Quantitative read-across structure–property relationship (q-RASPR): a novel approach to estimate the bioaccumulative potential for diverse classes of industrial chemicals in aquatic organisms †
Received
25th June 2024
, Accepted 22nd October 2024
First published on 1st November 2024
Abstract
The Bioconcentration Factor (BCF) is used to evaluate the bioaccumulation potential of chemical substances in reference organisms, and it directly correlates with ecotoxicity. Traditional in vivo BCF estimation methods are costly, time-consuming, and involve animal sacrifice. Many in silico technologies are used to avoid the problems associated with in vivo testing. This study aims to develop a quantitative read across structure–property relationship (q-RASPR) model using a structurally diverse dataset consisting of 1303 compounds by combining quantitative structure–property relationship (QSPR) and read-across (RA) algorithms. The model incorporates simple, interpretable, and reproducible 2D molecular descriptors along with RASAR descriptors. The PLS-based q-RASPR model demonstrated robust performance with internal validation metrics (R2 = 0.727 and Q2(LOO) = 0.723) and external validation metrics (Q2F1 = 0.739, Q2F2 = 0.739, and CCC = 0.858). These results indicate that the q-RASPR model is statistically superior to the corresponding QSPR model. Furthermore, screening of 1694 compounds from the Pesticide Properties Database (PPDB) was performed using the PLS-based q-RASPR model for assessing the eco-toxicological bioaccumulative potential of various compounds, ensuring the external predictability of the developed model and confirming the real-world application of the developed model. This model offers a reliable tool for predicting the BCF of new or untested compounds, thereby helping to develop safe and environment-friendly chemicals.
Environmental significance
This study aims to establish a direct relationship between the bioaccumulative potential of various chemicals in aquatic organisms and their subsequent impact on human health through the food web. Accurate assessment of bioaccumulative and toxic compounds is crucial to mitigate adverse effects such as carcinogenicity, neurotoxicity, genotoxicity, immunotoxicity, and reproductive toxicity. Reducing the use of these harmful chemicals will protect aquatic life and public health. The present in silico approach, utilizing a PLS-based q-RASPR model, addresses the challenges related to animal testing, time, labor, and costs. This model effectively predicts the bioconcentration factor (BCF) of chemicals and offers valuable insights for designing environmentally safe chemicals, contributing to both environmental protection and human health.
|
Introduction
The inherent characteristics, as translated from PBT (persistent, bioaccumulative, and toxic), of various chemicals pose an increasing threat to both the aquatic and terrestrial ecosystems. Significant fractions of these chemicals can be detected over time in soil, crops, and in aquatic and terrestrial organisms, consequently deteriorating public health directly or indirectly. Upon release of these chemicals into the aquatic environment, a substantial amount can get bioaccumulated in fish and other aquatic organisms via their respiratory membranes, food, water, etc. Since the accumulation of high concentrations of chemicals at lower trophic levels of the food chain can have harmful consequences for higher trophic levels, knowledge about the bioaccumulative potential is important.1 The process of absorption of these chemicals from the environment by an aquatic organism only via its dermal and respiratory surfaces and not via the dietary route is known as bioconcentration. The BCF is defined as the ratio of the concentration of chemicals in reference aquatic organisms to the concentration of the corresponding freely dissolved chemicals in adjacent water media at steady-state.2 Usually, fish are used as the test model due to the availability of standardized guidelines and their significant role in the food web. BCF is measured in L kg−1.
where, Cf = concentration of chemicals within the fish (in mg kg−1), Cw = concentration of chemicals in the water compartment (in mg L−1).
The BCF value of a chemical can be determined under controlled laboratory conditions using OECD test guideline 305, which proposes measuring the concentration of chemicals in fish and the surrounding solution at an exposure time of up to 28 or 60 days, i.e. when steady state is reached.3 The European Union (EU) has established various guidelines for industries to decrease the threat posed by the emission of their chemical products. Estimation of the BCF for the assessment of various PBT and very Persistent very Bioaccumulative (vPvB) substances forms the main component of Annex XIII of EU REACH (Registration, Evaluation, Authorisation, and Restriction of Chemicals) regulations as given in EC no. 1907/2006.4 The chemicals having a BCF value in the range of 2000 L kg−1 to 4999 L kg−1 (3.30–3.699
log units) can be categorised as ‘bioaccumulative’ and those having a BCF of 5000 L kg−1 (3.7
log units) or beyond are categorized as ‘very bioaccumulative’ and the chemicals possessing a BCF of less than 2000 L kg−1 (3.30
log units) are considered to have non-significant bioaccumulative potential.4
In vivo/vitro estimation of the BCF involves sacrificing a large number of animal models. Nowadays, various models along with free software tools are available for predicting the log
BCF such as EPISUITE,5 VEGA,6 OPERA,7 and QSAR DataBank repository.8
Various computational methods, for example, QSAR/QSPR, read-across, and so on, are used to estimate the BCF and fill data gaps, decreasing the limitations of in vivo research. The integration of the concept of both QSAR, aiming at the development of a supervised learning model and RA which implicates similarity-based measures recognizing a close source compound for each target compound, has resulted in an emerging technique known as Read-Across Structure–Activity Relationship (RASAR).9 RASAR, a supervised learning algorithm has better predictivity as compared to conventional approaches. It links the physicochemical and molecular descriptors with similarity and error-based read-across measurements.10
Several QSPR models have already been proposed for the estimation of the BCF value of different compounds toward aquatic organisms. Gramatica et al.11 proposed MLR-OLS models for predicting the log
BCF value of 238 non-ionic organic compounds using various structural descriptors (i.e. 1D, 2D, 3D, and log
KOW). Purusottam et al.12 proposed a 2D QSAR model for predicting log
BCF using a dataset containing 55 different organophosphate pesticides. In another study, Kabiruddin et al.4 employed the BCF value of 122 pharmaceuticals to develop a regression-based QSPR model with 2D and fragment-based descriptors. Lunghini et al.13 used in silico design and data analysis (ISIDA) fragment descriptors as well as other machine learning algorithms to develop a QSPR model for the prediction of the BCF. Bertato et al.2 proposed QSAR models for regression and classification methods for the prediction of the BCF. PaDEL-descriptors were used to develop a multiple linear regression model by means of ordinary least squares (MLR-OLS) and artificial neural network (ANN) regression models as well as linear discriminant analysis (LDA) and ANN classification models.
Recent studies have explored various in silico approaches,14,15 to evaluate the bioconcentration factor (BCF) of chemical substances, employing advanced machine learning (ML) techniques. For instance, bioaccumulation patterns have been investigated through both uni- and multivariate analyses, as demonstrated by Grisoni et al. (2018).16 A predictive regression model (using 450 diverse compounds), utilizing recursive feature elimination (RFE) and support vector machine (SVM) algorithms,17 and a multiclassification model were reported to predict the acute toxicity of organic compounds.18,19 Furthermore, Yang (2022)20 applied the direct message passing neural network (DMPNN) to predict log
BCFs specifically for bisphenol analogues. Several studies, including those by Xu et al. (2023)21 and Toropova et al. (2013, 2020),22,23 utilized QSAR-QSIIR models and Monte Carlo simulations to assess the BCF for industrial pollutants and other organic compounds. While these models have provided valuable insights, they often face limitations regarding their accuracy in predicting BCFs for novel compounds and their ability to handle diverse chemical structures. Our study addresses these gaps by integrating molecular descriptors with advanced modeling techniques, thereby enhancing prediction accuracy and broadening the applicability of our model across different chemical categories and species.
In the current study, PLS-based QSPR and q-RASPR models were developed employing a dataset of 1333 chemical compounds, with log
BCF as an endpoint. Models were developed by stringently following OECD guidelines.24 The final PLS-based q-RASPR model was utilized for screening the PPDB database to identify the potentially toxic as well as safe chemicals and the results were validated with real-world data. Since the model was developed by employing a large dataset of diverse compounds, it can be stated that the model has a wide area of applicability. Based on the different internal and external validation parameters, the developed PLS-based q-RASPR model can be described as robust, predictive, and reliable.
Materials and methods
Data collection
The dataset of log
BCF values of 1333 heterogeneous compounds with the unit of L kg−1 bdwt was taken from the literature.13 Chemical structures were drawn with the help of Marvin Sketch software. Furthermore, the structures were converted to their aromatic form, and then explicit hydrogen atoms were added. The structures of all the dataset compounds were converted into a single .sdf file, which is a preliminary step for 2D descriptor calculation. The chemical diversity plot (molecular weight vs. LOGPcons) of the training and test sets to check the heterogeneity of the dataset is given in Fig. S1 of ESI 2.†
Descriptor calculation
2D molecular descriptors were calculated with the help of alvaDesc software.25 We calculated 2D molecular descriptors using alvaDesc software, which include (i) constitutional descriptors, (ii) ring descriptors, (iii) connectivity index, (iv) functional group counts, (v) atom-centred fragments, (vi) atom type E-states, (vii) 2D atom pairs, (viii) molecular properties and (xi) ETA indices. A pool of 2400 descriptors was obtained. From the initial pool of descriptors, we omitted some descriptors having constant or missing values as well as those with a paired absolute correlation larger than or equal to 0.950 and finally got a reduced pool of 856 descriptors (list is provided in ESI 1†).
Data pre-treatment and dataset division
After calculating the 2D descriptors, data pre-treatment was performed using DataPreTreatmentGUI 1.2 software.26 Data pre-treatment is a method of removing or omitting the descriptors that are less informative or insignificant. It involves the elimination of constant and highly correlated descriptors based on the variance cut-off (0.0001) and the inter-correlation cut-off (|r| > 0.95). This data pre-treatment step is very important before the procedure of feature selection, in order to avoid the inclusion of noisy descriptors. Some of the inorganic compounds, metals, and those having high residual values were deleted from the original dataset. The list of deleted compounds has been provided in ESI 1.† The dataset was then divided into training and test sets by various approaches like Kennard Stone, Euclidean distance-based, and activity/property-based employing datasetDivisionGUI1.2.jar.26 Among the above approaches, the best result was obtained through activity/property-based division. The training set (for model development) contained 978 compounds and the test set (for validating the developed model) comprised of 325 compounds.
Feature selection and QSPR model development
Feature selection, a vital step for QSPR model establishment, is necessary for selecting the most significant and manageable number of descriptors from the large pool of descriptors contributing to the response property. This can be performed using various strategies of variable selection.27 In this study, stepwise regression was performed for feature selection. In this case, stepwise regression was performed and some of the descriptors were selected. Then after excluding the selected descriptors, stepwise regression was again performed using the remaining pool of descriptors, and the process was repeated to get a reduced set of important descriptors using MINITAB software.28 The list of 51 descriptors obtained after the stepwise regression method which is provided in ESI 1.† Various MLR models with all probable combinations of 6 descriptors were generated with the employment of the Best Subset Selection v2.1 tool.26,29 Based on various internal and external validation metrics as well as the mean absolute error (MAEtest), a combination of 6 descriptors model was selected. The combination of 6 descriptors includes MLOGP (Moriguchi octanol–water partition coefficient), Cl-089 (atom-centered fragment descriptor indicating the presence of a Cl atom attached to a sp2 hybridized carbon), MaxdO (maximum E-state descriptor for the presence of carbonyl groups), X1A (average connectivity index of order 1), B03[C–N] (presence/absence of carbon and nitrogen atoms at topological distance 3), and MaxsOH (maximum E-state descriptor for the OH group). The final QSPR model was developed using the PLS regression method with the help of PLS_Single Y_version 1.0 software.26 As the descriptors are collinear and correlated, we employed the partial least squares (PLS) regression method for developing the QSPR model, to reduce any chance of inter-correlation between the modelled descriptors.30 The fundamental idea of PLS is the extraction of latent variables (LVs) which are functions of the original variables and account for the maximum expression of factor variation while modeling the response. LVs are extracted from the original set of descriptors and represent underlying patterns or relationships that contribute to the model's predictions. The LVs help to reduce dimensionality and address multicollinearity, ultimately enhancing the robustness and interpretability of the model. PLS regression involves the use of a reduced number of LVs and thus helps to generate a more robust model. Hence, this suggests that both X- and Y- variables are not independent and are realized through the LVs. With a reduced number of LVs the degree of freedom increases, hence leading to better results.31
Statistical validation metrics
The predictivity, stability, robustness, fitness, and reliability of the established models were assessed using internationally accepted internal and external validation parameters. Internal validation parameters include the determination coefficient (R2), the leave-one-out (LOO) cross-validated correlation coefficient (Q2(LOO)), and mean absolute error (MAEtrain). External validation parameters include the Q2F1 or R2pred, Q2F2, MAE(test), root mean square error of the test set (RMSEp), concordance correlation coefficient (CCC), rm2 metrics of the test set
etc. The threshold values of Q2(LOO), Q2F1, Q2F2, and
are 0.5, and that for CCC is 0.850, while the limit for Δrm(test)2 is 0.2.4,27
Read-across and RASPR descriptor calculation
Read-across is an unsupervised learning algorithm that does not involve the development of a model and does not affect the internal quality (training set validation), unlike the QSAR approach. It is solely based on the principle of molecular similarity, i.e., molecules with similar structures will tend to have similar biological activity and it provides more reliable and enhanced external predictivity (Q2F1, Q2F2, MAEtest, and RMSEp). The read-across approach makes the predictions for the test set compounds based on the training set compounds, eliminating the need to determine internal validation metrics. Here, we have only determined the external validation metrics such as Q2F1, Q2F2, and RMSEp to check the model's predictive ability and error measurement, respectively. The basic chemistry behind read-across predictions involves analog and category approaches. Basically, the analog approach uses single source compounds for predictions while the category approach uses multiple source compounds for prediction. In general, both approaches are based on the interpolation (when the query compounds are present in the range of the source compounds) and extrapolation (when the query compound is present outside the range of the source compounds) concepts.32 Optimization of the hyperparameters related to Euclidean distance, Gaussian kernel (σ), Laplacian kernel (γ), and the number of nearest source compounds is crucial for read-across based predictions.33 This approach gives prediction only for the training set by referencing test set compounds. The training set of the developed QSPR model is further separated into sub-train and sub-test sets for the weighted average predictions. The hyperparameters (σ and γ) were optimized based on the predictions of similarity and error-based measures using the Read-Across-v4.1 tool.34 The optimized settings obtained in the Gaussian kernel similarity approach (number of similar training compounds = 10, σ = 0.75, and γ = 0.75) were further used as input criteria for the RA predictions of original training and test sets. The optimized hyperparameters were employed to compute RASPR descriptors by means of RASAR-Desc-Calc-v2.0 software.10,35
Benefits of the read-across strategy.
The read-across approach predicts the toxicity of chemicals by leveraging similarities with well-characterized substances. This can be particularly useful in cases where experimental data are scarce or difficult to obtain. The read-across approach is a similarity-based non-animal testing approach that is widely accepted and encouraged by several regulatory bodies.34 By predicting the properties of untested chemicals, read-across reduces the need for extensive experimental testing, thereby saving time and resources. This aligns with the principles of the 3Rs (replacement, reduction, and refinement) in toxicology, as highlighted in various studies.10 The read-across method can be applied to a wide range of chemical classes, making it versatile. In our study, we utilized read-across to enhance the robustness of our predictions across a heterogeneous dataset, thus improving the model's generalizability.
Limitations of the read-across strategy.
The effectiveness of read-across relies heavily on the assumption that structurally similar compounds will exhibit similar biological properties.36 However, subtle structural differences or conformational flexibility can lead to divergent biological activities.10 This limitation means that predictions may sometimes be inaccurate, particularly for outlier compounds. Read-across assumes that similar structures share similar mechanisms of action, but this is not always the case. The presence of different functional groups or conformations can result in varying mechanisms, leading to potential inaccuracies in predictions.37 This limitation has been highlighted in studies such as those by Fukuchi et al.38 (2019) and Roy et al.10 (2022). The accuracy of read-across predictions is contingent on the availability and quality of existing data for structurally similar compounds. If the reference data are biased, incomplete, or of poor quality, the resulting predictions may be compromised. This limitation is particularly relevant in our study, where the dataset's heterogeneity necessitates careful selection of analogs for read-across.
q-RASPR model development
The 15 similarity and error-based RASPR descriptors (Table S1 in ESI 2†) were integrated with the descriptors of the QSPR model and q-RASPR models were generated9,10 with all probable combinations from the given pool of descriptors using BestSubsetSelectionModified_v2.1 software.26 The best model was selected on the basis of various internal and external validation metrics. The descriptors obtained in the final q-RASPR model include RA function (GK) (a composite function derived from Read-Across), Abs MaxPos–MaxNeg (absolute difference between the MaxPos and MaxNeg levels), Avg.Sim (GK) (average similarity level of the close source compounds), gm*SD similarity (product of the gm and SD similarity levels), and B03[C–N] (presence/absence of carbon and nitrogen atoms at topological distance 3). Furthermore, the final model was developed using the PLS method with the help of PLS_Single Y_version 1.0 software.29
Justification for using read-across and RASAR approaches
The motivation for adopting RA and RASAR in this study lies in their ability to address the limitations of conventional models. RA supplements data gaps by utilizing existing information on similar substances, while RASAR offers a modern, comprehensive approach to handle diverse chemical spaces and improve model performance. This combination not only aligns with current advancements in computational toxicology but also provides a more accurate and robust model for predicting toxicity.39,40
Applicability domain (AD)
OECD principle 3 states that each developed model should have a define domain of applicability. AD refers to the physicochemical, biological, or chemical space in which the model's training set is developed. This study employed the DModX (distance to model X) technique with the help of SIMCA-P software41 to estimate the domain of applicability of the final PLS-based q-RASPR model. The confidence level was maintained at 99%. The DModX approach uses X and Y residuals as investigative values to determine model quality. The compound found inside the AD can be reliably predicted and those located outside are referred to as ‘outliers’ and ‘outside AD’ for training and test sets, respectively.42
Y-randomization test
The Y-randomization test of the model was also performed using SIMCA-P software by 100 times arbitrary scrambling of the response value (log
BCF).43 A plot was generated between the original R2 and Q2LOO values against the 100 new R2 and Q2 values of the arbitrarily developed models. The intercepts, RY2 and QY2 should be less than the threshold limit (RY2 < 0.3 and QY2 < 0.05) which helps in detecting whether the models were developed by chance.
Screening of the external dataset
The final PLS-based q-RASPR model was then employed for the determination of log
BCF values of various pesticides obtained from the PPDB database.44 At first, pesticide data were taken from the PPDB database. Then curation of the dataset was performed employing the KNIME curation workflow29 to eliminate the mixtures and salts, finally resulting in a total of 1694 pesticides. Then the molecular descriptors were computed with the use of alvaDesc software. Screening of the database was performed using the Predictive Reliability Indicator (PRI) tool29 which provides an all-inclusive estimation and categorization of prediction quality for both the test set (with observed response) and external sets (without observed response). The PRI software defines the AD using a standardized approach, which helps ensure consistency and transparency in the modeling process. Specifically, the AD in PRI is determined based on the range and distribution of the descriptors used in the model. This approach involves assessing the overlap between the descriptor space of the training data and the new compounds being evaluated. By standardizing this definition, PRI provides a reliable measure of how well a new compound fits within the scope of the training data, which is crucial for assessing the validity and applicability of the model. This standardized method helps to maintain the robustness of predictions and enhances the overall transparency of the modeling process. The PRI tool helps to understand the quality of predictions for a true external set. It involves the use of three criteria45 that assist in classifying the quality of predictions for individual external set compounds.
Rule/criterion 1: Scoring based on the mean absolute leave-one-out prediction error (MAELOO) of the 10 closest compounds to an external set compound.
Rule/criterion 2: Scoring based on the applicability domain (AD) using a standardisation approach. This is based on the similarity of the external set compound with the training set.
Rule/criterion 3: Scoring based on the proximity of predictions to the training set's observed response mean.
After checking these criteria, a weighting scheme is applied for computing the composite score to assess the quality of prediction for each test compound based on all three individual scores. The composite score can be calculated as:
Composite score = W1 × scorerule1 + W2 × scorerule2 + W3 × scorerule3 |
where,
W1,
W2, and
W3 indicate the weightage of each of the three individual scores and score
rule1, score
rule2, and score
rule3 are the scores obtained after the application of the respective rules. A composite score of 3 is indicative of good prediction, a composite score of 2 is indicative of moderate prediction and a composite score of 1 is indicative of poor predictive ability of the model. Furthermore, the PRI software helps in determining whether the compounds are situated inside the AD or not; hence compounds lying outside can be removed from prediction. We have also checked the quality and reliability of the PLS-based q-RASPR model using different test sets from previous studies.
13 We have taken the training set of our model and checked the external validation parameters for the test set taken from previous literature.
13 The PRI tool was used to check the predictive ability of the model. The software tools employed in this study were chosen based on their user-friendliness, accessibility, and the specific functionalities they offer. Each tool was selected to address distinct aspects of the modeling process and to ensure the accuracy and reproducibility of our results. The use of these diverse tools allows for more precise and robust results in the context of our study.
46–49 The workflow of the present study is given in
Fig. 1.
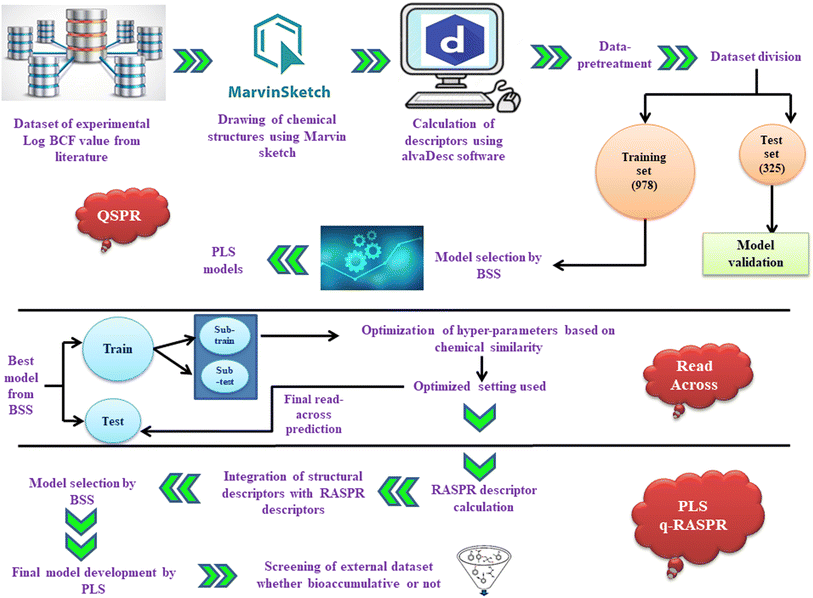 |
| Fig. 1 Workflow for the development of the PLS-based q-RASPR model. | |
Results and discussion
In the present study, we have employed the stepwise regression method for feature selection and obtained 51 descriptors from a large descriptors pool, as discussed in the “Materials and methods” section. A suitable combination of six descriptors (MLOGP, Cl-089, MaxdO, X1A, B03[C–N], and MaxsOH) was obtained using the best subset selection approach. The final PLS-based QSPR model was developed using the PLS regression method. Optimization of hyperparameters, crucial for RASPR descriptor calculations, was performed using the read-across approach. The optimized setting was then used to calculate the RASPR descriptors. Using the data-fusion approach, the 6 descriptors of the QSPR model were merged with the 15 RASPR descriptors (list is provided in ESI 1†). This was subjected to the best subset selection method and a combination of 5 descriptors was selected based on the various internal, external validation metrics as well as MAE criteria. The final PLS-based q-RASPR model was developed by using 5 descriptors (RA function (GK), Abs MaxPos–MaxNeg, Avg.Sim (GK), gm*SD similarity, and B03[C–N]) and validated rigorously using various internationally accepted validation metrics.
QSPR model development
The PLS-based QSPR model was developed by employing the training and test sets comprising 978 and 325 compounds, respectively. The model was developed by including six descriptors, and as the descriptors are correlated, the optimal number of components based on Q2LOO is 1, i.e. with 1 latent variable. The descriptors that are used in the development of the QSPR model include MLOGP, Cl-089, MaxdO, X1A, B03[C–N], and MaxsOH. The statistical metrics for the QSPR model are reported in Table 1. The equation (Eqn (1)) for the PLS-based QSPR model is given below.
Table 1 Statistical results of the developed modelsa
Model details and validation metrics |
PLS-QSPR model |
Read-across |
PLS-based q-RASPR model |
LVs: latent variable, no. of desc.: number of descriptors.
|
N
train/Ntest |
978/325 |
|
978/325 |
No. of descriptors |
6 |
|
5 |
Number of LVs |
1 |
|
2 |
R
2
|
0.654 |
|
0.727 |
Q
2
(LOO)
|
0.650 |
|
0.723 |
MAE(LOO) |
0.677 |
|
0.581 |
MAE-FITTED |
0.674 |
|
0.577 |
Q
2
F1
|
0.718 |
0.763 |
0.739 |
Q
2
F2
|
0.718 |
0.763 |
0.739 |
CCC |
0.837 |
|
0.858 |
|
0.614 |
|
0.648 |
Δrm(test)2 |
0.188 |
|
0.117 |
MAEtest |
0.589 |
0.524 |
0.563 |
RMSEp |
0.744 |
0.682 |
0.715 |
PLS-based QSPR model
|
Log BCF = 4.918 + 0.244 × (MLOGP) + 0.246 × (Cl-089) − 0.055 × (MaxdO) − 7.321 × (X1A) − 0.592 × (B03[C–N]) − 0.053 × (MaxsOH)
| (1) |
q-RASPR model development
After the development of the QSPR model, a q-RASPR model was developed using the same level of chemical informations to further improve the quality of the model. Here, we have combined the RASPR descriptors computed using the optimised hyperparameters obtained from the Gaussian kernel similarity approach (number of similar training compounds = 10, σ = 0.75) with the final PLS-based QSPR model's descriptors. With these combined descriptors, we got the subset of suitable descriptors with the help of the Best Subset Selection_v2.1 tool.29 The final q-RASPR model was developed employing the PLS regression method involving 5 descriptors, and as the descriptors are correlated, the optimal number of components based on Q2LOO is 2, i.e. with 2 latent variables. The descriptors which are employed for the development of the q-RASPR model include RA function (GK), Abs MaxPos–MaxNeg, Avg.Sim(GK), gm*SD similarity, and B03[C–N]. The equation (Eqn (2)) for the PLS-based q-RASPR model is given below.
PLS based q-RASPR model
|
Log BCF = 0.189 + 0.753 × RA function (GK) + 0.737 × Abs MaxPos–MaxNeg + 0.142 × Avg. Sim (GK) + 2.662 × gm*SD similarity − 0.035 × B03[C–N]
| (2) |
The PLS-based q-RASPR model was generated by strictly following the OECD guidelines and the statistical metrics of the model are reported in Table 1.
The statistical results (both internal and external validation metrics) of the model show that the model is robust, predictive, reliable, and reproducible. From the statistical metrics reported in Table 1, it is noteworthy that the PLS-based q-RASPR model is superior to the PLS-based QSPR model. A significant reduction in the value of MAE for both the training and test sets is observed in the RASPR model. Also, it is evident that, unlike the RASPR model, the QSPR model could not surpass the threshold value of CCC. In addition to the conventional validation methods, the Y-randomization test of the model has also been performed using SIMCA-P software (given in Fig. 3.), and the intercepts of R2Y and Q2Y (R2Y = −0.0148 and Q2Y = −0.0307) are less than the threshold limit which indicates that the model was not developed by chance correlation.
A scatter plot (Fig. 2) is developed using the Python matplotlib module representing the observed versus predicted log
BCF value of the training and test sets. The distribution of data points in both the training and test sets is balanced and uniform, which facilitates a fair evaluation of the model's performance. However, it is important to note that an equal distribution alone does not guarantee a good model fit. The model's effectiveness is also influenced by factors such as its complexity, potential overfitting or underfitting, and the quality of the features used. Despite these considerations, the overall performance metrics and validation results indicate that the model demonstrates a strong fit and performs well in predicting the endpoint.
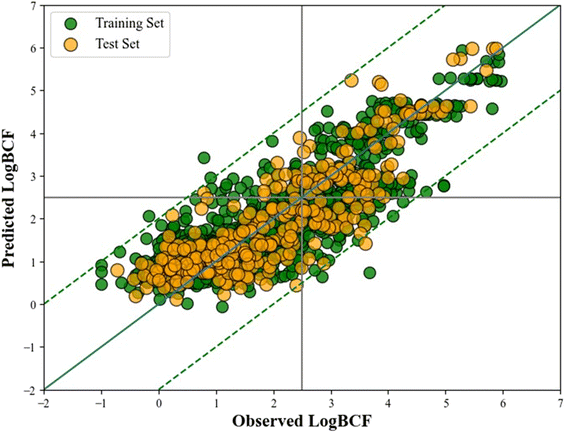 |
| Fig. 2 Scatter plot of the PLS-based q-RASPR model. | |
PLS plots
Various plots for the final PLS-based q-RASPR model have been developed using SIMCA-P software.40
Regression coefficient plot
This plot shows whether the model descriptors contribute positively or negatively to the endpoint (log
BCF). The regression coefficient plot was developed by plotting model descriptors against their respective regression coefficients on the Y-axis.50 Here, the regression coefficient plot (as given in Fig. S2 of ESI 2†) of the developed model indicates that RA function (GK), Abs MaxPos–MaxNeg, Avg.Sim (GK), and gm*SD similarity contribute positively while B03[C–N] contributes negatively to the response property (log
BCF).
Variable importance plot (VIP)
The VIP plot30 depicts the relative importance of the descriptors towards the property endpoint (log
BCF). Descriptors possessing VIP score >1 are considered the most vital towards the property endpoint (log
BCF). The relative order of importance of the modeled descriptors obtained from the VIP plot (depicted in Fig. 3) is as follows: RA function (GK) > gm*SD similarity > B03[C–N] > Abs MaxPos–MaxNeg > Avg.Sim (GK).
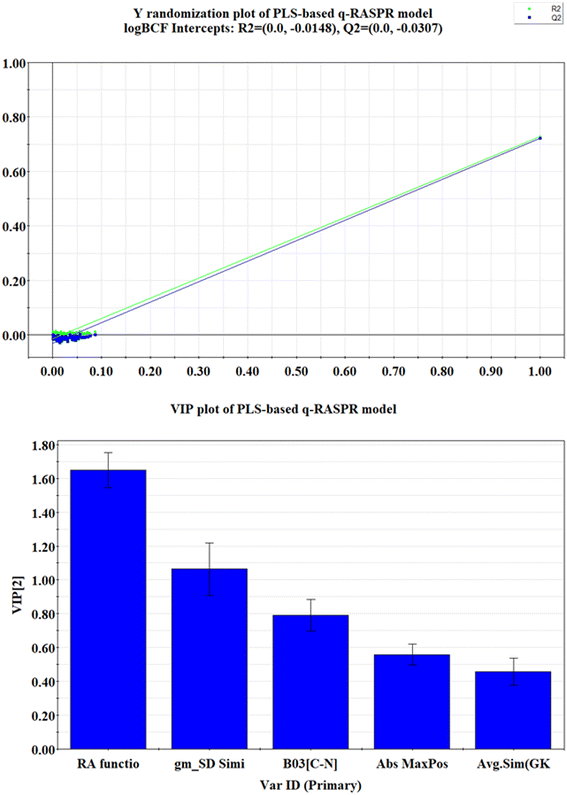 |
| Fig. 3 Y-randomization and VIP plots of the PLS-based q-RASPR model. | |
Loading plot
With the use of the first two latent variables, a loading plot has been developed which analyses the inter-relationship between the independent and dependent variables. Features with the maximum distance from the origin and located close to the endpoint are thought to have a higher influence on the response as well as the model.51 The loading plot (given in Fig. S3 of ESI 2†) of the final model suggests that the RA function and gm*SD similarity are more impactful towards the endpoint than the other modeled descriptors.
Score plot
The distribution of the compounds in the LV space of the first two components based on their scores is depicted in the score plot.52 The ellipse obtained in the graph represents the AD of the model, hence signifying that the compounds situated outside the ellipse are regarded as outliers. The graph obtained in the current study is given in Fig. S4 of ESI 2† and it indicates that compound numbers 13, 26, 85, 233, 270, 821, 867, 880, 897, 899, 910, 1084, 1095, 1176, and 1286 are the outliers for structural dissimilarity.
Analysis of the applicability domain of the PLS-based q-RASPR model
The analysis of the AD of the model was performed using the DModX (distance to model in X space) method with the help of SIMCA-P software (confidence level = 99% and D-critical value = 0.009999).37 The DModX plots of the training and test sets of the developed PLS-based q-RASPR model have been given in Fig. 4. From the DModX plot, it is observed that compound numbers 32, 143, 167, 480, 694, and 846 are outliers in the test set.
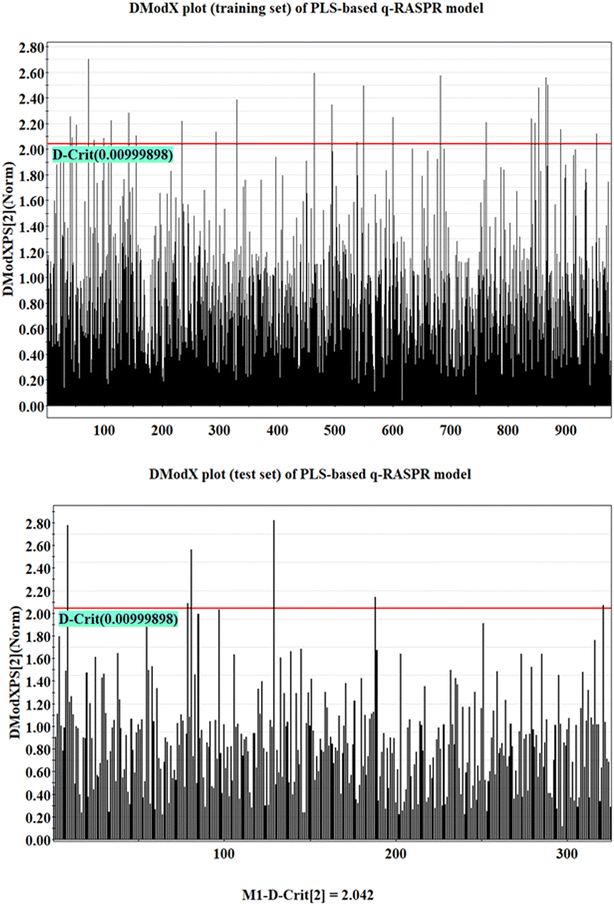 |
| Fig. 4 DModX plot of the training and test sets of the PLS-based q-RASPR model. | |
Insights into the modeled descriptors
The probable mechanistic interpretation of the developed models (as per OECD principle 5) has been attempted. From the above-mentioned PLS-based QSPR model, it is noteworthy that MLOGP and Cl-089 contribute positively and MaxdO, X1A, B03[C–N], and MaxsOH contribute negatively towards log
BCF. MLOGP (Moriguchi octanol–water partition coefficient (log
P)), is a descriptor of molecular properties, with the increase in the Moriguchi log
P (MLOGP) value enhancing lipophilicity, which ultimately makes the compound more bioaccumulative.53 Cl-089, an atom-centered fragment descriptor, refers to the hydrophobicity measures of a Cl atom attached to a sp2 hybridized carbon (C1) atom. Cl-089 showed positive contribution towards the log
BCF endpoint, which means that hydrophobicity makes the compound more bioaccumulative; conversely, a less/absence of this fragment decreases the bioaccumulation.54 MaxdO is the maximum atom type E-state of the fragment = O, signifying that compounds containing carbonyl groups, amides, or esters will decrease the value of log
BCF due to the formation of hydrogen bonds with water as it has a negative regression coefficient. X1A is the average connectivity index of order 1, and it describes the molecular branching and complexity.55 Negative contribution of this descriptor indicates that the log
BCF value will be decreased with the increase in molecular branching and complexity of the molecule. B03[C–N] indicates the presence or absence of carbon and nitrogen atoms at topological distance 3. Negative contribution of this descriptor suggested that an increase in the number of this fragment lowers the bioaccumulative potential of the compound. The nitrogen atom which is an electron donor readily forms hydrogen bonding with water, hence suggesting a lower bioaccumulative potential of the compound. MaxsOH is the E-state atom type descriptor signifying the intrinsic electronic properties of OH groups in different structures. The presence of OH groups enhances the polarity of the compound, making it hydrophilic and hindering cell membrane uptake, consequently decreasing the bioaccumulative tendency.4
From the PLS-based q-RASPR model, it is noteworthy that the RA function (GK), the read-across derived descriptor, obtained by using the Gaussian kernel-based similarity algorithm, which includes all the information of the QSPR model descriptors and shows positive contribution towards the endpoint. Thus, it suggests that with the increase in the numerical value of this RASPR descriptor, the log
BCF value increases as depicted for compound 1124 and vice versa for compound 155 (represented in Fig. 5). The RASPR descriptor Abs MaxPos–MaxNeg indicates the absolute difference between the values of MaxPos and MaxNeg among close source compounds. The high absolute difference between the values of MaxPos (maximum similarity of the positive close source compound) and MaxNeg (maximum similarity of the negative close source compound),10 enhance the bioaccumulative potential of the compound, which can be exemplified by compound 1121 and vice versa in the case of compound 530 (demonstrated in Fig. 5). The descriptor Avg.Sim (GK), a RASPR descriptor, represents the average degree of similarity between the close source compounds and contributes positively towards the log
BCF value. This suggests that with an increase in the value of this descriptor, the value of the response (log
BCF) also increases as demonstrated in compound 916, while the opposite occurs in compound 428 (provided in Fig. 5). The descriptor gm*SD similarity, a RASPR descriptor, is the product of the concordance measure gm (Banerjee–Roy coefficient) and SD similarity (weighted standard deviation of the response values of the close source compounds for a particular query compound). It has a positive contribution towards the log
BCF value, i.e., with an increase in the numerical value of this descriptor, the log
BCF value will also increase as observed in compound 94, while the opposite occurs in compound 769 (given in Fig. 5). B03[C–N] is a 2D atom pair descriptor that indicates the presence or absence of C and N atoms at topological distance 3 and contributes negatively towards the property endpoint (log
BCF). Generally, nitrogen atoms act as an electron donors which readily form hydrogen bonds with water and are excreted out from the body of the reference organism, ultimately leading to a decrease in the bioaccumulative potential of the compound as demonstrated by compound 29 while the opposite occurs in the case of compound 899 (given in Fig. 5).
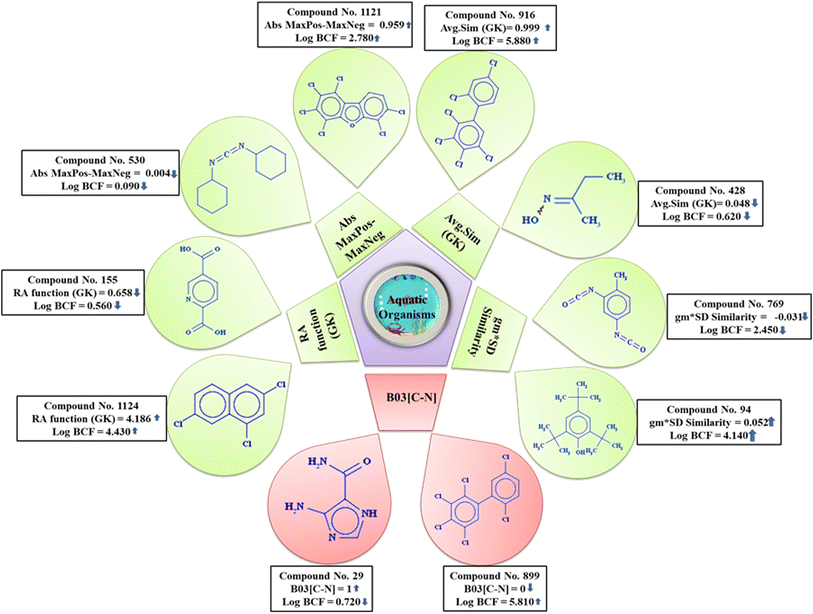 |
| Fig. 5 Mechanistic introspection of the modeled descriptors of the PLS-based q-RASPR model. | |
Screening of the external datasets
The final PLS-based q-RASPR model was used to screen the PPDB database (1694 pesticides) with the help of PRI software.29 AD assessment of the PPDB database was performed and it was found that 1645 compounds (97.11%) out of the 1694 compounds of the PPDB were present inside the AD, hence suggesting reliable estimation (prediction of the log
BCF value for 1694 compounds of the PPDB database is provided in ESI 1†). It was observed that the majority of compounds (99.88%) show “good” prediction quality. We have provided a list of 20 compounds with the highest log
BCF value and 20 compounds with the lowest log
BCF value in Table S2 of ESI 2.† The prediction for the 20 compounds with the highest log
BCF value suggests that the first 14 compounds are very bioaccumulative while the rest are moderately bioaccumulative. While the prediction for the 20 compounds with the lowest log
BCF value suggests that all of them are non-bioaccumulative. Moreover, the bioaccumulative potential of compounds (20 compounds with the highest log
BCF value and 20 compounds with the lowest log
BCF value) were corroborated with real-world data obtained from the PPDB,39 PubChem56 and other sources (reference for the same is given in Table S2 of ESI 2†) which suggests that the final PLS-based q-RASPR model is capable of making accurate predictions and hence can be used for prediction of the log
BCF value of new and untested chemicals. A graphical representation is provided in Fig. S5 of ESI 2† based on the prediction for all the PPDB compounds (1645) and it depicts that 1621 compounds (98.54%) are non-bioaccumulative, 10 compounds (0.61%) are bioaccumulative and 14 compounds (0.85%) fall under the category of very bioaccumulative chemicals. Also the validation metrics obtained after screening the external test set taken from previously published literature13 are reported: Q2F1 = 0.805, Q2F2 = 0.771,
Δrm(test)2 = 0.124, CCC = 0.877, and MAEtest = 0.588. From these obtained validation metrics, it is noteworthy that our model outperforms the results reported in previous studies. Also the standard deviation of the experimental log
BCF value compared to the predicted log
BCF value is less than 0.1.
Comparison of the developed PLS-based q-RASPR model with previous work
It is not possible to perform a strict comparison of the results obtained in this study with those from previously reported related studies due to the different modeling algorithms employed, various validation parameters reported, and the different arrangements of the training and test sets employed. Previously, Lunghini et al.13 reported the QSPR model for BCF prediction employing different machine-learning algorithms including random forest and support vector regression. ISIDA (in silico design and data analysis) fragment descriptors were used in this work for model development and their statistical metrics are provided in Table 2. In another similar study, Bertato et al.2 utilized the raw data (as used by Lunghini et al.)13 for the development of linear (MLR-OLS) and non-linear (ANN) models, and their statistical metrics are provided in Table 2.
Table 2 Comparison of the current study with previous literaturea
Previous work |
Type of model |
Train/test |
Number of descriptors |
Internal validation metrics |
External validation metrics |
R
2
|
Q
F2
2
|
MAE |
CCC |
RMSEp |
MLR-OLS: multiple linear regression, ANN: artificial neural network.
|
Lunghini et al.13 |
ISIDA consensus |
1095/204 |
— |
— |
— |
— |
— |
0.770 |
Bertato et al.2 |
MLR-OLS |
920/459 |
6 |
0.620 |
— |
— |
— |
0.780 |
ANN |
920/459 |
6 |
0.700 |
— |
— |
— |
0.760 |
Present work
|
PLS q-RASPR
|
978/325
|
5 (2 LVs)
|
0.727
|
0.739
|
0.563
|
0.858
|
0.715
|
In the current study, a PLS-based q-RASPR model has been developed to predict the log
BCF values of various chemicals using a dataset of 1303 diverse compounds. Developed models were stringently validated employing various globally accepted internal and external validation parameters. We have also reported some additional metrics (such as MAE and CCC) for external validation, which indicates the reliability and predictability of the established model. Furthermore, the calculated RMSEp metrics of the established model are lower as compared to previous studies, indicating that the established model is more accurate. The model was developed using a diverse and large set of chemicals, so it has a wide domain of applicability. The final PLS-based q-RASPR model was obtained with a relatively lower number of variables (5 descriptors with only 2 LVs) compared to the previous studies. The developed model was used for screening and prioritization of the PPDB database (real-world data), showing that the model is reliable and accurate and has a wide domain of applicability, and good prediction ability. A comparison of the statistical metrics from the current study with those from previously reported similar work has been given in Table 2.
Rationale and novelty of the present study
The rationale for this study lies in the pressing need to develop alternative methods for predicting the Bioconcentration Factor (BCF) of chemical substances, a crucial parameter for assessing their bioaccumulative and ecotoxicological potential. Traditional in vivo BCF testing methods are not only ethically challenging due to the use of animals but are also time-consuming and costly. This study aims to address these limitations by integrating the QSPR model with a read-across (RA) algorithm to create a novel q-RASPR model.
The novelty of this study is underscored by the use of structurally diverse datasets combined with simple, interpretable, and reproducible 2D molecular descriptors alongside RASAR descriptors. The key contribution of our study lies in the integration of read-across techniques with the quantitative structure–property relationship (QSPR) approach, resulting in the q-RASPR model. The RASPR descriptors provide an added layer of precision, making our model not just another QSAR model, but one that enhances the predictive reliability of BCF estimations across a wide chemical space. Ultimately, the value of our model is demonstrated through its capability to accurately predict the bioaccumulation potential of chemicals, as validated through the screening of the Pesticide Properties Database (PPDB). This makes our model not only relevant but also essential for industries and regulators needing reliable in silico methods for assessing ecotoxicological risks. Regarding chemical diversity, previous models11,12,57 often focused on specific chemical classes, such as non-ionic organic compounds or organophosphates with a lower number of data points. Our study, however, leverages a dataset of 1333 structurally diverse chemicals, capturing a broader range of functional groups, molecular sizes, and physicochemical properties. This diversity is not only demonstrated by the number of substances but also through the extensive representation of the chemical space, as well as the inclusion of substructures and functional groups relevant to bioaccumulation.
The accuracy of predictions depends heavily on the quality and comprehensiveness of the data. We have ensured that our dataset is robust and representative of the chemical properties being studied. Although read-across assumes that structurally similar compounds will have similar properties, our model incorporates a diverse range of descriptors to account for subtle structural differences. The predictions of our developed model are validated against known biological data to ensure that the assumed mechanisms are representative of the compounds' true activities. We have employed rigorous validation methods to manage model uncertainty, demonstrating that our approach provides reliable and accurate predictions despite these challenges.
Conclusion
Here, a PLS-based q-RASPR model has been developed using a diverse and large set of compounds strictly obeying the OECD principles. The developed model has been rigorously validated with globally accepted validation metrics, which indicates that the model is robust, efficient, reliable, reproducible, and interpretable. The PLS-based q-RASPR model is superior to the corresponding PLS-based QSPR model with respect to both the internal and external validation parameters. From the developed PLS-based QSPR and q-RASPR models, it was observed that lipophilicity, hydrophobicity and a high value of Abs MaxPos–MaxNeg, as well as Avg.Sim (GK) are responsible for the bioaccumulative tendency of chemicals. On the other hand, the presence of molecular branching, complexity, carbonyl groups, amides, esters and hydrophilic groups decreased the log
BCF value. The developed model can contribute significantly to the screening of various industrial chemicals and can be employed for the estimation of their log
BCF values. The PLS-based q-RASPR model was employed to screen the PPDB database to check the reliability of the model and its predictive potential was validated with real-world experimental data. Thus, this model can be employed to fill the data gaps for compounds whose BCF values have not been experimentally reported, hence addressing all the challenges associated with animal experimentation, time, labor, and expenses. Hence, the proposed model can be applied for predicting the ecotoxicological risk of various chemicals on the basis of bioaccumulative potential assessment for aquatic organisms as well as for designing safer, greener and novel (less bio-accumulative) chemicals, strictly adhering to the RRR principles (reduction, refinement and replacement).
Future research directions
• Future research could explore the integration of the developed PLS-based q-RASPR model with advanced machine learning techniques to develop hybrid models that offer improved robustness and predictive accuracy.
• Quantifying the uncertainty associated with q-RASPR predictions will be crucial for enhancing model reliability and supporting more informed decision-making processes. The application of the q-RASPR model could be expanded to predict additional environmental fate and effects parameters, such as bioaccumulation factors in terrestrial organisms.
• Creating software or web-based platforms to facilitate the practical use of the q-RASPR model by regulatory agencies and industry professionals would be beneficial.
• Future studies could investigate the integration of q-RASPR predictions into existing risk assessment frameworks to enhance their effectiveness and comprehensiveness.
These directions aim to enhance the development and applicability of the developed PLS-based q-RASPR model in various contexts and ensure its utility in broader research and regulatory scenarios.
Data availability
Additional data have been made available in the ESI.†
Conflicts of interest
The authors declare that they have no competing interests.
Acknowledgements
SD gratefully acknowledges financial assistance from the AICTE, New Delhi, in the form of a scholarship. AK thanks the GPC Regulatory India Private Limited for financial support in the form of a project (GPC Regulatory India Private Limited sponsored research, Ref No-P-1/RS/171/22, date-07.09.2022). PKO is thankful to the DTC lab and Prof. Kunal Roy for providing technical assistance and guidance.
References
- G. Piir, S. Sild, A. Roncaglioni, E. Benfenati and U. Maran, QSAR model for the prediction of bio-concentration factor using aqueous solubility and descriptors considering various electronic effects, SAR QSAR Environ. Res., 2010, 21(7–8), 711–729, DOI:10.1080/1062936X.2010.528596.
- L. Bertato, N. Chirico and E. Papa, Predicting the bioconcentration factor in fish from molecular structures, Toxics, 2022, 10(10), 581, DOI:10.3390/toxics10100581.
- S. El-Amrani, M. Pena-Abaurrea, J. Sanz-Landaluze, L. Ramos, J. Guinea and C. Camara, Bioconcentration of pesticides in zebrafish eleutheroembryos (Danio rerio), Sci. Total Environ., 2012, 425, 184–190, DOI:10.1016/j.scitotenv.2012.02.065.
- K. Khan, V. Kumar, E. Colombo, A. Lombardo, E. Benfenati and K. Roy, Intelligent consensus predictions of bioconcentration factor of pharmaceuticals using 2D and fragment-based descriptors, Environ. Int., 2022, 170, 107625, DOI:10.1016/j.envint.2022.107625.
-
EPA U, Estimation Programs Interface Suite™ for Microsoft® Windows, V 4.11, United States Environmental Protection Agency, Washington, DC, USA, 2012 Search PubMed.
-
E. Benfenati, A. Manganaro and G. C. Gini, VEGA-QSAR: AI Inside a Platform for Predictive Toxicology, 2013, vol. 1107, pp. 21–28 Search PubMed.
- K. Mansouri, C. M. Grulke, R. S. Judson and A. J. Williams, OPERA models for predicting physicochemical properties and environmental fate endpoints, J. Cheminf., 2018, 10, 1–9, DOI:10.1186/s13321-018-0263-1.
- V. Ruusmann, S. Sild and U. Maran, QSAR DataBank repository: open and linked qualitative and quantitative structure–activity relationship models, J. Cheminf., 2015, 7, 1, DOI:10.1186/s13321-015-0082-6.
- T. Luechtefeld, D. Marsh, C. Rowlands and T. Hartung, Machine learning of toxicological big data enables read-across structure activity relationships (RASAR) outperforming animal test reproducibility, Toxicol. Sci., 2018, 165(1), 198–212, DOI:10.1093/toxsci/kfy152.
- A. Banerjee and K. Roy, First report of q-RASAR modeling toward an approach of easy interpretability and efficient transferability, Mol. Diversity, 2022, 26(5), 2847–2862, DOI:10.1007/s11030-022-10478-6.
- P. Gramatica and E. Papa, An update of the BCF QSAR model based on theoretical molecular descriptors, QSAR Comb. Sci., 2005, 24(8), 953–960, DOI:10.1002/qsar.200530123.
- P. Banjare, B. Matore, J. Singh and P. P. Roy,
In silico local QSAR modeling of bioconcentration factor of organophosphate pesticides, In Silico Pharmacology, 2021, 9(1), 28, DOI:10.1007/s40203-021-00087-w.
- F. Lunghini, G. Marcou, P. Azam, R. Patoux, M. H. Enrici, F. Bonachera, D. Horvath and A. Varnek, QSPR models for bioconcentration factor (BCF): are they able to predict data of industrial interest?, SAR QSAR Environ. Res., 2019, 30(7), 507–524, DOI:10.1080/1062936X.2019.1626278.
- P. Ambure, A. K. Halder, H. Gonzalez Diaz and M. N. Cordeiro, QSAR-Co: an open source software for developing robust multitasking or multitarget classification-based QSAR models, J. Chem. Inf. Model., 2019, 59(6), 2538–2544 CrossRef CAS PubMed.
- A. K. Halder and M. N. Dias Soeiro Cordeiro, QSAR-Co-X: an open source toolkit for multitarget QSAR modelling, J. Cheminf., 2021, 13, 1–8, DOI:10.1186/s13321-021-00508-0.
- F. Grisoni, V. Consonni and M. Vighi, Detecting the bioaccumulation patterns of chemicals through data-driven approaches, Chemosphere, 2018, 208, 273–284, DOI:10.1016/j.chemosphere.2018.05.157.
- H. Ai, X. Wu, L. Zhang, M. Qi, Y. Zhao, Q. Zhao, J. Zhao and H. Liu, QSAR modelling study of the bioconcentration factor and toxicity of organic compounds to aquatic organisms using machine learning and ensemble methods, Ecotoxicol. Environ. Saf., 2019, 179, 71–78, DOI:10.1016/j.ecoenv.2019.04.035.
- X. Li, G. Liu, Z. Wang, L. Zhang, H. Liu and H. Ai, Ensemble multiclassification model for aquatic toxicity of organic compounds, Aquat. Toxicol., 2023, 255, 106379, DOI:10.1016/j.aquatox.2022.106379.
- A. K. Halder, A. S. Moura and M. N. Cordeiro, Moving average-based multitasking in silico classification modeling: where do we stand and what is next?, Int. J. Mol. Sci., 2022, 23(9), 4937, DOI:10.3390/ijms23094937.
- L. Yang, P. Chen, K. He, R. Wang, G. Chen, G. Shan and L. Zhu, Predicting bioconcentration factor and estrogen receptor bioactivity of bisphenol a and its analogues in adult zebrafish by directed message passing neural networks, Environ. Int., 2022, 169, 107536, DOI:10.1016/j.envint.2022.107536.
- J. Y. Xu, K. Wang, S. H. Men, Y. Yang, Q. Zhou and Z. G. Yan, QSAR-QSIIR-based prediction of bioconcentration factor using machine learning and preliminary application, Environ. Int., 2023, 177, 108003, DOI:10.1016/j.envint.2023.108003.
- A. P. Toropova, A. A. Toropov, S. E. Martyanov, E. Benfenati, G. Gini, D. Leszczynska and J. Leszczynski, CORAL: Monte Carlo method as a tool for the prediction of the bioconcentration factor of industrial pollutants, Mol. Inf., 2013, 32(2), 145–154, DOI:10.1002/minf.201200069.
- A. P. Toropova, P. R. Duchowicz, L. M. Saavedra, E. A. Castro and A. A. Toropov, The use of the index of ideality of correlation to build up models for bioconcentration factor, Mol. Inf., 2020, 39(7), 1900070, DOI:10.1002/minf.201900070.
- G. Gómez-Jiménez, K. Gonzalez-Ponce, D. J. Castillo-Pazos, A. Madariaga-Mazon, J. Barroso-Flores, F. Cortes-Guzman and K. Martinez-Mayorga, The OECD principles for (Q) SAR models in the context of knowledge discovery in databases (KDD), Adv. Protein Chem. Struct. Biol., 2018, 113, 85–117, DOI:10.1016/bs.apcsb.2018.04.001.
-
A. Mauri, alvaDesc: A Tool to Calculate and Analyze Molecular Descriptors and Fingerprints, in Ecotoxicological QSARs, 2020, pp. 801–820, DOI:10.1007/978-1-0716-0150-1_32.
- P. Ambure, R. B. Aher, A. Gajewicz, T. Puzyn and K. Roy, “NanoBRIDGES” software: open access tools to perform QSAR and nano-QSAR modeling, Chemom. Intell. Lab. Syst., 2015, 147, 1–3, DOI:10.1016/j.chemolab.2015.07.007.
- M. P. Gonzalez, C. Teran, L. Saiz-Urra and M. Teijeira, Variable selection methods in QSAR: an overview, Curr. Top. Med. Chem, 2008, 8(18), 1606–1627, DOI:10.2174/156802608786786552.
- M. Goodarzi, B. Dejaegher and Y. V. Heyden, Feature selection methods in QSAR studies, J. AOAC Int., 2012, 95(3), 636–651, DOI:10.5740/jaoacint.SGE_Goodarzi.
-
https://www.teqip.jdvu.ac.in/QSAR_Tools/DTCLab
.
- S. Wold, M. Sjöström and L. Eriksson, PLS-regression: a basic tool of chemometrics, Chemom. Intell. Lab. Syst., 2001, 58(2), 109–130, DOI:10.1016/S0169-7439(01)00155-1.
- B. C. Deng, Y. H. Yun, Y. Z. Liang, D. S. Cao, Q. S. Xu, L. Z. Yi and X. Huang, A new strategy to prevent over-fitting in partial least squares models based on model population analysis, Anal. Chim. Acta, 2015, 880, 32–41, DOI:10.1016/j.aca.2015.04.045.
- G. Patlewicz, N. Ball, E. D. Booth, E. Hulzebos, E. Zvinavashe and C. Hennes, Use of category approaches, read-across and (Q) SAR: general considerations, Regul. Toxicol. Pharmacol., 2013, 67(1), 1–2, DOI:10.1016/j.yrtph.2013.06.002.
- A. Gajewicz, How to judge whether QSAR/read-across predictions can be trusted: a novel approach for establishing a model's applicability domain, Environ. Sci.: Nano, 2018, 5(2), 408–421, 10.1039/C7EN00774D.
- M. Chatterjee, A. Banerjee, P. De, A. Gajewicz-Skretna and K. Roy, A novel quantitative read-across tool designed purposefully to fill the existing gaps in nanosafety data, Environ. Sci.: Nano, 2022, 9(1), 189–203, 10.1039/D1EN00725D.
-
https://www.sites.google.com/jadavpuruniversity.in/dtc-lab-software/home
.
- S. Das, A. Samal and P. K. Ojha, Chemometrics-driven prediction and prioritization of diverse pesticides on chickens for addressing hazardous effects on public health, J. Hazard. Mater., 2024, 471, 134326, DOI:10.1016/j.jhazmat.2024.134326.
- A. Banerjee and K. Roy, How to correctly develop q-RASAR models for predictive cheminformatics, Expert Opin. Drug Discovery, 2024, 19(9), 1017–1022, DOI:10.1080/17460441.2024.2376651.
- J. Fukuchi, A. Kitazawa, K. Hirabayashi and M. Honma, A practice of expert review by read-across using QSAR Toolbox, Mutagenesis, 2019, 34(1), 49–54, DOI:10.1093/mutage/gey046.
- A. Gallagher and S. Kar, Unveiling first report on in silico modeling of aquatic toxicity of organic chemicals to Labeo rohita (Rohu) employing QSAR and q-RASAR, Chemosphere, 2024, 349, 140810, DOI:10.1016/j.chemosphere.2023.140810.
- S. Yang and S. Kar, First report on chemometric modeling of tilapia fish aquatic toxicity to organic chemicals: toxicity data gap filling, Sci. Total Environ., 2024, 907, 167991, DOI:10.1016/j.scitotenv.2023.167991.
-
Z. Wu, D. Li, J. Meng and H. Wang, Introduction to SIMCA-P and its application, in Handbook of Partial Least Squares: Concepts, Methods and Applications, 2010, pp. 757–774, DOI:10.1007/978-3-540-32827-8_33.
- D. Gadaleta, G. F. Mangiatordi, M. Catto, A. Carotti and O. Nicolotti, Applicability domain for QSAR models: where theory meets reality, Int. J. Quant. Struct.-Prop. Relat., 2016, 1(1), 45–63, DOI:10.4018/IJQSPR.2016010102.
- C. Rücker, G. Rücker and M. Meringer, y-Randomization and its variants in QSPR/QSAR, J. Chem. Inf. Model., 2007, 47(6), 2345–2357, DOI:10.1021/ci700157b.
-
https://www.sitem.herts.ac.uk/aeru/ppdb/
.
- P. De, S. Kar, P. Ambure and K. Roy, Prediction reliability of QSAR models: an overview of various validation tools, Arch. Toxicol., 2022, 96(5), 1279–1295, DOI:10.1007/s00204-022-03252-y.
- S. Begum, P. Jaswanthi, B. V. Lakshmi and K. Bharathi, QSAR studies on indole-azole analogues using DTC tools; imidazole ring is more favorable for aromatase inhibition, J. Indian Chem. Soc., 2021, 98(1), 100016, DOI:10.1016/j.jics.2021.100016.
- Z. Liu, J. Gao, C. Li, L. Xu, X. Lv, H. Deng, Y. Gao, H. Wang, H. Li and Z. Wang, Application of QSAR models for acute toxicity of tetrazole compounds administrated orally and intraperitoneally in rat and mouse, Toxicology, 2023, 500, 153679, DOI:10.1016/j.tox.2023.153679.
- F. A. Ikwu, G. A. Shallangwa and P. A. Mamza, QSAR, QSTR, and molecular docking studies of the anti-proliferative activity of phenylpiperazine derivatives against DU145 prostate cancer cell lines, Beni-Suef University Journal of Basic and Applied Sciences, 2020, 9, 1–2, DOI:10.1186/s43088-020-00054-y.
- F. A. Ugbe, G. A. Shallangwa and I. A. Adamu Uzairu, Combined QSAR modeling, molecular docking screening, and pharmacokinetics analyses for the design of novel 2, 6-diarylidene cyclohexanone analogs as potent anti-leishmanial agents, Prog. Chem. Biochem. Res., 2023, 6(1), 11–30, DOI:10.22034/pcbr.2022.366493.1234.
- F. Davrieux, D. Dufour, P. Dardenne, J. Belalcazar, M. Pizarro, J. Luna, L. Londoño, A. Jaramillo, T. Sánchez, N. Morante and F. Calle, LOCAL regression algorithm improves near infrared spectroscopy predictions when the target constituent evolves in breeding populations, J. Near Infrared Spectrosc., 2016, 24(2), 109–117, DOI:10.1255/jnirs.1213.
- C. Yoo and M. Shahlaei, The applications of PCA in QSAR studies: a case study on CCR5 antagonists, Chem. Biol. Drug Des., 2018, 91(1), 137–152, DOI:10.1111/cbdd.13064.
-
H. Hotelling, The Generalization of Student’s Ratio, in Breakthroughs in Statistics, Springer Series in Statistics, ed. S. Kotz, N. L. JohnsonSpringer, New York, NY, 1992, DOI:10.1007/978-1-4612-0919-5_4.
- M. Zapadka, M. Kaczmarek, B. Kupcewicz, P. Dekowski, A. Walkowiak, A. Kokotkiewicz, M. Łuczkiewicz and A. Buciński, An application of QSRR approach and multiple linear regression method for lipophilicity assessment of flavonoids, J. Pharm. Biomed. Anal., 2019, 164, 681–689, DOI:10.1016/j.jpba.2018.11.024.
- S. Kar, O. Deeb and K. Roy, Development of classification and regression based QSAR models to predict rodent carcinogenic potency using oral slope factor, Ecotoxicol. Environ. Saf., 2012, 82, 85–95, DOI:10.1016/j.ecoenv.2012.05.013.
- R. Put, Q. S. Xu, D. L. Massart and Y. Vander Heyden, Multivariate adaptive regression splines (MARS) in chromatographic quantitative structure–retention relationship studies, J. Chromatogr. A, 2004, 1055(1–2), 11–19, DOI:10.1016/j.chroma.2004.07.112.
-
https://www.pubchem.ncbi.nlm.nih.gov/
.
- S. Pore, A. Pelloux, M. Chatterjee, A. Banerjee and K. Roy, Machine learning-based q-RASAR predictions of the bioconcentration factor of organic molecules estimated following the organisation for economic co-operation and development guideline 305, J. Hazard. Mater., 2024, 479, 135725, DOI:10.1016/j.jhazmat.2024.135725.
|
This journal is © The Royal Society of Chemistry 2025 |
Click here to see how this site uses Cookies. View our privacy policy here.