has not seen during training, is employed to evaluate its generalization ability. Only no significant drop in performance on the testing set ensures that the model is robust and can accurately predict outcomes for new data.
Table 4 Typical examples for AI-assisted MOFs
Method types |
Application |
R
2
|
GBM for gradient-boosting machine; ADA for adaptive; GPR for Gaussian process regression; GBDT for Gradient boosting decision tree. |
XGBoost335 |
As adsorption |
0.93 |
LightGBM336 |
As adsorption |
0.996 |
ADA-GPR337 |
Hg/Ni removal |
0.998 |
ANN298 |
Phosphate adsorption |
0.73–0.96 |
ANN297 |
Water purification |
0.9062 |
RF338 |
Water adsorption |
0.941 |
RF339 |
Methane adsorption |
0.98 |
RF303 |
HCHO capture |
0.867 |
XGBoost340 |
CO2/N2/CH4 adsorption |
0.9945/0.9948/0.9911 |
GBDT341 |
H2/CH4/CO2 adsorption |
0.864 |
GPR342 |
CO2 adsorption |
0.98 |
General regression neural network343 |
H2 storage |
0.9986 |
GNN344 |
CO2 adsorption |
0.95 |
CNN345 |
Methane adsorption |
0.947 |
ANN338 |
Water adsorption |
0.982 |
ANN300 |
H2 storage |
0.93 |
RF309 |
Ethane/ethylene separation |
0.89 |
XGBoost346 |
N2/O2 separation |
0.93 |
XGBoost310 |
Xe/Kr separation |
0.973 |
Tree-based pipeline optimization tool311 |
He/H2/CH4/N2 separation |
0.99/0.80/0.73/0.84 |
ANN347 |
Xe/Kr separation |
0.972 |
ANN305 |
CO2/N2 separation |
0.905 |
Categorical boosting348 |
Ibuprofen adsorption |
0.76 |
ANN349 |
Glucose detection |
0.9996 |
RF267 |
Conductivity |
0.97 |
CNN312 |
Bandgap prediction |
0.876 |
Although there have been many works applying AI to the design of new MOFs, AI itself is also a growing discipline. Therefore, the application of more advanced AI algorithms for MOF design is necessary. In addition, interdisciplinary cooperation also needs to be improved. Many works only show the potential of different AI models, but there are few examples of reaching the laboratory to achieve synthesizing new MOF structures with performance breakthroughs, leading to a potential risk of the optimal structure not being able to be synthesized due to its unstable nature. Furthermore, the automated integration platform combining synthetic robots and ML algorithms for novel MOF synthesis has not been achieved yet. Such a platform will largely accelerate the synthesis of novel MOFs with the liberation of human labour.
5. Conclusion and outlook
In summary, MOFs have experienced remarkable growth and innovation over the past decades, establishing themselves as versatile materials with a wide range of applications. Advancements in their design and synthesis have been driven by a combination of large-scale synthesis attempts, functional-oriented modifications, and the integration of ML and AI for predictive modelling. Large-scale synthesis efforts have successfully constructed numerous novel structures, providing valuable experience in the design and synthesis of these materials. Functional-oriented modifications have further expanded the utility of MOFs by tailoring their properties for specific applications, leading to the development of MOFs with enhanced stability, activity, and other properties that meet the demands of complex industrial processes and environmental sustainability. The integration of ML and AI in MOF research represents a significant leap forward, with AI-driven predictions accelerating the discovery of novel MOF structures, optimizing synthesis conditions, and predicting material properties with high accuracy, which has significantly reduced the time and resources required for experimental trial and error.
In the coming years, the field of MOF design and synthesis is expected to evolve through several transformative trends, but it will also face critical challenges that must be addressed for the full potential of these materials to be realized. One major trend is the deeper integration of ML and AI with computational chemistry, which will continue to accelerate the discovery of novel MOF structures with highly specialized properties, particularly for emerging applications in energy storage, carbon capture, catalysis, and biomedicine. However, a key challenge will be the development of accurate, large-scale datasets needed to train AI models, as well as addressing the complexity of predicting MOF behavior in real-world conditions where multiple variables interact, which may need plenty of work for AI and researchers to explore collaboratively. Another emerging trend is the push towards sustainable and green synthesis methods, which are essential for scaling MOF production while minimizing environmental impact. Developing energy-efficient, low-waste, and solvent-free methods remains a significant challenge, especially when transitioning from laboratory-scale synthesis to large-scale industrial processes. Additionally, MOFs’ long-term stability under harsh operational conditions, such as in industrial catalysis or environmental remediation, presents another challenge. Addressing these challenges will require interdisciplinary collaboration, innovative research methodologies, and significant investment in both fundamental and applied sciences.
The future of MOF design and synthesis is poised to be even more dynamic and impactful, with several promising directions on the horizon:
• Deeper integration with computational techniques: the continued advancement of ML and AI will further refine the predictive capabilities for MOF design. Enhanced computational power and more sophisticated models will enable the discovery of novel MOFs with unprecedented properties, tailored for emerging applications in energy, environment, and biomedicine.
• Sustainable and environmentally friendly synthesis: as environmental concerns become more pressing, the development of sustainable and green synthesis methods for MOFs will gain prominence. Efforts to utilize renewable resources, reduce energy consumption, and minimize waste will be critical in MOF production, especially large-scale synthesis, more environmentally friendly.
• In situ characterization and real-time monitoring: advances in in situ characterization techniques will provide deeper insights into the dynamic behavior of MOFs during synthesis. Real-time monitoring of MOF synthesis will enhance the understanding of the formation and growth processes, allowing for better control of synthesis conditions and more efficient production of MOFs.
Overall, the design and synthesis of MOFs stand at the cusp of a new era, driven by innovative methodologies and interdisciplinary efforts. The convergence of large-scale attempts, functional modifications, and AI predictions will continue to propel the field forward, offering transformative solutions to global challenges and ushering in a new age of advanced materials.
Data availability
No primary research results, software or code have been included and no new data were generated or analysed as part of this review.
Conflicts of interest
There are no conflicts to declare.
Acknowledgements
This work was supported by the Robert A. Welch Foundation through an endowed chair (A-0030) to H.-C. Z.
Notes and references
- H.-C. Zhou, J. R. Long and O. M. Yaghi, Chem. Rev., 2012, 112, 673–674 CrossRef CAS PubMed.
- Q. Qian, P. A. Asinger, M. J. Lee, G. Han, K. M. Rodriguez, S. Lin, F. M. Benedetti, A. X. Wu, W. S. Chi and Z. P. Smith, Chem. Rev., 2020, 120, 8161–8266 CrossRef CAS PubMed.
- X. Zhang, Z. Chen, X. Liu, S. L. Hanna, X. Wang, R. Taheri-Ledari, A. Maleki, P. Li and O. K. Farha, Chem. Soc. Rev., 2020, 49, 7406–7427 RSC.
- Z. Hu, B. J. Deibert and J. Li, Chem. Soc. Rev., 2014, 43, 5815–5840 RSC.
- Q. Wang and D. Astruc, Chem. Rev., 2020, 120, 1438–1511 CrossRef CAS PubMed.
- H. Furukawa, K. E. Cordova, M. O’Keeffe and O. M. Yaghi, Science, 2013, 341, 1230444 CrossRef PubMed.
- K. Adil, Y. Belmabkhout, R. S. Pillai, A. Cadiau, P. M. Bhatt, A. H. Assen, G. Maurinb and M. Eddaoudi, Chem. Soc. Rev., 2017, 46, 3402–3430 RSC.
- B. Chen, S. Xiang and G. Qian, Acc. Chem. Res., 2010, 43, 1115–1124 CrossRef CAS PubMed.
- E. Barea, C. Montoro and J. A. R. Navarro, Chem. Soc. Rev., 2014, 43, 5419–5430 RSC.
- T. Islamoglu, Z. Chen, M. C. Wasson, C. T. Buru, K. O. Kirlikovali, U. Afrin, M. R. Mian and O. K. Farha, Chem. Rev., 2020, 120, 8130–8160 CrossRef CAS PubMed.
- J. Du, F. Li and L. Sun, Chem. Soc. Rev., 2021, 50, 2663–2695 RSC.
- T. D. Bennett and A. K. Cheetham, Acc. Chem. Res., 2014, 47, 1555–1562 CrossRef CAS PubMed.
- M. S. Denny Jr., J. C. Moreton, L. Benz and S. M. Cohen, Nat. Rev. Mater., 2016, 1, 16078 CrossRef.
- X. Zhao, Y. Wang, D.-S. Li, X. Bu and P. Feng, Adv. Mater., 2018, 30, 1705189 CrossRef PubMed.
- S. Qiu, M. Xue and G. Zhu, Chem. Soc. Rev., 2014, 43, 6116–6140 RSC.
- M. Eddaoudi, J. Kim, N. Rosi, D. Vodak, J. Wachter, M. O’Keeffe and O. M. Yaghi, Science, 2002, 295, 469–472 CrossRef CAS PubMed.
- Y. S. Wei, M. Zhang, R. Zou and Q. Xu, Chem. Rev., 2020, 120, 12089–12174 CrossRef CAS PubMed.
- A. J. Howarth, Y. Liu, P. Li, Z. Li, T. C. Wang, J. T. Hupp and O. K. Farha, Nat. Rev. Mater., 2016, 1, 15018 CrossRef CAS.
- D. Zhao, D. J. Timmons, D. Yuan and H.-C. Zhou, Acc. Chem. Res., 2011, 44, 123–133 CrossRef CAS PubMed.
- P. Silva, S. M. F. Vilela, J. P. C. Tomébc and F. A. A. Paz, Chem. Soc. Rev., 2015, 44, 6774–6803 RSC.
- Z. Han, K. Wang, H.-C. Zhou, P. Cheng and W. Shi, Nat. Protoc., 2023, 18, 1621–1640 CrossRef CAS PubMed.
- H. K. Chae, D. Y. Siberio-Pérez, J. Kim, Y. Go, M. Eddaoudi, A. J. Matzger, M. O'Keeffe and O. M. Yaghi, Nature, 2004, 427, 523–527 CrossRef CAS PubMed.
- Z. Han, K. Wang, Y. Guo, W. Chen, J. Zhang, X. Zhang, G. Siligardi, S. Yang, Z. Zhou, P. Sun, W. Shi and P. Cheng, Nat. Commun., 2019, 10, 5117 CrossRef PubMed.
- H. Furukawa, F. Gándara, Y.-B. Zhang, J. Jiang, W. L. Queen, M. R. Hudson and O. M. Yaghi, J. Am. Chem. Soc., 2014, 136, 4369–4381 CrossRef CAS PubMed.
- Z. Han, K. Wang, H. Min, J. Xu, W. Shi and P. Cheng, Angew. Chem., Int. Ed., 2022, 61, e202204066 CrossRef CAS PubMed.
- H. Li, M. Eddaoudi, T. L. Groy and O. M. Yaghi, J. Am. Chem. Soc., 1998, 120, 8571–8572 CrossRef CAS.
- D. M. Young, U. Geiser, A. J. Schultz and H. H. Wang, J. Am. Chem. Soc., 1998, 120, 1331–1332 CrossRef CAS.
- O. M. Yaghi, R. Jernigan, H. Li, C. E. Davis and T. L. Groy, J. Chem. Soc., Dalton Trans., 1997, 14, 2383–2384 RSC.
- O. M. Yaghi and H. Li, J. Am. Chem. Soc., 1995, 117, 10401–10402 CrossRef CAS.
- O. M. Yaghi, C. E. Davis, G. Li and H. Li, J. Am. Chem. Soc., 1997, 119, 2861–2868 CrossRef CAS.
- O. M. Yaghi, G. Li and H. Li, Nature, 1995, 378, 703–706 CrossRef CAS.
- T. D. Bennett and S. Horike, Nat. Rev. Mater., 2018, 3, 431–440 CrossRef.
- C. Zhang, W. Li and L. Li, Angew. Chem., Int. Ed., 2021, 60, 7488–7501 CrossRef CAS PubMed.
- Z. Han, K. Wang, M. Wang, T. Sun, J. Xu, H.-C. Zhou, P. Cheng and W. Shi, Chem, 2023, 9, 2561–2572 CAS.
- Z. Han, R. Zhang, J. Jiang, Z. Chen, Y. Ni, W. Xie, J. Xu, Z. Zhou, J. Chen, P. Cheng and W. Shi, J. Am. Chem. Soc., 2023, 145, 10149–10158 CrossRef CAS PubMed.
- X. Li, K. Chen, R. Guo and Z. Wei, Chem. Rev., 2023, 123, 10432–10467 CrossRef CAS PubMed.
- M. Gutiérrez, Y. Zhang and J.-C. Tan, Chem. Rev., 2022, 122, 10438–10483 CrossRef PubMed.
- Z. Han, K. Wang, Y. Chen, J. Li, S. J. Teat, S. Yang, W. Shi and P. Cheng, CCS Chem., 2022, 4, 3238–3245 CrossRef CAS.
- A. Knebel and J. Caro, Nat. Nanotechnol., 2022, 17, 911–923 CrossRef CAS PubMed.
- Z. Han, J. Li, W. Lu, K. Wang, Y. Chen, X. Zhang, L. Lin, X. Han, S. J. Teat, M. D. Frogley, S. Yang, W. Shi and P. Cheng, Angew. Chem., Int. Ed., 2022, 61, e202115585 CrossRef CAS PubMed.
- I. Ahmed, G. Jeon and F. Piccialli, IEEE Trans. Ind. Inform., 2022, 18, 5031–5042 Search PubMed.
- R. I. Mukhamediev, Y. Popova, Y. Kuchin, E. Zaitseva, A. Kalimoldayev, A. Symagulov, V. Levashenko, F. Abdoldina, V. Gopejenko, K. Yakunin, E. Muhamedijeva and M. Yelis, Mathematics, 2022, 10, 2552 CrossRef.
- H. Wang, T. Fu, Y. Du, W. Gao, K. Huang, Z. Liu, P. Chandak, S. Liu, P. van Katwyk, A. Deac, A. Anandkumar, K. Bergen, C. P. Gomes, S. Ho, P. Kohli, J. Lasenby, J. Leskovec, T.-Y. Liu, A. Manrai, D. Marks, B. Ramsundar, L. Song, J. Sun, J. Tang, P. Veličković, M. Welling, L. Zhang, C. W. Coley, Y. Bengio and M. Zitnik, Nature, 2023, 620, 47–60 CrossRef CAS PubMed.
- C. J. Haug and J. M. Drazen, N. Engl. J. Med., 2023, 388, 1201–1208 CrossRef CAS PubMed.
- J. N. Acosta, G. J. Falcone, P. Rajpurkar and E. J. Topol, Nat. Med., 2022, 28, 1773–1784 CrossRef CAS PubMed.
- M. Salvagno, F. S. Taccone and A. G. Gerli, Crit. Care, 2023, 27, 75 CrossRef PubMed.
- R. J. Chen, J. J. Wang, D. F. K. Williamson, T. Y. Chen, J. Lipkova, M. Y. Lu, S. Sahai and F. Mahmood, Nat. Biomed. Eng., 2023, 7, 719–742 CrossRef PubMed.
- M. Moor, O. Banerjee, Z. S. H. Abad, H. M. Krumholz, J. Leskovec, E. J. Topol and P. Rajpurkar, Nature, 2023, 616, 259–265 CrossRef CAS PubMed.
- F. Wong, C. de la Fuente-Nunez and J. J. Collins, Science, 2023, 381, 164–170 CrossRef CAS PubMed.
- A. Shmatko, N. G. Laleh, M. Gerstung and J. N. Kather, Nat. Cancer, 2022, 3, 1026–1038 CrossRef PubMed.
- H. Deng, S. Grunder, K. E. Cordova, C. Valente, H. Furukawa, M. Hmadeh, F. Gándara, A. C. Whalley, Z. Liu, S. Asahina, H. Kazumori, M. O’Keeffe, O. Terasaki, J. F. Stoddart and O. M. Yaghi, Science, 2012, 336, 1018–1023 CrossRef CAS PubMed.
- B. Li, Z. Zhang, Y. Li, K. Yao, Y. Zhu, Z. Deng, F. Yang, X. Zhou, G. Li, H. Wu, N. Nijem, Y. J. Chabal, Z. Lai, Y. Han, Z. Shi, S. Feng and J. Li, Angew. Chem., Int. Ed., 2012, 51, 1412–1415 CrossRef CAS PubMed.
- H. Zhu, L. Wang, X. Jie, D. Liu and Y. Cao, ACS Appl. Mater. Interfaces, 2016, 8, 22696–22704 CrossRef CAS PubMed.
- Z. Dou, J. Yu, Y. Cui, Y. Yang, Z. Wang, D. Yang and G. Qian, J. Am. Chem. Soc., 2014, 136, 5527–5530 CrossRef CAS PubMed.
- M. Dan-Hardi, C. Serre, T. Frot, L. Rozes, G. Maurin, C. Sanchez and G. Ferey, J. Am. Chem. Soc., 2009, 131, 10857–10859 CrossRef CAS PubMed.
- K. Liu, S. Zhang, X. Hu, K. Zhang, A. Roy and G. Yu, Environ. Sci. Technol., 2015, 49, 8657–8665 CrossRef CAS PubMed.
- Q.-G. Zhai, X. Bu, C. Mao, X. Zhao and P. Feng, J. Am. Chem. Soc., 2016, 138, 2524–2527 CrossRef CAS PubMed.
- Q. Shi, Z. Chen, Z. Song, J. Li and J. Dong, Angew. Chem., Int. Ed., 2011, 50, 672–675 CrossRef CAS PubMed.
- A. Kumar, D. G. Madden, M. Lusi, K.-J. Chen, E. A. Daniels, T. Curtin, J. J. Perry IV and M. J. Zaworotko, Angew. Chem., Int. Ed., 2015, 54, 14372–14377 CrossRef CAS PubMed.
- M. Wriedt, J. P. Sculley, A. A. Yakovenko, Y. Ma, G. J. Halder, P. B. Balbuena and H.-C. Zhou, Angew. Chem., Int. Ed., 2012, 51, 9804–9808 CrossRef CAS PubMed.
- K. S. Park, Z. Ni, A. P. Cote, J. Y. Choi, R. Huang, F. J. Uribe-Romo, H. K. Chae, M. O’Keeffe and O. M. Yaghi, Proc. Natl. Acad. Sci. U. S. A., 2006, 103, 10186–10191 CrossRef CAS PubMed.
- Z. Hu, Y. Peng, Z. Kang, Y. Qian and D. Zhao, Inorg. Chem., 2015, 54, 4862–4868 CrossRef CAS PubMed.
- H.-L. Jiang, Y. Tatsu, Z.-H. Lu and Q. Xu, J. Am. Chem. Soc., 2010, 132, 5586–5587 CrossRef CAS PubMed.
- N. L. Rosi, J. Kim, M. Eddaoudi, B. Chen, M. O’Keeffe and O. M. Yaghi, J. Am. Chem. Soc., 2005, 127, 1504–1518 CrossRef CAS PubMed.
- H.-L. Jiang, N. Tsumori and Q. Xu, Inorg. Chem., 2010, 49, 10001–10006 CrossRef CAS PubMed.
- W. Liu, X. Dai, Z. Bai, Y. Wang, Z. Yang, L. Zhang, L. Xu, L. Chen, Y. Li, D. Gui, J. Diwu, J. Wang, R. Zhou, Z. Chai and S. Wang, Environ. Sci. Technol., 2017, 51, 3911–3921 CrossRef CAS PubMed.
- C. Cárdenas and P. W. Ayers, Phys. Chem. Chem. Phys., 2013, 15, 13959–13968 RSC.
- J. L. Reed, J. Phys. Chem. A, 2012, 116, 7147–7153 CrossRef CAS PubMed.
- T. A. Engesser, M. R. Lichtenthaler, M. Schleep and I. Krossing, Chem. Soc. Rev., 2016, 45, 789–899 RSC.
- T. A. Engesser and I. Krossing, Coord. Chem. Rev., 2013, 257, 946–955 CrossRef CAS.
- G. Ferey, C. Mellot-Draznieks, C. Serre, F. Millange, J. Dutour, S. Surble and I. Margiolaki, Science, 2005, 309, 2040–2042 CrossRef CAS PubMed.
- B. Wang, A. P. Cote, H. Furukawa, M. O’Keeffe and O. M. Yaghi, Nature, 2008, 453, 207–211 CrossRef CAS PubMed.
- P. Deria, W. Bury, I. Hod, C.-W. Kung, O. Karagiaridi, J. T. Hupp and O. K. Farha, Inorg. Chem., 2015, 54, 2185–2192 CrossRef CAS PubMed.
- Y. Liu, V. Ch Kravtsov, R. Larsen and M. Eddaoudi, Chem. Commun., 2006, 1488–1490 RSC.
- J.-B. Lin, T. T. T. Nguyen, R. Vaidhyanathan, J. Burner, J. M. Taylor, H. Durekova, F. Akhtar, R. K. Mah, O. Ghaffari-Nik, S. Marx, N. Fylstra, S. S. Iremonger, K. W. Dawson, P. Sarkar, P. Hovington, A. Rajendran, T. K. Woo and G. K. H. Shimizu, Science, 2021, 374, 1464–1469 CrossRef CAS PubMed.
- C.-Y. Sun, X.-L. Wang, C. Qin, J.-L. Jin, Z.-M. Su, P. Huang and K.-Z. Shao, Chem. – Eur. J., 2013, 19, 3639–3645 CrossRef CAS PubMed.
- Q. Zhu, T. Sheng, R. Fu, C. Tan, S. Hu and X. Wu, Chem. Commun., 2010, 46, 9001–9003 RSC.
- Z. Han, X. Yu, H. Min, W. Shi and P. Cheng, Chem. J. Chin. Univ., 2022, 43, 20210342 Search PubMed.
- X. Si, C. Jiao, F. Li, J. Zhang, S. Wang, S. Liu, Z. Li, L. Sun, F. Xu, Z. Gabelica and C. Schick, Energy Environ. Sci., 2011, 4, 4522–4527 RSC.
- J. P. S. Mowat, S. R. Mille, A. M. Z. Slawin, V. R. Seymour, S. E. Ashbrook and P. A. Wright, Microporous Mesoporous Mater., 2011, 142, 322–333 CrossRef CAS.
- B. Zheng, J. Bai, J. Duan, L. Wojtas and M. J. Zaworotko, J. Am. Chem. Soc., 2011, 133, 748–751 CrossRef CAS PubMed.
- W. Du, Z. Zhu, Y.-L. Bai, Z. Yang, S. Zhu, J. Xu, Z. Xie and J. Fang, Chem. Commun., 2018, 54, 5972–5995 RSC.
- S.-Y. Zhang, W. Shi, P. Cheng and M. J. Zaworotko, J. Am. Chem. Soc., 2015, 137, 12203–12206 CrossRef CAS PubMed.
- J. An, S. J. Geib and N. L. Rosi, J. Am. Chem. Soc., 2009, 131, 8376–8377 CrossRef CAS PubMed.
- J. Huang, H. Ding, Y. Xu, D. Zeng, H. Zhu, D.-M. Zang, S.-S. Bao, Y. Ma and L.-M. Zheng, Nat. Commun., 2017, 8, 2131 CrossRef PubMed.
- S. Yuan, Y.-P. Chen, J.-S. Qin, W. Lu, L. Zou, Q. Zhang, X. Wang, X. Sun and H.-C. Zhou, J. Am. Chem. Soc., 2016, 138, 8912–8919 CrossRef CAS PubMed.
- K. Koh, A. G. Wong-Foy and A. J. Matzger, Angew. Chem., Int. Ed., 2008, 47, 677–680 CrossRef CAS PubMed.
- J. Campbell and B. Tokay, Microporous Mesoporous Mater., 2017, 251, 190–199 CrossRef CAS.
- A. Schaate, P. Roy, A. Godt, J. Lippke, F. Waltz, M. Wiebcke and P. Behrens, Chem. – Eur. J., 2011, 17, 6643–6651 CrossRef CAS PubMed.
- S.-Y. Zhang, D. Li, D. Guo, H. Zhang, W. Shi, P. Cheng, L. Wojtas and M. J. Zaworotko, J. Am. Chem. Soc., 2015, 137, 15406–15409 CrossRef CAS PubMed.
- Z. Zheng, H. L. Nguyen, N. Hanikel, K. K.-Y. Li, Z. Zhou, T. Ma and O. M. Yaghi, Nat. Protoc., 2023, 18, 136–156 CrossRef CAS PubMed.
- B. P. Carpenter, A. R. Talosig, B. Rose, G. D. Palma and J. P. Patterson, Chem. Soc. Rev., 2023, 52, 6918–6937 RSC.
- Z. Han, Z. Yan, K. Wang, H. Min, X. Kang, K. Lv, X. Zhang, Z. Zhou, S. Yang, W. Shi and P. Cheng, Sci. China: Chem., 2022, 65, 1088–1093 CrossRef CAS.
- Y. Guo, Z. Han, H. Min, Z. Chen, T. Sun, L. Wang, W. Shi and P. Cheng, Small Struct., 2022, 3, 2100113 CrossRef CAS.
- P. T. Phan, J. Hong, N. Tran and T. H. Le, Nanomaterials, 2023, 13, 352 CrossRef CAS PubMed.
- N. Stock and S. Biswas, Chem. Rev., 2012, 112, 933–969 CrossRef CAS PubMed.
- S. H. Jhung, J.-H. Lee, J. W. Yoon, C. Serre, G. Férey and J.-S. Chang, Adv. Mater., 2007, 19, 121–124 CrossRef CAS.
- E. V. Perez, K. J. Balkus Jr., J. P. Ferraris and I. H. Musselman, J. Membr. Sci., 2009, 328, 165–173 CrossRef CAS.
- R. Ameloot, L. Stappers, J. Fransaer, L. Alaerts, B. F. Sels and D. E. De Vos, Chem. Mater., 2009, 21, 2580–2582 CrossRef CAS.
- A. Pichon, A. Lazuen-Garay and S. L. James, CrystEngComm, 2006, 8, 211–214 RSC.
- T. Friscic, D. G. Reid, I. Halasz, R. S. Stein, R. E. Dinnebier and M. J. Duer, Angew. Chem., Int. Ed., 2010, 49, 712–715 CrossRef CAS PubMed.
- W. Yuan, T. Friscic, D. Apperley and S. L. James, Angew. Chem., Int. Ed., 2010, 49, 3916–3919 CrossRef CAS PubMed.
- R. Zhang, C.-A. Tao, R. Chen, L. Wu, X. Zou and J. Wang, Nanomaterials, 2018, 8, 1067 CrossRef PubMed.
- N. A. Khan and S. H. Jhung, Coord. Chem. Rev., 2015, 285, 11–23 CrossRef CAS.
- J. H. Bang and K. S. Suslick, Adv. Mater., 2010, 22, 1039–1059 CrossRef CAS PubMed.
- S. Hajra, M. Sahu, A. M. Padhan, I. S. Lee, D. K. Yi, P. Alagarsamy, S. S. Nanda and H. J. Kim, Adv. Funct. Mater., 2021, 31, 2101829 CrossRef CAS.
- E. S. Grape, J. G. Flores, T. Hidalgo, E. Martínez-Ahumada, A. Gutiérrez-Alejandre, A. Hautier, D. R. Williams, M. O’Keeffe, L. Öhrström, T. Willhammar, P. Horcajada, I. A. Ibarra and A. K. Inge, J. Am. Chem. Soc., 2020, 142, 16795–16804 CrossRef CAS PubMed.
- M. Sánchez-Sánchez, N. Getachew, K. Díaz, M. Díaz-García, Y. Chebude and I. Díaz, Green Chem., 2015, 17, 1500–1509 RSC.
- P. A. Bayliss, I. A. Ibarra, E. Pérez, S. Yang, C. C. Tang, M. Poliakoff and M. Schröder, Green Chem., 2014, 16, 3796–3802 RSC.
- I. A. Ibarra, P. A. Bayliss, E. Perez, S. Yang, A. J. Blake, H. Nowell, D. R. Allan, M. Poliakoff and M. Schröder, Green Chem., 2012, 14, 117–122 RSC.
- J. H. Fu, Z. Zhong, D. Xie, Y. J. Guo, D. X. Kong, Z. X. Zhao, Z. X. Zhao and M. Li, Angew. Chem., Int. Ed., 2020, 59, 20489–20498 CrossRef CAS PubMed.
- M. J. Dong, M. Zhao, S. Ou, C. Zou and C. D. Wu, Angew. Chem., Int. Ed., 2014, 53, 1575–1579 CrossRef CAS PubMed.
- B. Wang, X. L. Lv, D. Feng, L. H. Xie, J. Zhang, M. Li, Y. Xie, J. R. Li and H.-C. Zhou, J. Am. Chem. Soc., 2016, 138, 6204–6216 CrossRef CAS PubMed.
- Y. Li, Z. Yang, Y. Wang, Z. Bai, T. Zheng, X. Dai, S. Liu, D. Gui, W. Liu, M. Chen, L. Chen, J. Diwu, L. Zhu, R. Zhou, Z. Chai, T. E. Albrecht-Schmitt and S. Wang, Nat. Commun., 2017, 8, 1354 CrossRef PubMed.
- Z. Hu, W. P. Lustig, J. Zhang, C. Zheng, H. Wang, S. J. Teat, Q. Gong, N. D. Rudd and J. Li, J. Am. Chem. Soc., 2015, 137, 16209–16215 CrossRef CAS PubMed.
- S. Wu, Y. Lin, J. Liu, W. Shi, G. Yang and P. Cheng, Adv. Funct. Mater., 2018, 28, 1707169 CrossRef.
- J. Chen, Y. Zhu and S. Kaskel, Angew. Chem., Int. Ed., 2021, 60, 5010–5035 CrossRef CAS PubMed.
- Y. Zhao, H. Zeng, X.-W. Zhu, W. Lu and D. Li, Chem. Soc. Rev., 2021, 50, 4484–4513 RSC.
- T. Rasheed and F. Nabeel, Coord. Chem. Rev., 2019, 401, 213065 CrossRef CAS.
- K. Binnemans, Chem. Rev., 2009, 109, 4283–4374 CrossRef CAS PubMed.
- D. Parker, Coord. Chem. Rev., 2000, 205, 109–130 CrossRef CAS.
- S. A. A. Razavi and A. Morsali, Coord. Chem. Rev., 2020, 415, 213299 CrossRef CAS.
- K. Y. Zhang, Q. Yu, H. Wei, S. Liu, Q. Zhao and W. Huang, Chem. Rev., 2018, 118, 1770–1839 CrossRef CAS PubMed.
- M. D. Allendorf, C. A. Bauer, R. K. Bhakta and R. J. T. Houk, Chem. Soc. Rev., 2009, 38, 1330–1352 RSC.
- J. Heine and K. Muller-Buschbaum, Chem. Soc. Rev., 2013, 42, 9232–9242 RSC.
- C.-Y. Sun, X.-L. Wang, X. Zhang, C. Qin, P. Li, Z.-M. Su, D.-X. Zhu, G.-G. Shan, K.-Z. Shao, H. Wu and J. Li, Nat. Commun., 2013, 4, 2717 CrossRef PubMed.
- P. Chandrasekhar, A. Mukhopadhyay, G. Savitha and J. N. Moorthy, Chem. Sci., 2016, 7, 3085–3091 RSC.
- N. D. Rudd, Y. Liu, K. Tan, F. Chen, Y. J. Chabal and J. Li, ACS Sustainable Chem. Eng., 2019, 7, 6561–6568 CrossRef CAS.
- H. Min, Z. Han, M. Wang, Y. Li, T. Zhou, W. Shi and P. Cheng, Inorg. Chem. Front., 2020, 7, 3379–3385 RSC.
- H. Min, T. Sun, W. Cui, Z. Han, P. Yao, P. Cheng and W. Shi, Inorg. Chem., 2023, 62, 8739–8745 CrossRef CAS PubMed.
- Y. Liu, W. Xuan and Y. Cui, Adv. Mater., 2010, 22, 4112–4135 CrossRef CAS PubMed.
- Z.-G. Gu, S. Grosjean, S. Brase, C. Wo and L. Heinke, Chem. Commun., 2015, 51, 8998–9001 RSC.
- X. Zhang, N. Xu, S.-Y. Zhang, X.-Q. Zhao and P. Cheng, RSC Adv., 2014, 4, 40643–40650 RSC.
- J. M. Fraile, J. I. García and J. A. Mayoral, Chem. Rev., 2009, 109, 360–417 CrossRef CAS PubMed.
- R. J. Kuppler, D. J. Timmons, Q.-R. Fang, J.-R. Li, T. A. Makal, M. D. Young, D. Yuan, D. Zhao, W. Zhuang and H.-C. Zhou, Coord. Chem. Rev., 2009, 253, 3042–3066 CrossRef CAS.
- M. Heitbaum, F. Glorius and I. Escher, Angew. Chem., Int. Ed., 2006, 45, 4732–4762 CrossRef CAS PubMed.
- R. E. Morris and X. H. Bu, Nat. Chem., 2010, 2, 353–361 CrossRef CAS PubMed.
- J. S. Seo, D. Whang, H. Lee, S. I. Jun, J. Oh, Y. J. Jeon and K. Kim, Nature, 2000, 404, 982–986 CrossRef CAS PubMed.
- Z. Gu, C. Zhan, J. Zhang and X. H. Bu, Chem. Soc. Rev., 2016, 45, 3122–3144 RSC.
- J. Zhang, Y. G. Yao and X. Bu, Chem. Mater., 2007, 19, 5083–5089 CrossRef CAS.
- S. Lee, E. A. Kapustin and O. M. Yaghi, Science, 2016, 353, 808–811 CrossRef CAS PubMed.
- L. Ma, C. Abney and W. Lin, Chem. Soc. Rev., 2009, 38, 1248–1256 RSC.
- M. Banerjee, S. Das, M. Yoon, H. J. Choi, M. H. Hyun, S. M. Park, G. Seo and K. Kim, J. Am. Chem. Soc., 2009, 131, 7524–7525 CrossRef CAS PubMed.
- J. Zhao, H. Li, Y. Han, R. Li, X. Ding, X. Feng and B. Wang, J. Mater. Chem. A, 2015, 3, 12145–12148 RSC.
- Z. Han, W. Shi and P. Cheng, Chin. Chem. Lett., 2018, 29, 819–822 CrossRef CAS.
- K. Jayaramulu, S. Mukherjee, D. M. Morales, D. P. Dubal, A. K. Nanjundan, A. Schneemann, J. Masa, S. Kment, W. Schuhmann, M. Otyepka, R. Zbořil and R. A. Fischer, Chem. Rev., 2022, 122, 17241–17338 CrossRef CAS PubMed.
- A. López-Olvera, J. G. Flores, J. Aguilar-Pliego, C. K. Brozek, A. Gutiérrez-Alejandre and I. A. Ibarra, Chem. Mater., 2021, 33, 6269–6276 CrossRef.
- R. A. Peralta, P. Lyu, A. López-Olvera, J. L. Obeso, C. Leyva, N. C. Jeong, I. A. Ibarra and G. Maurin, Angew. Chem., Int. Ed., 2022, 61, e202210857 CrossRef CAS PubMed.
- H. Sepehrmansourie, H. Alamgholiloo, N. N. Pesyan and M. A. Zolfigol, Appl. Catal., B, 2023, 321, 122082 CrossRef CAS.
- R. A. Peralta, M. T. Huxley, P. Lyu, M. L. Díaz-Ramírez, S. H. Park, J. L. Obeso, C. Leyva, C. Y. Heo, S. Jang, J. H. Kwak, G. Maurin, I. A. Ibarra and N. C. Jeong, ACS Appl. Mater. Interfaces, 2023, 15, 1410–1417 CrossRef CAS PubMed.
- S. P. Gouda, K. Ngaosuwan, S. Assabumrungrat, M. Selvaraj, G. Halder and S. L. Rokhum, Renewable Energy, 2022, 197, 161–169 CrossRef CAS.
- J. Zhou, J. Li, L. Kan, L. Zhang, Q. Huang, Y. Yan, Y. Chen, J. Liu, S.-L. Li and Y.-Q. Lan, Nat. Commun., 2022, 13, 4681 CrossRef CAS PubMed.
- S. Li, C. Wang, Y. Liu, Y. Liu, M. Cai, W. Zhao and X. Duan, Chem. Eng. J., 2023, 455, 140943 CrossRef CAS.
- J. Y. Lee, O. K. Farha, J. Roberts, K. A. Scheidt, S. T. Nguyen and J. T. Hupp, Chem. Soc. Rev., 2009, 38, 1450–1459 RSC.
- Y. Shen, T. Pan, L. Wang, Z. Ren, W. Zhang and F. Huo, Adv. Mater., 2021, 33, 2007442 CrossRef CAS PubMed.
- C.-D. Wu and M. Zhao, Adv. Mater., 2017, 29, 1605446 CrossRef PubMed.
- L. Jiao, Y. Wang, H.-L. Jiang and Q. Xu, Adv. Mater., 2018, 30, 1703663 CrossRef PubMed.
- Y. Cui, Y. Zhao, J. Wu and H. Hou, Nano Today, 2023, 52, 101972 CrossRef CAS.
- Y. Qin, Z. Li, Y. Duan, J. Guo, M. Zhao and Z. Tang, Matter, 2022, 5, 3260–3310 CrossRef CAS.
- M. Sadakiyo, Nanoscale, 2022, 14, 3398–3406 RSC.
- T. G. Glover, G. W. Peterson, B. J. Schindler, D. Britt and O. M. Yaghi, Chem. Eng. Sci., 2011, 66, 163–170 CrossRef.
- S. Yang, J. Sun, A. J. Ramirez-Cuesta, S. K. Callear, W. I. F. David, D. P. Anderson, R. Newby, A. J. Blake, J. E. Parker, C. C. Tang and M. Schröder, Nat. Chem., 2012, 4, 887–894 CrossRef CAS PubMed.
- S. Yang, L. Liu, J. Sun, K. M. Thomas, A. J. Davies, M. W. George, A. J. Blake, A. H. Hill, A. N. Fitch, C. C. Tang and M. Schröder, J. Am. Chem. Soc., 2013, 135, 4954–4957 CrossRef CAS PubMed.
- K. Tan, P. Canepa, Q. Gong, J. Liu, D. H. Johnson, A. Dyevoich, P. K. Thallapally, T. Thonhauser, J. Li and Y. J. Chabal, Chem. Mater., 2013, 25, 4653–4662 CrossRef CAS.
- Z. A. Castillo, F. J. Muñoz-Lara, M. C. Muñoz, D. Aravena, A. B. Gaspar, J. F. Sánchez-Royo, E. Ruiz, M. Ohba, R. Matsuda and S. Kitagawa, Inorg. Chem., 2013, 52, 12777–12783 CrossRef PubMed.
- A. Torres-Knoop, R. Krishna and D. Dubbeldam, Angew. Chem., Int. Ed., 2014, 53, 7774–7778 CrossRef CAS PubMed.
- B. Saccoccia, A. M. Bohnsack, N. W. Waggoner, K. H. Cho, J. S. Lee, D. Y. Hong, V. M. Lynch, J. S. Chang and S. M. Humphrey, Angew. Chem., Int. Ed., 2015, 54, 5394–5398 CrossRef CAS PubMed.
- S. Q. Wang, S. Mukherjee, E. Patyk-Kazmierczak, S. Darwish, A. Bajpai, Q. Y. Yang and M. J. Zaworotko, Angew. Chem., Int. Ed., 2019, 58, 6630–6634 CrossRef CAS PubMed.
- Z. Y. Gu and X. P. Yan, Angew. Chem., Int. Ed., 2010, 49, 1477–1480 CrossRef CAS PubMed.
- M. I. Gonzalez, M. T. Kapelewski, E. D. Bloch, P. J. Milner, D. A. Reed, M. R. Hudson, J. A. Mason, G. Barin, C. M. Brown and J. R. Long, J. Am. Chem. Soc., 2018, 140, 3412–3422 CrossRef CAS PubMed.
- X. Cui, Z. Niu, C. Shan, L. Yang, J. Hu, Q. Wang, P. C. Lan, Y. Li, L. Wojtas, S. Ma and H. Xing, Nat. Commun., 2020, 11, 5456 CrossRef CAS PubMed.
- X. Li, J. Wang, N. Bai, X. Zhang, X. Han, I. da Silva, C. G. Morris, S. Xu, D. M. Wilary, Y. Sun, Y. Cheng, C. A. Murray, C. C. Tang, M. D. Frogley, G. Cinque, T. Lowe, H. Zhang, A. J. Ramirez-Cuesta, K. M. Thomas, L. W. Bolton, S. Yang and M. Schröder, Nat. Commun., 2020, 11, 4280 CrossRef CAS PubMed.
- Y. Zhang, S. Yuan, X. Feng, H. Li, J. Zhou and B. Wang, J. Am. Chem. Soc., 2016, 138, 5785–5788 CrossRef CAS PubMed.
- M. Savage, Y. Cheng, T. L. Easun, J. E. Eyley, S. P. Argent, M. R. Warren, W. Lewis, C. Murray, C. C. Tang, M. D. Frogley, G. Cinque, J. Sun, S. Rudic, R. T. Murden, M. J. Benham, A. N. Fitch, A. J. Blake, A. J. Ramirez-Cuesta, S. Yang and M. Schröder, Adv. Mater., 2016, 28, 8705–8711 CrossRef CAS PubMed.
- W. P. Mounfield, C. Han, S. H. Pang, U. Tumuluri, Y. Jiao, S. Bhattacharyya, M. R. Dutzer, S. Nair, Z. Wu, R. P. Lively, D. S. Sholl and K. S. Walton, J. Phys. Chem. C, 2016, 120, 27230–27240 CrossRef CAS.
- X. Cui, Q. Yang, L. Yang, R. Krishna, Z. Zhang, Z. Bao, H. Wu, Q. Ren, W. Zhou, B. Chen and H. Xing, Adv. Mater., 2017, 29, 1606929 CrossRef PubMed.
- S. Glomb, D. Woschko, G. Makhloufi and C. Janiak, ACS Appl. Mater. Interfaces, 2017, 9, 37419–37434 CrossRef CAS PubMed.
- S. Gorla, M. L. Díaz-Ramírez, N. S. Abeynayake, D. M. Kaphan, D. R. Williams, V. Martis, H. A. Lara-García, B. Donnadieu, N. Lopez, I. A. Ibarra and V. Montiel-Palma, ACS Appl. Mater. Interfaces, 2020, 12, 41758–41764 CrossRef CAS PubMed.
- J. H. Carter, X. Han, F. Y. Moreau, I. Silva, A. Nevin, H. G. W. Godfrey, C. C. Tang, S. Yang and M. Schröder, J. Am. Chem. Soc., 2018, 140, 15564–15567 CrossRef CAS PubMed.
- E. Martínez-Ahumada, M. L. Díaz-Ramírez, H. Lara-García, D. R. Williams, V. Martis, V. Jancik, E. Lima and I. A. Ibarra, J. Mater. Chem. A, 2020, 8, 11515–11520 RSC.
- G. L. Smith, J. E. Eyley, X. Han, X. Zhang, J. Li, N. M. Jacques, H. G. W. Godfrey, S. P. Argent, L. J. M. McPherson, S. J. Teat, Y. Cheng, M. D. Frogley, G. Cinque, S. J. Day, C. C. Tang, T. L. Easun, S. Rudić, A. J. Ramirez-Cuesta, S. Yang and M. Schröder, Nat. Mater., 2019, 18, 1358–1365 CrossRef CAS PubMed.
- J. A. Zárate, E. Sánchez-González, D. R. Williams, E. González-Zamora, V. Martis, A. Martínez, J. Balmaseda, G. Maurin and I. A. Ibarra, J. Mater. Chem. A, 2019, 7, 15580–15584 RSC.
- M. J. Yin, X. H. Xiong, X. F. Feng, W. Y. Xu, R. Krishna and F. Luo, Inorg. Chem., 2021, 60, 3447–3451 CrossRef CAS PubMed.
- X. Han, H. G. W. Godfrey, L. Briggs, A. J. Davies, Y. Cheng, L. L. Daemen, A. M. Sheveleva, F. Tuna, E. J. L. McInnes, J. Sun, C. Drathen, M. W. George, A. J. Ramirez-Cuesta, K. M. Thomas, S. Yang and M. Schröder, Nat. Mater., 2018, 17, 691–696 CrossRef CAS PubMed.
- X. Han, Y. Hong, Y. Ma, W. Lu, J. Li, L. Lin, A. M. Sheveleva, F. Tuna, E. J. L. McInnes, C. Dejoie, J. Sun, S. Yang and M. Schröder, J. Am. Chem. Soc., 2020, 142, 15235–15239 CrossRef CAS PubMed.
- J. Jiang, H. Furukawa, Y.-B. Zhang and O. M. Yaghi, J. Am. Chem. Soc., 2016, 138, 10244–10251 CrossRef CAS PubMed.
- G. Li, H. Kobayashi, J. M. Taylor, R. Ikeda, Y. Kubota, K. Kato, M. Takata, T. Yamamoto, S. Toh, S. Matsumura and H. Kitagawa, Nat. Mater., 2014, 13, 802–806 CrossRef CAS PubMed.
- V. F. Yusuf, N. I. Malek and S. K. Kailasa, ACS Omega, 2022, 7, 44507–44531 CrossRef CAS PubMed.
- Y. Lin, C. Kong, Q. Zhang and L. Chen, Adv. Energy Mater., 2017, 7, 1601296 CrossRef.
- W. Zhang, Y. Hu, J. Ge, H.-L. Jiang and S.-H. Yu, J. Am. Chem. Soc., 2014, 136, 16978–16981 CrossRef CAS PubMed.
- B. Li, H.-M. Wen, W. Zhou, J. Q. Xu and B. Chen, Chem, 2016, 1, 557–580 CAS.
- F. Gándara, H. Furukawa, S. Lee and O. M. Yaghi, J. Am. Chem. Soc., 2014, 136, 5271–5274 CrossRef PubMed.
- T. Tian, Z. Zeng, D. Vulpe, M. E. Casco, G. Divitini, P. A. Midgley, J. Silvestre-Albero, J.-C. Tan, P. Z. Moghadam and D. Fairen-Jimenez, Nat. Mater., 2018, 17, 174–179 CrossRef CAS PubMed.
- D. Alezi, Y. Belmabkhout, M. Suyetin, P. M. Bhatt, J. Weseliński, V. Solovyeva, K. Adil, I. Spanopoulos, P. N. Trikalitis, A.-H. Emwas and M. Eddaoudi, J. Am. Chem. Soc., 2015, 137, 13308–13318 CrossRef CAS PubMed.
- S. Zhou, O. Shekhah, A. Ramírez, P. Lyu, E. Abou-Hamad, J. Jia, J. Li, P. M. Bhatt, Z. Huang, H. Jiang, T. Jin, G. Maurin, J. Gascon and M. Eddaoudi, Nature, 2022, 606, 706–712 CrossRef CAS PubMed.
- F.-L. Li, P. Wang, X. Huang, D. J. Young, H.-F. Wang, P. Braunstein and J.-P. Lang, Angew. Chem., Int. Ed., 2019, 58, 7051–7056 CrossRef CAS PubMed.
- Z. Xue, K. Liu, Q. Liu, Y. Li, M. Li, C.-Y. Su, N. Ogiwara, H. Kobayashi, H. Kitagawa, M. Liu and G. Li, Nat. Commun., 2019, 10, 5048 Search PubMed.
- H.-F. Wang, L. Chen, H. Pang, S. Kaskel and Q. Xu, Chem. Soc. Rev., 2020, 49, 1414–1448 RSC.
- K. Ge, S. Sun, Y. Zhao, K. Yang, S. Wang, Z. Zhang, J. Cao, Y. Yang, Y. Zhang, M. Pan and L. Zhu, Angew. Chem., Int. Ed., 2021, 60, 12097–12102 CrossRef CAS PubMed.
- W. Cheng, Z.-P. Wu, D. Luan, S.-Q. Zang and X. W. Lou, Angew. Chem., Int. Ed., 2021, 60, 26397–26402 CrossRef CAS PubMed.
- R. Zhao, Z. Liang, R. Zou and Q. Xu, Joule, 2018, 2, 2235–2259 Search PubMed.
- M. Zhong, L. Kong, N. Li, Y.-Y. Liu, J. Zhu and X.-H. Bu, Coord. Chem. Rev., 2019, 388, 172–201 CrossRef CAS.
- S. Bai, X. Liu, K. Zhu, S. Wu and H. Zhou, Nat. Energy, 2016, 1, 16094 CrossRef CAS.
- C. Zhang, L. Shen, J. Shen, F. Liu, G. Chen, R. Tao, S. Ma, Y. Peng and Y. Lu, Adv. Mater., 2019, 31, 1808338 CrossRef PubMed.
- H. Jiang, X.-C. Liu, Y. Wu, Y. Shu, X. Gong, F.-S. Ke and H. Deng, Angew. Chem., Int. Ed., 2018, 57, 3916–3921 CrossRef CAS PubMed.
- M. S. Yao, W.-H. Li and G. Xu, Coord. Chem. Rev., 2021, 426, 213479 CrossRef CAS.
- X. Fang, B. Zong and S. Mao, Nano-Micro Lett., 2018, 10, 64 CrossRef PubMed.
- S. Tajik, H. Beitollahi, F. G. Nejad, I. Sheikhshoaie, A. S. Nugraha, H. W. Jang, Y. Yamauchi and M. Shokouhimehr, J. Mater. Chem. A, 2021, 9, 8195–8220 RSC.
- S. Kempahanumakkagari, K. Vellingiri, A. Deep, E. E. Kwon, N. Bolan and K.-H. Kim, Coord. Chem. Rev., 2018, 357, 105–129 CrossRef CAS.
- C.-S. Liu, J. Li and H. Pang, Coord. Chem. Rev., 2020, 410, 213222 CrossRef CAS.
- Y. Sun, W. Xu, F. Lang, H. Wang, F. Pan and H. Hou, Small, 2024, 20, 2305879 CrossRef CAS PubMed.
- A. Bajpai, P. Chandrasekhar, S. Govardhan, R. Banerjee and J. N. Moorthy, Chem. – Eur. J., 2015, 21, 2759–2765 CrossRef CAS PubMed.
- D. Sun, F. Sun, X. Deng and Z. Li, Inorg. Chem., 2015, 54, 8639–8643 CrossRef CAS PubMed.
- C. K. Brozek, L. Bellarosa, T. Soejima, T. V. Clark, N. López and M. Dincă, Chem. – Eur. J., 2014, 20, 6871–6874 CrossRef CAS PubMed.
- Z. Zhang, Y. Xiao, M. Cui, J. Tang, Z. Fei, Q. Liu, X. Chen and X. Qiao, Dalton Trans., 2019, 48, 14971–14974 RSC.
- S.-Y. Jia, Y.-F. Zhang, Y. Liu, F.-X. Qin, H.-T. Ren and S.-H. Wu, J. Hazard., 2013, 262, 589–597 CrossRef CAS PubMed.
- B. Lee, D. Moon and J. Park, Angew. Chem., Int. Ed., 2020, 59, 13793–13799 CrossRef CAS PubMed.
- S. Choi, T. Watanabe, T.-H. Bae, D. S. Sholl and C. W. Jones, J. Phys. Chem. Lett., 2012, 3, 1136–1141 CrossRef CAS PubMed.
- A. Gheorghe, B. Strudwick, D. M. Dawson, S. E. Ashbrook, S. Woutersen, D. Dubbeldam and S. Tanase, Chem. – Eur. J., 2020, 26, 13957–13965 CrossRef CAS PubMed.
- N. Wang, A. Mundstock, Y. Liu, A. Huang and J. Caro, Chem. Eng. Sci., 2015, 124, 27–36 CrossRef CAS.
- J. Pang, Z. Di, J.-S. Qin, S. Yuan, C. T. Lollar, J. Li, P. Zhang, M. Wu, D. Yuan, M. Hong and H.-C. Zhou, J. Am. Chem. Soc., 2020, 142, 15020–15026 CrossRef CAS PubMed.
- J. Li, S. Yuan, J.-S. Qin, J. Pang, P. Zhang, Y. Zhang, Y. Huang, H. F. Drake, W. R. Liu and H.-C. Zhou, Angew. Chem., Int. Ed., 2020, 59, 9319–9323 CrossRef CAS PubMed.
- J. Pang, S. Yuan, J.-S. Qin, C. T. Lollar, N. Huang, J. Li, Q. Wang, M. Wu, D. Yuan, M. Hong and H.-C. Zhou, J. Am. Chem. Soc., 2019, 141, 3129–3136 CrossRef CAS PubMed.
- L. Feng, Y. Wang, K. Zhang, K.-Y. Wang, W. Fan, X. Wang, J. A. Powell, B. Guo, F. Dai, L. Zhang, R. Wang, D. Sun and H.-C. Zhou, Angew. Chem., Int. Ed., 2019, 58, 16682–16690 CrossRef CAS PubMed.
- L. Feng, S. Yuan, J.-S. Qin, Y. Wang, A. Kirchon, D. Qiu, L. Cheng, S. T. Madrahimov and H.-C. Zhou, Matter, 2019, 1, 156–167 CrossRef.
- S. J. Garibay, Z. Wang, K. K. Tanabe and S. M. Cohen, Inorg. Chem., 2009, 48, 7341–7349 CrossRef CAS PubMed.
- J. Bonnefoy, A. Legrand, E. A. Quadrelli, J. Canivet and D. Farrusseng, J. Am. Chem. Soc., 2015, 137, 9409–9416 CrossRef CAS PubMed.
- R. J. Marshall, T. Richards, C. L. Hobday, C. F. Murphie, C. Wilson, S. A. Moggach, T. D. Bennett and R. S. Forgan, Dalton Trans., 2016, 45, 4132–4135 Search PubMed.
- R. J. Marshall, S. L. Griffin, C. Wilson and R. S. Forgan, Chem. – Eur. J., 2016, 22, 4870–4877 Search PubMed.
- D. Britt, C. Lee, F. J. Uribe-Romo, H. Furukawa and O. M. Yaghi, Inorg. Chem., 2010, 49, 6387–6389 Search PubMed.
- T. Toyao, K. Miyahara, M. Fujiwaki, T.-H. Kim, S. Dohshi, Y. Horiuchi and M. Matsuoka, J. Phys. Chem. C, 2015, 119, 8131–8137 Search PubMed.
- T. Liu and B. Yan, Ind. Eng. Chem. Res., 2020, 59, 1764–1771 CrossRef CAS.
- H. Ali-Moussa, R. N. Amador, J. Martinez, F. Lamaty, M. Carboni and X. Bantreil, Mater. Lett., 2017, 197, 171–174 CrossRef CAS.
- Z. Zhang, L. Zhang, L. Wojtas, P. Nugent, M. Eddaoudi and M. J. Zaworotko, J. Am. Chem. Soc., 2012, 134, 924–927 CrossRef CAS PubMed.
- S. Takaishi, E. J. DeMarco, M. J. Pellin, O. K. Farha and J. T. Hupp, Chem. Sci., 2013, 4, 1509–1513 RSC.
- M. Xu, P. Cai, S.-S. Meng, Y. Yang, D.-S. Zheng, Q.-H. Zhang, L. Gu, H.-C. Zhou and Z.-Y. Gu, Angew. Chem., Int. Ed., 2022, 61, e202207786 CrossRef CAS PubMed.
- L. Luo, W.-S. Lo, X. Si, H. Li, Y. Wu, Y. An, Q. Zhu, L.-Y. Chou, T. Li and C.-K. Tsung, J. Am. Chem. Soc., 2019, 141, 20365–20370 Search PubMed.
- R. Goswami, A. Karmakar, S. Rajput, M. Singh, S. Kundu and S. Neogi, Mater. Chem. Front., 2023, 7, 881–896 RSC.
- J. B. DeCoste, G. W. Peterson, H. Jasuja, T. G. Glover, Y. Huang and K. S. Walton, J. Mater. Chem. A, 2013, 1, 5642–5650 RSC.
- K.-Y. Wang, Z. Yang, J. Zhang, S. Banerjee, E. A. Joseph, Y.-C. Hsu, S. Yuan, L. Feng and H.-C. Zhou, Nat. Protoc., 2023, 18, 604–625 CrossRef CAS PubMed.
- O. Karagiaridi, W. Bury, J. E. Mondloch, J. T. Hupp and O. K. Farha, Angew. Chem., Int. Ed., 2014, 53, 4530–4540 CrossRef CAS PubMed.
- W. Wu, J. Su, M. Jia, Z. Li, G. Liu and W. Li, Sci. Adv., 2020, 6, eaax7270 CrossRef CAS PubMed.
- A. F. Gross, E. Sherman, S. L. Mahoney and J. J. Vajo, J. Phys. Chem. A, 2013, 117, 3771–3776 CrossRef CAS PubMed.
- H. T. T. Nguyen, T. N. Tu, M. V. Nguyen, T. H. N. Lo, H. Furukawa, N. N. Nguyen and M. D. Nguyen, ACS Appl. Mater. Interfaces, 2018, 10, 35462–35468 CrossRef CAS PubMed.
- Z. Shao, C. Huang, J. Dang, Q. Wu, Y. Liu, J. Ding and H. Hou, Chem. Mater., 2018, 30, 7979–7987 CrossRef CAS.
- D. Balestri, P. P. Mazzeo, R. Perrone, F. Fornari, F. Bianchi, M. Careri, A. Bacchi and P. Pelagatti, Angew. Chem., Int. Ed., 2021, 60, 10194–10202 CrossRef CAS PubMed.
- A. P. Nelson, D. A. Parrish, L. R. Cambrea, L. C. Baldwin, N. J. Trivedi, K. L. Mulfort, O. K. Farha and J. T. Hupp, Cryst. Growth Des., 2009, 9, 4588–4591 CrossRef CAS.
- J. Ma, A. P. Kalenak, A. G. Wong-Foy and A. J. Matzger, Angew. Chem., Int. Ed., 2017, 56, 14618–14621 Search PubMed.
- X. Zhang, V. Vieru, X. Feng, J.-L. Liu, Z. Zhang, B. Na, W. Shi, B.-W. Wang, A. K. Powell, L. F. Chibotaru, S. Gao, P. Cheng and J. R. Long, Angew. Chem., Int. Ed., 2015, 54, 9861–9865 Search PubMed.
- J. Martí-Rujas, S. Bonafede, D. Tushi and M. Cametti, Chem. Commun., 2015, 51, 12357–12360 RSC.
- B. T. Naik, M. F. Hashmi and N. D. Bokde, Appl. Sci., 2022, 12, 4429 CrossRef CAS.
- P. Rajpurkar, E. Chen, O. Banerjee and E. J. Topol, Nat. Med., 2022, 28, 31–38 CrossRef CAS PubMed.
- Z. Shi, W. Yang, X. Deng, C. Cai, Y. Yan, H. Liang, Z. Liu and Z. Qiao, Mol. Syst. Des. Eng., 2020, 5, 725–742 RSC.
- C. Janiesch, P. Zschech and K. Heinrich, Electron. Mark., 2021, 31, 685–695 CrossRef.
- I. H. Sarker, SN Comput. Sci., 2021, 2, 160 CrossRef PubMed.
- Y. Kang, H. Park, B. Smit and J. Kim, Nat. Mach. Intell., 2023, 5, 309–318 CrossRef.
- C. Zhang, Y. Xie, C. Xie, H. Dong, L. Zhang and J. Lin, MRS Bull., 2022, 47, 432–439 CrossRef.
- Y. G. Chung, E. Haldoupis, B. J. Bucior, M. Haranczyk, S. Lee, H. Zhang, K. D. Vogiatzis, M. Milisavljevic, S. Ling, J. S. Camp, B. Slater, J. I. Siepmann, D. S. Sholl and R. Q. Snurr, J. Chem. Eng. Data, 2019, 64, 5985–5998 CrossRef CAS.
- P. Z. Moghadam, A. Li, S. B. Wiggin, A. Tao, A. G. P. Maloney, P. A. Wood, S. C. Ward and D. Fairen-Jimenez, Chem. Mater., 2017, 29, 2618–2625 CrossRef CAS.
- C. R. Groom, I. J. Bruno, M. P. Lightfoot and S. C. Ward, Acta Crystallogr., Sect. B: Struct. Sci., Cryst. Eng. Mater., 2016, 72, 171–179 CrossRef CAS PubMed.
- P. Z. Moghadam, A. Li, X.-W. Liu, R. Bueno-Perez, S.-D. Wang, S. B. Wiggin, P. A. Wood and D. Fairen-Jimenez, Chem. Sci., 2020, 11, 8373–8387 RSC.
- L. T. Glasby, K. Gubsch, R. Bence, R. Oktavian, K. Isoko, S. M. Moosavi, J. L. Cordiner, J. C. Cole and P. Z. Moghadam, Chem. Mater., 2023, 35, 4510–4524 CrossRef CAS PubMed.
-
G. Sastre and F. Daeyaert, AI-guided design and property prediction for zeolites and nanoporous materials, John Wiley & Sons, 2023 Search PubMed.
- Y. G. Chung, J. Camp, M. Haranczyk, B. J. Sikora, W. Bury, V. Krungleviciute, T. Yildirim, O. K. Farha, D. S. Sholl and R. Q. Snurr, Chem. Mater., 2014, 26, 6185–6192 CrossRef CAS.
- H. Daglar, H. C. Gulbalkan, G. Avci, G. O. Aksu, O. F. Altundal, C. Altintas, I. Erucar and S. Keskin, Angew. Chem., Int. Ed., 2021, 60, 7828–7837 CrossRef CAS PubMed.
- S. Kancharlapalli and R. Q. Snurr, ACS Appl. Mater. Interfaces, 2023, 15, 28084–28092 CrossRef CAS PubMed.
- Y. He, E. D. Cubuk, M. D. Allendorf and E. J. Reed, J. Phys. Chem. Lett., 2018, 9, 4562–4569 CrossRef CAS PubMed.
- Y. Kang and J. Kim, Nat. Commun., 2024, 15, 4705 Search PubMed.
- Y. Luo, S. Bag, O. Zaremba, A. Cierpka, J. Andreo, S. Wuttke, P. Friederich and M. Tsotsalas, Angew. Chem., Int. Ed., 2022, 61, e202200242 CrossRef CAS PubMed.
- N. S. Bobbitt, K. Shi, B. J. Bucior, H. Chen, N. Tracy-Amoroso, Z. Li, Y. Sun, J. H. Merlin, J. I. Siepmann, D. W. Siderius and R. Q. Snurr, J. Chem. Eng. Data, 2023, 68, 483–498 Search PubMed.
- C. E. Wilmer, M. Leaf, C. Y. Lee, O. K. Farha, B. G. Hauser, J. T. Hupp and R. Q. Snurr, Nat. Chem., 2012, 4, 83–89 CrossRef CAS PubMed.
- Y. J. Colón, D. A. Gómez-Gualdrón and R. Q. Snurr, Cryst. Growth Des., 2017, 17, 5801–5810 CrossRef.
-
C. Baerlocher, L. B. McCusker and D. H. Olson, Atlas of zeolite framework types. Elsevier, 2007 Search PubMed.
- S. Grazulis, D. Chateigner, R. T. Downs, A. F. T. Yokochi, M. Quiros, L. Lutterotti, E. Manakova, J. Butkus, P. Moeck and A. L. Bail, J. Appl. Cryst., 2009, 42, 726–729 Search PubMed.
-
M. Mohammed, M. B. Khan and E. B. M. Bashier, Machine learning: algorithms and applications. Crc Press, 2016 Search PubMed.
- X. Zhang, K. M. Jablonka and B. Smit, Digital Discovery, 2024, 3, 1410–1420 RSC.
- G. Zhang, Y. Liang, G. Cui, B. Dou, W. Lu, Q. Yang and X. Yan, Energy Rep., 2023, 9, 2852–2860 CrossRef.
- M. Ernst, J. D. Evans and G. Grynova, Chem. Phys. Rev., 2023, 4, 041303 Search PubMed.
- J. A. Greathouse, T. L. Kinnibrugh and M. D. Allendorf, Ind. Eng. Chem. Res., 2009, 48, 3425–3431 CrossRef CAS.
- N. A. Ramsahye, G. Maurin, S. Bourrelly, P. L. Llewellyn, T. Devic, C. Serre, T. Loiseau and G. Ferey, Adsorption, 2007, 13, 461–467 CrossRef CAS.
- H. Park, X. Yan, R. Zhu, E. A. Huerta, S. Chaudhuri, D. Cooper, I. Foster and E. Tajkhorshid, Commun. Chem., 2024, 7, 21 CrossRef CAS PubMed.
- M. Gheytanzadeh, A. Baghban, S. Habibzadeh, A. Esmaeili, O. Abida, A. Mohaddespour and M. T. Munir, Sci. Rep., 2021, 11, 15710 CrossRef CAS PubMed.
- M. Gheytanzadeh, A. Baghban, S. Habibzadeh, K. Jabbour, A. Esmaeili, A. Mohaddespour and O. Abida, Sci. Rep., 2022, 12, 6615 CrossRef CAS PubMed.
- X. Lu, Z. Xie, X. Wu, M. Li and W. Cai, Chem. Eng. Sci., 2022, 259, 117813 CrossRef CAS.
- Z. Zhang and B. Yan, ACS Sens., 2023, 8, 3585–3594 CrossRef CAS PubMed.
- R. Wang, Y. Zou, C. Zhang, X. Wang, M. Yang and D. Xu, Microporous Mesoporous Mater., 2022, 331, 111666 CrossRef CAS.
- H. Wang, Y. Xie, D. Li, H. Deng, Y. Zhao, M. Xin and J. Lin, J. Chem. Inf. Model., 2020, 60, 2004–2011 CrossRef CAS PubMed.
- K. Choudhary and B. DeCost, npj Comput. Mater., 2021, 7, 185 CrossRef.
- K. Choudhary, T. Yildirim, D. W. Siderius, A. G. Kusne, A. McDannald and D. L. Ortiz-Montalvo, Comput. Mater. Sci., 2022, 210, 111388 CrossRef CAS.
- Z. Zheng, O. Zhang, H. L. Nguyen, N. Rampal, A. H. Alawadhi, Z. Rong, T. Head-Gordon, C. Borgs, J. T. Chayes and O. M. Yaghi, ACS Cent. Sci., 2023, 9, 2161–2170 CrossRef CAS PubMed.
- Z. Zheng, Z. Rong, N. Rampal, C. Borgs, J. T. Chayes and O. M. Yaghi, Angew. Chem., Int. Ed., 2023, 62, e202311983 CrossRef CAS PubMed.
- S. M. Moosavi, A. Chidambaram, L. Talirz, M. Haranczyk, K. C. Stylianou and B. Smit, Nat. Commun., 2019, 10, 539 CrossRef CAS PubMed.
- N. P. Domingues, S. M. Moosavi, L. Talirz, K. M. Jablonka, C. P. Ireland, F. M. Ebrahim and B. Smit, Commun. Chem., 2022, 5, 170 CrossRef CAS PubMed.
- L. Pilz, C. Natzeck, J. Wohlgemuth, N. Scheuermann, P. G. Weidler, I. Wagner, C. Wöll and M. Tsotsalas, Adv. Mater. Interfaces, 2023, 10, 2201771 CrossRef CAS.
- X. He, J. Chen, S. Albin, Z. Zhu and W.-N. Wang, Adv. Powder Tech., 2021, 32, 266–271 CrossRef CAS.
- H. Demir and S. Keskin, Macromol. Mater. Eng., 2024, 309, 2300225 CrossRef CAS.
- L. Yao, Y. Li, Q. Cheng, Z. Chen and J. Song, Desalination, 2022, 532, 115729 CrossRef CAS.
- G. Alatrista, C. Pratt and A. E. Hanandeh, Chemosphere, 2023, 339, 139674 CrossRef CAS PubMed.
- S. Ghude and C. Chowdhury, Chem. – Eur. J., 2023, 29, e202301840 CrossRef CAS PubMed.
- Z. Yıldız and H. Uzun, Microporous Mesoporous Mater., 2015, 208, 50–54 CrossRef.
- Y. Cao, H. A. Dhahad, S. G. Zare, N. Farouk, A. E. Anqi, A. Issakhov and A. Raise, Int. J. Hydrogen Energy, 2021, 46, 36336–36347 CrossRef CAS.
- M. Pardakhti, E. Moharreri, D. Wanik, S. L. Suib and R. Srivastava, ACS Comb. Sci., 2017, 19, 640–645 CrossRef CAS PubMed.
- X. Yuan, X. Deng, C. Cai, Z. Shi, H. Liang, S. Li and Z. Qiao, Green Energy Environ., 2021, 6, 759–770 CrossRef CAS.
- Z. Gulsoy, K. B. Sezginel, A. Uzun, S. Keskin and R. Yildirim, ACS Comb. Sci., 2019, 21, 257–268 CrossRef CAS PubMed.
- R. Anderson, J. Rodgers, E. Argueta, A. Biong and D. A. Gómez-Gualdrón, Chem. Mater., 2018, 30, 6325–6337 CrossRef CAS.
- F. Cipcigan, J. Booth, R. N. Barros Ferreira, C. Ribeiro dos Santos and M. Steiner, Digital Discovery, 2024, 3, 449–455 RSC.
- T. D. Burns, K. N. Pai, S. G. Subraveti, S. P. Collins, M. Krykunov, A. Rajendran and T. K. Woo, Environ. Sci. Technol., 2020, 54, 4536–4544 CrossRef CAS PubMed.
- J. Burner, J. Luo, A. White, A. Mirmiran, O. Kwon, P. G. Boyd, S. Maley, M. Gibaldi, S. Simrod, V. Ogden and T. K. Woo, Chem. Mater., 2023, 35, 900–916 CrossRef CAS.
- P. Halder and J. K. Singh, Energy Fuels, 2020, 34, 14591–14597 CrossRef CAS.
- H. Liang, K. Jiang, T. A. Yan and G. H. Chen, ACS Omega, 2021, 6, 9066–9076 Search PubMed.
- H. Daglar and S. Keskin, ACS Appl. Mater. Interfaces, 2022, 14, 32134–32148 Search PubMed.
- A. S. Rosen, S. M. Iyer, D. Ray, Z. Yao, A. Aspuru-Guzik, L. Gagliardi, J. M. Notestein and R. Q. Snurr, Matter, 2021, 4, 1578–1597 CrossRef CAS.
- Y. Ma, Y. Leng, D. Huo, D. Zhao, J. Zheng, P. Zhao, H. Yang, F. Li and C. Hou, Food Chem., 2023, 429, 136850 CrossRef CAS PubMed.
- C. M. Draznieks, J. M. Newsam, A. M. Gorman, C. M. Freeman and G. Férey, Angew. Chem., Int. Ed., 2000, 39, 2270–2275 Search PubMed.
- B. J. Sikora, C. E. Wilmer, M. L. Greenfield and R. Q. Snurr, Chem. Sci., 2012, 3, 2217–2223 RSC.
- D. A. Gomez-Gualdron, O. V. Gutov, V. Krungleviciute, B. Borah, J. E. Mondloch, J. T. Hupp, T. Yildirim, O. K. Farha and R. Q. Snurr, Chem. Mater., 2014, 26, 5632–5639 CrossRef CAS.
- D. A. Gómez-Gualdrón, Y. J. Colón, X. Zhang, T. C. Wang, Y.-S. Chen, J. T. Hupp, T. Yildirim, O. K. Farha, J. Zhang and R. Q. Snurr, Energy Environ. Sci., 2016, 9, 3279–3289 Search PubMed.
- E. Taw and J. B. Neaton, Adv. Theory Simul., 2022, 5, 2100515 CrossRef CAS.
- M. Fernandez and A. S. Barnard, ACS Comb. Sci., 2016, 18, 243–252 CrossRef CAS PubMed.
- G. S. Fanourgakis, K. Gkagkas, E. Tylianakis, E. Klontzas and G. Froudakis, J. Phys. Chem. A, 2019, 123, 6080–6087 CrossRef CAS PubMed.
- X. Wu, S. Xiang, J. Su and W. Cai, J. Phys. Chem. C, 2019, 123, 8550–8559 Search PubMed.
- P. G. Boyd, A. Chidambaram, E. García-Díez, C. P. Ireland, T. D. Daff, R. Bounds, A. Gładysiak, P. Schouwink, S. M. Moosavi, M. M. Maroto-Valer, J. A. Reimer, J. A. R. Navarro, T. K. Woo, S. Garcia, K. C. Stylianou and B. Smit, Nature, 2019, 576, 253–256 CrossRef CAS PubMed.
- Z. Yao, B. Sánchez-Lengeling, N. S. Bobbitt, B. J. Bucior, S. G. H. Kumar, S. P. Collins, T. Burns, T. K. Woo, O. K. Farha, R. Q. Snurr and A. Aspuru-Guzik, Nat. Mach. Intell., 2021, 3, 76–86 CrossRef.
- H. Ohno and Y. Mukae, J. Phys. Chem. C, 2016, 120, 23963–23968 CrossRef CAS.
- X. Yuan, L. Li, Z. Shi, H. Liang, S. Li and Z. Qiao, Adv. Powder Mater., 2022, 1, 100026 CrossRef.
- J. Lin, H. Zhang, M. Asadi, K. Zhao, L. Yang, Y. Fan, J. Zhu, Q. Liu, L. Sun, W. J. Xie, C. Duan, F. Mo and J.-H. Dou, Chem. Mater., 2024, 36, 5436–5445 CrossRef CAS.
- Y. Bao, R. L. Martin, C. M. Simon, M. Haranczyk, B. Smit and M. W. Deem, J. Phys. Chem. C, 2015, 119, 186–195 CrossRef CAS.
- Y. G. Chung, D. A. Gómez-Gualdrón, P. Li, K. T. Leperi, P. Deria, H. Zhang, N. A. Vermeulen, J. F. Stoddart, F. You, J. T. Hupp, O. K. Farha and R. Q. Snurr, Sci. Adv., 2016, 2, e1600909 CrossRef PubMed.
- S. P. Collins, T. D. Daff, S. S. Piotrkowski and T. K. Woo, Sci. Adv., 2016, 2, e1600954 CrossRef PubMed.
- J. Park, Y. Lim, S. Lee and J. Kim, Chem. Mater., 2023, 35, 9–16 CrossRef CAS.
- S. K. Singh, A. T. Sose, F. Wang, K. K. Bejagam and S. A. Deshmukh, J. Chem. Theory Comput., 2023, 19, 6686–6703 CrossRef CAS PubMed.
- S. Lee, B. Kim, H. Cho, H. Lee, S. Y. Lee, E. S. Cho and J. Kim, ACS Appl. Mater. Interfaces, 2021, 13, 23647–23654 Search PubMed.
- S. Han and J. Kim, ACS Omega, 2023, 8, 4278–4284 Search PubMed.
- X. Zhang, K. Zhang and Y. Lee, ACS Appl. Mater. Interfaces, 2020, 12, 734–743 CrossRef CAS PubMed.
- T. Xiong, J. Cui, Z. Hou, X. Yuan, H. Wang, J. Chen, Y. Yang, Y. Huang, X. Xu, C. Su and L. Leng, J. Environ. Manage., 2023, 347, 119065 CrossRef CAS PubMed.
- J. Abdi and G. Mazloom, Sci. Rep., 2022, 12, 16458 Search PubMed.
- M. M. Ibrahim, M. A. Alnuwaiser, E. B. Elkaeed, H. Kotb, S. Alshehri and M. A. S. Abourehab, Arabian J. Chem., 2022, 15, 104261 CrossRef CAS.
- Z. Liu, D. Shen, S. Cai, Z. Tu and S. Li, J. Mater. Chem. A, 2023, 11, 19455–19464 RSC.
- M. Pardakhti, E. Moharreri, D. Wanik, S. L. Suib and R. Srivastava, ACS Comb. Sci., 2017, 19, 640–645 CrossRef CAS PubMed.
- L. Li, Y. Zhao, H. Yu, Z. Wang, Y. Zhao and M. Jiang, Langmuir, 2023, 39, 6756–6766 CrossRef CAS PubMed.
- T. Bailey, A. Jackson, R. A. Berbece, K. Wu, N. Hondow and E. Martin, J. Chem. Inf. Model., 2023, 63, 4545–4551 CrossRef CAS PubMed.
- M. Gheytanzadeh, A. Baghban, S. Habibzadeh, A. Esmaeili, O. Abida, A. Mohaddespour and M. T. Munir, Sci. Rep., 2021, 11, 15710 CrossRef CAS PubMed.
- Y. Cao, H. A. Dhahad, S. G. Zare, N. Farouk, A. E. Anqi, A. Issakhov and A. Raise, Int. J. Hydrogen Energy, 2021, 46, 36336–36347 CrossRef CAS.
- K. Choudhary, T. Yildirim, D. W. Siderius, A. G. Kusne, A. McDannald and D. L. Ortiz-Montalvo, Comput. Mater. Sci., 2022, 210, 111388 CrossRef CAS.
- R. Wang, Y. Zou, C. Zhang, X. Wang, M. Yang and D. Xu, Micro. Meso. Mater., 2022, 331, 111666 CrossRef CAS.
- Y. Yan, Z. Shi, H. Li, L. Li, X. Yang, S. Li, H. Liang and Z. Qiao, Chem. Eng. J., 2022, 427, 131604 CrossRef CAS.
- Z. Liu, K. Zhang, Q. Xia, X. Wang, B. Huang and H. Xi, Chem. Eng. Sci., 2021, 243, 116772 CrossRef CAS.
- X. Liu, Y. Wang, J. Yuan, X. Li, S. Wu, Y. Bao, Z. Feng, F. Ou and Y. He, Bioengineering, 2022, 9, 517 CrossRef CAS PubMed.
- Y. Ma, Y. Leng, D. Huo, D. Zhao, J. Zheng, P. Zhao, H. Yang, F. Li and C. Hou, Food Chem., 2023, 429, 136850 CrossRef CAS PubMed.
|
This journal is © The Royal Society of Chemistry 2025 |
Click here to see how this site uses Cookies. View our privacy policy here.