DOI:
10.1039/D1RA05994G
(Paper)
RSC Adv., 2021,
11, 34788-34794
Investigation of the individual human sulfatome in plasma and urine samples reveals an age-dependency†
Received
7th August 2021
, Accepted 12th October 2021
First published on 28th October 2021
Abstract
Metabolic microbiome interaction with the human host has been linked to human physiology and disease development. The elucidation of this interspecies metabolite exchange will lead to identification of beneficial metabolites and disease modulators. Their discovery and quantitative analysis requires the development of specific tools and analysis of specific compound classes. Sulfated metabolites are considered a readout for the co-metabolism of the microbiome and their host. This compound class is part of the human phase II clearance process of xenobiotics and is the main focus in drug or doping metabolism and also includes dietary components and microbiome-derived compounds. Here, we report the targeted analysis of sulfated metabolites in plasma and urine samples in the same individuals to identify the core sulfatome and similarities between these two sample types. This analysis of 27 individuals led to the identification of the core sulfatome of 41 metabolites in plasma and urine samples as well as an age effect for 15 metabolites in both sample types.
Introduction
The metabolism of xenobiotics has evolved to efficiently remove non-endogenous hydrophobic compounds from the human body.1 The xenobiotic clearance process in humans involves different enzymatic processes that are classified as phase I and phase II metabolic conversions.2,3 Phase I modification enzymes include oxidases such as cytochrome P450 (CYP450) and phase II modifying enzymes add hydrophilic modifications, e.g. glucuronides, sulfates or amino acids to oxidized compounds.1 These converted metabolites are then cleared from the human body mostly via excretion through urine.4,5 The main research interest of phase II modifications has been for the metabolism of drugs, nutritional components, toxins, and doping agents.6–10 Phase II modifications have recently also been linked to the co-metabolism of the gut microbiome with their human host. Mainly sulfation but also glucuronidation are important modifications for metabolites derived from the co-metabolism of the microbiome and its host.11–13
In the past decades, most studies have investigated the compound class of sulfated metabolites in targeted analyses of selected metabolites as the investigation of a broad range of these compounds has been challenging.14,15 We have recently developed methodologies for the analysis of diverse structures of sulfated metabolites using enzymatic sample pretreatment for investigation of urine samples and enzymatic treatment to specifically identify metabolites with a difference in m/z of 79.9568 Da.11 Our arylsulfatase-based method has been developed to identify sulfated aromatic metabolites in different studies, which led to identification of more than 230 sulfated metabolites with structures validated at different confidence levels.16,17 Determination of the metabolite structure (metabolite ID) at level 1 requires a synthetic standard for UPLC-MS/MS co-injection experiments, level 2 validation matches the mass spectrometric fragmentation pattern of a metabolite with a fragmentation pattern in databases, metabolites at level 3 identified a potential metabolite structure that contains a sulfate ester and solely the presence of a sulfate ester was classified at level 4. We have recently developed a targeted sulfated metabolome (sulfatome) analysis for urine samples of a dietary intervention study, for which we have generated a reference library of 252 sulfated metabolites including more than 70 synthetic sulfates.18 This library can now be used for quick and efficient identification of sulfated metabolites in other sample cohorts as well as any sample matrix.
In the present study, we have utilized this sulfate library for investigation of plasma and urine samples collected from the same individuals. This analysis provides the sulfatome composition of plasma samples from 27 individuals for the first time. We have determined the metabolite profiles for the core sulfatome at an individual level in both matrices and identified an age effect for a series of sulfated metabolites in this cohort. Based on this analysis and our in-house library of sulfated metabolites, we provide new correlations of the phase II metabolism of xenobiotics for urine and plasma samples as well as new insights in the co-metabolism of the microbiome with its host.
Experimental section
Reagents and chemicals
All reagents and solvents were purchased from Sigma-Aldrich or Fischer Scientific and were used without further purification. Mass spectrometry grade solvents were used for UHPLC-ESI-MS analysis. Solutions were concentrated in vacuo on a Speedvac Concentrator Plus System (Eppendorf, Hamburg, Germany). High-resolution mass spectra were acquired on a SYNAPT G2-S High-Definition Mass Spectrometry (HDMS) using an electrospray ionization (ESI) source with an ACQUITY UPLC I-class system and equipped with a Waters ACQUITY UPLC® HSS T3 column (2.1 μm, 100 × 1.8 mm).
Sample preparation
An internal standard (10 μl; 13C9-tyrosine – 10 μg ml−1) was added to each plasma and urine samples aliquot (50 μl) for normalization. LC-MS grade methanol (240 μl) was added and the mixture was vortexed before being cooled to −20 °C for 1 h. The mixture was then centrifuged (5 min, 18
620 g, 4 °C) and the supernatant isolated. The solvents were removed under reduced pressure, and the residue re-dissolved in a solution of acetonitrile in water (50 μl, 5% MeCN/H2O) and transferred to LC-MS vials prior to mass spectrometric analysis.
UPLC-MS analysis
Mass spectrometric analysis was performed on an Acquity UPLC system connected to a Synapt G2 Q-TOF mass spectrometer, both from Waters Corporation (Milford, MA, USA). The system was controlled using the MassLynx software package v 4.1, also from Waters. The separation was performed on an Acquity UPLC® HSS T3 column (1.8 μm, 100 × 2.1 mm) from Waters Corporation. The mobile phase consisted of (A) 0.1% formic acid in Milli-Q water and (B) 0.1% formic acid in LC-MS-grade methanol. The column temperature was 40 °C with the gradient: 0–2 min, 0% B; 2–15 min, 0–100% B; 15–16 min, 100% B; 16–17 min, 100–0% B; 17–21 min, 0% B, with a flow rate of 0.2 mL min−1. The samples were introduced into the q-TOF using negative electrospray ionization. The capillary voltage was set to −2.50 kV and the cone voltage was 40 V. The source temperature was 100 °C, the cone gas flow 50 L min−1 and the desolvation gas flow 600 L h−1. The instrument was operated in MSE mode, the scan range was m/z = 50–1200, and the scan time was 0.3 s. In low energy mode, the collision energy was 10 V and in high energy mode the collision energy was increased from 25 to 45 V. A solution of sodium format (0.5 mM in 2-propanol
:
water, 90
:
10, v/v) was used to calibrate the instrument and a solution of leucine–encephalin (2 ng μL−1 in acetonitrile: 0.1% formic acid in water, 50
:
50, v/v) was used for the lock mass correction at an injection rate of 30 s.
Data analysis
Step 1. Data analysis was performed using the XCMS metabolomics software package under R (version 1.4.414). The results were processed in Excel 2016 and potential sulfate esters were identified using the following criteria compared with our previously compiled sulfated metabolite library of level 1 and level 2 validated metabolites:11,16,18,19 (i) mass error <20 ppm and (ii) retention time difference <0.1 min. The sulfate esters were confirmed by MS/MS fragmentation experiments. In low energy mode, the collision energy was 10 V and in high energy mode the collision energy was ramped from 30 to 40 V.
Step 2. Data analysis was performed using the XCMS metabolomics software package under R (version 1.1.414).20 A list of mass spectrometric features was obtained with m/z, retention time and peak area information.
Ethical approval
Plasma and urine samples were obtained from high-risk pancreatic cancer patients in accordance with the World Medical Association Declaration of Helsinki and all patients gave written informed consent. Approval for the study was obtained from the ethical committee at Karolinska University Hospital (ethical approval number: Dnr2017/290-31). Plasma and urine samples were collected using routine clinical collection protocols and all patient codes have been removed in this publication. All samples were stored at −80 °C.
Results and discussion
Sulfated metabolite identification
We sought to investigate the individual sulfatome composition in plasma and urine samples using our comprehensive compound library of 252 sulfated metabolites (Table S1†). This library is mainly composed of sulfated phenolic and other aromatic compounds as two different arylsulfatases were used for their discovery in human samples.16 To obtain an overview of the sulfate distribution, we have collected plasma and urine samples from 27 individuals. The distribution of the sample metadata including age and biological sex is detailed in Table 1. Both sample types were extracted separately according to standard protocols and analyzed by ultra-performance liquid chromatography coupled with tandem mass spectrometry (UPLC-MS/MS, Fig. 1). The collected mass spectrometric data was analyzed with the XCMS framework in R for peak picking, alignment and integration.20,21 Metabolite ID was initially performed by comparison of our in-house library of sulfated metabolites with the data output from the XCMS analysis. The criteria for metabolite identification were set to (i) mass error <20 ppm and (ii) retention time <0.1 min. Due to the different matrix effects in human samples, chemical structures for hits that did not fit the retention time requirements were additionally validated via mass spectrometric fragmentation experiments.
Table 1 Information of investigated human samples
|
Group I |
Group II |
Group III |
|
Age |
38–49 |
50–59 |
60–75 |
Total |
Participants |
7 |
10 |
10 |
27 |
(%) |
25.93 |
37.04 |
37.04 |
|
Average |
43.5 |
53.2 |
67.4 |
|
Standard deviation |
3.8 |
1.7 |
3.5 |
|
Female |
6 |
3 |
6 |
15 |
Male |
1 |
4 |
4 |
9 |
No information provided |
0 |
3 |
0 |
3 |
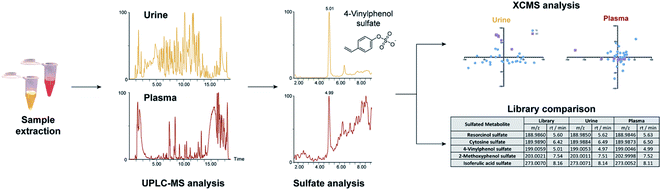 |
| Fig. 1 Method overview for the targeted sulfatome analysis in urine and plasma samples collected from the same individuals. | |
Sulfated metabolite classes
Our analysis identified more sulfated metabolites at the highest two confidence levels (1 + 2) in both sample types (Fig. 2A). In addition, the total number of sulfates detected was 161 in urine samples and 71 in plasma samples. In urine, more than half (91) were identified at either levels 1 or 2 and 70 at levels 3 + 4. On the other hand, in plasma 52 metabolites were detected and their structure determined at level 1 + 2 as well as 19 at level 3 + 4. This result is in accordance with literature, as urine is the major biofluid for the excretion of metabolites including phase II modified metabolites with higher hydrophilicity.22 As an additional explanation, plasma samples represent a snapshot of the subjects' ongoing metabolism, whereas urine contains the accumulated end products after excretion.22 Furthermore, the concentration of the majority of sulfated metabolites is also expected to be lower in plasma.
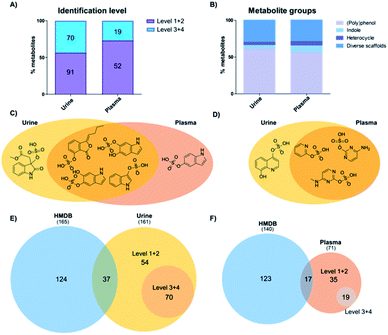 |
| Fig. 2 Identification of sulfated metabolites in human samples. (A) Confidence levels of identified sulfates in urine and plasma. (B) Metabolite classification according to the chemical structure. (C) Indoles identified in urine (yellow) and plasma (red). (D) Diverse scaffold molecules identified in urine and plasma. (E) Comparison between the metabolites identified in HMDB (blue) and urine (yellow) according to level of validation. (F) Comparison between metabolites identified in HMDB (blue) and plasma (red). | |
As most of the sulfated metabolite structures were validated with high structural confidence (levels 1 + 2), we determined the specific metabolite classes that these compounds belong to. The 91 urine metabolites and 52 plasma metabolites were divided into four major metabolite classes: (i) polyphenols; (ii) indoles; (iii) heterocycles; and (iv) diverse scaffolds. The distribution of metabolite classes was similar in both matrices (Fig. 2B). Metabolites classified as (poly)phenols contain one or more phenolic hydroxyl groups in the scaffold. This compound class expectedly made up more than 50% in each sample type as these metabolites are mostly cleared as phase II modifications. Classified as indoles were compounds that contain an indole scaffold. These analogues are mostly derived from microbiome metabolism and were found with equal numbers in both matrices.23,24 A second regioisomer of indoxyl sulfate was detected in plasma samples and methyl dioxindole-3-acetate sulfate was only present in urine (Fig. 2C). The three heterocycle sulfates present in both urine and plasma samples were pyridine-2-yl sulfate, cytosine sulfate and 5-methylcytosine sulfate (Fig. 2D). Quinolone-4,8-diol sulfate was only present in urine samples. We finally categorized a series of metabolites as ‘diverse scaffolds’ that did not fit in any of the other three categories. In this group, almost twice as many metabolites were identified in urine (27) when compared with plasma (15). For example, aminophenol sulfate, tyramine sulfate and tyrosine sulfate were detected in both investigated sample types, while sulfate conjugates of 4-cyanophenyl, N-acetylgalactosamine or nigellic acid were only present in urine samples.
To identify the metabolite coverage of this study, we compared our results with the sulfated metabolites reported in the largest human metabolite database, HMDB.25 This is an important factor as this database is commonly used to match the metabolite hits in global metabolomics analyses. We systematically searched for metabolites in HMDB (urine and plasma) based on one of these partial names: (i) –sulfate suffix; (ii) –sulphate suffix; (iii) sulfooxy prefix. Duplicate metabolites in this database search were removed. Comparison of the total number of metabolites identified in urine samples is similar to the number of metabolites detected in HMDB (161 vs. 165/Fig. 2E). In plasma samples our analysis led to a smaller number of metabolites in comparison with HMDB (71 vs. 140/Fig. 2F). Consideration of metabolites at levels 1 and 2 with complete structural information were compared with metabolites in HMDB. A large number of sulfates described in HMDB were not detected in the investigated samples. Reasons for this difference are that several sulfates described in HMDB are aliphatic or regioisomers of sulfates validated with our library. An additional factor is that most of these metabolites were discovered in targeted analyses from independent studies. In most cases these were focused on drug metabolism and metabolites were thus better detected due to higher concentrations.
The core sulfatome profile
We identified 65 sulfates common in both urine and plasma samples (Fig. 3). Metabolite distribution on an individual level was investigated and metabolites were considered to be present above a threshold of 15
000 ion counts to ensure a sufficient signal-to-noise (S/N) ratio for validation of metabolite peaks. The first interesting observation is the fact that the common sulfates between plasma and urine are present in almost all urine samples at least present more than 80% of individuals. These can be considered the human urine core sulfatome. This is in contrast to plasma samples, where the distribution of sulfates differs individually. We identified 27 metabolites that are present in all subjects and both biological samples. An additional 14 metabolites were detected in both plasma and urine samples in more than 80% of individuals. With this high presence in both main human sample types, we consider these 41 compounds as the human core sulfatome. The other 24 metabolites were mostly present in urine samples but were present in plasma samples on an individual level. Only in rare cases, we observed that a sulfated metabolite was individually present in plasma and not detected in urine. Smaller sulfates such as conjugates of aminophenol, resorcinol, hydroquinone, and 4-vinylphenol were present in all urine samples, but inconsistently distributed in the investigated plasma samples. This was also identified for hippuric acid sulfate as well as for the two regioisomers acetamidophenol and 2-hydroxyacetamidophen. Metabolites with a higher molecular weight were also in general detect in almost all urine samples but individually detected in plasma samples.
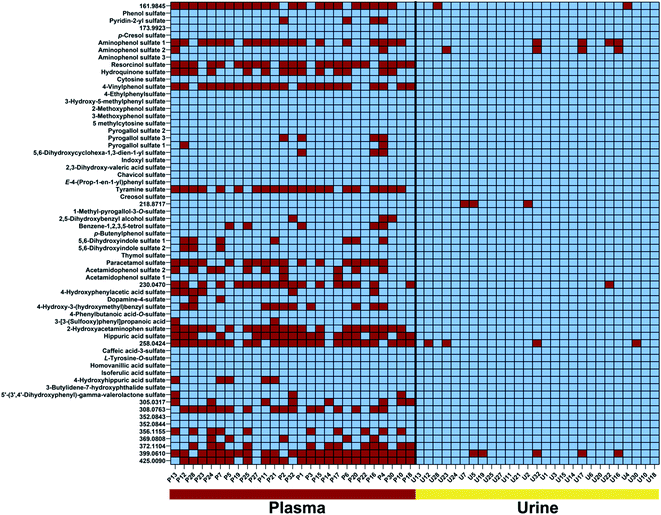 |
| Fig. 3 Colour-coded presentation of 65 sulfated metabolites present (blue) or absent (red) in plasma and urine samples in all individuals. Metabolites are listed according to their molecular weight. High-resolution mass values are provided for sulfated metabolites with an unidentified metabolite structure. | |
Several compounds that are present in all investigated samples of both sample types include phenol sulfate, p-cresol sulfate, isoferulic acid sulfate, and indoxyl sulfate. Interestingly, all four metabolites are known products of host and microbiome co-metabolism.11,12,16,26 These sulfates can thus be considered as universal metabolites for the investigation of the metabolic interplay between host and gut microbes.27 Furthermore, these compounds have also been linked to disease development.28–30
Sulfate distribution – age and biological sex
We next investigated the age and biological sex effect on the sulfatome in these 27 subjects (Table 1). To compare the sulfate distribution and perform a differential analysis, we divided the subjects into three distinct age categories: (i) 38–49 years (group I); (ii) 50–59 years (group II); (iii) 60–75 years (group III). The number of total patients in these three groups is similar with an average age of 54.8 ± 10.0 years. For this analysis, the data was autoscaled in Metaboanalyst.31 The distribution of these subjects across the two main components in the principal component analysis (PCA) for urine (Fig. 4A) and plasma (Fig. 4D) did not yield a clear separation in this unsupervised multivariate analysis across the three age groups. However, some tendencies were common for the plasma and the urine sulfatome. The younger age group clusters closer together than the two other groups. We hypothesize that this could indicate that at a younger age the sulfatome is more stable than at a higher stage in life, while other factors such as the diet or lifestyle of the subjects are unknown. The heatmaps for both sample types with the top 25 sulfated metabolites based on the one-way ANOVA test separates the majority of samples from the oldest age group in urine demonstrated by the highest values for these compounds (Fig. 4B). This clear clustering by one group was not observed in plasma (Fig. 4E). The influence of age on the sulfate content is demonstrated for eight metabolites for urine (Fig. 4C) and plasma (Fig. 4F). The four most altered metabolites based on a one-way ANOVA test for urine sulfates are aminohydroxyphenyl sulfate, pyrogallol sulfate as well as two regioisomers of 5,6-dihydroxyindole sulfate 1 and 2. Significantly increased quantities for these sulfate metabolites were observed in older subjects. The full description of the statistical analysis performed for urine samples is described in Table S2.†
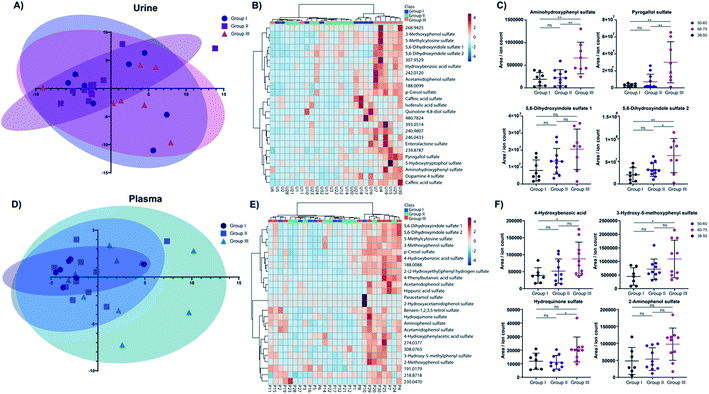 |
| Fig. 4 Age effect of the sulfatome. (A) Principal component analysis for the three age groups I, II and III for urine samples. (B) Heatmap with stratification of the samples for the top 25 sulfated metabolites in urine. (C) Dot plots of mass spectrometric values for the top 4 metabolites altered in urine for each age group. (D) Principal component analysis for the three age groups I, II and III for plasma samples. (E) Heatmap with stratification of the samples for the top 25 sulfated metabolites in plasma. (F) Dot plots of mass spectrometric values for the top 4 metabolites altered in plasma for each age group. (C and F) Student's t-test; p-values: * – >0.05; ** – >0.01; *** – >0.001; ns – not significant. | |
The same tendency was observed for the four plasma metabolites 4-hydroxybenzoic acid sulfate, 3-hydroxy-5-methoxyphenyl sulfate, hydroquinone sulfate, and 2-aminophenol sulfate. The post testing enumerates statistically significant differences between age group I and age group III for 4-hydroxybenzoic acid sulfate and 3-hydroxy-5-methoxyphenyl sulfate (details in Table S3†). Hydroquinone sulfate was altered statistically significantly between groups II and III. 2-Aminophenol sulfate is consistent with the tendency verified for the other three metabolites. However, statistical significance evaluation obtained for these comparisons were slightly above the significance threshold (group I vs. group III – p = 0.0537; group II vs. group III – p = 0.0592).
In total, we identified 12 significantly altered metabolites in urine and 5 metabolites in plasma. The two metabolites p-cresol sulfate and 4-hydroxybenzoic acid sulfate were upregulated in both sample types at a higher age. Further investigation in larger cohorts should be performed to validate these concentration differences for these metabolites with age. Noteworthy, no biological sex differences were observed for sulfated metabolites in either sample type (Fig. S1, Tables S4 and S5†). A Pearson correlation test between age and all metabolite levels was performed to further increase the confidence on the observed alterations for urinary (Table S4†) and plasma samples (Table S5†). All metabolites described in Fig. 4C and E were also significantly positive correlation with age in this statistical analysis.
Our findings of this sulfatome study are consistent with reports on single metabolites described previously. A study by Swann et al. found that the microbial metabolite p-cresol sulfate is increased in older subjects in an American and a Taiwanese population cohort.32 In our study, this molecule was found to also be altered in older subjects in urine. This tendency is also visible for the analysis of p-cresol sulfate in plasma samples (one-way ANOVA p-value = 0.0492). In another example, concentrations of the unconjugated metabolite 4-hydroxybenzoic acid were described to be inversely correlated with age.33 We identified that hydroxybenzoic acid sulfate was increased with age, which is surprising but concentrations of the sulfated conjugate can differ compared to their aglycon.34 While phenol sulfate was increased at an older age in a Japanese male cohort study, we determined unaltered levels of this metabolite.35 This inconsistent observation can be a result of many factors including dietary preferences, genetic or metabolic differences.16 In a separate study, the two metabolites indoxyl sulfate and p-cresol sulfate were increased in subjects older than 65.36 Our data analysis also identified several sulfated indole-containing metabolites that were age-dependently altered in both sample types.
Conclusions
In this study, we report the individual sulfated metabolome for 27 volunteers in plasma and urine samples. Our findings from the mass spectrometric analysis reveal clear differences in the sulfated metabolite content in urine and plasma samples. Furthermore, we provide the first profile of the human core sulfatome, composed of 41 metabolites that are present in both sample types that also include microbiome-derived metabolites. In this cohort, we also identified an age-effect for 12 compounds in urine and 5 in plasma, while we did not observe any significantly altered biological sex differences. This core sulfatome can be used for monitoring physiological changes, dietary intervention as well as the co-metabolism of the microbiome with humans.
Author contributions
D. G. conceived and designed the study. M. S. P. C. designed experiments. M. S. P. C. and B. T. performed experiments and mass spectrometric experiments. M. S. P. C. performed bioinformatic data analysis. D. G. acquired funding and supervised the project, M. V. and J.-M. L. were responsible for ethical approval, human patient sample collection and selection, M. S. P. C. and D. G. wrote the manuscript with contributions from B. T., M. V. and J.-M. L.
Conflicts of interest
There are no conflicts of interest to declare.
Acknowledgements
We are grateful for funding by the Swedish Research Council (VR 2016-04423/VR 2020-04707), the Swedish Cancer Foundation (19 0347 Pj), and a generous start-up grant from Science for Life Laboratory to D. G.
References
- P. Anzenbacher and E. Anzenbacherová, Cell. Mol. Life Sci., 2001, 58, 737–747 CrossRef CAS PubMed.
- S. Crettol, N. Petrovic and M. Murray, Curr. Pharm. Des., 2010, 16, 204–219 CrossRef CAS PubMed.
- C. Xu, C.-T. Li and A.-N. Kong, Arch. Pharmacal Res., 2005, 28, 249–268 CrossRef CAS PubMed.
- I. Meister, P. Zhang, A. Sinha, C. M. Skold, A. M. Wheelock, T. Izumi, R. Chaleckis and C. E. Wheelock, Anal. Chem., 2021, 93, 5248–5258 CrossRef CAS PubMed.
- D. Globisch, A. Y. Moreno, M. S. Hixon, A. A. K. Nunes, J. R. Denery, S. Specht, A. Hoerauf and K. D. Janda, Proc. Natl. Acad. Sci. U. S. A., 2013, 110, 4218–4223 CrossRef CAS PubMed.
- M. Flasch, C. Bueschl, L. Woelflingseder, H. E. Schwartz-Zimmermann, G. Adam, R. Schuhmacher, D. Marko and B. Warth, ACS Chem. Biol., 2020, 15, 970–981 CrossRef CAS PubMed.
- M. Thevis and W. Schanzer, Mol. Cell. Endocrinol., 2018, 464, 34–45 CrossRef CAS PubMed.
- M. S. P. Correia, M. Rao, C. Ballet and D. Globisch, ChemBioChem, 2019, 20, 1678–1683 CrossRef CAS PubMed.
- K. Levsen, H. M. Schiebel, B. Behnke, R. Dotzer, W. Dreher, M. Elend and H. Thiele, J. Chromatogr. A, 2005, 1067, 55–72 CrossRef CAS PubMed.
- L. P. Conway, V. Rendo, M. S. P. Correia, I. A. Bergdahl, T. Sjoblom and D. Globisch, Angew. Chem., Int. Ed., 2020, 59, 14342–14346 CrossRef CAS PubMed.
- C. Ballet, M. S. P. Correia, L. P. Conway, T. L. Locher, L. C. Lehmann, N. Garg, M. Vujasinovic, S. Deindl, J. M. Lohr and D. Globisch, Chem. Sci., 2018, 9, 6233–6239 RSC.
- W. R. Wikoff, A. T. Anfora, J. Liu, P. G. Schultz, S. A. Lesley, E. C. Peters and G. Siuzdak, Proc. Natl. Acad. Sci. U. S. A., 2009, 106, 3698–3703 CrossRef CAS PubMed.
- K. Kikuchi, D. Saigusa, Y. Kanemitsu, Y. Matsumoto, P. Thanai, N. Suzuki, K. Mise, H. Yamaguchi, T. Nakamura, K. Asaji, C. Mukawa, H. Tsukamoto, T. Sato, Y. Oikawa, T. Iwasaki, Y. Oe, T. Tsukimi, N. N. Fukuda, H. J. Ho, F. Nanto-Hara, J. Ogura, R. Saito, S. Nagao, Y. Ohsaki, S. Shimada, T. Suzuki, T. Toyohara, E. Mishima, H. Shima, Y. Akiyama, Y. Akiyama, M. Ichijo, T. Matsuhashi, A. Matsuo, Y. Ogata, C. C. Yang, C. Suzuki, M. C. Breeggemann, J. Heymann, M. Shimizu, S. Ogawa, N. Takahashi, T. Suzuki, Y. Owada, S. Kure, N. Mano, T. Soga, T. Wada, J. B. Kopp, S. Fukuda, A. Hozawa, M. Yamamoto, S. Ito, J. Wada, Y. Tomioka and T. Abe, Nat. Commun., 2019, 10, 1835 CrossRef PubMed.
- N. Garg, A. Hansson, H. K. Knych, S. D. Stanley, M. Thevis, U. Bondesson, M. Hedeland and D. Globisch, Org. Biomol. Chem., 2018, 16, 698–702 RSC.
- J. D. Mougous, M. D. Leavell, R. H. Senaratne, C. D. Leigh, S. J. Williams, L. W. Riley, J. A. Leary and C. R. Bertozzi, Proc. Natl. Acad. Sci. U. S. A., 2002, 99, 17037–17042 CrossRef CAS PubMed.
- M. S. P. Correia, A. Jain, W. Alotaibi, P. Young Tie Yang, A. Rodriguez-Mateos and D. Globisch, Free Radical Biol. Med., 2020, 160, 745–754 CrossRef CAS PubMed.
- E. L. Schymanski, J. Jeon, R. Gulde, K. Fenner, M. Ruff, H. P. Singer and J. Hollender, Environ. Sci. Technol., 2014, 48, 2097–2098 CrossRef CAS PubMed.
- M. S. P. Correia, W. Lin, A. J. Aria, A. Jain and D. Globisch, Metabolites, 2020, 10, 415 CrossRef CAS PubMed.
- A. Jain, M. S. P. Correia, H. Meistermann, M. Vujasinovic, J. M. Lohr and D. Globisch, J. Pharm. Biomed. Anal., 2021, 195, 113818 CrossRef CAS PubMed.
- H. Gowda, J. Ivanisevic, C. H. Johnson, M. E. Kurczy, H. P. Benton, D. Rinehart, T. Nguyen, J. Ray, J. Kuehl, B. Arevalo, P. D. Westenskow, J. Wang, A. P. Arkin, A. M. Deutschbauer, G. J. Patti and G. Siuzdak, Anal. Chem., 2014, 86, 6931–6939 CrossRef CAS PubMed.
- J. Ivanisevic, H. P. Benton, D. Rinehart, A. Epstein, M. E. Kurczy, M. D. Boska, H. E. Gendelman and G. Siuzdak, Metabolomics, 2015, 11, 1029–1034 CrossRef CAS PubMed.
- V. L. Stevens, E. Hoover, Y. Wang and K. A. Zanetti, Metabolites, 2019, 9, 156 CrossRef CAS PubMed.
- T. Pavlova, V. Vidova, J. Bienertova-Vasku, P. Janku, M. Almasi, J. Klanova and Z. Spacil, Anal. Chim. Acta, 2017, 987, 72–80 CrossRef CAS PubMed.
- A. Kumar and V. Sperandio, mBio, 2019, 10, e01031-19 CrossRef PubMed.
- D. S. Wishart, Y. D. Feunang, A. Marcu, A. C. Guo, K. Liang, R. Vázquez-Fresno, T. Sajed, D. Johnson, C. Li, N. Karu, Z. Sayeeda, E. Lo, N. Assempour, M. Berjanskii, S. Singhal, D. Arndt, Y. Liang, H. Badran, J. Grant, A. Serra-Cayuela, Y. Liu, R. Mandal, V. Neveu, A. Pon, C. Knox, M. Wilson, C. Manach and A. Scalbert, Nucleic Acids Res., 2018, 46, D608–D617 CrossRef CAS PubMed.
- T. Huc, A. Nowinski, A. Drapala, P. Konopelski and M. Ufnal, Pharmacol. Res., 2018, 130, 172–179 CrossRef CAS PubMed.
- J. A. Rothwell, J. Perez-Jimenez, V. Neveu, A. Medina-Remón, N. M’Hiri, P. García-Lobato, C. Manach, C. Knox, R. Eisner, D. S. Wishart and A. Scalbert, Database, 2013, 2013, bat070 CrossRef PubMed.
- R. Vanholder, E. Schepers, A. Pletinck, E. V. Nagler and G. Glorieux, J. Am. Soc. Nephrol., 2014, 25, 1897–1907 CrossRef CAS PubMed.
- S. C. Hung, K. L. Kuo, C. C. Wu and D. C. Tarng, J. Am. Heart Assoc., 2017, 6, e005022 Search PubMed.
- E. Van Rymenant, J. Van Camp, B. Pauwels, C. Boydens, L. Vanden Daele, K. Beerens, P. Brouckaert, G. Smagghe, A. Kerimi, G. Williamson, C. Grootaert and J. Van de Voorde, J. Nutr. Biochem., 2017, 44, 44–51 CrossRef CAS PubMed.
- J. Chong, D. S. Wishart and J. Xia, Curr. Protoc. Bioinf., 2019, 68, e86 Search PubMed.
- J. R. Swann, K. Spagou, M. Lewis, J. K. Nicholson, D. A. Glei, T. E. Seeman, C. L. Coe, N. Goldman, C. D. Ryff, M. Weinstein and E. Holmes, J. Proteome Res., 2013, 12, 3166–3180 CrossRef CAS PubMed.
- E. A. Thévenot, A. Roux, Y. Xu, E. Ezan and C. Junot, J. Proteome Res., 2015, 14, 3322–3335 CrossRef PubMed.
- K. D. R. Setchell, N. M. Brown, X. Zhao, S. L. Lindley, J. E. Heubi, E. C. King and M. J. Messina, Am. J. Clin. Nutr., 2011, 94, 1284–1294 CrossRef CAS PubMed.
- K. Saito, K. Maekawa, J. M. Kinchen, R. Tanaka, Y. Kumagai and Y. Saito, Biol. Pharm. Bull., 2016, 39, 1179–1186 CrossRef CAS PubMed.
- A. Wyczalkowska-Tomasik, B. Czarkowska-Paczek, J. Giebultowicz, P. Wroczynski and L. Paczek, Geriatr. Gerontol. Int., 2017, 17, 1022–1026 CrossRef PubMed.
Footnote |
† Electronic supplementary information (ESI) available. See DOI: 10.1039/d1ra05994g |
|
This journal is © The Royal Society of Chemistry 2021 |
Click here to see how this site uses Cookies. View our privacy policy here.