DOI:
10.1039/D4PM00323C
(Review Article)
RSC Pharm., 2025,
2, 437-454
AI-driven innovations in pharmaceuticals: optimizing drug discovery and industry operations
Received
26th November 2024
, Accepted 2nd February 2025
First published on 3rd February 2025
Abstract
The integration of artificial intelligence into the pharmaceutical industry has led to significant transformation in the process of drug discovery and development and management of the pharmaceutical sector. Artificial intelligence has accelerated the process of drug discovery by several folds owing to its ability to analyse large datasets and predict drug–target(receptor) interactions, which effectively reduces the time and expenditure. AI enables clinical trial design and patient recruitment through predictive analytics during the trial. It also allows for real-time tracking of patient outcomes and predicts the effectiveness of a trial. Artificial intelligence-driven automation also assists in manufacturing and supply chain processes, enabling inventory optimization and predictive maintenance and thereby improving the productivity as well as affordability of these processes. The current review discusses various key applications, prospects, and challenges of AI in the pharmaceutical industry, focussing on its transformative potential while addressing the need for ethical and regulatory frameworks to ensure equitable and safe AI adoption.
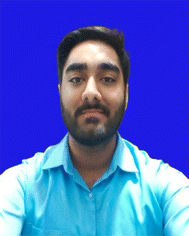 Jaskaran Preet Singh Saini | Jaskaran Preet is an undergraduate student at the School of Pharmacy, Chitkara University, Himachal Pradesh, India. His current research interests include application of AI systems in pharmaceutical manufacturing and pharmaceutical management. |
 Ankita Thakur | Ankita Thakur is a Junior Research Fellow at the School of Pharmacy, Chitkara University, Himachal Pradesh, India. She did her master's in Pharmaceutics from Baddi University of Emerging Sciences and Technology, HP, India, where she was awarded a gold medal for academic excellence. She also has experience in academia as an Assistant Professor. Her research focuses on the development of novel drug delivery systems, especially nano-based formulations for challenging molecules. |
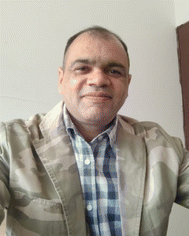 Deepak Yadav | Dr Deepak Yadav is Professor of Pharmaceutics at School of Pharmacy, Chitkara University, Himachal Pradesh, India. He earned practical experience as a pharmaceutical formulation scientist in multinational pharmaceutical companies. Besides, he completed postdoc fellowships, one from the Hebrew University of Jerusalem, Israel, and another one from the University of Nottingham, UK. His current research interests include development, characterization and evaluation of conventional and novel drug delivery systems for unmet clinical needs, and application of AI systems in pharmaceutical sciences. |
1. Introduction
The development of artificial intelligence (AI) is changing the drug discovery, development, and management paradigm in the pharmaceutical industry. Thus far, drug discovery and development have been conventionally long and expensive processes that have taken as long as 10–15 years, and billions of dollars need to be spent for one molecule to come to the market (Fig. 1).1 Artificial intelligence has emerged as a powerful tool that can steer this process by streamlining each stage, including drug discovery and clinical trials, manufacturing, and even supply chain management. Its ability to analyse big data and find patterns to offer efficient and precise predictions points towards even more innovations in the industry.
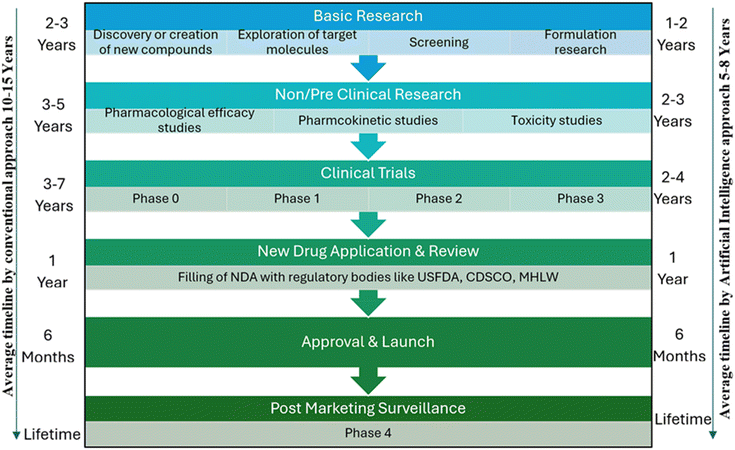 |
| Fig. 1 Comparative representation of how AI can impact drug discovery and approval processes in terms of timelines for different key steps. | |
AI-based technologies, which include machine learning, natural language processing, and deep learning, are being applied to address the critical issues in pharmaceuticals. For instance, artificial intelligence-based drug discovery platforms accelerate the identification of likely candidates by modelling complex systems of biology and estimating the drug–target interactions with greater precision. Further, AI is making clinical trials faster and more efficient by streamlining the processes of recruitment, trial design, and real-time monitoring, thereby shortening the cycles of drug development while cutting costs.
In the pharmaceutical manufacturing field, AI holds potential for revolutionizing processes through predictive maintenance, process automation, and quality control, and it is used in the supply chain management that optimizes logistics, predicts demand, and manages inventory effectively. Improving patients’ results using AI is be the second focus area. AI, upon analysing the genetic and clinical data, tailors the treatments to individual patients, hence increasing the efficacy and decreasing the adverse effects.2,3
Although AI adoption has significant advantages, it faces certain challenges in the pharmaceutical sector. While many positive outcomes have resulted from AI integration in this sector, there are always challenges that accompany these advancements. Critical areas that need to be addressed to fully realize the potential of AI include data privacy issues, issues with bias in algorithms, and regulatory frameworks governing AI-driven processes. However, the combinations of breakthroughs in quantum computing may open new avenues by which AI would become significantly more powerful and beneficial to drug discovery and development activities.3–5
This article will delve deep into the influence of AI on the pharmaceutical industry, from discovery through phases of development, manufacturing, and personalized medicine (Fig. 2), with regard to the ways and mechanisms through which such impacts may differ at various levels. Most probably, it will discuss the challenges and ethical considerations when AI is embraced and look forward to future developments in the succeeding decades that will shape the industry.
 |
| Fig. 2 Different processes involved in the pharmaceutical industry where AI can be potentially applied. | |
2. How AI works?
AI works by artificial neural networks (ANNs), machine learning (ML) and deep learning (DL) methods through data analysis, learning and application. Text, graphics, sound, and other data types that can be encoded can all be included in the input data format. Inspired by the networks of biological neurons, an artificial neural network (ANN) is a computational model in which inputs are converted into output values by the neurons. Even in a complicated, noisy, and highly nonlinear environment, the ANN can learn and self-correct. The ANN is commonly used in scenarios where the dataset is extensive and cannot be addressed using linear functions. Multilayers are structured as concealed layers of neurons that have a diverse range of neuron counts. Input variables are chosen from the existing information (for example, drug discovery and drug parameters) such as concentration, compounds, and ligands, and are refined through several training stages to achieve the output. The anticipated value is examined and contrasted with the known value. The disparity between predicted and actual values is traced back until the difference is minimal to obtain the estimated drug values. This is an extremely intricate simultaneous procedure. Thus, ANN is a computational or mathematical model that relies on a machine learning (ML) technique.6
Like the human learning model, machine learning (ML) and deep learning (DL) may progressively identify various data features, deduce the patterns contained within, and continuously update their model parameters until a reliable model is created. These techniques do not need direct programming. ML creates a unique algorithm that concentrates on the data's characteristics and turns them into machine-readable knowledge that gives researchers fresh perspectives. Based on the application contexts, the models can be divided into regression models and classification models. The distinction between regression and classification tasks primarily depends on whether the output variable is continuous or discrete. Based on the type of learning algorithm needed to address the issue, models are classified into three groups: supervised learning, unsupervised learning, and reinforcement learning. Supervised learning is a process that utilizes labelled data to train a model on the connection between input and its predefined output, enabling it to forecast the categories or continuous variables of future inputs. In contrast, unsupervised techniques are employed to detect patterns in datasets without labels and to examine a dataset's possible structures, facilitating the clustering of data for additional analysis. Reinforcement learning builds models by continually engaging in interactive learning, using punishments for setbacks and incentives for achievements. There are several popular algorithms available for researchers to select from. A Random Forest algorithm creates an entire hierarchical structure from a collection of unconnected decision trees, each of which is in charge of a distinct challenge during model development.7 The decision trees’ majority vote determines the ultimate choice. One popular machine learning approach is support vector machines (SVMs). A number of additional machine learning models including logistic regression (LR), linear discriminant analysis (LDA), principal component analysis (PCA), and partial least-squares (PLS) have been used in pharmaceutical and biological data processing.8,9
Deep learning (DL) has gained popularity because of its impressive capabilities in generalization and feature extraction; its process for learning and making predictions is conducted in a seamless, end-to-end manner. In contrast to the conventional machine learning (ML) approach, which often involves several separate modules, DL produces output data (output-end) directly from input data (input-end) during the training of the model and continuously refines and enhances the model based on the discrepancies between the output and the actual value, until the desired outcome is achieved. A deep neural network (DNN) is a type of feed-forward neural network comprising closely connected input, hidden, and output layers. A recurrent neural network (RNN) is a type of artificial neural network, where connected nodes create a directed or undirected graph over a time sequence. An RNN features a feedback element that enables signals from one layer to be sent back to the prior layer. It is the sole neural network equipped with internal memory, aiding in the challenge of learning and retaining long-term information.10
An autoencoder (AE), which comprises an encoder and a decoder, is used to learn effective representations of input data. The encoder produces an encoding by processing the input, which the decoder then uses to reconstruct the original input. Typically, an AE is employed for purposes such as data compression and dimensionality reduction by utilizing the representation methods (i.e., the encoding) of a given dataset.11 A dataset intended for a model is generally separated into a training set, a validation set, and a test set. These sets serve the specific functions of training the model, fine-tuning the model, and assessing the model's performance, respectively.
3. Success and failure rate of AI
It is obvious that models are only a rough depiction of reality and are only useful in specific situations. Complex experimental techniques must be used to determine the materials and process parameters. Each model and simulation technique must be proven to be accurate, dependable, and capable of making predictions. For the majority of engineering applications, an agreement between simulation and experiment in the range of 10% is deemed sufficiently accurate. ANNs were employed by Ebube et al. to forecast the physiochemical characteristics of amorphous polymers.12 The model was able to predict accurately (with an error of 0–8%) the correlations between glass transition temperatures, viscosity, and water absorption patterns and the chemical composition of the polymer. For IVIVC, using in vitro data and parameters derived from the physiological traits of human volunteers, DeMatas et al. created an ANN model that forecasted the in vivo effectiveness of dry powder inhaler formulations. The ANN model was a highly successful IVIVC tool for inhaled medications, according to the results (R2 ≈ 80%), although substantial enhancements to the model can be achieved by incorporating important input variables, using larger datasets, and increasing the number of subjects.13 Multiple ANNs were created and evaluated in clinical environments for the detection, treatment, and prognosis of cancer. Colorectal cancer was identified through the evaluation of fluorescence (from biological fluorophores) with the use of ANNs. The sensitivity and specificity were determined to be 99.2% and 99.4%, respectively.14 In gastric cancer, the prediction of cancer metastasis was conducted through lymph node biopsy. The precision of ANN-1 was 79%, the sensitivity stood at 88%, and the specificity was 55%.15 In pancreatic cancer, the neural computing method achieved a training accuracy of 91.14% and a testing accuracy of 84.27%, along with a positive predictive value of 96.25% and a negative predictive value of 57.22%.16 Wessel, Jurs, Tolan, and Muskal used an artificial neural network (ANN) to predict the intestinal absorption of new compounds, resulting in an error rate of 16%. This result is viewed as acceptable, especially given the varied structure within the dataset.6,17
4. AI and its applications in drug discovery
The discovery of drugs is typically slow and costly, taking years and vast amounts of resources before there is a glimmer of hope in the form of an identified candidate that might make it to clinical trials. With AI, one could, in theory shave years off timelines, reduce costs, and give drug candidates a better chance of success. AI can handle massive data to predict how chemical compounds could interact and work to model biological systems, which positions it to change the drug-discovery game.
4.1 Accelerating drug discovery
Drug discovery is a laborious process that searches chemical compounds in an effort to find those that may well function therapeutically against some diseases. It takes years if carried out classically since researchers will more often than not rely on trial and error. AI is a game-changer. ML algorithms are used in order to analyse large datasets of chemical compounds for predicting potential biological activity (Table 1). AI models are especially identified to be applicable in SAR modelling, which is the technique for predicting the impact of molecular structure on biological activity.18
Table 1 AI Applications in drug discovery
AI Model/method |
Application |
Case study |
Impact |
Machine learning |
Screening chemical compounds |
IBM Watson for Drug Discovery |
Accelerated candidate identification |
Deep learning |
Predicting drug–target interactions |
AlphaFold by Deep Mind (Google) |
Accurate protein structure predictions |
Molecular dynamics |
Simulating drug efficacy in silico |
BenevolentAI for COVID-19 drug repurposing |
Faster identification of drug candidates |
4.1.1 AI and chemical screening.
Machine Learning Algorithms can quickly sieve through huge libraries of chemicals and look for the most promising compounds. Some algorithms applied in tasks of chemical screening include Random Forest, SVM, and neural networks. For example, SVM can classify molecules according to some of their structural features, which helps predict biological activity. Deep learning, a subset of ML, holds even greater promise; models based on CNNs, for instance, can very effectively analyse the 3D structures of compounds to predict the drug efficacy.19
4.1.2 Structure–activity relationship modelling.
Another big use of AI is the structure–activity relationship modelling in which changes in the structure of a molecule reflect its interaction with biological targets. AI algorithms do this automatically by scanning patterns on already existing data that would give predictions on new compounds. By modelling SARs, AI models help research scientists pinpoint the best candidates for drug development, hopefully saving time and other resources.20
4.2 AI Models for drug–target interactions
Perhaps the most difficult step of drug discovery is to understand the drug–target interaction, which simply put, means predicting the chances of a drug binding to a biological target, such as a protein. In this, AI excels, particularly through its deep learning and molecular dynamics simulation tools.
4.2.1 Deep learning models.
Recurrent neural networks (RNNs) and generative adversarial networks (GANs) are gaining popularity for drug–target interaction prediction. They are capable of learning large chemical and biological database to identify potential drug candidates that will interact best with disease/target cells. It not only accelerates the identification process of effective drugs but also facilitates the design of novel molecules with desirable properties.21
4.2.2 Applications in molecular dynamics simulations.
AI also finds applications in molecular dynamics simulations; it predicts the behaviour of molecules over some period of time within the biological system. In this regard, through the simulation of motion, whether by atoms or by molecules, AI can enable the researcher to comprehend the stability as well as the efficacy of drug candidates before they are put into a laboratory test. Ligand-Based Virtual Screening (LBVS) has been reported.22–24 In their research, Li et al. implemented various machine learning techniques to develop classification models aimed at identifying liver X receptor (LXR) agonists.25 This endeavor involved the utilization of optimized property descriptors and topological fingerprints to effectively characterize small molecules within the database, resulting in the creation of a total of 324 models using four distinct algorithms: Naive Bayes (NB), Support Vector Machine (SVM), K-Nearest Neighbors (KNN), and Recursive Partitioning (RP). From these, the top 15 models were chosen for further evaluation, with ten of them achieving an accuracy exceeding 90%.26 BRGNN has been successfully trained to predict the biological activity in various cancer targets.27,28 These neural networks were assessed through cross-validation and test set prediction experiments, achieving accuracy rates exceeding 65% across all datasets.29–31 The models of BRGNN trained on 11,12-cyclic carbamate derivatives of 6-O-methylerythromycin A exhibited a prediction accuracy of 70% for LHRH antagonist activity.32 Additionally, BRGNN models investigating the inhibition of acetylcholinesterase by tacrine analogs33 and huprine compounds34 utilized a comprehensive array of 3D descriptors. The model for tacrines achieved an average test accuracy of approximately 71%,30 while the dataset of huprine analogs consistently yielded a high accuracy of 85%.34 Furthermore, BRGNNs were effectively trained to predict the inhibitory activity against HIV-1 protease of cyclic urea derivatives,30 employing 2D descriptors and attaining a validation accuracy of 70%. The PASS (Prediction of Activity Spectra for Substances) tool was developed to evaluate the biological activity spectrum of small molecules with molecular weights ranging from 50 to 1250 Dalton. The findings provided a classification of potential pharmacological effects, mechanisms of action, toxic and adverse effects, antitargets, and metabolic pathways, with an average prediction accuracy of approximately 95%.35 SmartMLP-1.5 successfully distinguished between anticancer and nonactive compounds with an accuracy of 94.04%.29
4.3 Examples and case studies
4.3.1 AlphaFold by Google DeepMind.
AlphaFold is the pioneer in AI in drug discovery made by DeepMind, an AI system that predicts protein 3D structures from its amino acid sequence with never-before-seen accuracy. Proteins are involved in most biological processes, and knowing their 3D structures would make it easier to design effective drugs. Previously, it took years of hard work by scientists to arrive at protein structures. AlphaFold predicts those structures incredibly fast and accurately, which has a very huge impact on drug discovery (Fig. 3). Indeed, it accelerates the rate of discovery of drug targets and a step further, speeds up the design of the molecules that can interact with them.36
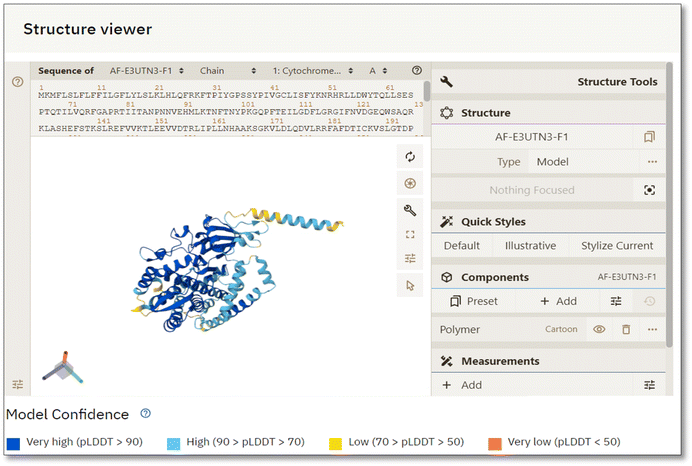 |
| Fig. 3 Screenshot of AlphaFold depicting the 3D structure of the protein CYP-450A (feeding input of a series of amino acids will result in the 3D structure prediction of the protein in space). | |
4.3.2 The case of DSP-1181.
The development of DSP-1181 is an AI-designed drug candidate for obsessive-compulsive disorder (OCD). It is a remarkable case of integrating artificial intelligence into drug discovery. Exscientia, in partnership with Sumitomo Dainippon Pharma, designed and optimized the drug using AI within 12 months; instead of the typical 4–5 years, it would take using traditional drug discovery processes. The AI platform analysed huge data sets, pinpointed target interactions, and optimized promising molecules, ensuring to achieve specificity and lowering costs and failure rates. In early 2020, DSP-1181 was the first AI-designed drug to be entered into human clinical trials, thereby heralding a new era in pharmaceutical development. This case epitomizes how AI can revolutionize the acceleration of drug discovery and increase the efficiency to solve long-standing medical problems.37
4.4 Impact of AI on drug discovery efficiency
There are myriads of advantages of including AI in drug discovery. AI saves enormous time, money, and effort put into a discovery by sieving the promising candidates with resultant focus resources on those candidates. It deals with vast quantities of data much faster than a human can, whereby researchers can quickly identify likely drug candidates and filter out unlikely ones. This leads to the potential for a more targeted approach with less expenditure in lab testing and clinical trials. Besides this, the predictability of AI in drug–target interactions and molecular dynamic simulation improves the accuracy of predictions, and hence, the success rate of drug development.
5. AI in drug development and clinical trials
Since its inception AI has been readily studied for its ability to enhance the conduction of clinical trials, and certain modifications to the current AI models can make them more compatible with clinical trials.
5.1 Conventional drug development and clinical trial phases
Drug development and its associated clinical trials are now faster with more resources as compared to the old-fashioned, time-consuming, and resource-consumptive procedures. The ability to use machine learning models, predictive analytics, and AI-based monitoring tools facilitates pharmaceutical companies accelerating the end-to-end clinical trial design, patient recruitment, and monitoring, resulting in new therapies hitting the markets faster (Table 2).38
Table 2 Applications of AI in clinical trials
Clinical trial stage |
AI tools/method |
Function |
Example |
Patient recruitment |
Predictive analytics, EHR analysis |
Identify ideal candidates based on genetic and clinical data |
Tempus for cancer trials |
Trial design optimization |
In silico simulation. ML models |
Optimize trial structure (e.g. dosage duration) |
Pfizer AI-optimized trial for vaccines |
Real time monitoring |
NLP, image recognition |
Monitor patient outcomes and adverse reactions in real time |
AI powered wearable devices |
5.2 Optimizing clinical trial design
These are also the costliest and most time-consuming activities of drug development, taking years and millions of dollars. The new applications of AI provide a solution to both problems. It can optimize the design of a clinical trial, predict patient outcomes, and even conduct virtual trials through in silico simulations. ML models driven by AI can analyse huge datasets from previous trials, electronic health records, and genomic data to identify patterns that researchers can use in their research for developing more effective trials.39
5.2.1
In silico simulations.
AI allows in silico or computer-based trials in which algorithms could mimic human trials by using large volumes of biological data, thereby enabling scientists to predict the safety and efficacy of drugs even before real physical trials begin. Pharmaceutical companies could quickly check several clinical scenarios and figure out the most promising candidates through fewer physical trials by saving much time and resources.39
5.2.2 Design optimization.
The machine-learning algorithms can be used to optimize the parameters of the clinical trials, for example, selecting perfect demographics and determining the right dose and the right time of the clinical trial. AI assists in creating the designs, so that maximum chances of success are there with minimum risk on patients as well as on resources, in addition to modelling based on historical time outcomes.39 Machine learning models can simulate different clinical trial designs to predict potential outcomes, helping researchers choose the most efficient protocol. AI can analyze electronic health records (EHRs) to identify patients most likely to meet trial inclusion criteria, improving patient matching and recruitment efficiency.40 AI models can predict which patients are more likely to drop out of a trial, allowing researchers to proactively address potential issues and improve retention rates. AI can identify high-potential recruitment areas based on demographics and access healthcare facilities. AI can analyze historical data to more accurately estimate the required sample sizes, potentially reducing the number of participants needed.41 It can enable real-time adjustments to clinical trial parameters such as sample size or treatment arms based on interim data analysis, leading to faster and more accurate results. It can detect errors and inconsistencies in clinical trial data, improving data quality and reliability. AI algorithms can flag potential safety concerns early on, allowing for timely interventions.42
5.3 Predictive modelling for patient recruitment and outcomes
Recruitment of patients to clinical trials has been identified as one of the major bottlenecks in the process of drug development. One of the areas where AI has proven itself to be valuable is in hastening and more accurately identifying potential candidates for a particular clinical trial. Different data sources would be used through the AI models such as electronic health records (EHRs), genetic profiles, and social determinants of health to select candidates that may most probably respond to the treatment being investigated.43 In a current oncology trial, the application of Mendel AI has resulted in a 24% to 50% increase in the accurate identification of patients who may be eligible, in contrast to conventional methods. Additionally, the process of assessing potential eligibility with Mendel AI is completed in mere minutes, whereas the standard prescreening duration averages 19 days for breast cancer patients and 263 days for those with lung cancer.44 The implementation of automated electronic health record (EHR) text-mining for participant identification in cardiovascular trials could lead to a significant 79.9% decrease in the number of patients requiring screening.40,45 A study involving pediatric oncology patients demonstrated that the use of an AI system achieved a 90% reduction in the workload associated with trial recruitment.46 Furthermore, the ACTES system was found to decrease patient screening time by 34% by minimizing the time allocated to administrative duties, discussions, and unstructured collaboration.47 Additionally, research conducted by Whitty indicated that AI systems, when utilized in two completed oncology studies focused on breast and lung cancer at the Comprehensive Blood and Cancer Center, resulted in a 24% to 50% increase in the identification of eligible patients compared to traditional practices.39,44,48
5.3.1 Improved recruitment.
AI algorithms can automate the recruitment process by quickly filtering thousands of EHRs and genetic data in real time. As reported, for instance, companies such as Deep 6 AI have used NLP to gather significant patient data from clinical records, thereby speeding up recruitment processes by identifying eligible patients much faster than with traditional manual methods.49
5.3.2 Predictive models for outcome.
It can predict the patient's reaction to treatment based on a history of clinical data as well as genetic markers, meaning that it will be more accurate in selecting candidates who are more likely to result in positive outcomes. The researcher is able to predict which patients will best respond and the probability of side effects, thus minimizing risks and maximizing the effectiveness of the trials.50
5.4 AI-driven monitoring tools
Monitoring of data on patients once the trial starts is necessary to ensure safety and efficacy. This real-time tracking of patient progress can now be done easier with the advent of AI tools, particularly through the use of NLP and image recognition technologies, which can discover adverse effects more rapidly than traditional means.
5.4.1 Real-time monitoring.
AI-based systems can monitor in real time the data of wearables, EHRs, and medical images for anomalies or early signs of adverse effects. This way, teams running clinical trials can quickly respond to safety issues and change the course of treatment if necessary to better patient safety. AI-based monitoring reduces dropout rates among clinical trial participants by providing closer, individualized care all along the trial cycle process.20,43
5.4.2 NLP and image recognition.
Of course, NLP would tease out very valuable insights in the form of unstructured clinical trial data, for example, patient feedback, physician notes, and even the clinical trial report. AI-driven image recognition can analyse medical scans, identify the slightest change in the progression of disease or response to treatment, and help coordinators modify therapies in real time.
5.5 Case studies
5.5.1 BenevolentAI and drug repurposing.
One of the best examples of AI usage in drug development is so-called area of drug repurposing, particularly with the advent of COVID-19. BenevolentAI, based in the UK, used algorithms to scan through extensive biomedical datasets, identifying existing drugs that could be repurposed to treat COVID-19. Its AI models flagged that baricitinib, a rheumatoid arthritis drug, would find utility in the treatment of COVID-19, which was later revalidated in clinical experiments and received emergency use approval.51
5.5.2 Exscientia and personalized medicine.
Another AI-driven discovery company for drugs is Exscientia, which applies AI to predict patient responses to cancer treatments. By analysing the genetic and biomarker data of a patient, Exscientia designs clinical trials for each patient; this gives much more tailored, effective treatment outcomes.52
6. AI in manufacturing and supply chain management
Artificial intelligence is nowadays applied more significantly in the optimization of pharmaceutical production and in managing the pharmaceutical supply chain. Pharmaceutical companies can facilitate smoother, cost-effective, and efficient operations with the support of AI technology. AI enhances predictive maintenance, process automation, inventory management, and quality control, thus enabling consistent and high-quality pharmaceutical production. Such examples are those in the sector wherein AI has the potential to transform the industry's prospects for better-market-driven responses, compliance, and reduced risk.
6.1 Role of AI in pharmaceutical manufacturing industry
Manufacturing in the pharmaceutical industry is highly regulated for quality, safety, and efficiency. AI's role in manufacturing revolves around improving predictive maintenance, process optimization, and quality control. The aim here is predictive maintenance:
6.1.1 Predictive maintenance.
Traditionally, maintenance has been fairly reactive, which means that equipment was typically only repaired after it had broken down. As you can expect, this can sometimes lead to a halt in production. AI shifts the model to proactive for this reason. With the ML algorithms analysing real-time data from machinery sensors, companies can predict when equipment will likely fail, thereby replacing and repairing parts in time to avoid failure, hence minimizing downtime, improving overall equipment effectiveness, and ensuring smooth production flows.53,54
6.1.2 Optimization of processes.
AI will optimize the production processes of pharmaceuticals that are complex, including chemical synthesis and formulation. AI models such as reinforcement learning and neural networks can control in real time the factors of manufacturing to ensure consistent quality of products and maximize yields. The technology can also be used to simulate different manufacturing scenarios, an aid to a company in choosing the most efficient process in the reduction of production timelines.20,55,56
6.1.3 Quality control.
The drug product must be of quality as well as safety. AI improves the quality control by monitoring data coming from the production line and flags deviation in standard parameter values. Machine learning models thus get trained to identify anomalies or defects in chemical, physical or physical presentation/packaging of the drug, and hence, corrective action is undertaken in real time. AI further reduces the chances of human error, the biggest risk factor in the quality check which is manually carried out.20,57
6.1.4 Impact of AI to emerging technologies such as 3D printing, PAT and continuous manufacturing processes.
In 3D printing pharmaceuticals, AI is primarily used to optimize the design and manufacturing process by predicting optimal printing parameters, analyzing quality control data, and enabling personalized medicine by tailoring drug release profiles based on individual patient needs, essentially creating a more efficient and precise way to produce customized medications through 3D printing technology.58–61 Artificial intelligence is capable of creating complex 3D-printed tablets with internal structures that regulate drug release over time.62 The 3D printers can produce tablets with multiple drug layers using AI, allowing for precise release profiles.63 AI can create a 3D-printed medication that is customized for each patient based on their medical data.64
The collaboration of PAT, process data science, and AI establishes an effective monitoring system for the continuous manufacturing line. It provides several benefits that will result in decreased production expenses. This will involve decreased final product testing due to continuous monitoring and testing, which allows for the application of PAT principles. This leads to the capacity to release products with less final product testing, facilitating lower inventory and quicker recovery of production costs. The benefit is most significant for pharmaceutical companies planning to manufacture a product for an extended period. Thoughtful planning paired with informed leadership, eager to collaborate with regulators, will be advantageous for shareholders and patients.
AI tools that are beneficial in Process Analytical Technology (PAT) and continuous manufacturing encompass expert systems, fuzzy logic approaches, neural networks, case-based reasoning, genetic algorithms, and ambient intelligence. These methodologies, combined with computational power, emulate human-like reasoning and decision-making in uncertain situations. They find extensive applications in process control, optimization, monitoring, parameter estimation, prediction, chemometrics, process scale-up and scale-down, as well as the development of soft sensors. Fuzzy control systems offer real-time decision support to operators and facilitate automatic control. Current applications include fault diagnosis and control, as well as pattern recognition in various processes. Moreover, Model Predictive Control (MPC) enables real-time management of quality attributes.65
Although ANNs have already undergone testing for a number of uses in the most typical manufacturing processes, there are currently few real-time applications for PAT. The first stage in the production of the API in industrial pharmaceutical manufacturing is the synthesis of organic compounds. ANN-based synthesis optimization has been the subject of numerous studies.66–70 Information from data-rich PAT tools such as in-line microscopic images has been extracted using ANNs. When used for contamination classification, a ResNet CNN demonstrated >98% accuracy in classifying crystals found in PVM images.71 Additionally, CNN-based in-line image analysis could be used to measure the particle size distribution and predict the crystal growth rate.72 For a continuous wet granulation process, the use of AI has greatly decreased noise, improved process monitoring, and enhanced process understanding.73 Therefore, AI may play a significant role in the future development of intelligent, self-sufficient pharmaceutical production lines. In addition to lowering the environmental load through faster, more economic production or waste reduction, automated systems may also reduce human exposure to hazardous processes or pharmaceutical ingredients such as hormones or cytostatics.74
For pharmaceutical applications, the US FDA categorizes AI software as medical devices that must adhere to Good Manufacturing Practice (GMP)75 and the requirements for validating software.76–78 This indicates that an AI system must handle the information required for PAT and continuous manufacturing processing systems,79 all while maintaining its validated status. As indicated in the previous discussion, the regulatory endorsement of this system necessitates close collaboration among experienced manufacturing regulators, software regulators, and company personnel. Consequently, this implies that gaining regulatory approval will take longer than normal and the volume of data submitted to regulators will be substantial.65
6.2 Process automation
The pharmaceutical production lines morph into smart factories with AI-driven automation, where machines and systems can adjust processes autonomously based on live data. These AI-armed factories use sensor data, robotics, and IoT devices to fully automate the production of drugs, thereby making them easier to improve in terms of efficiency, precision, and scalability.
6.2.1 AI-driven robotics.
Robotics contributes a lot to the automation of recurrent risky operations such as packaging, labelling, and handling of materials. Robots driven by AI are not prone to fatigue; hence they can work round-the-clock with consistent production levels and minimal human errors. Furthermore, robots can handle toxic substances and hazardous environments better than humans without risking contamination or accidents.80
6.2.2 Smart manufacturing.
AI makes real-time adjustments in parameters such as temperature, pressure, or mixing speed because sensor feedback dictates. While this kind of flexibility can be so important to consistency in the batches being made, it can also be critical for biologics, where small changes could mean drastically affecting the quality of finished products. In a case study conducted by Novartis in 2020, the use of AI-enabled automation at its manufacturing site reflected a 15% improvement in production efficiency and a 20% cut in running expenses.
6.3 Inventory and supply chain optimization
Pharmaceutical supply chains include numerous layers and levels, making them complex. It requires smooth management for the delivery of raw materials as well as final products on time. AI is critical in inventory management and demand forecasting and optimization of the overall supply chain:
6.3.1 Demand forecasting.
The AI tools, including predictive analytics and machine learning models, help the demand to be forecasted more accurately through an analysis based on past sales data, market trends, and external factors such as seasonal variations or global health crisis. Predicting demand may either lead to overproduction or shortage, leading to delay in drug availability.81
6.3.2 Inventory optimization.
The intelligent inventory management systems can provide pharmaceutical companies with the right quantities of raw materials and finished goods at the right moment. An intelligent inventory management system allows such companies to fully automate reorders for materials from real-time data, so they can avoid stockouts or overstocking. For example, Pfizer has used AI for the optimization of its inventory and supply chain for vaccine production, so that raw materials are always on hand just in case without building up too much in inventory.82
6.3.3 Enhancing supply chain resilience.
AI makes supply chains more resilient by scanning what might disrupt them by way of geopolitical risks, raw material shortages, or supplier failures. AI systems can model different scenarios and recommend alternative sourcing strategies or logistic routes; hence, drug manufacturing and distribution would continue.81,83
6.4 Reducing costs and improving efficiency
AI integration into pharmaceutical manufacturing would then imply the following huge opportunities for cost savings through enhancing production accuracy, minimizing waste, and optimizing use of resources.
6.4.1 Better quality in production.
Algorithms based on artificial intelligence, including machine learning models, have kept tight control over production parameters. AI can read sensor data online as part of manufacturing equipment in real time and identify inefficiencies or other variance issues that could otherwise influence drug quality. Continuous adjustments ensure consistency in product quality, lowering the risk of costly product recalls and batch failure. This also lowers the potential for human error, the biggest cost driver in pharmaceutical production.57
6.4.2 Waste minimization.
The most significant role of AI in the waste minimization process is played through its predictive capabilities. Predictive failures of the equipment and scheduling predictive maintenance by AI prevent increased time wastage in unplanned downtime and machine faults, which eventually result in the wastage of products. Apart from that, AI-driven quality control systems could detect early defects during the production process and prevent defective products from moving further down the line. As revealed by a study published in the International Journal of Production Economics, AI-based methods can actually reduce up to 25% waste compared to traditional methods if subpar batches are not allowed to proceed to production.
6.4.3 Resource optimization.
The AI-based systems assist the companies in optimizing the raw material and human capital usage. AI can calculate the exact quantities of the raw material to be consumed, so that waste is avoided. It also does not overproduce by using the demand forecasting models, which accurately predict the requirement of the market in the future. It becomes a significantly resource-efficient usage, which further minimizes the cost through the supply chain.81,83
6.5 Case study examples: Pfizer and Novartis
6.5.1 Pfizer.
They used AI in the designing and manufacturing stages of the COVID vaccine to optimize its manufacturing process and supply chain. Pfizer could predict possible disruption, guarantee the timeliness of raw materials at the right moment, and increase the volume of production without compromising on the quality of the product due to AI.82
6.5.2 Novartis.
The organization has installed AI at most of its global manufacturing plants to support the automation of processes and predictive maintenance. It managed to significantly reduce losses in terms of lost time through the use of AI in its production lines. Resource utilization increased with AI, indicating a major step forward with regard to very tight regulatory standards.
7. AI in personalized medicine and pharmacovigilance
AI is revolutionizing both personalized medicine and pharmacovigilance. This is how AI can tailor a patient's medical treatments while further enhancing post-market drug safety surveillance. The introduction of AI based on machine learning, natural language processing, and big data analytics to facilitate real-time, data-driven decision-making has further helped to increase the benefits from the improvements in patient outcomes, early adverse drug reaction detection, or efficient monitoring of drug safety.84,85
7.1 Personalized medicine
Adjusting health care to the specific needs of every individual in the context of personalized medicine involves tailoring decisions regarding medical care, treatment, practice, or product to each patient. Furthermore, AI is particularly crucial for realizing personalized medicine through the use of genomics and other biological data.
7.1.1 AI and genomics.
Using AI, you could process a large amount of data related to genetic information. Here you could determine very specific biomarkers to show potential response towards a particular drug, and so on. Machine learning models, especially deep learning algorithms, can predict what kind of response a person would have to certain drugs based on his or her genome, lifestyle, and medical history. It leads to a better selection of drugs that have a higher probability of working for a given patient, but with a reduced possibility of adverse reaction.
7.1.2 AI platforms for personalized medicine.
These are AI platforms such as IBM Watson Health and Tempus, which use complex data analytics to match patients with the most effective treatments. Such a platform integrates genomic data, electronic health records, and clinical research to help physicians make or tailor their decisions according to a patient's condition. For instance, Tempus merges AI with molecular data in an effort to enable oncologists to discover the most likely anticancer therapy to recommend to a patient based on his genetic makeup.86
7.1.3 Roche's impact on cancer treatment.
Roche is the first to introduce AI-driven personalized medicine solutions. The subsidiary of the company, Foundation Medicine, uses AI in analysing tumor samples to identify mutations treated with particular drugs. This has enabled more effective treatment in cancer through a tailored regimen. Interventions were significantly reduced accompanied by the side effects.87
7.2 Pharmacovigilance and safety monitoring
Pharmacovigilance is monitoring the effects of licensed medicinal drugs after they have been marketed, especially to identify, assess, understand, and prevent adverse effects or any other drug-related problems. AI actually is important to enhance pharmacovigilance as it is a method through which data collection and analysis can be automatically done.
7.2.1 Post-market data analysis.
AI systems can analyse vast datasets including adverse event reports, EHRs, social media discussions, and patient feedback, much better than human operators to catch safety signals much more efficiently. For instance, NLP models can scan through free-text medical records for unseen adverse drug reactions not picked up by conventional reporting mechanisms. Timely data analysis will thus enable pharmaceutical companies to have proactive measures against a potential threat to clinical safety.43
7.2.2 AI-powered pharmacovigilance platforms.
Companies like Aetion and BenevolentAI have developed AI-powered pharmacovigilance platforms. These are tools capable of analysing post-marketing drug data in relation to detecting rare or unexpected adverse events that otherwise would have gone undetected. They aid in the optimum and efficient use of pharmacovigilance with continued learning through continuous streams of new data through ML algorithms.84,85
7.3 Real-time monitoring of adverse drug reactions
One of the most promising applications of AI in pharmacovigilance is the real-time monitoring of adverse drug reactions. The systems of pharmacovigilance are basically manual reporting by healthcare professionals or patients, which results in delays far too great to identify dangerous side effects. AI, instead, can analyse data streams through various sources in real time and raise an alert on potential ADRs as soon as they appear.
7.3.1 NLP for ADR detection.
NLP algorithms can be used in very useful ways in this field. From clinical notes to online forums, social media, and even patient surveys, unstructured text data can be mined towards the detection of patterns in language which are suggestive of adverse drug reactions. Patient feedback can make a healthcare provider and agency alert to several emergent safety issues before these become widespread through real-time monitoring.
7.3.2 Machine learning for predictive safety monitoring.
Machine learning models can also be used to predict the likelihood of an ADR event resulting from patient demographics, co-morbidities, and medication history. Importantly, machine learning can take data from multiple sources, especially genetic data to assess the ADR risk for individual patients with more personal safety monitoring. For instance, predictive models help the pharmaceuticals manage safety better through IBM Watson Health's analysis of adverse events and prediction of patient outcomes (Table 3).86
Table 3 AI in pharmacovigilance and personalized medicine
AI platform |
Application |
Data sources used |
Outcome |
IBM Watson Health |
Personalized treatment recommendation |
Genomic data, EHRs |
Tailored treatment plans based on patient data |
NLP models |
Real time adverse event detection |
Patient feedback, medical records |
Early detection of adverse drug reactions |
Aetion |
Pharmacovigilance |
Post-market surveillance data |
Continuous monitoring and faster ADR identification |
7.4 Case study examples
7.4.1 Roche's AI tools.
Roche has been at the steering wheel of utilizing AI for its pharmacovigilance efforts. AI-based solutions by the company can scour through gigantic real-world datasets to track and detect adverse drug reactions in real time. The applications of AI by Roche went beyond Foundation Medicine, to pharmacovigilance, tracking genetic mutations in patients suspected to be linked with some drugs that could lead to adverse responses. It is a way of fine-tuning safer treatment options for patients.87
7.4.2 FDA sentinel initiative.
The United States Food and Drug Administration has been using AI models to examine EHRs and insurance claims as part of its Sentinel Initiative for the early detection of adverse events. By using machine learning, the Sentinel Initiative thus facilitated the timely determination of drug safety issues during the post-market surveillance process.
8. AI in pharmaceutical management
Artificial Intelligence, also known as AI, has changed drug development and, ultimately, clinical trials as well as the wider scope of operational and management functions in pharmaceutical companies. By deploying AI into management functions, pharmaceutical companies can drive greater operational efficiency, increase data-driven decision-making, and ensure compliance with regulatory requirements for bottom-line improvement in performance and reduction of risks.
8.1 Operational efficiency
Efficiency in operations is a must factor across the different departments of the pharmaceutical industry, such as R&D, financial operation, supply chain, and HR. In current times, AI is being used to automate and streamline such operations:
8.1.1 Research and development efficiency.
AI is used to optimize the management of R&D processes by automating routine, administrative activities such as data entry and tracking experiments. Automation minimizes the scope of errors, puts staff in better positions to handle crucial tasks, and increases the accuracy of R&D timelines.88
8.1.2 Supply chain optimization.
AI-based solutions enable an organization to track and predict how business disruptions are manifesting in supply chains and avoid bottlenecks for pharmaceutical firms. AI can also automate the management of the inventory, so that essential materials would not run out and are not overstocked in the shelves.81,83
8.1.3 Human resources and financial operations.
AI helps with HR activities within the organization such as payroll processing, employee scheduling and recruitment. At financial operation level AI algorithms analyse budgets and financial statements, forecast future costs and support decision making on resource optimization. This means pharma companies can better manage their finances and make the most of their resources.89
8.2 Data-driven decision-making
Data-driven decision-making is a key area in pharmaceutical management that AI has come to highly impact on. Coupled with the capacity of AI, which can process huge amounts of data, pharmaceutical managers will be able to utilize predictive analytics in terms of insights for the boosting of decision-making. These AI tools analyse types of internal and external data, whether they are market trends, sales figures, or research data. From these insights, the AI tools help in strategizing and planning as well as in the allocation of resources.
8.2.1 Predictive analytics.
AI algorithms take the historical data and forecast the outcome of the future. For example, based on historical data, a machine learning algorithm can predict the success rate of new drugs, thus perfecting investment decisions.
8.2.2 Sales and marketing optimization.
AI tools such as Salesforce or Veeva Systems help the company to monitor the sales performance to predict future sales, as well as identify the potential market. Such tools also avail designs for targeted and efficient marketing strategies through the analysis of the data of the customers.
8.3 Regulatory compliance and risk management
One of the issues tackled by the regulatory is ensuring that the practices implemented by those pharmaceutical companies comply with specific guidelines laid out by organizations such as FDA (U.S. Food and Drug Administration) and EMA (European Medicines Agency). Hence, compliance with these regulations also rests significantly on AI as it is helping the pharmaceutical companies by which you are staying up to the mark of norms and standards.
8.3.1 Compliance monitoring.
AI continuously monitors operations to ensure that the ones being monitored are conforming to regulatory requirements. For instance, a software package powered by AI can evaluate gigantic heaps of data, such as clinical trial records or manufacturing logs, to determine issues with compliance and flag up these for corrective action. This prevents the company from facing severe penalties related to the non-compliance of its operations with regulatory requirements.90
8.3.2 Risk prediction.
AI-based systems can predict and prevent various risks, such as legal issues, financial instability or even product recalls. All these happen according to data obtained from various sources such as previous audits, market tendencies, and activities of competitors, where AI tools bring in suggestions on proactive measures to take in relation to such a risk before the problem escalates.
8.3.3 Fraud detection.
This is also a capability of the AI to analyse large datasets in real time, making it very effective in detecting fraud or non-compliant behaviour. The simple example of this is when pharmaceutical firms apply AI for identification purposes in financial records to ensure that they abide by internal and external regulations.
8.4 AI tools in pharmaceutical management
Pharmaceutical companies have various AI tools and platforms, especially designed for their operations management. What also makes these platforms powerful is they automate mundane tasks and delivering analytics for decision-making and compliance management.
8.4.1 SAP.
SAP is ERP software used worldwide, integrating modules with AI for supply chain management, HR, and financial operations. Its AI capabilities allow it to monitor in real-time resource flow and performance metrics, thus enhancing the efficiency of operation for pharmaceutical companies.
8.4.2 Salesforce.
It is a customer relationship management (CRM) sale tracking application, using AI capabilities. Pharmaceuticals use Salesforce to track sales performance, optimize the marketing plan, and ensure regulatory compliance. Pharmaceutical managers are able to analyse customer as well as sales data in order to forecast what is expected in the near future.
8.4.3 Veeva systems.
Veeva is the portfolio of cloud-based solutions for the pharmaceutical and life sciences industries. Veeva's modules, powered by AI, help companies streamline clinical data management, regulatory submissions, and quality control.
9. Challenges and ethical considerations
The pharmaceutical world is witnessing significant changes with the development of Artificial Intelligence. However, it also brings several challenges and ethical issues. Pharmaceutical companies are facing numerous data privacy-related concerns, regulatory compliance, algorithmic biases, and many such factors in their efforts to utilize AI suitably and responsibly.
9.1 Data privacy and security
Pharmaceutical companies deal with bulk sensitive information regarding patients during drug discovery, clinical trials, and personalized medicine applications. The use of AI, especially machine learning models that take considerable amounts of data for training, raises concerns over data privacy, breaches, and misuse. Robust data security is thus a critical issue, and the companies must follow various strategies for the protection of patient information.
9.1.1 Data encryption.
Data encryption allows protection of patient data in that, if intercepted, they are still unreadable without proper decryption keys. The pharmaceutical companies are making greater use of the end-to-end encryption in protecting patient records and other sensitive information transmitted and stored.91
9.1.2 Anonymization techniques.
Another significant approach is data anonymization for the patient information. The process of removing personally identifiable information (PII) from datasets ensures a company that its data used for training AI models or developing drugs cannot be traced back to any patient. Data masking and synthetic data generation techniques enable researchers to use a realistic dataset without compromising the patients’ rights to privacy.91
9.1.3 Regulatory frameworks (GDPR, HIPAA).
In the current context, regulatory authorities refer to the General Data Protection Regulation (GDPR) in Europe and the Health Insurance Portability and Accountability Act (HIPPA) in the U.S. They broadly specify certain constraints associated with data privacy and security; GDPR emphasizes issues associated with consent and access rights as well as their erasure, while HIPAA highlights certain regulations regarding sharing patient's data and storing it within health care settings. These regulations are critical for ensuring that AI systems comply with legal standards and maintain patient trust.91
9.2 Regulatory hurdles
The pharma industry is still in the infancy stage of AI adoption, while regulators try to catch up with the rapid technological advancement. Each of the three principal areas, namely drug discovery based on AI, clinical trials, and patient care, is taking the regulatory evaluation into new territories, unlike the traditional approaches that involved drug development with well-defined regulatory guidelines.
9.2.1 Lack of standardization.
There is currently no standard framework established for the evaluation of AI tools in drug development. The regulator body including the U.S. Food and Drug Administration is currently putting together the guideline that will include the dynamic nature of AI algorithms during deployment, as it learns with new data incorporated later. The uncertainty this brought has so far led to the deferment of the general use of AI in the pharmaceutical sector.
9.2.2 Lack of transparency in decision making.
AI models are always not transparent, which makes it difficult for regulatory bodies to comprehensively assess a decision-making process. For patient safety and in the development of drugs, AI-driven decisions should be safe, effective, and explainable. Hence, for the assurance of safety and effectiveness, regulatory bodies are seeking transparent mechanisms of AI models and systems that account for errors.
9.3 Ethical implications
Using AI within the pharmaceutical industry opens a particularly wide and weighty door concerning ethical issues, especially that of bias in algorithms and machine-driven dependency.
9.3.1 Bias of AI algorithms.
The quality of AI algorithms is the quality of the training data. Datasets are biased; the resulting AI model may also be biased, and so similarly, bias in drug development and patient care may result. For instance, evidence has shown that some AI diagnostic tools are less precise for certain racial or gender groups raising valid questions about whether AI applications in healthcare are fair and equitable.92 Determining an exact percentage of data bias in artificial intelligence utilized within the pharmaceutical sector and clinical trials is a challenge, given the intricate characteristics of data and the diverse uses of AI.93,94 However, numerous experts suggest that a significant proportion of AI models employed in healthcare including pharmaceuticals may be influenced by bias stemming from the data on which they are trained. This bias could result in error rates that vary from 10% to over 30%, contingent upon the particular application and the quality of the data involved.
Research indicates that a significant number of AI models used in healthcare, particularly in clinical trials, are prone to substantial bias due to the use of non-representative datasets. This bias often originates from the initial selection of patient populations for clinical trials, which frequently lack demographic diversity, potentially resulting in distorted outcomes when these models are trained.95 Even with efforts to construct diverse datasets, unconscious biases may still affect both data collection and interpretation. Various factors contribute to data bias, including limited patient demographics and gender bias, as historical trends in clinical trials have often favored male participants, which can skew drug efficacy evaluations for female patients, as well as racial and ethnic disparities.96 In the realm of pharmaceutical manufacturing, even a minor degree of bias in AI systems can lead to significant repercussions, affecting drug efficacy, safety, and patient outcomes. More intricate AI models may be particularly vulnerable to bias if they are not meticulously designed and validated. To address such data bias, it is essential to implement diverse data collection strategies, conduct thorough data quality assessments, make algorithmic adjustments such as employing weighting algorithms to rectify data imbalances, and ensure transparency and comprehensive reporting.
Data bias of this nature can result in significant ethical consequences such as misdiagnosis, biased treatment options, ineffective medications for specific demographic groups, and potential harm to the company's reputation if not managed appropriately. Ethical obligations encompass the proactive reduction of bias through the collection of diverse datasets, thorough evaluation of models, and clear communication of limitations.97 Consequently, pharmaceutical companies using AI must engage in practices that promote diverse data collection, implement quality assurance measures, employ techniques for bias detection, ensure transparent reporting, and conduct regular monitoring while seeking expert evaluations from ethics committees to examine the possible ethical ramifications of AI applications.98,99 Failure to address data bias may lead to legal challenges, cause damage to reputation, and result in increased health disparities.
9.3.2 Human oversight.
Although it may prove to be advantageous in enhancing the quality of decisions concerning the pharmaceutical business, human oversight and decision-making remain crucial. AI should supplement, not supplant human judgment. Moreover, ensuring healthcare professionals are included in the decision-making process can help mitigate risks associated with algorithmic errors or biases.91
9.3.3 Transparency and ability to explain.
The algorithms that are produced by AI, particularly by deep learning models, are often referred to as “black boxes” because they lack transparency. For ethical deployment of AI in drug discovery and for providing care to patients, AI models must be explainable, that is, healthcare professionals and regulators need to understand how AI reaches its conclusions. If such transparency is absent, there may likely be over-reliance on AI without proper oversight, which can be dangerous.100
9.4 The way for regulatory agencies to adopt AI
The FDA and EMEA have the opportunity to integrate artificial intelligence into manufacturing and clinical trials by developing comprehensive guidelines that encompass the creation, validation, and continuous oversight of AI-driven systems. These guidelines should prioritize transparency, data integrity, and patient safety, while also offering a structured approach for manufacturers to submit applications that incorporate AI technologies for evaluation and approval.101 Regulatory bodies can set forth criteria to assess the accuracy, sensitivity, and specificity of AI models used in manufacturing and clinical trials, taking into account potential biases present in the data sets.102 Furthermore, agencies should mandate stringent data collection protocols, appropriate data annotation, and thorough documentation of data origins to ensure the dependability of AI models trained on such information. The guidelines must emphasize the establishment of rigorous validation techniques to verify the performance and reliability of AI systems in practical applications including ongoing performance assessment after market introduction.103 Additionally, there should be a concerted effort to identify and address potential risks linked to AI deployment, such as algorithmic bias, concerns regarding data privacy, and vulnerabilities related to cybersecurity.104
Specific guidelines may be established for the utilization of artificial intelligence in the analysis of real-time sensor data to detect and forecast potential challenges within the manufacturing process, thereby facilitating proactive modifications and enhancing quality control.105 The implementation of AI-driven systems can enable automatic adjustments to manufacturing parameters based on real-time data, ensuring consistent product quality. Additionally, AI guidelines can be issued to anticipate equipment failures in manufacturing environments, allowing for preventative maintenance and reducing production downtime.106 In the context of clinical trials, guidelines can be developed for patient recruitment and stratification, real-time monitoring of clinical data, and the application of AI in the analysis of medical images such as X-rays and MRI scans to aid in diagnosis and track disease progression. Close collaboration with industry stakeholder, including pharmaceutical companies, medical device manufacturers, and AI developers will be essential to ensure that these guidelines are both practical and feasible. Furthermore, it is important to design guidelines that can adapt to advancements in AI technology.107
9.5 Case study
9.5.1 Racial bias in medical algorithms.
In 2019, in the United States of America, a commercially used healthcare algorithm exhibited racial bias. Healthcare system made mysterious decisions regarding withholding further care from Black patients. Designated to predict the patients most likely to benefit from further care, the model was less likely to prescribe additional care for those patients if they happen to be Black compared to their white counterparts even when both had similar ailments. This was because the model was trained from biased data that represented prevalent healthcare disparities.92
9.5.2 Gender bias in AI diagnostic tools.
Some fields of medicine were shown to have better AI diagnostic tools for men than for women. Heart disease presents somewhat differently in men than in women, so it is diagnosed according to different manifestations, but most of the models used were trained based on data collected from mostly males. This has therefore resulted in misdiagnosis or delayed diagnosis for the heart diseases in women especially in an accident and emergency department or in assessing risk factors. The difference in the diagnostic models might have caused a worse health for women. Due to the above discussion, there was more concern on the issue of gender inequality in AI tool development and the need for diverse datasets with equal data from both male and female.
9.5.3 AI misdiagnoses in radiology.
AI intended for medical imaging, for example, to detect lung cancer or pneumonia from X-rays or CT scans, had a propensity for making false diagnosis due to the artificial intelligence models that had not been trained with rich datasets having variables such as wide ranges of age or ethnic backgrounds or other comorbid conditions. For instance, in one such instance, there was an AI tool utilized in certain hospitals, which proved to be much more prone to missing pneumonia signs in older patients or those having other underlying respiratory conditions, for which it had not been properly accounted during the training data. This only adds fuel to the fire of debate regarding the authenticity and reliability of AI tools, particularly in life-or-death conditions when timely diagnosis is of paramount importance. This calls for more intense testing and validation as well as continued monitoring of these AI systems in clinical environments.
10. Training needs of pharmaceutical and clinical trial professionals to adopt AI
The integration of artificial intelligence in pharmaceutical manufacturing and clinical trials necessitates comprehensive training for industrial personnel across several domains. This includes foundational knowledge in data science, familiarity with specific AI algorithms pertinent to their roles, understanding of regulatory compliance associated with AI, data management techniques, critical thinking abilities for interpreting AI-generated results, and awareness of ethical implications related to AI applications in healthcare.108,109
In the context of pharmaceutical manufacturing, personnel will require training focused on process monitoring and control through AI. This involves leveraging AI models to forecast and optimize manufacturing parameters such as temperature, pressure, and ingredient ratios in real time. Additionally, quality control will benefit from AI applications, including AI-driven image analysis for defect detection and quality assurance in both drug substances and products. Training will also be essential for predictive maintenance, enabling personnel to use AI for anticipating equipment failures and scheduling preventive maintenance. Furthermore, data integration and analysis training will be necessary to connect manufacturing data from various sources for the training and deployment of AI models.110
Training will be essential for clinical trial personnel to effectively recruit and stratify patients using artificial intelligence, which will assist in identifying appropriate candidates for clinical trials based on their demographics and medical histories.40 Subsequently, personnel will need to undergo training focused on optimizing clinical trial design, utilizing AI to create more efficient trials that require fewer participants and can be completed within shorter timeframes.111 Additional training will encompass real-time patient monitoring, enabling the analysis of patient data from clinical therapies and other sources through AI to identify potential adverse events at an early stage. Furthermore, training in data analysis and interpretation will be necessary, where AI algorithms will be employed to analyze intricate clinical trial data and derive significant insights.112 Similarly, IT personnel and data scientists will require training in advanced AI methodologies, gaining practical experience in constructing, validating, and deploying AI models within a production setting, as well as understanding cloud computing for data storage and processing to facilitate large-scale AI applications, alongside data security and privacy considerations.39 These training programs can be delivered through a combination of online courses, tutorials, in-person workshops, seminars, mentorship initiatives, simulation exercises, customized training sessions, and ongoing learning opportunities.
11. Conclusion and future prospects
This future of AI in the pharmaceutical industry is full of potential changes that will reshape many aspects of developing a drug, conducting clinical trials of that drug, its manufacturing, and the care that comes after. Growth in AI technologies will bring further discoveries in quantum computing, reinforcement learning, and personalized medicine.
The most notable development area will be the incorporation of quantum computers into drug discovery. Quantum computers will enable an AI algorithm to execute complex molecular simulations at an unprecedented speed and accuracy that a classical computer could not, solving certain problems in oncology and neurodegenerative diseases. AI applications in personalized medicine will expand further with the use of multi-omics data to predict specific responses by patients to personalized therapies.
From manufacturing to supply chain, smart factories would incorporate AI-driven adjustments of the given processes using real-time data for efficient resource utilization with reduced waste. Similarly, by predictive demand and prevention of shortage scenarios, resilience in supply chains will also increase.
However, with these come problems. For example, data privacy, bias of algorithms, and transparency of AI models raise ethical issues. There would be a need for the FDA and EMA among others to align their frameworks in new settings with that objective to assess the safety and efficacy of AI-driven tools in pharmaceuticals.
In summary, AI already showed transformative power across the pharmaceutical landscape through solutions related to drug development and patient safety that are faster, more efficient, and personalized. As AI technologies continue to evolve, it is necessary that the industry collaborates with regulatory bodies and answers ethical questions to actually tap into the deeper resources of AI for improved benefit to healthcare outcomes. With the capacity to streamline operations, accelerate innovation, and improve the care of patients, AI will be the core cornerstone of the future pharmaceutical industry.
Abbreviations
AI | Artificial intelligence |
CNN | Convolutional neural network |
GANs | Generative adversarial networks |
ML | Machine learning |
NLP | Natural language processing |
DL | Deep learning |
SAR | Structural activity relationship |
HTS | High-throughput screening |
SVM | Support vector machine |
RNNs | Recurrent neural networks |
NDA | New drug application |
USFDA | United States Food and Drug Administration |
CDSCO | Central Drug Standards and Control Organization |
MHLW | Ministry of Health and Labour Welfare |
PMDA | Pharmaceuticals and Medical Devices Agency |
EHR | Electronic health record |
ADR | Adverse drug reaction |
R&D | Research and development |
HR | Human resources |
EMA | European Medicines Agency |
GDPR | General data protection regulation |
HIPPA | Health insurance portability and accountability act |
IoT | Internet of things |
RL | Reinforcement learning |
NIBR | Novartis Institutes for BioMedical Research |
CT | Clinical trials |
CTMS | Clinical trial management system |
CRO | Contract research organization |
PMS | Post-marketing surveillance |
Data availability
No primary research results or new data were generated or analysed as part of this review.
Conflicts of interest
There are no conflicts of interest to declare.
References
- K.-K. Mak and M. R. Pichika, Drug Discovery Today, 2019, 24, 773–780 Search PubMed.
- P. Schneider, W. P. Walters, A. T. Plowright, N. Sieroka, J. Listgarten, R. A. Goodnow, J. Fisher, J. M. Jansen, J. S. Duca, T. S. Rush, M. Zentgraf, J. E. Hill, E. Krutoholow, M. Kohler, J. Blaney, K. Funatsu, C. Luebkemann and G. Schneider, Nat. Rev. Drug Discovery, 2020, 19, 353–364 Search PubMed.
- E. J. Topol, Nat. Med., 2019, 25, 44–56 Search PubMed.
- J. He, S. L. Baxter, J. Xu, J. Xu, X. Zhou and K. Zhang, Nat. Med., 2019, 25, 30–36 Search PubMed.
- K. Kourou, T. P. Exarchos, K. P. Exarchos, M. V. Karamouzis and D. I. Fotiadis, Comput. Struct. Biotechnol. J., 2015, 13, 8–17 Search PubMed.
-
M. Puri, Y. Pathak, V. K. Sutariya, S. Tipparaju and W. Moreno, Artificial neural network for drug design, delivery and disposition, Academic Press, 2015 Search PubMed.
- J. Ubels, T. Schaefers, C. Punt, H.-J. Guchelaar and J. de Ridder, Bioinformatics, 2020, 36, i601–i609 Search PubMed.
- B. Liu, H. He, H. Luo, T. Zhang and J. Jiang, Stroke Vasc. Neurol., 2019, 4, 206–213 Search PubMed.
- D. Cirillo and A. Valencia, Curr. Opin. Biotechnol., 2019, 58, 161–167 Search PubMed.
- B.-J. Hou and Z.-H. Zhou, IEEE Trans. Neural Netw. Learn. Syst., 2020, 31, 2267–2279 Search PubMed.
- C. Sun, Y. Cao, J.-M. Wei and J. Liu, Bioinformatics, 2021, 37, 3618–3625 Search PubMed.
- N. K. Ebube, G. Owusu-Ababio and C. M. Adeyeye, Int. J. Pharm., 2000, 196, 27–35 Search PubMed.
- M. De Matas, Q. Shao, C. H. Richardson and H. Chrystyn, Eur. J. Pharm. Sci., 2008, 33, 80–90 Search PubMed.
- L. Kwek, S. Fu, T. Chia, C. Diong, C. Tang and S. Krishnan, Appl. Opt., 2005, 44, 4004–4008 Search PubMed.
- E. H. Bollschweiler, S. P. Mönig, K. Hensler, S. E. Baldus, K. Maruyama and A. H. Hölscher, Ann. Surg. Oncol., 2004, 11, 506–511 Search PubMed.
- A. Săftoiu, P. Vilmann, F. Gorunescu, J. Janssen, M. Hocke, M. Larsen, J. Iglesias-Garcia, P. Arcidiacono, U. Will and M. Giovannini, Clin. Gastroenterol. Hepatol., 2012, 10, 84–90 Search PubMed.
- M. D. Wessel, P. C. Jurs, J. W. Tolan and S. M. Muskal, J. Chem. Inf. Comput. Sci., 1998, 38, 726–735 Search PubMed.
-
T. Cova, C. Vitorino, M. Ferreira, S. Nunes, P. Rondon-Villarreal and A. Pais, in Artificial Intelligence in Drug Design, ed. A. Heifetz, Springer US, New York, NY, 2022, pp. 321–347, DOI:10.1007/978-1-0716-1787-8_14.
- M. Takenaka, T. Kakue, T. Shimobaba and T. Ito, IEEE Access, 2021, 9, 36766–36774 Search PubMed.
- D. Paul, G. Sanap, S. Shenoy, D. Kalyane, K. Kalia and R. K. Tekade, Drug Discovery Today, 2021, 26, 80–93 Search PubMed.
- J. M. Stokes, K. Yang, K. Swanson, W. Jin, A. Cubillos-Ruiz, N. M. Donghia, C. R. MacNair, S. French, L. A. Carfrae, Z. Bloom-Ackermann, V. M. Tran, A. Chiappino-Pepe, A. H. Badran, I. W. Andrews, E. J. Chory, G. M. Church, E. D. Brown, T. S. Jaakkola, R. Barzilay and J. J. Collins, Cell, 2020, 180, 688–702 Search PubMed.
- J. Fang, L. Wang, Y. Li, W. Lian, X. Pang, H. Wang, D. Yuan, Q. Wang, A.-L. Liu and G.-H. Du, PLoS One, 2017, 12, e0178347 Search PubMed.
- A. Bender, H. Y. Mussa and R. C. Glen, SLAS Discovery, 2005, 10, 658–666 Search PubMed.
- A. Abdo, B. Chen, C. Mueller, N. Salim and P. Willett, J. Chem. Inf. Model., 2010, 50, 1012–1020 Search PubMed.
- Y. Li, L. Wang, Z. Liu, C. Li, J. Xu, Q. Gu and J. Xu, Mol. BioSyst., 2015, 11, 1241–1250 Search PubMed.
- M. Lu, J. Yin, Q. Zhu, G. Lin, M. Mou, F. Liu, Z. Pan, N. You, X. Lian and F. Li, Engineering, 2023, 27, 37–69 Search PubMed.
- M. Fernández, A. Tundidor-Camba and J. Caballero, J. Chem. Inf. Model., 2005, 45, 1884–1895 Search PubMed.
- M. Fernández, J. Caballero and A. Tundidor-Camba, Bioorg. Med. Chem., 2006, 14, 4137–4150 Search PubMed.
- H. González-Díaz, I. Bonet, C. Terán, E. De Clercq, R. Bello, M. M. García, L. Santana and E. Uriarte, Eur. J. Med. Chem., 2007, 42, 580–585 Search PubMed.
- M. Fernández, M. C. Carreiras, J. L. Marco and J. Caballero, J. Enzyme Inhib. Med. Chem., 2006, 21, 647–661 Search PubMed.
- M. Fernández and J. Caballero, Bioorg. Med. Chem., 2007, 15, 6298–6310 Search PubMed.
- Y.-P. Pang, P. Quiram, T. Jelacic, F. Hong and S. Brimijoin, J. Biol. Chem., 1996, 271, 23646–23649 Search PubMed.
- M. Fernández and J. Caballero, Bioorg. Med. Chem., 2006, 14, 280–294 Search PubMed.
- M. Fernández and J. Caballero, Chem. Biol. Drug Des., 2006, 68, 201–212 Search PubMed.
- D. A. Filimonov, A. A. Lagunin, T. A. Gloriozova, A. V. Rudik, D. S. Druzhilovskii, P. V. Pogodin and V. V. Poroikov, Chem. Heterocycl. Compd., 2014, 50, 444–457 Search PubMed.
- J. Jumper, R. Evans, A. Pritzel, T. Green, M. Figurnov, O. Ronneberger, K. Tunyasuvunakool, R. Bates, A. Žídek, A. Potapenko, A. Bridgland, C. Meyer, S. A. A. Kohl, A. J. Ballard, A. Cowie, B. Romera-Paredes, S. Nikolov, R. Jain, J. Adler, T. Back, S. Petersen, D. Reiman, E. Clancy, M. Zielinski, M. Steinegger, M. Pacholska, T. Berghammer, S. Bodenstein, D. Silver, O. Vinyals, A. W. Senior, K. Kavukcuoglu, P. Kohli and D. Hassabis, Nature, 2021, 596, 583–589 Search PubMed.
- T. Burki, The Lancet Digital Health, 2020, 2, e226–e227 Search PubMed.
-
F. US
, The Drug Development Process, https://www.fda.gov/patients/learn-about-drug-and-device-approvals/drug-development-process, (accessed 26.01.2025).
- S. Harrer, P. Shah, B. Antony and J. Hu, Trends Pharmacol. Sci., 2019, 40, 577–591 Search PubMed.
- X. Lu, C. Yang, L. Liang, G. Hu, Z. Zhong and Z. Jiang, J. Am. Med. Inform. Assoc., 2024, 31, 2749–2759 Search PubMed.
-
X. Liu, C. Shi, U. Deore, Y. Wang, M. Tran, I. Khalil and M. Devarakonda, A Scalable AI approach for clinical trial cohort optimization, Paper presented at: Joint European Conference on Machine Learning and Knowledge Discovery in Databases, 2021.
- B. D. Dubois, J. Bioinf. Artif. Intell., 2024, 4, 72–83 Search PubMed.
-
R. Harpaz, K. Haerian, H. S. Chase and C. Friedman, presented in part at the Proceedings of the 1st ACM International Health Informatics Symposium, 2010, 2010.
- D. Calaprice-Whitty, K. Galil, W. Salloum, A. Zariv and B. Jimenez, Ther. Innov. Regul. Sci., 2020, 54, 69–74 Search PubMed.
- W. B. van Dijk, A. T. Fiolet, E. Schuit, A. Sammani, T. K. J. Groenhof, R. van der Graaf, M. C. de Vries, M. Alings, J. Schaap and F. W. Asselbergs, J. Clin. Epidemiol., 2021, 132, 97–105 Search PubMed.
- Y. Ni, J. Wright, J. Perentesis, T. Lingren, L. Deleger, M. Kaiser, I. Kohane and I. Solti, BMC Med. Inf. Decis. Making, 2015, 15, 1–10 Search PubMed.
- Y. Ni, M. Bermudez, S. Kennebeck, S. Liddy-Hicks and J. Dexheimer, JMIR Med. Inform., 2019, 7, e14185 Search PubMed.
- A. Ismail, T. Al-Zoubi, I. El Naqa and H. Saeed, BJR Open, 2023, 5, 1–5 Search PubMed.
-
D. AI
, AI powered patient recruitment & RWD, https://deep6.ai/, (accessed 26.01.2025).
- A. Blanco-González, A. Cabezón, A. Seco-González, D. Conde-Torres, P. Antelo-Riveiro, Á. Piñeiro and R. Garcia-Fandino, Pharmaceuticals, 2023, 16, 891 Search PubMed.
-
Benevolent
, COVID-19 research, https://www.benevolent.com/about-us/sustainability/covid-19/, (accessed 26.01.2025).
-
Exscientia
, Precision Medicine, https://www.exscientia.com/precision-medicine/, (accessed 26.01.2025).
-
G. Calzavara, E. Oliosi and G. Ferrari, A Time-aware Data Clustering Approach to Predictive Maintenance of a Pharmaceutical Industrial Plant, Paper presented at: 2021 International Conference on Artificial Intelligence in Information and Communication (ICAIIC); 13–16 April, 2021, 2021.
- I. Kavasidis, E. Lallas, V. C. Gerogiannis, T. Charitou and A. Karageorgos, Procedia Comput. Sci., 2023, 220, 576–583 Search PubMed.
-
M. Puri, S. Manwatkar, P. Karpe and S. Kulkarni, in Biosystems, Biomedical & Drug Delivery Systems: Characterization, Restoration and Optimization, ed. S. Kulkarni, A. K. Haghi and S. Manwatkar, Springer Nature Singapore, Singapore, 2024, pp. 179–196, DOI:10.1007/978-981-97-2596-0_9.
- D. Yadav, K. Sonkar, J. Kumar and A. Chaudhary, Eur. J. Parenter. Pharm. Sci., 2023, 28(4), 1–20 Search PubMed.
- M. Vaghela, S. Rathi, R. Shirole, J. Verma, Shaheen, S. Panigrahi and S. Singh, Chin. J. Appl. Physiol., 2024, 40, e20240005 Search PubMed.
-
F. M. Talaat and E. Hassan, Artificial intelligence in 3D printing, in Enabling Machine Learning Applications in Data Science, Algorithms for Intelligent Systems, Singapore: Springer Nature, 2021, pp. 77–88 Search PubMed.
- Z. Zhu, D. W. H. Ng, H. S. Park and M. C. McAlpine, Nat. Rev. Mater., 2021, 6, 27–47 Search PubMed.
- B. R. Hunde and A. D. Woldeyohannes, Results Eng., 2022, 14, 100478 Search PubMed.
- I. Rojek, D. Mikołajewski, E. Dostatni and M. Macko, Materials, 2020, 13, 5437 Search PubMed.
- M. Elbadawi, L. E. McCoubrey, F. K. Gavins, J. J. Ong, A. Goyanes, S. Gaisford and A. W. Basit, Adv. Drug Delivery Rev., 2021, 175, 113805 Search PubMed.
-
A. Banerjee, H. K. Haridas, A. SenGupta and N. Jabalia, Emerging Applications of 3D Printing During CoVID 19 Pandemic, 2022, pp. 57–79 Search PubMed.
- Z. Grof and F. Štěpánek, Comput. Chem. Eng., 2021, 154, 107492 Search PubMed.
-
G. Subramanian, Process control, intensification, and digitalisation in continuous biomanufacturing, John Wiley & Sons, 2021 Search PubMed.
- H. Valizadeh, M. Pourmahmood, J. S. Mojarrad, M. Nemati and P. Zakeri-Milani, Drug Dev. Ind. Pharm., 2009, 35, 396–407 Search PubMed.
- Z. Zhou, X. Li and R. N. Zare, ACS Cent. Sci., 2017, 3, 1337–1344 Search PubMed.
- D. Q. Gbadago, J. Moon, M. Kim and S. Hwang, Chem. Eng. J., 2021, 409, 128163 Search PubMed.
- W. C. Wong, E. Chee, J. Li and X. Wang, Mathematics, 2018, 6, 242 Search PubMed.
- I. Baranilingesan, Curr. Sci., 2021, 120(8), 1324–1333 Search PubMed.
- H. Salami, M. A. McDonald, A. S. Bommarius, R. W. Rousseau and M. A. Grover, Org. Process Res. Dev., 2021, 25, 1670–1679 Search PubMed.
-
S. Chen, T. Liu, D. Xu, Y. Huo and Y. Yang, Image based measurement of population growth rate for l-glutamic acid crystallization, Paper presented at: 2019 Chinese Control Conference (CCC), 2019.
- Y. Roggo, M. Jelsch, P. Heger, S. Ensslin and M. Krumme, Eur. J. Pharm. Biopharm., 2020, 153, 95–105 Search PubMed.
- B. Nagy, D. L. Galata, A. Farkas and Z. K. Nagy, AAPS J., 2022, 24, 74 Search PubMed.
-
S. Ramakrishna, L. Tian, C. Wang, S. Liao and W. E. Teo, Medical devices: regulations, standards and practices, Woodhead Publishing, 2015 Search PubMed.
- US FDA, Guidance for Industry Part 11, Electronic Records; Electronic Signatures – Scope and Application, 2003, FDA-2003-D-0143.
- US FDA, Considerations for the Use of Artificial Intelligence to Support Regulatory Decision-Making for Drug and Biological Products Guidance for Industry and Other Interested Parties, 2025, FDA-2024-D-4689.
- US FDA, Center for Drug Evaluation and Research, Artificial Intelligence in Drug Manufacturing, 2023, FDA-2023-N-0487.
-
S. S. Kuwahara, Continuous Biomanufacturing–Innovative Technologies and Methods: Innovative Technologies and Methods, 2017, 533–548 Search PubMed.
-
N. Bhatnagar, Role of Robotic Process Automation in Pharmaceutical Industries, Paper presented at: The International Conference on Advanced Machine Learning Technologies and Applications (AMLTA2019), 2020, https://link.springer.com/chapter/10.1007/978-3-030-14118-9_50.
- O. Joel, A. Oyewole, O. Odunaiya and O. Soyombo, Int. J. Manag. Entrep. Res., 2024, 6, 707–721 Search PubMed.
-
M. DeMello
, Artificial Intelligence at Pfizer – 3 Use Cases, https://emerj.com/artificial-intelligence-at-pfizer/, (accessed 26.01.2025).
- N. Shah, Comput. Chem. Eng., 2004, 28, 929–941 Search PubMed.
- M. Salas, J. Petracek, P. Yalamanchili, O. Aimer, D. Kasthuril, S. Dhingra, T. Junaid and T. Bostic, Pharm. Med., 2022, 36, 295–306 Search PubMed.
- L. Liang, J. Hu, G. Sun, N. Hong, G. Wu, Y. He, Y. Li, T. Hao, L. Liu and M. Gong, Drug Saf., 2022, 45, 511–519 Search PubMed.
- Y. Chen, J. D. Elenee Argentinis and G. Weber, Clin. Ther., 2016, 38, 688–701 Search PubMed.
-
F. H.-L. R. Ltd, A Year in Review 2020, Basel, Switzerland, 2021 Search PubMed.
- I. Singh, J. Kaur, S. Kaur, B. R. Barik and R. Pahwa, Braz. Arch. Biol. Technol., 2023, 66, 1–16 Search PubMed.
-
S. Singh, P. Shakdwipee and D. Shrimali, Harnessing Artificial Intelligence in Financial Operations: Opportunities and Challenges Conference paper First Online: 31 October 2024, pp. 547–560, https://link.springer.com/chapter/10.1007/978-981-97-6678-9_48?fromPaywallRec=false.
- P. Ullagaddi, J. Adv. Med. Pharm. Sci., 2024, 26, 75–86 Search PubMed.
-
F. Pasquale, New Laws of Robotics, Defending Human Expertise in the Age of AI, Harvard University Press, 2020 Search PubMed.
- Z. Obermeyer, B. Powers, C. Vogeli and S. Mullainathan, Science, 2019, 366, 447–453 Search PubMed.
- N. Nwebonyi and F. McKay, BMC Med. Ethics, 2024, 25, 113 Search PubMed.
- A. S. Albahri, A. M. Duhaim, M. A. Fadhel, A. Alnoor, N. S. Baqer, L. Alzubaidi, O. S. Albahri, A. H. Alamoodi, J. Bai and A. Salhi, Inf. Fusion, 2023, 96, 156–191 Search PubMed.
- R. Challen, J. Denny, M. Pitt, L. Gompels, T. Edwards and K. Tsaneva-Atanasova, BMJ Qual. Saf., 2019, 28, 231–237 Search PubMed.
- J. Zhang and Z.-m. Zhang, BMC Med. Inf. Decis. Making, 2023, 23, 7 Search PubMed.
- R. B. Parikh, S. Teeple and A. S. Navathe, J. Am. Med. Assoc., 2019, 322, 2377–2378 Search PubMed.
- L. Belenguer, AI Ethics, 2022, 2, 771–787 Search PubMed.
- R. M. Ratwani, K. Sutton and J. E. Galarraga, J. Am. Med. Assoc., 2024, 332, 1051–1052 Search PubMed.
- D. F. Dariush and Z. Shaghayegh, Iran. J. Public Health, 2021, 50, i–v Search PubMed.
- H. B. Harvey and V. Gowda, Acad. Radiol., 2020, 27, 58–61 Search PubMed.
- R. S. Patil, S. B. Kulkarni and V. L. Gaikwad, Drug Discovery Today, 2023, 103700 Search PubMed.
- H. B. Harvey and V. Gowda, Radiol. Clin., 2021, 59, 1075–1083 Search PubMed.
- A. Romagnoli, F. Ferrara, R. Langella and A. Zovi, Pharm. Res., 2024, 41, 721–730 Search PubMed.
- M. Aboy, T. Minssen and E. Vayena, npj Digit. Med., 2024, 7, 237 Search PubMed.
- D. W. Opderbeck, Fordham L. Rev., 2019, 88, 553 Search PubMed.
- L. Nene, B. T. Flepisi, S. J. Brand, C. Basson and M. Balmith, Clin. Ther., 2024, e6–e14 Search PubMed.
- L. K. Vora, A. D. Gholap, K. Jetha, R. R. S. Thakur, H. K. Solanki and V. P. Chavda, Pharmaceutics, 2023, 15, 1916 Search PubMed.
- Z. Jan, F. Ahamed, W. Mayer, N. Patel, G. Grossmann, M. Stumptner and A. Kuusk, Expert Syst. Appl., 2023, 216, 119456 Search PubMed.
- P. Bhatt, S. Singh, V. Kumar, K. Nagarajan, S. K. Mishra, P. K. Dixit, V. Kumar and S. Kumar, Curr. Artif. Intell., 2024, 2, E051223224198 Search PubMed.
-
S. Anuyah, M. K. Singh and H. Nyavor, arXiv, 2024, preprint, arXiv:2412.07050, DOI:10.30574/wjarr.2024.24.3.3671.
-
R. E. Hariry, R. V. Barenji and A. Paradkar, Handbook of Smart Materials, Technologies, and Devices: Applications of Industry 4.0, 2020, pp. 1–22 Search PubMed.
|
This journal is © The Royal Society of Chemistry 2025 |
Click here to see how this site uses Cookies. View our privacy policy here.