DOI:
10.1039/D4RA07949C
(Paper)
RSC Adv., 2025,
15, 1438-1446
Towards homogenous multiwell plate based pH sensors using a responsive triangulenium dye and an ATTO-647 reference dye†
Received
8th November 2024
, Accepted 24th December 2024
First published on 16th January 2025
Abstract
pH remains the most important chemical parameter and must be monitored for positive outcomes in areas as different as cheese making and in vitro fertilisation (IVF). Where blood gas analysers enable patient monitoring, starter cultures in cheese manufacturing are still monitored using conventional pH electrodes. Here, we present a homogeneous multiwell plate sensor for monitoring pH, with the same sensitivity as a pH electrode. The homogenous sensor operates in small liquid samples and uses two components: a pH responsive triangulenium dye on a polystyrene nanoparticle, and a freely diffusing commercial reference dye. Sensor measurements were made in triplicate to investigate and document the performance and robustness of the individual components, before we moved on to investigate the multiwell plate sensor design. The pH sensor was first tested in cuvettes, before moving to microwells and smaller volumes. The target is to monitor pH in IVF cultures, and the sensor proved to be operational in the pH range found in in vitro fertilisation buffers, but the references dye was shown not to be suitable for sensors. We conclude that the homogeneous sensor design is sound, but reaching the required precision of ΔpH = 0.01 can only be done with a different reference dye.
Introduction
The importance of sensors in industrial applications cannot be overstated. Sensors measure temperature, pressure, and the concentration of specific chemical species. They are indispensable for monitoring changes in agricultural systems,1,2 beer brewing,3 drug development, and pharmaceutical production.4,5 Another example of the critical need for quality sensors is that an ordinary car contains more than 100 sensors that perform continuous measurements.6–10 In bioproduction, chemosensors enable the monitoring of concentrations of a selection of chemical species.11–13 In particular pH remains a highly important parameter for industrial bioproduction and bioprocessing.9,14–17 In an industrial context, optical sensors offer many advantages. They are often low-cost, non-invasive and stable for longer than conventional sensors.10,18–20 Several optical chemosensors for the measurement of dissolved oxygen and pH have been introduced to the market.15,17,21–23
For repeated, parallel and high throughput sensing, multiwell plate sensors for pH have been shown to be advantageous, most of these use a thin film deposited in each well of the plate.24–27 Homogeneous multiwell-plate sensors offer several additional advantages.28 They use commercial software, eliminate mass-transport issues, and are readily fabricated by sequential additions of the sensor components.29–32 The sensing action can exploit any optical sensor as the responsive component. Here, we focus on optical pH sensors, where there are many reports of nanooptodes for fluorescence sensing,33–40 and responsive bioimaging.31,38,39,41,42 Nanooptodes, or nanoparticle based optical sensors, have several advantages. First, surface modifications can ensure biocompatibility and full solubility of all the sensor components.43–45 And second, nanoparticle sensors can accommodate a wide variety of different dyes.29–32 This is important, as a homogeneous multiwell-plate pH sensor must rapidly be formed upon sample addition, and the dyes must be robust for extended measurements.46 These two issues are addressed in this work.
Further challenges can be addressed using homogenous multiwell-plate sensors based on responsive nanoparticles. More complicated analytes that cannot be measured in a heterogeneous system due to mass transport limitations, should be readily detected by a homogenous sensor. As several areas of the industry have a need for sterile single-use sensing platforms,15,17 we look to develop homogeneous multiwell-plate optical sensors. Here, we looked to the needs of monitoring in vitro fertilisation (IVF) treatments. Improved methods of sensing pH of incubation mediums are needed, as the only option today is to use blood-gas analysers developed to meet a completely different demand.47,48
During embryo culturing, pH must be controlled indirectly using the partial pressure of CO2, which only influences the culture medium indirectly through diffusion through the oil covering the small volume containing the IVF embryo.49–51 pH in IVF is critical,49,50,52–54 must be kept constant—the recommended pH is 7.1–7.4—, and there is regulatory demand that the pH is measured throughout the process. Current means of measuring pH in IVF incubation are limited,49,50,55,56 and the indirect measurement of CO2 gas concentration in incubation chamber is favoured. The process of embryo culturing takes four days,57 and a single-use pH sensor is required as a minimum to be stable in this timespan, must be able to be placed in the incubator, and be read without disturbing the embryo. Further, the sensor must be very precise (error < 0.01 pH) in the narrow pH range from 7.1 to 7.4, corresponding a proton concentration between 40 nM and 79 nM.
Here, we attempt to realize such a sensor using a pH responsive triangulenium dye and a commercial reference dye. As mentioned above, the main challenges are creating a stable suspension of the dyes in the culture medium and reliable readout. Both are achieved if the sensor is truly homogenous, and all sensor components are stable. We address the challenge by using a nanoparticle platform, see Fig. 1, with the highly photostable triangulenium dye. These dyes are known to produce robust sensors, however, the reference dye remains a challenge.20,58–65 We have previously reported optical pH sensors based on the highly photostable diazaoxatriangulenium (DAOTA) dye, both using custom hardware and spectrometers.20,58,66,67 The triangulenium dyes in general, have proven to be a series of exceptionally photostable fluorophores. In addition to this, they possess unique photophysical properties as long fluorescent lifetimes and high quantum yields.60,61,65,68 Working in typical IVF buffers such as HEPES and MOPS, the hypothesis was that using a robust, commercial fluorescent dyes would alleviate the issues of finding a useable reference dye. This turned out not to be the case.
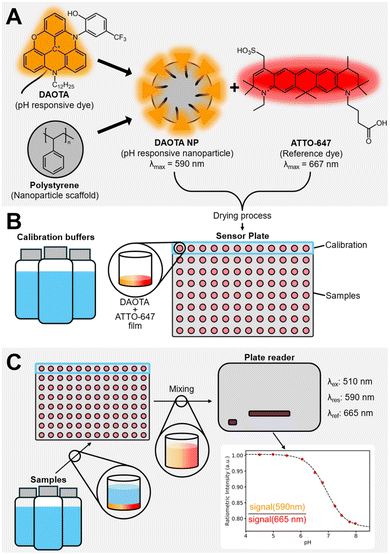 |
| Fig. 1 (A) Sensor components: water-soluble polystyrene nanoparticles functionalised with a pH responsive diazaoxatriangulenium (DAOTA) dye and the water-soluble ATTO-647 dye. (B) The deposition and drying of pH responsive and reference dyes into a multiwell plate, resulting in the final pH sensor formulation. The multiwell sensor consists of a calibration series (top row), with the remaining wells available for samples. (C) Using the pH sensor in a commercial plate reader, showing sample deposition, mixing, reading and representative data and response curve. | |
In this work, we present a homogeneous single-use pH sensor in a multiwell plate, with the DAOTA fluorophore, but moving to a nanoparticle-based approach. This allows us to circumvent the lipophilic nature of the DAOTA dyes by creating polystyrene-based nanoparticles (NPs)69–71 and further combat issues with fluorescent probes, such as leaching of the dye into surrounding media, while maintaining optimal access between the analyte and the receptor of the sensor. The NPs are coated with Triton X-100. A non-ionic surfactant that was been used extensively in bioremediation research and has proven to be less toxic than ionic counterparts and an effective solubilizing agent.72,73 The NP surfaces are decorated with the pH responsive DAOTA dye. The sensing components are completed by combining the responsive NPs with a freely diffusing ATTO-647 reference dye. The mixture is deposited on the bottom of a multiwell plate, ready for sensing. In this sensing formulation, the responsive and reference dyes are physically decoupled, eliminating possible energy transfer pathways, such as FRET, between the two dyes.69,74 Fig. 1 shows the sensor components and the schematic of the multiwell sensing setup.
The advantages of this pH sensing formulation include: (i) low cost of production, compared to conventional electrochemical pH probes. (ii) High sample throughput, by switching to a multiwell based sensing platform. Furthermore, by switching to a multiwell based platform, we are mimicking the instrumental setup used for embryo incubation in IVF treatment. From an experimental point of view, this also simplifies parameter studies for sensor optimization.
The performance of the homogenous NP-based pH sensor was evaluated in a series of cuvette experiments before translation to a microwell-based setup. This is the actual sensor format for IVF and allowed for a significantly increased sample throughput. The sensor fabrication was optimized, and finally the response and sensing precision was demonstrated, using a selection of Good's buffers, commonly used in biological systems and IVF treatment. We found that ATTO-647 is not stable and that the format works for sensing pH.
Methods and materials
Reagents and materials
The pH responsive DAOTA dye with pKa = 6.05 was prepared as hexafluorophosphate salts as previously reported b(N-dodecyl-N′-(2-hydroxy-5-trifluoromethylphenyl)-diazaoxatriangulenium hexafluorophosphate, C38H38F3N2O2·PF6, M = 756.69 g mol−1).75 ATTO-647 was purchased from ATTO-TEC. Polystyrene (Mw: 35
000), Triton X-100, 4-(2-hydroxyethyl)-1-piperazineethane-sulfonic acid (HEPES), toluene and Pur-A-Lyzer Maxi Dialysis tubes (3 ml, MWCO: 12–14 kDa) were purchased from Sigma-Aldrich. 3-Morpholinopropane-1-sulfonic acid (MOPS) 0.5 M, pH 7.0 and 2-hydroxy-3-(morpholin-4-yl)propane-1-sulfonic acid (MOPSO) 0.2 M, pH 7.0 were purchased from Thermo Fisher Scientific. 0.02 M universal buffer was prepared using boric, acetic, and phosphoric acid.
Greiner Bio-One 96-well black F-bottom multiwell plates were purchased from VWR and Kartell 96-well clear multiwell plates were purchased from Buch & Holm.
Dynamic light scattering
Multi-angle Dynamic Light Scattering (DLS) was done using a Malvern Zetasizer Ultra. Scattered light was collected at angles of 12.78°, 90° and 174.7° from the incident light beam. 30 collection runs with a measurement time of 3.36 s were done.
For each sample this process was repeated 3 times.
Scanning electron microscopy
Scanning electron microscopy (SEM) samples were prepared on silica wafers as follows: wafers were rinsed and cleaned using soap and water, followed by rinsing with absolute ethanol and dried with nitrogen. Following this, any leftover impurities were removed by treatment with a Piezo Brush PZ2 plasma gun for one minute. Wafers were finally rinsed with absolute ethanol and dried with nitrogen.One drop of sample solution was deposited unto a prepared wafer and left for five minutes. Remaining liquid was removed, and the sample dried with nitrogen.
SEM images were obtained on a Jeol 7800F-Prime electron microscope using Lower Electron Detection (LED) mode.
Plate reader measurements
Prepared 96 well assays were measured in a PHERAstar FSX plate reader from BMG Labtech. An optic module with excitation at λ = 510 nm and dual emission at λ = 590 nm (DAOTA)/665 nm (ATTO-647, bandwidth for all: 10 nm) was used. The PMT gain value and focus height was determined using the built in procedure, resulting in an emission signal strength of 85% of the detector maximum for the DAOTA channel. The signal was detected using the top optic. Additional details along with data from the plate reader measurements can be found as ESI.†
Fluorescence spectroscopy
Emission and excitation spectra were obtained with a Cary Eclipse fluorescence spectrometer using disposable PMMA cuvettes. Emission spectra were obtained by excitation at λ = 510 nm, mimicking the excitation slit used during the plate reader measurements.
Nanoparticle synthesis
The synthesis was done using a sonication microemulsion method following the procedure, previously reported by D. Bartoš et al.69 Polystyrene (PS) beads (Mw: 35
000 g mol−1) were dissolved in toluene, resulting in a 0.8 w/w% solution. The DAOTA dye was dissolved in the PS/toluene solution to a concentration of 0.3 mM. Triton X-100 was dissolved in water for a 5.35 mg ml−1 solution. The DAOTA/PS solutions were mixed with the Triton X-100 solution in a 1
:
7 ratio by pulsed sonication for 1 min, using a XS-sonic, FS-300N sonicator probe (cycling sonication of 1 s on and 1 s off), followed by magnet stirring for 1 h. The stirring and sonication process was done five times in total. Subsequently, the mixture was left for toluene evaporation by magnet stirring at room temperature for 24 hours. The obtained product was dialysed for a total of 3 days, using 3 ml dialysis tubes (MWCO: 12–14 kDa) in 2 L water. The water was changed after 2 and 24 hours. In total four different samples of nanoparticles were prepared, see Table 1. The first three batches were used on determining the optimal parameters for measurements using a plate reader and the final batch for evaluation of the sensor performance on IVF buffers.
Table 1 Nanoparticle synthesis batches with corresponding synthesis parameters
NP batch |
I |
II |
III |
IV |
PS mg ml−1 in toluene |
7 |
7 |
7 |
7 |
DAOTA mg ml−1 in toluene |
0.25 |
0.25 |
0.25 |
0.25 |
Triton X-100 mg ml−1 in water |
5.35 |
5.35 |
5.35 |
5.35 |
Mixture (toluene/water) |
2/14 ml |
2/14 ml |
2/14 ml |
2/14 ml |
Dialysis volume |
2 L |
2 L |
2 L |
2 L |
Multiwell plate fabrication
Eight batches of multiwell plate sensors were fabricated with several variations (see Table S1†). For each batch, three individual sensors were made to evaluate the fabrication robustness. The ESI† contains the characterisation of each batch.
The fabrication of a 96-well plate was done using an automated liquid handling platform (TECAN Freedom EVO). To optimise the sensing platform, series with different volumetric ratios of DAOTA NP/ATTO-647 were made. The optimisation of the sensor took seven iterations, batch I–VII in Table 2. The layout of the multiwell plate sensor for optimisation purposes is shown in Fig. 2, different ratios of responsive and reference dye—from 100
:
0, 80
:
20, 60
:
40, 40
:
60, 20
:
80, to 0
:
100 DAOTA/ATTO-647—were tested on the same plate. A row of blanks was included for each loading ratio.
Table 2 Sample list including known and unknown samples used for testing the final sensor design
Sample |
Type |
pH range |
Number |
Triplicates |
MOPS |
Unknown |
6.53–7.39 |
4 |
Yes |
MOPS |
Known |
6.75–7.62 |
4 |
Yes |
HEPES |
Unknown |
6.87–8.13 |
4 |
Yes |
MOPSO |
Unknown |
6.21–7.56 |
4 |
Yes |
Universal |
Calibration |
4.50–7.98 |
10 |
No |
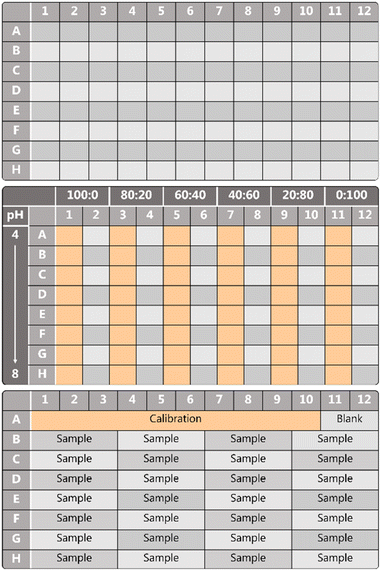 |
| Fig. 2 An overview of the multiwell plate-based sensor design. Top: 96-well plate dimensions. Center: Loading method for evaluation of the optimal ratio of pH responsive DAOTA NPs to ATTO-647 reference dye. Dye loaded and used wells are indicated in orange. Bottom: Final sensor layout, showing the calibration series and method of depositing sample triplicates in the multiwell plate. | |
To test the final sensor another fabrication—batch VIII in Table 2—was performed. Here the layout is simple as the sensor with a fixed DAOTA/ATTO-647 60
:
40 was distributed in all wells.
After loading, the sensors were dried at 50 °C overnight, resulting in a film at the bottom of each well. Two kinds of multiwell plates were used: 96-well Greiner bio-one black F-bottom (1) and 96-well Kartell – clear (2).
Sensor characterisation
Cuvette experiments. To evaluate the performance and robustness of sensor mixtures a pH titration series was done, and the emission spectrum recorded at each point. Response curves were determined from summation of the measured emission intensities at 580–600 nm, for the responsive dye, and 655–675 nm for the reference dye.
Multiwell plate experiments. To evaluate the performance and robustness of the I–VII sensor plates (see Table 2), the pH response signal was measured using universal buffer adjusted to a range of pH values from 4–8. The pH of each buffer solution was measured using a conventional potentiometric pH probe as reference.The buffer solutions were deposited in the multiwell plates using the automated pipetting system, 100 μl in each well, using the template as can be seen in Fig. 2. Each row is filled with the same buffer starting from low pH (pH ∼ 4) to high pH (pH ∼ 8).
After buffer deposition, the multiwell plates were sonicated using a sonicator bath for 3 minutes, to re-disperse the DAOTA:ATTO-647 film in the buffer solution before measuring using the plate reader as described above.
Sensor testing. To evaluate the performance and robustness of the final sensor plates (batch VIII, Table 2), the pH was measured in a series of samples using a universal buffer calibration series. The sensor layout is shown in Fig. 2, and the list of samples are shown in Table 2. The data is available as ESI.†A selection of Good's buffers used for in vitro fertilisation (MOPS, MOPSO and HEPES), were prepared and pH was adjusted to a range of values within each buffer's respective buffer range. The sensor characterisations were performed on batch VIII, with a 10-point standard curve determined in universal buffer for calibration, see Table 2 for details.
The sample and calibration solutions were deposited in the multiwell plates, 100 μl in each well, using the template as can be seen in Fig. 2. The pH of each sample was also measured using a conventional potentiometric pH probe before and five days after the measurement. All data is available in the ESI.† These also include the error from the conventional pH probe.
After sample deposition, the multiwell plates were sonicated using a sonicator bath for 3 minutes to re-disperse the DAOTA:ATTO-647 film. The samples were measured as described above.
Fitting procedure and propagation of errors
To generate the evaluation function for the pH sensor, the data was fitted to a sigmoidal response function: |
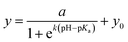 | (1) |
Here, y is the obtained response signal, a is the signal span, k is the shape factor of the curve and y0 is the baseline signal. By rewriting the evaluation function of the pH sensor was obtained. Here, pH is described with the measured signal y as the variable: |
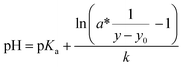 | (2) |
As written in eqn (2), the evaluation function provides pH by input of the measured signal intensity y.
χ2-Fitting was done to obtain the response function of the pH sensor. This was done from triplicate measurements of calibrations buffers. Non-linear propagation of errors was used to determine the error on pH-value readout of the evaluation function, from the measured optical signal intensity. The result of the error propagation is confidence intervals reported in for response curves and evaluation functions. The data for all batches is included in the ESI.†
The ratiometric signals obtained from the unknown samples were converted to pH using an evaluation function generated from the evaluation function generated from the response of calibration buffers on the same plate. The inherent error determined from the sensor characterisation from batch VII.
Results and discussion
The sensor components are shown in Fig. 1. The pH responsive triangulenium dye (DAOTA λfl = 590 nm, εmax = 15
940 M−1 cm−1, φFl = 65%, B = 10
000 M−1 cm−1) has been explored extensively elsewhere and is known to be an extremely stable fluorophore.58,68,75–79 The ATTO-647 (λfl = 667 nm, εmax = 1.2 × 105 M−1 cm−1, φFl = 20%, B = 24
000 M−1 cm−1) reference dye is known as one of the most stable red emitting dyes, but is known to have some stability issues above neutral pH.80 To ensure a homogenous assay, the triangulenium dye was immobilised on water-soluble nanoparticles, see Fig. 1. The lipophilic alkyl chain on the triangulenium dye irreversibly physisorbs the dye in the PS of the NP surface. The fact that extended dialysis does not remove the dyes, ensures that the nanoparticle shown in Fig. 1 is a stable species. The DLS results, see the ESI,† show that the NP are monodisperse and forms a stable suspension without aggregation. The response of the triangulenium dyes is maintained on the NP, see below. The PET mechanism of the response have been investigated in great detail elsewhere.75,76 This combination of dyes is new. Previously, TDI(bis(2,6-diisopropyl-phenyl)terrylene diimide) and DMQA(dimethoxy-quinacridinium) have been explored.20,67 We started by investigating the loading ratio between DAOTA-NP and ATTO-647 in cuvette-based experiments evaluated using a benchtop fluorometer.
SEM and DLS data (ESI Fig. S1 and Table S2† respectively) show that the Triton-X functionalised NPs have average sizes from 30–90 nm. In our hands, the relevant optical response is similar across all fabrications.69,74
The emission spectra of different ratios of DAOTA NPs and ATTO-647 were measured at varying pH values (Fig. 3). A fluorescence emission intensity drop is seen for DAOTA around 590 nm when pH is increased. This confirms that the pH response is maintained for the DAOTA NPs, as the response is identical to what was previously shown for the free dye.58,67,75 Considering the first panel in Fig. 3 it can clearly be seen, that a stronger reference signal is required for sensing purposes. Even though ATTO-647 is a brighter fluorophore than DAOTA, the fact that we excite at the edge of the ATTO-647 absorption band significantly reduces the ATTO-647 signal.
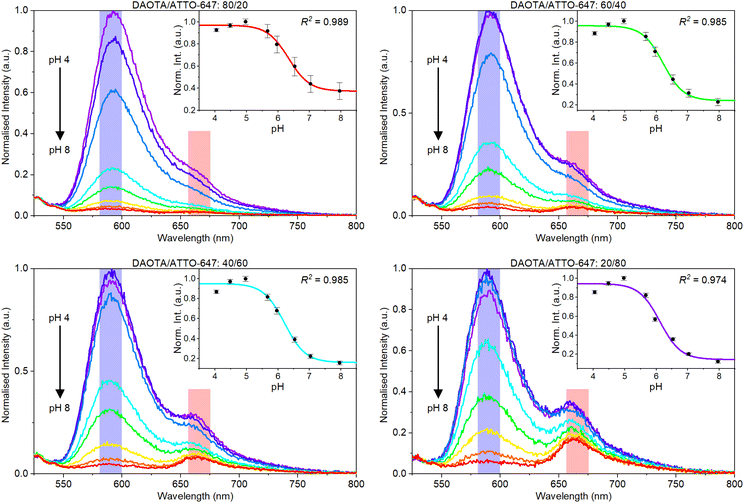 |
| Fig. 3 Fluorescence emission spectra of pH titrations (λEx = 510 nm), showing the effect of changing the DAOTA/ATTO-647 loading ratio on emission spectra and response curves (insets). The response curves were calculated as the ratio between the summed response signal (blue area) and the summed reference signal (red area), emulating the emission bands that were used during plate reader measurements. Error bars are calculated from the instrumental response error. | |
The insets in Fig. 3 show the normalised pH response obtained as the ratio between the DAOTA and ATTO-647 signals. These response data are generated from the summed intensities of each dye response (blue and pink respectively in Fig. 4) that correspond to the bandpass filters used in plate reader measurements. Visual inspection of the data indicates that more ATTO-647 is needed, even though the response data and response curves look reasonable for all DAOTA/ATTO-647 ratios.
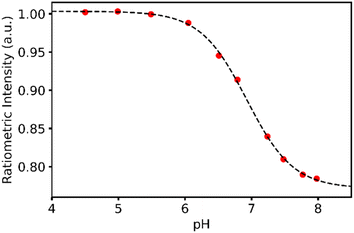 |
| Fig. 4 Response data and response curve for the calibration buffers used to determine the pH of IVF buffers. | |
Translating from cuvette to multiwell plate proved problematic. The DAOTA response dominates the signal obtained in the plate reader in both the DAOTA and reference channels. The response from the sensor resulted in a response that is too low for sensing purposes for batch I–VI. This manifests as large-to-infinite errors in the evaluation function, see ESI† for details. Consequently, large amounts of ATTO-647 had to be loaded in the multiwell plate to achieve a relevant sensitivity.
Characterising the multiwell plate pH assay
With sufficient ATTO-647 in the sensor (batch VII), we tested the response of different volumetric ratios of DAOTA/ATTO-647 (see ESI Fig. S20†). The best ratio was found to be 60
:
40. This was determined using nonlinear error propagation and investigating the error in the evaluation function. For each function the nonlinear error propagation results in a confidence interval, which is shown as a shaded area around each function. For the evaluation function, dashed lines centered around the pKa ±0.5 pH indicate the pH region of interest. Considering the functions shown in Fig. S20† it was concluded that the inherent error around in the pH region of interest for this sensor is 0.2. Further, we realise that we cannot achieve a more accurate response function unless we; (i) change the composition of the sensor, (ii) have higher precision in the individual date points, or (iii) have more response data to fit the response function. (ii) and (iii) are problematic to achieve, as (ii) requires better equipment and we are already using a state-of-the-art plate reader, and (iii) requires very high precision in the pH of the reference buffers. At this point we must accept that we are limited by the performance of ATTO-647, yet we conclude that and error of 0.2 is acceptable for a pH sensor prototype.
Testing the pH assay
We are targeting easy determination of pH during in vitro fertilisation. So, to evaluate the performance of the sensor we made a sensor and tested in in vitro fertilisation buffers with known and unknown pH. The calibration was done using universal buffer, and the samples were run in triplicates.
The calibration data is shown in Fig. 4. The unknown samples were evaluated using the response function folded with the known fabrication error. The result of the measurements and this exercise is shown in Fig. 5.
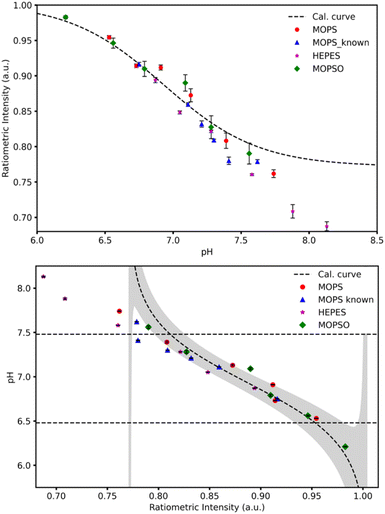 |
| Fig. 5 Top: Calibration response function and measured data from known and unknown samples of IVF buffers. Bottom: Evaluation function and determined pH values of known and unknown samples of IVF buffers. The grey area is the error on the sensor obtained from the optimised sensor design (batch VII). Data points are plotted at the pH values obtained from a reference pH probe and errors are the standard deviations obtained from triplicate samples in the plate propagated to correspond to sensor signal error (top) and pH error (bottom). | |
Considering the data in Fig. 5, we immediately realise that the sensor has a problem at pH > 7, as we see the real pH–pH determined using a conventional pH probe—of the samples deviate from the pH determined by the homogenous multiwell plate sensor. The signal in the reference channel is unphysical, and we must conclude that the reference dye is hydrolysing in the IVF buffers. Even though we selected the most stable fluorescent dye available to us, it is apparently not suited in this sensor format.
In the calibration data in Fig. 4, we excluded two data points due our observation that the reference dye was not stable above pH 8 across all buffers. And in the IVF buffers the problem becomes apparent already from pH = 7.
The manufacturer reports that ATTO-647 degrades by nucleophilic attack in solvents like DMF and DMSO, while it in alkaline solution will react with hydroxide. We assumed that on the timescales and pH range we are interested in, this would be a minor effect. However, when we consider the data on the unknown samples shown in Fig. 5, the instability is evident already at neutral pH, and we must conclude that the sensor is only operational to pH ∼ 7.3.
To overcome this limitation of the reference dye, we propose moving the reference dye to a NP platform similar to what has been done with the pH responsive dye. Encapsulation within polystyrene NPs will reduce possible degradation from interaction with the solvent. Previously, we have had success with encapsulating a series of dyes within polystyrene NPs.69
Conclusions
While heterogeneous multiwell-plate pH sensors are known, and few reports of homogenous multiwell-plate pH sensors exist, this is the first report of a homogenous multiwell-plate pH sensors based on robust pH responsive dyes on a nanoparticle platform. A homogenous multiwell plate pH sensor was developed using a new reference dye. A robust design of the active sensor components was achieved, a homogenous sensor was made, but the ATTO-647 reference dye did not solve the issues previously seen with other reference dyes. We must conclude that the ATTO-647 dye is not stable and cannot be used in a wide range pH sensor.
Thus, the pH sensor we report on here has a range from 6.5 to 7.3, and the pH can be determined with a single point measurement error as low as 0.02 (reproducibility), even though the systematic error of the current embodiment of the sensor is 0.2 (precision). This is suitable to follow dairy production e.g. cheese making, but for monitoring IVF we need further improvements, that is a better reference dye system. This should also lead to an error below ΔpH = 0.02.
With future homogenous multiwell-plate sensors developed using this methodology, only the selection of dyes will limit the possible applications. Sugar-responsive molecules, will allow for determination of blood sugar, residual sugar in fermentations and lactose content in dairy. Heavy metal responsive dyes, will make sensors for environmental monitoring og e.g. lead and mercury. And optimized pH-sensors will be important brewing, biotechnological production and diagnostics.
Data availability
All data is available as ESI.†
Conflicts of interest
The authors are in progress of protecting relevant IP, and the responsive triangulenium dye is patented by the University of Copenhagen (WO2015058777A1).
Acknowledgements
We thank Gustav Skødt for preparing the blind samples. We thank Carlsbergfondet, Novo Nordisk Fonden and The University of Copenhagen for financial support.
Notes and references
- T. Merl, Y. Hu, J. Pedersen, S. E. Zieger, M. L. Bornø, A. Tariq, S. G. Sommer and K. Koren, Environ. Sci.:Adv., 2023, 2, 1210–1219 CAS.
- T. Merl, C. J. Sedlacek, P. Pjevac, L. Fuchslueger, T. Sandén, H. Spiegel, K. Koren and A. T. Giguere, Soil Biol. Biochem., 2024, 189, 109273 CrossRef CAS.
- W. Stilman, G. Wackers, S. B. Sichani, M. Khorshid, F. Theßeling, J. Vereman, L. Andruck, D. Elian, P. Cornelis, J. V. Impe, K. Verstrepen, I. Van de Voorde and P. Wagner, Sens. Actuators, B, 2022, 373, 132690 CrossRef CAS.
- G. Maduraiveeran, M. Sasidharan and V. Ganesan, Biosens. Bioelectron., 2018, 103, 113–129 CrossRef CAS PubMed.
- P. Biechele, C. Busse, D. Solle, T. Scheper and K. Reardon, Eng. Life Sci., 2015, 15, 469–488 CrossRef CAS.
- O. S. Wolfbeis, Angew. Chem., Int. Ed., 2013, 52, 9864–9865 CrossRef CAS PubMed.
- A. Dienstfrey and P. D. Hale, Meas. Sci. Technol., 2014, 25, 035001 CrossRef.
- O. S. Wolfbeis, J. Mater. Chem., 2005, 15, 2657–2669 RSC.
- B. H. Diehl, M. A. La Pack, T. Y. Wang, R. E. Kottmeier, S. M. Kaneshiro, M. C. Brandenstein, Y. Zhang, Y. C. Chiu, S. Yoon and V. M. Saucedo, BioPharm Int., 2015, 28, 28–31 Search PubMed.
- W. L. Rumsey, J. M. Vanderkooi and D. F. Wilson, Science, 1988, 241, 1649–1651 CrossRef CAS PubMed.
- Unisense, https://unisense.com/ Search PubMed.
- Endress+Hauser, https://www.endress.com/en Search PubMed.
- M. Toledo, https://www.mt.com/us/en/home.html.
- A. Koch, I. Pahl, M. Tanner, A. Burkhard, J. Kubischik, T. Peuker, D. Eibl, J. Kauling, W. Meusel, P. Kampeis, D. Müller, L. Böttcher, D. Frense, G. Jobst, I. Moser, H. Müller, T. Nacke, K. Preuß, D. Tillich, H. Weichert, R. Eibl, K. Muffler, P. Neubauer, R. Pörtner and R. Ulber, Single-use Technology in Biopharmaceutical Production, 2012 Search PubMed.
- H. Weichert, J. Lüders, M. Becker, T. Adams and J. Weyand, BioProcess Int., 2014, 12, 20–24 Search PubMed.
- N. H. Janzen, M. Schmidt, C. Krause and D. Weuster-Botz, Bioprocess Biosyst. Eng., 2015, 38, 1685–1692 CrossRef CAS PubMed.
- C. Card, K. Clark and J. Furey, BioProcess Int., 2011, 9, 36–42 Search PubMed.
- X.-d. Wang and O. S. Wolfbeis, Anal. Chem., 2020, 92, 397–430 CrossRef CAS PubMed.
- H. S. Mader and O. S. Wolfbeis, Anal. Chem., 2010, 82, 5002–5004 CrossRef CAS PubMed.
- M. Rosenberg, B. W. Laursen, C. G. Frankær and T. J. Sørensen, Adv. Mater. Technol., 2018, 3, 1800205 CrossRef.
- J. Lin, TrAC, Trends Anal. Chem., 2000, 19, 541–552 CrossRef CAS.
- D. Wencel, T. Abel and C. McDonagh, Anal. Chem., 2014, 86, 15–29 CrossRef CAS PubMed.
- U. Kosch, I. Klimant, T. Werner and O. S. Wolfbeis, Anal. Chem., 1998, 70, 3892–3897 CrossRef CAS.
- H. R. Kermis, Y. Kostov, P. Harms and G. Rao, Biotechnol. Prog., 2002, 18, 1047–1053 CrossRef CAS PubMed.
- S. Arain, G. T. John, C. Krause, J. Gerlach, O. S. Wolfbeis and I. Klimant, Sens. Actuators, B, 2006, 113, 639–648 CrossRef CAS.
- B. Vilozny, A. Schiller, R. A. Wessling and B. Singaram, J. Mater. Chem., 2011, 21, 7589–7595 RSC.
- S. Wu, S. Wu, Z. Yi, F. Zeng, W. Wu, Y. Qiao, X. Zhao, X. Cheng and Y. Tian, Sensors, 2018, 18, 564 CrossRef PubMed.
- A. S. Kocincová, S. Nagl, S. Arain, C. Krause, S. M. Borisov, M. Arnold and O. S. Wolfbeis, Biotechnol. Bioeng., 2008, 100, 430–438 CrossRef PubMed.
- Z. Luo, L. Zhang, R. Zeng, L. Su and D. Tang, Anal. Chem., 2018, 90, 9568–9575 CrossRef CAS PubMed.
- X.-S. Zheng, P. Hu, Y. Cui, C. Zong, J.-M. Feng, X. Wang and B. Ren, Anal. Chem., 2014, 86, 12250–12257 CrossRef CAS PubMed.
- W. Wu, J. Shen, Z. Gai, K. Hong, P. Banerjee and S. Zhou, Biomaterials, 2011, 32, 9876–9887 CrossRef CAS PubMed.
- Y. Liu, L. Feng, T. Liu, L. Zhang, Y. Yao, D. Yu, L. Wang and N. Zhang, Nanoscale, 2014, 6, 3231–3242 RSC.
- R. V. Benjaminsen, H. Sun, J. R. Henriksen, N. M. Christensen, K. Almdal and T. L. Andresen, ACS Nano, 2011, 5, 5864–5873 CrossRef CAS PubMed.
- A. Steinegger, O. S. Wolfbeis and S. M. Borisov, Chem. Rev., 2020, 120, 12357–12489 CrossRef CAS PubMed.
- Y. Bao, H. De Keersmaecker, S. Corneillie, F. Yu, H. Mizuno, G. Zhang, J. Hofkens, B. Mendrek, A. Kowalczuk and M. Smet, Chem. Mater., 2015, 27, 3450–3455 CrossRef CAS.
- K. Zhou, Y. Wang, X. Huang, K. Luby-Phelps, B. D. Sumer and J. Gao, Angew. Chem., Int. Ed., 2011, 50, 6109–6114 CrossRef CAS PubMed.
- X.-d. Wang, R. J. Meier and O. S. Wolfbeis, Angew. Chem., Int. Ed., 2013, 52, 406–409 CrossRef CAS PubMed.
- K. J. Robinson, G. T. Huynh, B. P. Kouskousis, N. L. Fletcher, Z. H. Houston, K. J. Thurecht and S. R. Corrie, ACS Sens., 2018, 3, 967–975 CrossRef CAS PubMed.
- T. Näreoja, T. Deguchi, S. Christ, R. Peltomaa, N. Prabhakar, E. Fazeli, N. Perälä, J. M. Rosenholm, R. Arppe, T. Soukka and M. Schäferling, Anal. Chem., 2017, 89, 1501–1508 CrossRef PubMed.
- C. G. Frankær and T. J. Sørensen, Analyst, 2019, 144, 2208–2225 RSC.
- F. Pini, L. Francés-Soriano, V. Andrigo, M. M. Natile and N. Hildebrandt, ACS Nano, 2023, 17, 4971–4984 CrossRef CAS PubMed.
- A. A. Ansari, V. K. Thakur and G. Chen, Coord. Chem. Rev., 2021, 436, 213821 CrossRef CAS.
- C. Ding, A. Zhu and Y. Tian, Acc. Chem. Res., 2014, 47, 20–30 CrossRef CAS PubMed.
- Z. Bai, R. Chen, P. Si, Y. Huang, H. Sun and D.-H. Kim, ACS Appl. Mater. Interfaces, 2013, 5, 5856–5860 CrossRef CAS PubMed.
- Y. Zhou, Q. Li, Y. Wu, X. Li, Y. Zhou, Z. Wang, H. Liang, F. Ding, S. Hong, N. F. Steinmetz and H. Cai, ACS Nano, 2023, 17, 8004–8025 CrossRef CAS PubMed.
- V. Gutsal, S. Sieuwerts and R. Bibiloni, J. Dairy Res., 2018, 85, 453–459 CrossRef CAS PubMed.
- L. Chansel-Debordeaux, M. Carles, J. Moreau, C. Depuydt, S. Gallo, E. Genvrin, R. Léandri and N. Gatimel, J. Assist. Reprod. Genet., 2023, 40, 1677–1687 CrossRef PubMed.
- H. L. Higdon, D. W. Blackhurst and W. R. Boone, Fertil. Steril., 2008, 89, 703–710 CrossRef PubMed.
- J. E. Swain, Reprod. BioMed. Online, 2010, 21, 6–16 CrossRef PubMed.
- J. E. Swain, Hum. Reprod. Update, 2012, 18, 333–339 CrossRef CAS PubMed.
- S. P. L. Sörensen, Biochem. Z., 1909, 21, 131–304 Search PubMed.
- J. M. Squirrell, M. Lane and B. D. Bavister, Biol. Reprod., 2001, 64, 1845–1854 CrossRef CAS PubMed.
- K. Y. B. Ng, R. Mingels, H. Morgan, N. Macklon and Y. Cheong, Hum. Reprod. Update, 2017, 24, 15–34 CrossRef PubMed.
- B. Dale, Y. Menezo, J. Cohen, L. DiMatteo and M. Wilding, Hum. Reprod., 1998, 13, 964–970 CrossRef CAS PubMed.
- M. C. Summers and J. D. Biggers, Hum. Reprod. Update, 2003, 9, 557–582 CrossRef CAS PubMed.
- F. Berthelot and M. Terqui, Reprod., Nutr., Dev., 1996, 36, 241–251 CrossRef CAS PubMed.
- VitroLife, https://www.vitrolife.com/ivf-journey/culture/ Search PubMed.
- T. J. Sørensen, M. Rosenberg, C. G. Frankær and B. W. Laursen, Adv. Mater. Technol., 2019, 4, 1800561 CrossRef.
- J. Bosson, J. Gouin and J. Lacour, Chem. Soc. Rev., 2014, 43, 2824–2840 RSC.
- B. W. Laursen and F. C. Krebs, Angew. Chem., Int. Ed., 2000, 39, 3432–3434 CrossRef CAS.
- B. W. Laursen and F. C. Krebs, Chem.–Eur. J., 2001, 7, 1773–1783 CrossRef CAS.
- T. J. Sørensen, B. W. Laursen, R. Luchowski, T. Shtoyko, I. Akopova, Z. Gryczynski and I. Gryczynski, Chem. Phys. Lett., 2009, 476, 46–50 CrossRef PubMed.
- S. Dileesh and K. R. Gopidas, J. Photochem. Photobiol., A, 2004, 162, 115–120 CrossRef CAS.
- I. Bora, S. A. Bogh, M. Rosenberg, M. Santella, T. J. Sørensen and B. W. Laursen, Org. Biomol. Chem., 2016, 14, 1091–1101 RSC.
- S. A. Bogh, M. Simmermacher, M. Westberg, M. Bregnhøj, M. Rosenberg, L. De Vico, M. Veiga, B. W. Laursen, P. R. Ogilby, S. P. A. Sauer and T. J. Sørensen, ACS Omega, 2017, 2, 193–203 CrossRef CAS PubMed.
- C. G. Frankær, K. J. Hussain, M. Rosenberg, A. Jensen, B. W. Laursen and T. J. Sørensen, ACS Sens., 2018, 3, 692–699 CrossRef PubMed.
- C. G. Frankær, K. J. Hussain, T. C. Dörge and T. J. Sørensen, ACS Sens., 2019, 4, 26–31 CrossRef PubMed.
- E. Thyrhaug, T. J. Sørensen, I. Gryczynski, Z. Gryczynski and B. W. Laursen, J. Phys. Chem. A, 2013, 117, 2160–2168 CrossRef CAS PubMed.
- D. Bartoš, L. Wang, A. S. Anker, M. Rewers, O. Aalling-Frederiksen, K. M. Ø. Jensen and T. J. Sørensen, PeerJ Mater. Sci., 2022, 4, e22 CrossRef.
- L. Wang, K. Jensen, N. Hatzakis, M. Zhang and T. J. Sørensen, ACS Sens., 2022, 7, 1506–1513 CrossRef CAS PubMed.
- D. Bartoš, M. Rewers, L. Wang and T. J. Sørensen, Sens. Diagn., 2022 10.1039/d1sd00002k.
- D. Garon, S. Krivobok, D. Wouessidjewe and F. Seigle-Murandi, Chemosphere, 2002, 47, 303–309 CrossRef CAS PubMed.
- L. Abu-Ghunmi, M. Badawi and M. Fayyad, J. Surfactants Deterg., 2014, 17, 833–838 CrossRef CAS.
- L. Wang, P. Nawrocki, L. G. Nielsen, L. Grenier and T. J. Sørensen, Chem. Commun., 2022, 58, 9198–9201 RSC.
- C. G. Frankær, M. Rosenberg, M. Santella, K. J. Hussain, B. W. Laursen and T. J. Sørensen, ACS Sens., 2019, 4, 764–773 CrossRef PubMed.
- M. Rosenberg, A. K. R. Junker, T. J. Sørensen and B. W. Laursen, ChemPhotoChem, 2019, 3, 233–242 CrossRef CAS.
- I. Dalfen, R. I. Dmitriev, G. Holst, I. Klimant and S. M. Borisov, Anal. Chem., 2019, 91, 808–816 CrossRef CAS PubMed.
- M. Santella, E. Della Pia, J. K. Sørensen and B. W. Laursen, Beilstein J. Org. Chem., 2019, 15, 2133–2141 CrossRef CAS PubMed.
- C. Nicolas, G. Bernardinelli and J. Lacour, J. Phys. Org. Chem., 2010, 23, 1049–1056 CrossRef CAS.
- C. Trayford, D. Crosbie, T. Rademakers, C. van Blitterswijk, R. Nuijts, S. Ferrari, P. Habibovic, V. LaPointe, M. Dickman and S. van Rijt, ACS Appl. Nano Mater., 2022, 5, 3237–3251 CrossRef CAS PubMed.
Footnote |
† Electronic supplementary information (ESI) available: All plate reader data, detailed experimental descriptions and additional analysis. See DOI: https://doi.org/10.1039/d4ra07949c |
|
This journal is © The Royal Society of Chemistry 2025 |
Click here to see how this site uses Cookies. View our privacy policy here.